Historically, data scientists have been the linchpins in the world of AI and machine learning, responsible for everything from data collection and curation to model training and validation. However, as the field matures, we’re witnessing a significant shift towards specialisation, particularly in data engineering and the strategic role of Large Language Models (LLMs) in data curation and labelling. The integration of AI into applications is also reshaping the landscape of software development and application design.
The Growth of Embedded AI
AI is being embedded into applications to enhance user experience, optimise operations, and provide insights that were previously inaccessible. For example, natural language processing (NLP) models are being used to power conversational chatbots for customer service, while machine learning algorithms are analysing user behaviour to customise content feeds on social media platforms. These applications leverage AI to perform complex tasks, such as understanding user intent, predicting future actions, or automating decision-making processes, making AI integration a critical component of modern software development.
This shift towards AI-embedded applications is not only changing the nature of the products and services offered but is also transforming the roles of those who build them. Since the traditional developer may not possess extensive AI skills, the role of data scientists is evolving, moving away from data engineering tasks and increasingly towards direct involvement in development processes.
The Role of LLMs in Data Curation
The emergence of LLMs has introduced a novel approach to handling data curation and processing tasks traditionally performed by data scientists. LLMs, with their profound understanding of natural language and ability to generate human-like text, are increasingly being used to automate aspects of data labelling and curation. This not only speeds up the process but also allows data scientists to focus more on strategic tasks such as model architecture design and hyperparameter tuning.
The accuracy of AI models is directly tied to the quality of the data they’re trained on. Incorrectly labelled data or poorly curated datasets can lead to biased outcomes, mispredictions, and ultimately, the failure of AI projects. The role of data engineers and the use of advanced tools like LLMs in ensuring the integrity of data cannot be overstated.
The Impact on Traditional Developers
Traditional software developers have primarily focused on writing code, debugging, and software maintenance, with a clear emphasis on programming languages, algorithms, and software architecture. However, as applications become more AI-driven, there is a growing need for developers to understand and integrate AI models and algorithms into their applications. This requirement presents a challenge for developers who may not have specialised training in AI or data science. This is seeing an increasing demand for upskilling and cross-disciplinary collaboration to bridge the gap between traditional software development and AI integration.
Clear Role Differentiation: Data Engineering and Data Science
In response to this shift, the role of data scientists is expanding beyond the confines of traditional data engineering and data science, to include more direct involvement in the development of applications and the embedding of AI features and functions.
Data engineering has always been a foundational element of the data scientist’s role, and its importance has increased with the surge in data volume, variety, and velocity. Integrating LLMs into the data collection process represents a cutting-edge approach to automating the curation and labelling of data, streamlining the data management process, and significantly enhancing the efficiency of data utilisation for AI and ML projects.
Accurate data labelling and meticulous curation are paramount to developing models that are both reliable and unbiased. Errors in data labelling or poorly curated datasets can lead to models that make inaccurate predictions or, worse, perpetuate biases. The integration of LLMs into data engineering tasks is facilitating a transformation, freeing them from the burdens of manual data labelling and curation. This has led to a more specialised data scientist role that allocates more time and resources to areas that can create greater impact.
The Evolving Role of Data Scientists
Data scientists, with their deep understanding of AI models and algorithms, are increasingly working alongside developers to embed AI capabilities into applications. This collaboration is essential for ensuring that AI models are effectively integrated, optimised for performance, and aligned with the application’s objectives.
- Model Development and Innovation. With the groundwork of data preparation laid by LLMs, data scientists can focus on developing more sophisticated and accurate AI models, exploring new algorithms, and innovating in AI and ML technologies.
- Strategic Insights and Decision Making. Data scientists can spend more time analysing data and extracting valuable insights that can inform business strategies and decision-making processes.
- Cross-disciplinary Collaboration. This shift also enables data scientists to engage more deeply in interdisciplinary collaboration, working closely with other departments to ensure that AI and ML technologies are effectively integrated into broader business processes and objectives.
- AI Feature Design. Data scientists are playing a crucial role in designing AI-driven features of applications, ensuring that the use of AI adds tangible value to the user experience.
- Model Integration and Optimisation. Data scientists are also involved in integrating AI models into the application architecture, optimising them for efficiency and scalability, and ensuring that they perform effectively in production environments.
- Monitoring and Iteration. Once AI models are deployed, data scientists work on monitoring their performance, interpreting outcomes, and making necessary adjustments. This iterative process ensures that AI functionalities continue to meet user needs and adapt to changing data landscapes.
- Research and Continued Learning. Finally, the transformation allows data scientists to dedicate more time to research and continued learning, staying ahead of the rapidly evolving field of AI and ensuring that their skills and knowledge remain cutting-edge.
Conclusion
The integration of AI into applications is leading to a transformation in the roles within the software development ecosystem. As applications become increasingly AI-driven, the distinction between software development and AI model development is blurring. This convergence needs a more collaborative approach, where traditional developers gain AI literacy and data scientists take on more active roles in application development. The evolution of these roles highlights the interdisciplinary nature of building modern AI-embedded applications and underscores the importance of continuous learning and adaptation in the rapidly advancing field of AI.
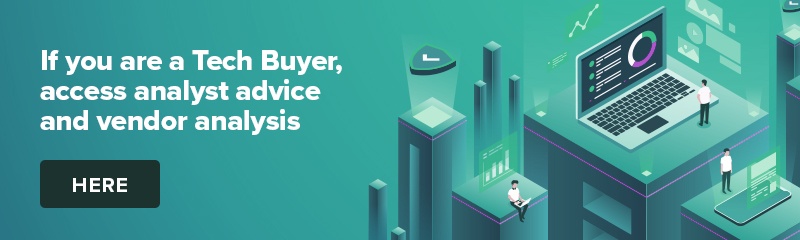
In the Ecosystm Predicts: Building an Agile & Resilient Organisation: Top 5 Trends in 2024, Principal Advisor Darian Bird said, “The emergence of Generative AI combined with the maturing of deepfake technology will make it possible for malicious agents to create personalised voice and video attacks.” Darian highlighted that this democratisation of phishing, facilitated by professional-sounding prose in various languages and tones, poses a significant threat to potential victims who rely on misspellings or oddly worded appeals to detect fraud. As we see more of these attacks and social engineering attempts, it is important to improve defence mechanisms and increase awareness.
Understanding Deepfake Technology
The term Deepfake is a combination of the words ‘deep learning’ and ‘fake’. Deepfakes are AI-generated media, typically in the form of images, videos, or audio recordings. These synthetic content pieces are designed to appear genuine, often leading to the manipulation of faces and voices in a highly realistic manner. Deepfake technology has gained spotlight due to its potential for creating convincing yet fraudulent content that blurs the line of reality.
