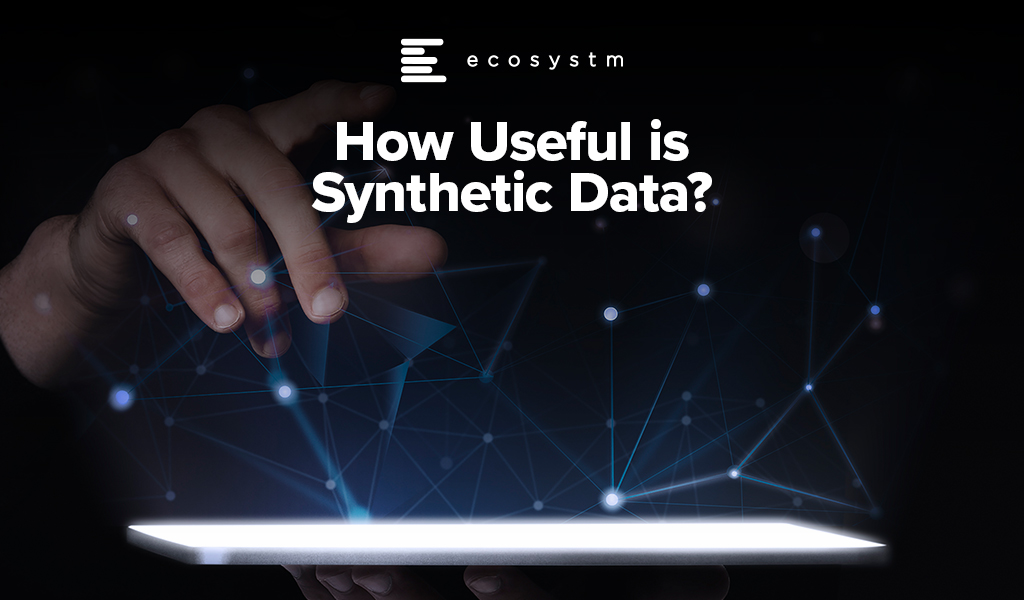
When non-organic (man-made) fabric was introduced into fashion, there were a number of harsh warnings about using polyester and man-made synthetic fibres, including their flammability.
In creating non-organic data sets, should we also be creating warnings on their use and flammability? Let’s look at why synthetic data is used in industries such as Financial Services, Automotive as well as for new product development in Manufacturing.
Synthetic Data Defined
Synthetic data can be defined as data that is artificially developed rather than being generated by actual interactions. It is often created with the help of algorithms and is used for a wide range of activities, including as test data for new products and tools, for model validation, and in AI model training. Synthetic data is a type of data augmentation which involves creating new and representative data.
Why is it used?
The main reasons why synthetic data is used instead of real data are cost, privacy, and testing. Let’s look at more specifics on this:
- Data privacy. When privacy requirements limit data availability or how it can be used. For example, in Financial Services where restrictions around data usage and customer privacy are particularly limiting, companies are starting to use synthetic data to help them identify and eliminate bias in how they treat customers – without contravening data privacy regulations.
- Data availability. When the data needed for testing a product does not exist or is not available to the testers. This is often the case for new releases.
- Data for testing. When training data is needed for machine learning algorithms. However, in many instances, such as in the case of autonomous vehicles, the data is expensive to generate in real life.
- Training across third parties using cloud. When moving private data to cloud infrastructures involves security and compliance risks. Moving synthetic versions of sensitive data to the cloud can enable organisations to share data sets with third parties for training across cloud infrastructures.
- Data cost. Producing synthetic data through a generative model is significantly more cost-effective and efficient than collecting real-world data. With synthetic data, it becomes cheaper and faster to produce new data once the generative model is set up.
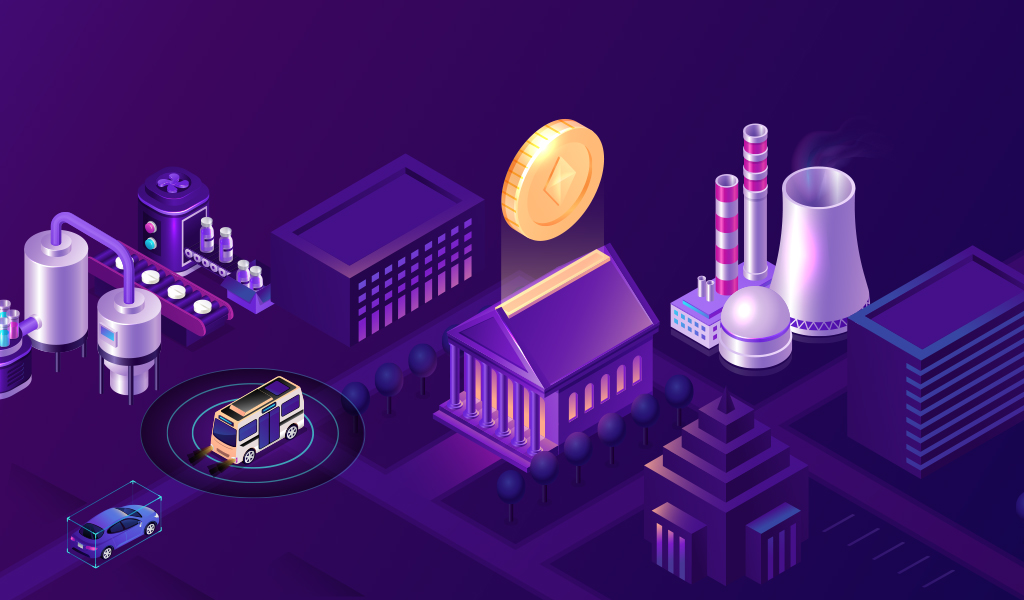
Why should it cause concern?
If real dataset contains biases, data augmented from it will contain biases, too. So, identification of optimal data augmentation strategy is important.
If the synthetic set doesn’t truly represent the original customer data set, it might contain the wrong buying signals regarding what customers are interested in or are inclined to buy.
Synthetic data also requires some form of output/quality control and internal regulation, specifically in highly regulated industries such as the Financial Services.
Creating incorrect synthetic data also can get a company in hot water with external regulators. For example, if a company created a product that harmed someone or didn’t work as advertised, it could lead to substantial financial penalties and, possibly, closer scrutiny in the future.
Conclusion
Synthetic data allows us to continue developing new and innovative products and solutions when the data necessary to do so wouldn’t otherwise be present or available due to volume, data sensitivity or user privacy challenges. Generating synthetic data comes with the flexibility to adjust its nature and environment as and when required in order to improve the performance of the model to create opportunities to check for outliers and extreme conditions.