In my earlier post this week, I referred to the need for a grown-up conversation on AI. Here, I will focus on what conversations we need to have and what the solutions to AI disruption might be.
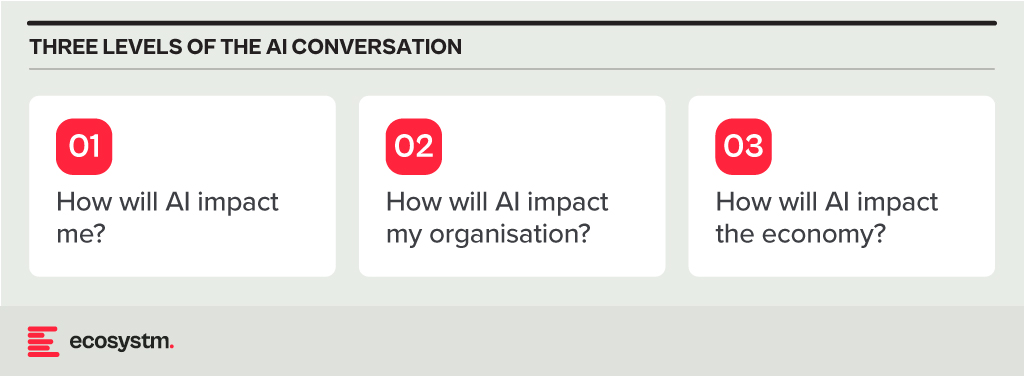
The Impact of AI on Individuals
AI is likely to impact people a lot! You might lose your job to AI. Even if it is not that extreme, it’s likely AI will do a lot of your job. And it might not be the “boring bits” – and sometimes the boring bits make a job manageable! IT helpdesk professionals, for instance, are already reporting that AIOps means they only deal with the difficult challenges. While that might be fun to start with, some personality types find this draining, knowing that every problem that ends up in the queue might take hours or days to resolve.
Your job will change. You will need new skills. Many organisations don’t invest in their employees, so you’ll need to upskill yourself in your own time and at your own cost. Look for employers who put new skill acquisition at the core of their employee offering. They are likelier to be more successful in the medium-to-long term and will also be the better employers with a happier workforce.
The Impact of AI on Organisations
Again – the impact on organisations will be huge. It will change the shape and size of organisations. We have already seen the impact in many industries. The legal sector is a major example where AI can do much of the job of a paralegal. Even in the IT helpdesk example shared earlier, where organisations with a mature tech environment will employ higher skilled professionals in most roles. These sectors need to think where their next generation of senior employees will come from, if junior roles go to AI. Software developers and coders are seeing greater demand for their skills now, even as AI tools increasingly augment their work. However, these skills are at an inflection point, as solutions like TuringBots have already started performing developer roles and are likely to take over the job of many developers and even designers in the near future.
Some industries will find that AI helps junior roles act more like senior employees, while others will use AI to perform the junior roles. AI will also create new roles (such as “prompt engineers”), but even those jobs will be done by AI in the future (and we are starting to see that).
HR teams, senior leadership, and investors need to work together to understand what the future might look like for their organisations. They need to start planning today for that future. Hint: invest in skills development and acquisition – that’s what will help you to succeed in the future.
The Impact of AI on the Economy
Assuming the individual and organisational impacts play out as described, the economic impacts of widespread AI adoption will be significant, similar to the “Great Depression”. If organisations lay off 30% of their employees, that means 30% of the economy is impacted, potentially leading to drying up of some government and an increase in government spend on welfare etc. – basically leading to major societal disruption.
The “AI won’t displace workers” narrative strikes me as the technological equivalent of climate change denial. Just like ignoring environmental warnings, dismissing the potential for AI to significantly impact the workforce is a recipe for disaster. Let’s not fall into the same trap and be an “AI denier”.
What is the Solution?