Deepfake algorithms are powered by Generative Adversarial Networks (GANs) and continuously enhance synthetic content to closely resemble real data. Through iterative training on extensive datasets, these algorithms refine features such as facial expressions and voice inflections, ensuring a seamless emulation of authentic characteristics.
Deepfakes Becoming Increasingly Convincing
Hyper-realistic deepfakes, undetectable to the human eye and ear, have become a huge threat to the financial and technology sectors. Deepfake technology has become highly convincing, blurring the line between real and fake content. One of the early examples of a successful deepfake fraud was when a UK-based energy company lost USD 243k through a deepfake audio scam in 2019, where scammers mimicked the voice of their CEO to authorise an illegal fund transfer.
Deepfakes have evolved from audio simulations to highly convincing video manipulations where faces and expressions are altered in real-time, making it hard to distinguish between real and fake content. In 2022, for instance, a deepfake video of Elon Musk was used in a crypto scam that resulted in a loss of about USD 2 million for US consumers. This year, a multinational company in Hong Kong lost over USD 25 million when an employee was tricked into sending money to fraudulent accounts after a deepfake video call by what appeared to be his colleagues.
Regulatory Responses to Deepfakes
Countries worldwide are responding to the challenges posed by deepfake technology through regulations and awareness campaigns.
- Singapore’s Online Criminal Harms Act, that will come into effect in 2024, will empower authorities to order individuals and Internet service providers to remove or block criminal content, including deepfakes used for malicious purposes.
- The UAE National Programme for Artificial Intelligence released a deepfake guide to educate the public about both harmful and beneficial applications of this technology. The guide categorises fake content into shallow and deep fakes, providing methods to detect deepfakes using AI-based tools, with a focus on promoting positive uses of advanced technologies.
- The proposed EU AI Act aims to regulate them by imposing transparency requirements on creators, mandating them to disclose when content has been artificially generated or manipulated.
- South Korea passed a law in 2020 banning the distribution of harmful deepfakes. Offenders could be sentenced to up to five years in prison or fined up to USD 43k.
- In the US, states like California and Virginia have passed laws against deepfake pornography, while federal bills like the DEEP FAKES Accountability Act aim to mandate disclosure and counter malicious use, highlighting the diverse global efforts to address the multifaceted challenges of deepfake regulation.
Detecting and Protecting Against Deepfakes
Detecting deepfake becomes increasingly challenging as technology advances. Several methods are needed – sometimes in conjunction – to be able to detect a convincing deepfake. These include visual inspection that focuses on anomalies, metadata analysis to examine clues about authenticity, forensic analysis for pattern and audio examination, and machine learning that uses algorithms trained on real and fake video datasets to classify new videos.
However, identifying deepfakes requires sophisticated technology that many organisations may not have access to. This heightens the need for robust cybersecurity measures. Deepfakes have seen an increase in convincing and successful phishing – and spear phishing – attacks and cyber leaders need to double down on cyber practices.
Defences can no longer depend on spotting these attacks. It requires a multi-pronged approach which combines cyber technologies, incidence response, and user education.
Preventing access to users. By employing anti-spoofing measures organisations can safeguard their email addresses from exploitation by fraudulent actors. Simultaneously, minimising access to readily available information, particularly on websites and social media, reduces the chance of spear-phishing attempts. This includes educating employees about the implications of sharing personal information and clear digital footprint policies. Implementing email filtering mechanisms, whether at the server or device level, helps intercept suspicious emails; and the filtering rules need to be constantly evaluated using techniques such as IP filtering and attachment analysis.
Employee awareness and reporting. There are many ways that organisations can increase awareness in employees starting from regular training sessions to attack simulations. The usefulness of these sessions is often questioned as sometimes they are merely aimed at ticking off a compliance box. Security leaders should aim to make it easier for employees to recognise these attacks by familiarising them with standard processes and implementing verification measures for important email requests. This should be strengthened by a culture of reporting without any individual blame.
Securing against malware. Malware is often distributed through these attacks, making it crucial to ensure devices are well-configured and equipped with effective endpoint defences to prevent malware installation, even if users inadvertently click on suspicious links. Specific defences may include disabling macros and limiting administrator privileges to prevent accidental malware installation. Strengthening authentication and authorisation processes is also important, with measures such as multi-factor authentication, password managers, and alternative authentication methods like biometrics or smart cards. Zero trust and least privilege policies help protect organisation data and assets.
Detection and Response. A robust security logging system is crucial, either through off-the shelf monitoring tools, managed services, or dedicated teams for monitoring. What is more important is that the monitoring capabilities are regularly updated. Additionally, having a well-defined incident response can swiftly mitigate post-incident harm post-incident. This requires clear procedures for various incident types and designated personnel for executing them, such as initiating password resets or removing malware. Organisations should ensure that users are informed about reporting procedures, considering potential communication challenges in the event of device compromise.
Conclusion
The rise of deepfakes has brought forward the need for a collaborative approach. Policymakers, technology companies, and the public must work together to address the challenges posed by deepfakes. This collaboration is crucial for making better detection technologies, establishing stronger laws, and raising awareness on media literacy.
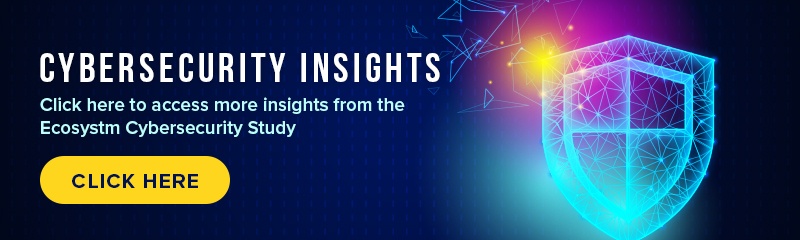
2024 will be another crucial year for tech leaders – through the continuing economic uncertainties, they will have to embrace transformative technologies and keep an eye on market disruptors such as infrastructure providers and AI startups. Ecosystm analysts outline the key considerations for leaders shaping their organisations’ tech landscape in 2024.
Navigating Market Dynamics

Continuing Economic Uncertainties. Organisations will focus on ongoing projects and consider expanding initiatives in the latter part of the year.
Popularity of Generative AI. This will be the time to go beyond the novelty factor and assess practical business outcomes, allied costs, and change management.
Infrastructure Market Disruption. Keeping an eye out for advancements and disruptions in the market (likely to originate from the semiconductor sector) will define vendor conversations.
Need for New Tech Skills. Generative AI will influence multiple tech roles, including AIOps and IT Architecture. Retaining talent will depend on upskilling and reskilling.
Increased Focus on Governance. Tech vendors are guide tech leaders on how to implement safeguards for data usage, sharing, and cybersecurity.
5 Key Considerations for Tech Leaders
#1 Accelerate and Adapt: Streamline IT with a DevOps Culture
Over the next 12-18 months, advancements in AI, machine learning, automation, and cloud-native technologies will be vital in leveraging scalability and efficiency. Modernisation is imperative to boost responsiveness, efficiency, and competitiveness in today’s dynamic business landscape.
The continued pace of disruption demands that organisations modernise their applications portfolios with agility and purpose. Legacy systems constrained by technical debt drag down velocity, impairing the ability to deliver new innovative offerings and experiences customers have grown to expect.
Prioritising modernisation initiatives that align with key value drivers is critical. Technology leaders should empower development teams to move beyond outdated constraints and swiftly deploy enhanced applications, microservices, and platforms.

#2 Empowering Tomorrow: Spring Clean Your Tech Legacy for New Leaders
Modernising legacy systems is a strategic and inter-generational shift that goes beyond simple technical upgrades. It requires transformation through the process of decomposing and replatforming systems – developed by previous generations – into contemporary services and signifies a fundamental realignment of your business with the evolving digital landscape of the 21st century.
The essence of this modernisation effort is multifaceted. It not only facilitates the integration of advanced technologies but also significantly enhances business agility and drives innovation. It is an approach that prepares your organisation for impending skill gaps, particularly as the older workforce begins to retire over the next decade. Additionally, it provides a valuable opportunity to thoroughly document, reevaluate, and improve business processes. This ensures that operations are not only efficient but also aligned with current market demands, contemporary regulatory standards, and the changing expectations of customers.

#3 Employee Retention: Consider the Strategic Role of Skills Acquisition
The agile, resilient organisation needs to be able to respond at pace to any threat or opportunity it faces. Some of this ability to respond will be related to technology platforms and architectures, but it will be the skills of employees that will dictate the pace of reform. While employee attrition rates will continue to decline in 2024 – but it will be driven by skills acquisition, not location of work.
Organisations who offer ongoing staff training – recognising that their business needs new skills to become a 21st century organisation – are the ones who will see increasing rates of employee retention and happier employees. They will also be the ones who offer better customer experiences, driven by motivated employees who are committed to their personal success, knowing that the organisation values their performance and achievements.

#4 Next-Gen IT Operations: Explore Gen AI for Incident Avoidance and Predictive Analysis
The integration of Generative AI in IT Operations signifies a transformative shift from the automation of basic tasks, to advanced functions like incident avoidance and predictive analysis. Initially automating routine tasks, Generative AI has evolved to proactively avoiding incidents by analysing historical data and current metrics. This shift from proactive to reactive management will be crucial for maintaining uninterrupted business operations and enhancing application reliability.
Predictive analysis provides insight into system performance and user interaction patterns, empowering IT teams to optimise applications pre-emptively, enhancing efficiency and user experience. This also helps organisations meet sustainability goals through accurate capacity planning and resource allocation, also ensuring effective scaling of business applications to meet demands.

#5 Expanding Possibilities: Incorporate AI Startups into Your Portfolio
While many of the AI startups have been around for over five years, this will be the year they come into your consciousness and emerge as legitimate solutions providers to your organisation. And it comes at a difficult time for you!
Most tech leaders are looking to reduce technical debt – looking to consolidate their suppliers and simplify their tech architecture. Considering AI startups will mean a shift back to more rather than fewer tech suppliers; a different sourcing strategy; more focus on integration and ongoing management of the solutions; and a more complex tech architecture.
To meet business requirements will mean that business cases will need to be watertight – often the value will need to be delivered before a contract has been signed.

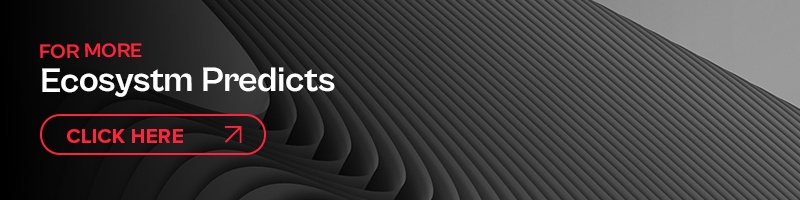
It’s been barely one year since we entered the Generative AI Age. On November 30, 2022, OpenAI launched ChatGPT, with no fanfare or promotion. Since then, Generative AI has become arguably the most talked-about tech topic, both in terms of opportunities it may bring and risks that it may carry.
The landslide success of ChatGPT and other Generative AI applications with consumers and businesses has put a renewed and strengthened focus on the potential risks associated with the technology – and how best to regulate and manage these. Government bodies and agencies have created voluntary guidelines for the use of AI for a number of years now (the Singapore Framework, for example, was launched in 2019).
There is no active legislation on the development and use of AI yet. Crucially, however, a number of such initiatives are currently on their way through legislative processes globally.
EU’s Landmark AI Act: A Step Towards Global AI Regulation
The European Union’s “Artificial Intelligence Act” is a leading example. The European Commission (EC) started examining AI legislation in 2020 with a focus on
- Protecting consumers
- Safeguarding fundamental rights, and
- Avoiding unlawful discrimination or bias
The EC published an initial legislative proposal in 2021, and the European Parliament adopted a revised version as their official position on AI in June 2023, moving the legislation process to its final phase.
This proposed EU AI Act takes a risk management approach to regulating AI. Organisations looking to employ AI must take note: an internal risk management approach to deploying AI would essentially be mandated by the Act. It is likely that other legislative initiatives will follow a similar approach, making the AI Act a potential role model for global legislations (following the trail blazed by the General Data Protection Regulation). The “G7 Hiroshima AI Process”, established at the G7 summit in Japan in May 2023, is a key example of international discussion and collaboration on the topic (with a focus on Generative AI).
Risk Classification and Regulations in the EU AI Act
At the heart of the AI Act is a system to assess the risk level of AI technology, classify the technology (or its use case), and prescribe appropriate regulations to each risk class.