The solutions revolve around two ideas, and these need to be adopted at an industry level and driven by governments, unions, and businesses:
- Pay a living salary (for all citizens). Some countries already do this, with the Nordic nations leading the charge. And it is no surprise that some of these countries have had the most consistent long-term economic growth. The challenge today is that many governments cannot afford this – and it will become even less affordable as unemployment grows. The solution? Changing tax structures, taxing organisational earnings in-country (to stop them recognising local earnings in low-tax locations), and taxing wealth (not incomes). Also, paying essential workers who will not be replaced by AI (nurses, police, teachers etc.) better salaries will also help keep economies afloat. Easier said than done, of course!
- Move to a shorter work week (but pay full salaries). It is in the economic interest of every organisation that people stay gainfully employed. We have already discussed the ripple effect of job cuts. But if employees are given more flexibility, and working 3-day weeks, this not only spreads the work around more workers, but means that these workers have more time to spend money – ensuring continuing economic growth. Can every company do this? Probably not. But many can and they might have to. The concept of a 5-day work week isn’t that old (less than 100 years in fact – a 40-hour work week was only legislated in the US in the 1930s, and many companies had as little as 6-hour working days even in the 1950s). Just because we have worked this way for 80 years doesn’t mean that we will always have to. There is already a move towards 4-day work weeks. Tech.co surveyed over 1,000 US business leaders and found that 29% of companies with 4-day workweeks use AI extensively. In contrast, only 8% of organisations with a 5-day workweek use AI to the same degree.
AI Changes Everything
We are only at the beginning of the AI era. We have had a glimpse into the future, and it is both frightening and exciting. The opportunities for organisations to benefit from AI are already significant and will become even more as the technology improves and businesses learn to better adopt AI in areas where it can make an impact. But there will be consequences to this adoption. We already know what many of those consequences will be, so let’s start having those grown-up conversations today.
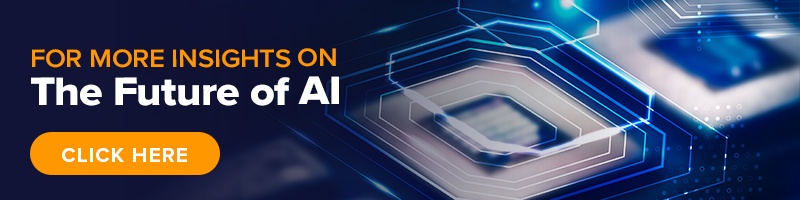
If you have seen me present recently – or even spoken to me for more than a few minutes, you’ve probably heard me go on about how the AI discussions need to change! At the moment, most senior executives, board rooms, governments, think tanks and tech evangelists are running around screaming with their hands on their ears when it comes to the impact of AI on jobs and society.
We are constantly being bombarded with the message that AI will help make knowledge workers more productive. AI won’t take people’s jobs – in fact it will help to create new jobs – you get the drift; you’ve been part of these conversations!
I was at an event recently where a leading cloud provider had a huge slide with the words: “Humans + AI Together” in large font across the screen. They then went on to demonstrate an opportunity for AI. In a live demo, they had the customer of a retailer call a store to check for stock of a dress. The call was handled by an AI solution, which engaged in a natural conversation with the customer. It verified their identity, checked dress stock at the store, processed the order, and even confirmed the customer’s intent to use their stored credit card.
So, in effect, on one slide, the tech provider emphasised that AI was not going to take our jobs, and two minutes later they showed how current AI capabilities could replace humans – today!
At an analyst event last week, representatives from three different tech providers told analysts how Microsoft Copilot is freeing up 10-15 hours a week. For a 40-hour work week, that’s a 25-38 time saving. In France (where the work week is 35 hours), that’s up to 43% of their time saved. So, by using a single AI platform, we can save 25-43% of our time – giving us the ability to work on other things.
What are the Real Benefits of AI?
The critical question is: What will we do with this saved time? Will it improve revenue or profit for businesses? AI might make us more agile, faster, more innovative but unless that translates to benefits on the bottom line, it is pointless. For example, adopting AI might mean we can create three times as many products. However, if we don’t make any more revenue and/or profit by having three times as many products, then any productivity benefit is worthless. UNLESS it is delivered through decreased costs.