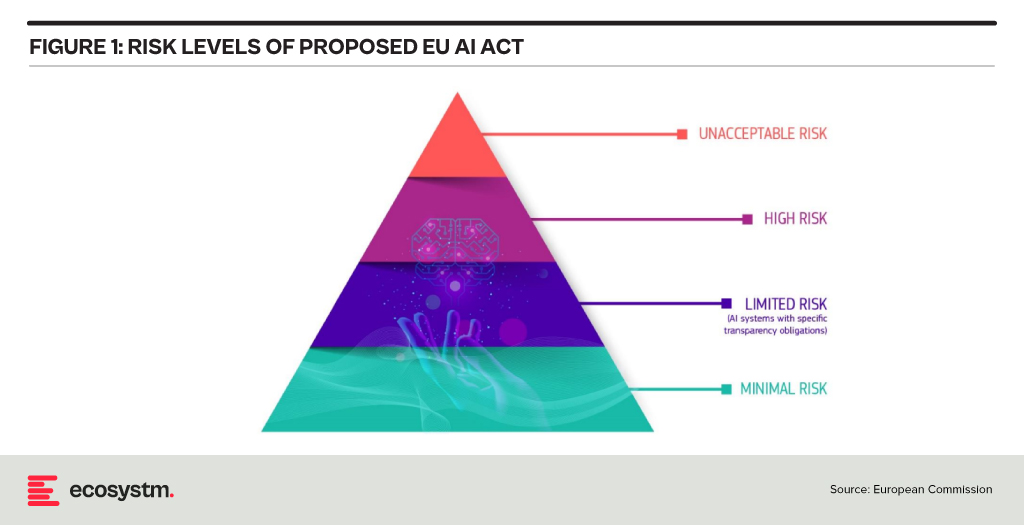
For each of these four risk levels, the AI Act proposes a set of rules and regulations. Evidently, the regulatory focus is on High-Risk AI systems.
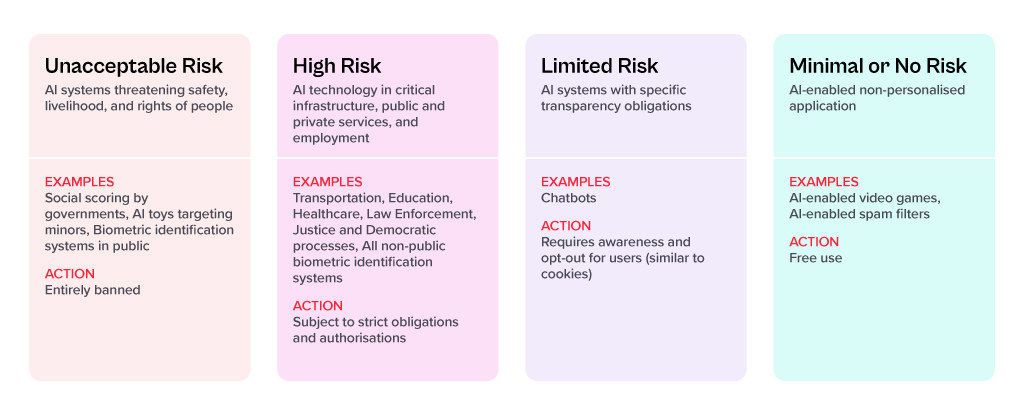
Contrasting Approaches: EU AI Act vs. UK’s Pro-Innovation Regulatory Approach
The AI Act has received its share of criticism, and somewhat different approaches are being considered, notably in the UK. One set of criticism revolves around the lack of clarity and vagueness of concepts (particularly around person-related data and systems). Another set of criticism revolves around the strong focus on the protection of rights and individuals and highlights the potential negative economic impact for EU organisations looking to leverage AI, and for EU tech companies developing AI systems.
A white paper by the UK government published in March 2023, perhaps tellingly, named “A pro-innovation approach to AI regulation” emphasises on a “pragmatic, proportionate regulatory approach … to provide a clear, pro-innovation regulatory environment”, The paper talks about an approach aiming to balance the protection of individuals with economic advancements for the UK on its way to become an “AI superpower”.
Further aspects of the EU AI Act are currently being critically discussed. For example, the current text exempts all open-source AI components not part of a medium or higher risk system from regulation but lacks definition and considerations for proliferation.
Adopting AI Risk Management in Organisations: The Singapore Approach
Regardless of how exactly AI regulations will turn out around the world, organisations must start today to adopt AI risk management practices. There is an added complexity: while the EU AI Act does clearly identify high-risk AI systems and example use cases, the realisation of regulatory practices must be tackled with an industry-focused approach.
The approach taken by the Monetary Authority of Singapore (MAS) is a primary example of an industry-focused approach to AI risk management. The Veritas Consortium, led by MAS, is a public-private-tech partnership consortium aiming to guide the financial services sector on the responsible use of AI. As there is no AI legislation in Singapore to date, the consortium currently builds on Singapore’s aforementioned “Model Artificial Intelligence Governance Framework”. Additional initiatives are already underway to focus specifically on Generative AI for financial services, and to build a globally aligned framework.
To Comply with Upcoming AI Regulations, Risk Management is the Path Forward
As AI regulation initiatives move from voluntary recommendation to legislation globally, a risk management approach is at the core of all of them. Adding risk management capabilities for AI is the path forward for organisations looking to deploy AI-enhanced solutions and applications. As that task can be daunting, an industry consortium approach can help circumnavigate challenges and align on implementation and realisation strategies for AI risk management across the industry. Until AI legislations are in place, such industry consortia can chart the way for their industry – organisations should seek to participate now to gain a head start with AI.
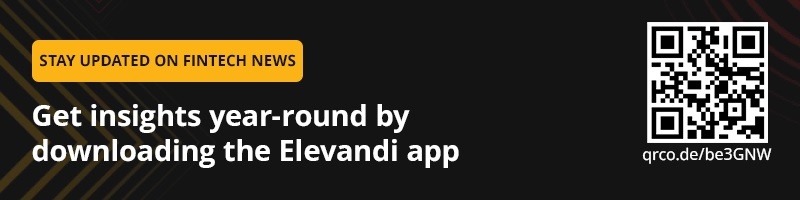
Traditional network architectures are inherently fragile, often relying on a single transport type to connect branches, production facilities, and data centres. The imperative for networks to maintain resilience has grown significantly, particularly due to the delivery of customer-facing services at branches and the increasing reliance on interconnected machines in operational environments. The cost of network downtime can now be quantified in terms of both lost customers and reduced production.
Distributed Enterprises Face New Challenges
As the importance of maintaining resiliency grows, so does the complexity of network management. Distributed enterprises must provide connectivity under challenging conditions, such as:
- Remote access for employees using video conferencing
- Local breakout for cloud services to avoid backhauling
- IoT devices left unattended in public places
- Customers accessing digital services at the branch or home
- Sites in remote areas requiring the same quality of service
Network managers require intelligent tools to remain in control without adding any unnecessary burden to end users. The number of endpoints and speed of change has made it impossible for human operators to manage without assistance from AI.
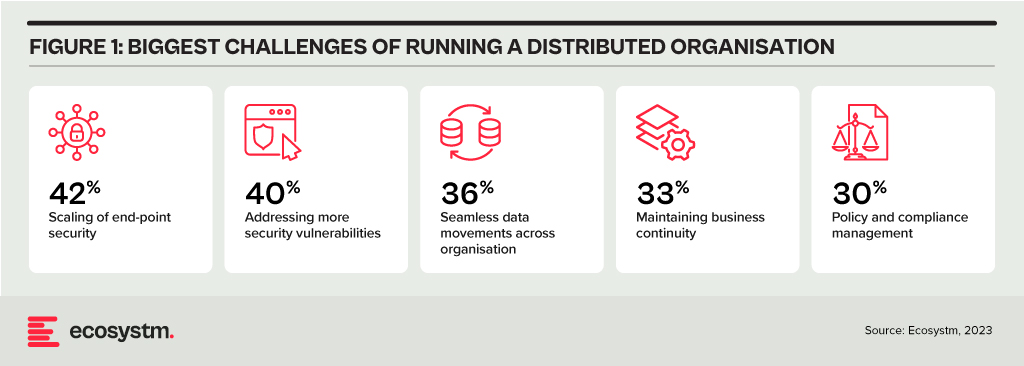
AI-Enhanced Network Management
Modern network operations centres are enhancing their visibility by aggregating data from diverse systems and consolidating them within a unified management platform. Machine learning (ML) and AI are employed to analyse data originating from enterprise networks, telecom Points of Presence (PoPs), IoT devices, cloud service providers, and user experience monitoring. These technologies enable the early identification of network issues before they reach critical levels. Intelligent networks can suggest strategies to enhance network resilience, forecast how modifications may impact performance, and are increasingly capable of autonomous responses to evolving conditions.
Here are some critical ways that AI/ML can help build resilient networks.
- Alert Noise Reduction. Network operations centres face thousands of alerts each day. As a result, operators battle with alert fatigue and are challenged to identify critical issues. Through the application of ML, contemporary monitoring tools can mitigate false positives, categorise interconnected alerts, and assist operators in prioritising the most pressing concerns. An operations team, augmented with AI capabilities could potentially de-prioritise up to 90% of alerts, allowing a concentrated focus on factors that impact network performance and resilience.
- Data Lakes. Networking vendors are building their own proprietary data lakes built upon telemetry data generated by the infrastructure they have deployed at customer sites. This vast volume of data allows them to use ML to create a tailored baseline for each customer and to recommend actions to optimise the environment.
- Root Cause Analysis. To assist network operators in diagnosing an issue, AIOps can sift through thousands of data points and correlate them to identify a root cause. Through the integration of alerts with change feeds, operators can understand the underlying causes of network problems or outages. By using ML to understand the customer’s unique environment, AIOps can progressively accelerate time to resolution.
- Proactive Response. As management layers become capable of recommending corrective action, proactive response also becomes possible, leading to self-healing networks. With early identification of sub-optimal conditions, intelligent systems can conduct load balancing, redirect traffic to higher performing SaaS regions, auto-scale cloud instances, or terminate selected connections.
- Device Profiling. In a BYOD environment, network managers require enhanced visibility to discover devices and enforce appropriate policies on them. Automated profiling against a validated database ensures guest access can be granted without adding friction to the onboarding process. With deep packet inspection, devices can be precisely classified based on behaviour patterns.
- Dynamic Bandwidth Aggregation. A key feature of an SD-WAN is that it can incorporate diverse transport types, such as fibre, 5G, and low earth orbit (LEO) satellite connectivity. Rather than using a simple primary and redundant architecture, bandwidth aggregation allows all circuits to be used simultaneously. By infusing intelligence into the SD-WAN layer, the process of path selection can dynamically prioritise traffic by directing it over higher quality or across multiple links. This approach guarantees optimal performance, even in the face of network degradation.
- Generative AI for Process Efficiency. Every tech company is trying to understand how they can leverage the power of Generative AI, and networking providers are no different. The most immediate use case will be to improve satisfaction and scalability for level 1 and level 2 support. A Generative AI-enabled service desk could provide uninterrupted support during high-volume periods, such as during network outages, or during off-peak hours.
Initiating an AI-Driven Network Management Journey
Network managers who take advantage of AI can build highly resilient networks that maximise uptime, deliver consistently high performance, and remain secure. Some important considerations when getting started include:
- Data Catalogue. Take stock of the data sources that are available to you, whether they come from network equipment telemetry, applications, or the data lake of a managed services provider. Understand how they can be integrated into an AIOps solution.
- Start Small. Begin with a pilot in an area where good data sources are available. This will help you assess the impact that AI could have on reducing alerts, improving mean time to repair (MTTR), increasing uptime, or addressing the skills gap.
- Develop an SD-WAN/SASE Roadmap. Many advanced AI benefits are built into an SD-WAN or SASE. Most organisations already have or will soon adopt SD-WAN but begin assessing the SASE framework to decide if it is suitable for your organisation.
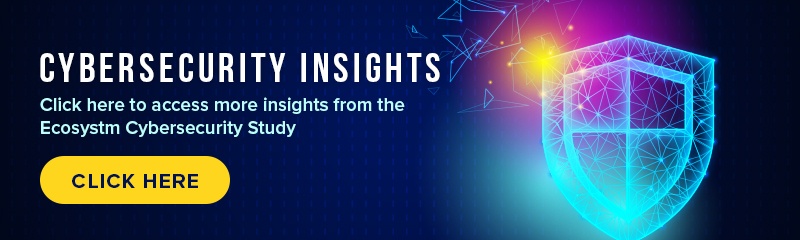
In this Insight, guest author Anirban Mukherjee lists out the key challenges of AI adoption in traditional organisations – and how best to mitigate these challenges. “I am by no means suggesting that traditional companies avoid or delay adopting AI. That would be akin to asking a factory to keep using only steam as power, even as electrification came in during early 20th century! But organisations need to have a pragmatic strategy around what will undoubtedly be a big, but necessary, transition.”
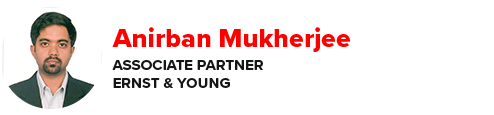
After years of evangelising digital adoption, I have more of a nuanced stance today – supporting a prudent strategy, especially where the organisation’s internal capabilities/technology maturity is in question. I still see many traditional organisations burning budgets in AI adoption programs with low success rates, simply because of poor choices driven by misplaced expectations. Without going into the obvious reasons for over-exuberance (media-hype, mis-selling, FOMO, irrational valuations – the list goes on), here are few patterns that can be detected in those organisations that have succeeded getting value – and gloriously so!
Data-driven decision-making is a cultural change. Most traditional organisations have a point person/role accountable for any important decision, whose “neck is on the line”. For these organisations to change over to trusting AI decisions (with its characteristic opacity, and stochastic nature of recommendations) is often a leap too far.
Work on your change management, but more crucially, strategically choose business/process decision points (aka use-cases) to acceptably AI-enable.
Technical choice of ML modeling needs business judgement too. The more flexible non-linear models that increase prediction accuracy, invariably suffer from lower interpretability – and may be a poor choice in many business contexts. Depending upon business data volumes and accuracy, model bias-variance tradeoffs need to be made. Assessing model accuracy and its thresholds (false-positive-false-negative trade-offs) are similarly nuanced. All this implies that organisation’s domain knowledge needs to merge well with data science design. A pragmatic approach would be to not try to be cutting-edge.
Look to use proven foundational model-platforms – such as those for NLP, visual analytics – for first use cases. Also note that not every problem needs AI; a lot can be sorted through traditional programming (“if-then automation”) and should be. The dirty secret of the industry is that the power of a lot of products marketed as “AI-powered” is mostly traditional logic, under the hood!
In getting results from AI, most often “better data trumps better models”. Practically, this means that organisations need to spend more on data engineering effort, than on data science effort. The CDO/CIO organisation needs to build the right balance of data competencies and tools.
Get the data readiness programs started – yesterday! While the focus of data scientists is often on training an AI model, deployment of the trained model online is a whole other level of technical challenge (particularly when it comes to IT-OT and real-time integrations).
It takes time to adopt AI in traditional organisations. Building up training data and model accuracy is a slow process. Organisational changes take time – and then you have to add considerations such as data standardisation; hygiene and integration programs; and the new attention required to build capabilities in AIOps, AI adoption and governance.
Typically plan for 3 years – monitor progress and steer every 6 months. Be ready to kill “zombie” projects along the way. Train the executive team – not to code, but to understand the technology’s capabilities and limitations. This will ensure better informed buyers/consumers and help drive adoption within the organisation.
I am by no means suggesting that traditional companies avoid or delay adopting AI. That would be akin to asking a factory to keep using only steam as power, even as electrification came in during early 20th century! But organisations need to have a pragmatic strategy around what will undoubtedly be a big, but necessary, transition.
These opinions are personal (and may change with time), but definitely informed through a decade of involvement in such journeys. It is not too early for any organisation to start – results are beginning to show for those who started earlier, and we know what they got right (and wrong).
I would love to hear your views, or even engage with you on your journey!
The views and opinions mentioned in the article are personal.
Anirban Mukherjee has more than 25 years of experience in operations excellence and technology consulting across the globe, having led transformations in Energy, Engineering, and Automotive majors. Over the last decade, he has focused on Smart Manufacturing/Industry 4.0 solutions that integrate cutting-edge digital into existing operations.
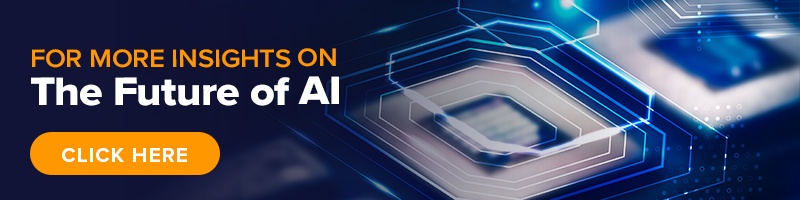
When non-organic (man-made) fabric was introduced into fashion, there were a number of harsh warnings about using polyester and man-made synthetic fibres, including their flammability.
In creating non-organic data sets, should we also be creating warnings on their use and flammability? Let’s look at why synthetic data is used in industries such as Financial Services, Automotive as well as for new product development in Manufacturing.
Synthetic Data Defined
Synthetic data can be defined as data that is artificially developed rather than being generated by actual interactions. It is often created with the help of algorithms and is used for a wide range of activities, including as test data for new products and tools, for model validation, and in AI model training. Synthetic data is a type of data augmentation which involves creating new and representative data.
Why is it used?
The main reasons why synthetic data is used instead of real data are cost, privacy, and testing. Let’s look at more specifics on this:
- Data privacy. When privacy requirements limit data availability or how it can be used. For example, in Financial Services where restrictions around data usage and customer privacy are particularly limiting, companies are starting to use synthetic data to help them identify and eliminate bias in how they treat customers – without contravening data privacy regulations.
- Data availability. When the data needed for testing a product does not exist or is not available to the testers. This is often the case for new releases.
- Data for testing. When training data is needed for machine learning algorithms. However, in many instances, such as in the case of autonomous vehicles, the data is expensive to generate in real life.
- Training across third parties using cloud. When moving private data to cloud infrastructures involves security and compliance risks. Moving synthetic versions of sensitive data to the cloud can enable organisations to share data sets with third parties for training across cloud infrastructures.
- Data cost. Producing synthetic data through a generative model is significantly more cost-effective and efficient than collecting real-world data. With synthetic data, it becomes cheaper and faster to produce new data once the generative model is set up.
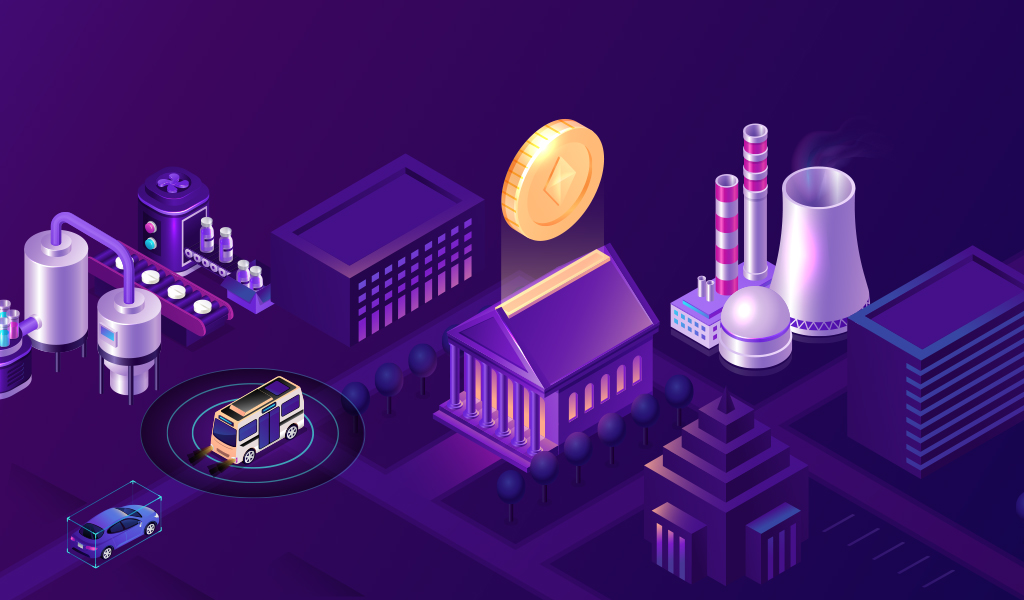
Why should it cause concern?
If real dataset contains biases, data augmented from it will contain biases, too. So, identification of optimal data augmentation strategy is important.
If the synthetic set doesn’t truly represent the original customer data set, it might contain the wrong buying signals regarding what customers are interested in or are inclined to buy.
Synthetic data also requires some form of output/quality control and internal regulation, specifically in highly regulated industries such as the Financial Services.
Creating incorrect synthetic data also can get a company in hot water with external regulators. For example, if a company created a product that harmed someone or didn’t work as advertised, it could lead to substantial financial penalties and, possibly, closer scrutiny in the future.
Conclusion
Synthetic data allows us to continue developing new and innovative products and solutions when the data necessary to do so wouldn’t otherwise be present or available due to volume, data sensitivity or user privacy challenges. Generating synthetic data comes with the flexibility to adjust its nature and environment as and when required in order to improve the performance of the model to create opportunities to check for outliers and extreme conditions.
The rollout of 5G combined with edge computing in remote locations will change the way maintenance is carried out in the field. Historically, service teams performed maintenance either in a reactive fashion – fixing equipment when it broke – or using a preventative calendar-based approach. Neither of these methods is satisfactory, with the former being too late and resulting in failure while the latter is necessarily too early, resulting in excessive expenditure and downtime. The availability of connected sensors has allowed service teams to shift to condition monitoring without the need for taking equipment offline for inspections. The advent of analytics takes this approach further and has given us optimised scheduling in the form of predictive maintenance.
The next step is prescriptive maintenance in which AI can recommend action based on current and predicted condition according to expected usage or environmental circumstances. This could be as simple as alerting an operator to automatically ordering parts and scheduling multiple servicing tasks depending on forecasted production needs in the short term.
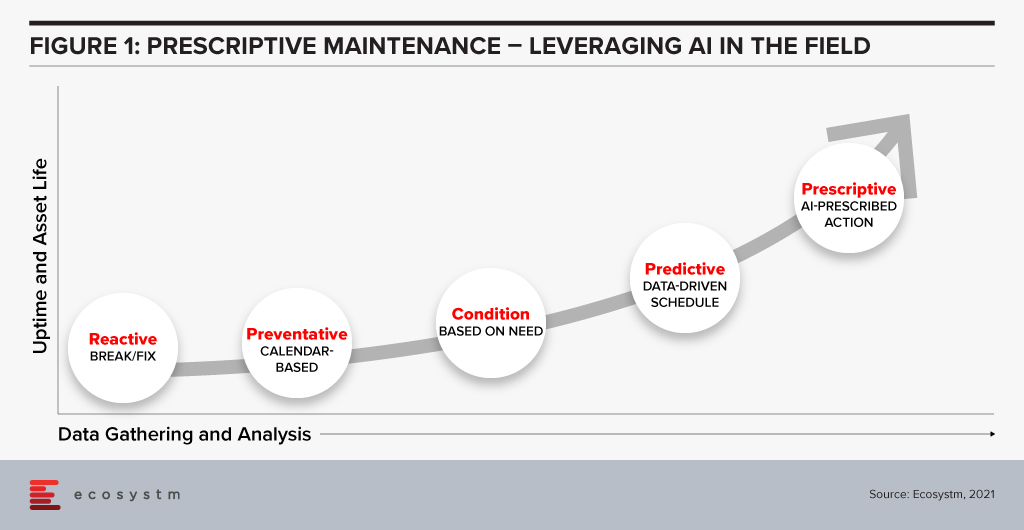
Prescriptive maintenance has only become possible with the advancement of AI and digital twin technology, but imminent improvements in connectivity and computing will take servicing to a new level. The rollout of 5G will give a boost to bandwidth, reduce latency, and increase the number of connections possible. Equipment in remote locations – such as transmission lines or machinery in resource industries – will benefit from the higher throughput of 5G connectivity, either as part of an operator’s network rollout or a private on-site deployment. Mobile machinery, particularly vehicles, which can include hundreds of sensors will no longer be required to wait until arrival before the condition can be assessed. Furthermore, vehicles equipped with external sensors can inspect stationary infrastructure as it passes by.
Edge computing – either carried out by miniature onboard devices or at smaller scale data centres embedded in 5G networks – ensure that intensive processing can be carried out closer to equipment than with a typical cloud environment. Bandwidth hungry applications, such as video and time series analysis, can be conducted with only meta data transmitted immediately and full archives uploaded with less urgency.
Prescriptive Maintenance with 5G and the Edge – Use Cases
- Transportation. Bridges built over railway lines equipped with high-speed cameras can monitor passing trains to inspect for damage. Data-intensive video analysis can be conducted on local devices for a rapid response while selected raw data can be uploaded to the cloud over 5G to improve inference models.
- Mining. Private 5G networks built-in remote sites can provide connectivity between fixed equipment, vehicles, drones, robotic dogs, workers, and remote operations centres. Autonomous haulage trucks can be monitored remotely and in the event of a breakdown, other vehicles can be automatically redirected to prevent dumping queues.
- Utilities. Emergency maintenance needs can be prioritised before extreme weather events based on meteorological forecasts and their impact on ageing parts. Machine learning can be used to understand location-specific effects of, for example, salt content in off-shore wind turbine cables. Early detection of turbine rotor cracks can recommend shutdown during high-load periods.

Data as an Asset
Effective prescriptive maintenance only becomes possible after the accumulation and integration of multiple data sources over an extended period. Inference models should understand both normal and abnormal equipment performance in various conditions, such as extreme weather, during incorrect operation, or when adjacent parts are degraded. For many smaller organisations or those deploying new equipment, the necessary volume of data will not be available without the assistance of equipment manufacturers. Moreover, even manufacturers will not have sufficient data on interaction with complementary equipment. This provides an opportunity for large operators to sell their own inference models as a new revenue stream. For example, an electrical grid operator in North America can partner with a similar, but smaller organisation in Europe to provide operational data and maintenance recommendations. Similarly, telecom providers, regional transportation providers, logistics companies, and smart cities will find industry players in other geographies that they do not naturally compete with.
Recommendations
- Employing multiple sensors. Baseline conditions and failure signatures are improved using machine learning based on feeds from multiple sensors, such as those that monitor vibration, sound, temperature, pressure, and humidity. The use of multiple sensors makes it possible to not only identify potential failure but also the reason for it and can therefore more accurately prescribe a solution to prevent an outage.
- Data assessment and integration. Prescriptive maintenance is most effective when multiple data sources are unified as inputs. Identify the location of these sources, such as ERP systems, time series on site, environmental data provided externally, or even in emails or on paper. A data fabric should be considered to ensure insights can be extracted from data no matter the environment it resides in.
- Automated action. Reduce the potential for human error or delay by automatically generating alerts and work orders for resource managers and service staff in the event of anomaly detection. Criticality measures should be adopted to help prioritise maintenance tasks and reduce alert noise.
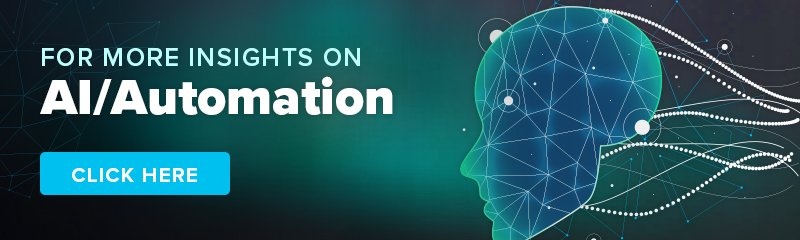
Many years ago – back in 2003 – I spent some quality time with BMC at their global analyst event in Phoenix, Arizona and they introduced the concept of “Business Service Management” (BSM). I was immediately a convert – that businesses can focus their IT Service Management initiatives on the business and customer services that the technology supports. Businesses that use BSM can have an understanding of the impact and importance of technology systems and assets because there is a direct link between these assets and the systems they support. A router that supports a customer payment platform suddenly becomes a much higher priority than one that supports an employee expense platform.
But for most businesses, this promise was never delivered. Creating a BSM solution became a highly manual process – mapping processes, assets, and applications. Many businesses that undertook this challenge reported that by the time they had mapped their processes, the map was out of date – as processes had changed; assets had been retired, replaced, or upgraded; software had been moved to the cloud or new modules had been implemented; and architectures had changed. Effectively their BSM mapping was often a pointless task – sometimes only delivering value in the slow to change systems – back-end applications and infrastructure that delivers limited value and has a defined retirement date.
The Growth of Digital Business Strategies
Our technology systems are becoming more important than ever as digital business strategies are realised and digital interactions with customers, employees, and partners significantly increase. Many businesses expect their digital investments to remain strong well into 2022 (Figure 1). More than ever, we need to understand the link between our tech systems and the business and customer services they support.
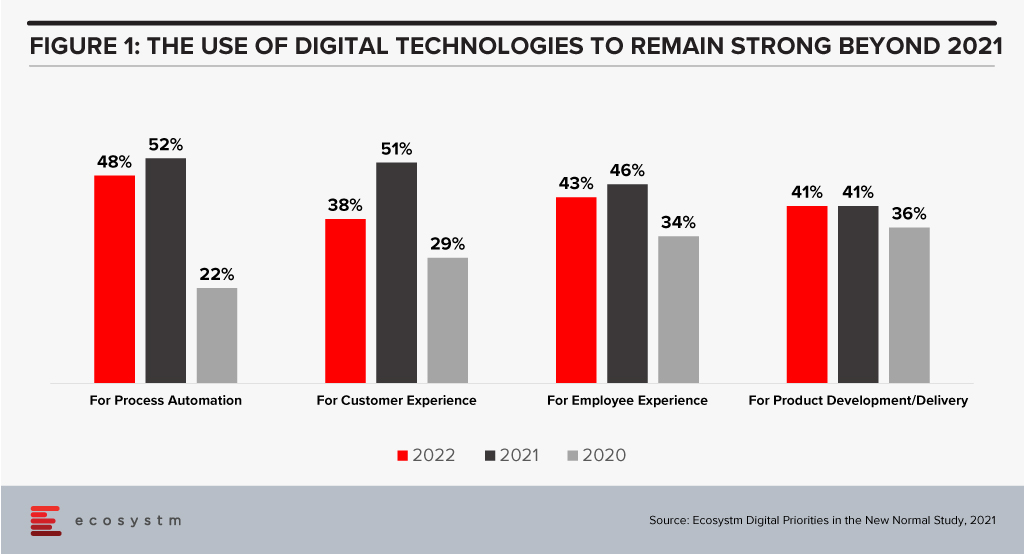
I recently had the opportunity to attend a briefing by ServiceNow regarding their new “AI-Powered Service Operations” that highlighted their service-aware CMDB – adding machine learning to their service mapping capabilities. The upgraded offering has the ability to map entire environments in hours or minutes – not months or weeks. And as a machine learning capability, it is only likely to get smarter – to learn from their customers’ use of the service and begin to recognise what applications, systems, and infrastructure are likely to be supporting each business service.
This heralds a new era in service management – one where the actual business and customer impact of outages is known immediately; where the decision to delay an upgrade or fix to a known problem can be made with a full understanding of the impacts. At one of my previous employers, email went down for about a week. It was finally attributed to an upgrade to network equipment that sat between the email system and the corporate network and the internet. The tech teams were scratching their heads for days as there was no documented link between this piece of hardware and the email system. The impact of the outage was certainly felt by the business – but had it happened at the end of the financial year, it could have impacted perhaps 10-20% of the business bookings as many deals came in at that time.
Being able to understand the link between infrastructure, cloud services, applications, databases, middleware and business processes and services is of huge value to every business – particularly as the percentage of business through digital channels and touchpoints continues to accelerate.
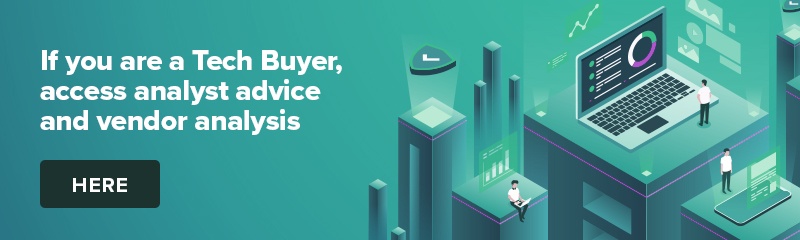