We won’t need as many humans in our contact centres if AI is taking calls. Ideally, AI will lead to more personalised customer experiences – which will drive less calls to the contact centre in the first place! Even sales-related calls may disappear as personal AI bots will find deals and automatically sign us up. Of course, AI also costs money, particularly in terms of computing power. Some of the productivity uplift will be offset by the extra cost of the AI tools and platforms.
Many benefits that AI delivers will become table stakes. For example, if your competitor is updating their product four times a year and you are updating it annually, you might lose market share – so the benefits of AI might be just “keeping up with the competition”. But there are many areas where additional activity won’t deliver benefits. Organisations are unlikely to benefit from three times more promotional SMSs or EDMs and design work or brand redesigns.
I also believe that AI will create new roles. But you know what? AI will eventually do those jobs too. When automation came to agriculture, workers moved to factories. When automation came to factories, workers moved to offices. The (literally) trillion-dollar question is where workers go when automation comes to the office.
The Wider Impact of AI
The issue is that very few senior people in businesses or governments are planning for a future where maybe 30% of jobs done by knowledge workers go to AI. This could lead to the failure of economies. Government income will fall off a cliff. It will be unemployment on levels not seen since the great depression – or worse. And if we have not acknowledged these possible outcomes, how can we plan for it?
This is what I call the “grown up conversation about AI”. This is acknowledging the opportunity for AI and its impacts on companies, industries, governments and societies. Once we acknowledge these likely outcomes we can plan for it.
And that’s what I’ll discuss shortly – look out for my next Ecosystm Insight: The Three Possible Solutions for AI-driven Mass Unemployment.
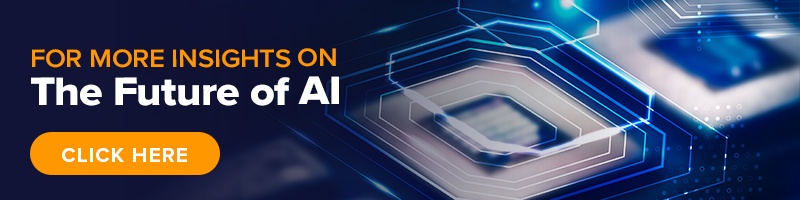
With over 70% of the world’s population predicted to live in cities by 2050, smart cities that use data, technology, and AI to streamline services are key to ensuring a healthy and safe environment for all who live, work, or visit them.
Fueled by rapid urbanisation, Southeast Asia is experiencing a smart city boom with an estimated 100 million people expected to move from rural areas to cities by 2030.
Despite their diverse populations and varying economic stages, ASEAN member countries are increasingly on the same page: they are all united by the belief that smart cities offer a solution to the complex urban and socio-economic challenges they face.
Read on to discover how Southeast Asian countries are using new tools to manage growth and deliver a better quality of life to hundreds of millions of people.
ASCN: A Network for Smarter Cities
The ASEAN Smart Cities Network (ASCN) is a collaborative platform where cities in the region exchange insights on adopting smart technology, finding solutions, and involving industry and global partners. They work towards the shared objective of fostering sustainable urban development and enhancing livability in their cities.
As of 2024, the ASCN includes 30 members, with new additions from Thailand and Indonesia.
“The ASEAN Smart Cities Network provides the sort of open platform needed to drive the smart city agenda. Different cities are at different levels of developments and “smartness” and ASEAN’s diversity is well suited for such a network that allows for cities to learn from one another.”
Taimur Khilji
UNITED NATIONS DEVELOPMENT PROGRAMME (UNDP)
Singapore’s Tech-Driven Future
The Smart Nation Initiative harnesses technology and data to improve citizens’ lives, boost economic competitiveness, and tackle urban challenges.
Smart mobility solutions, including sensor networks, real-time traffic management, and integrated public transportation with smart cards and mobile apps, have reduced congestion and travel times.
Ranked 5th globally and Asia’s smartest city, Singapore is developing a national digital twin to for better urban management. The 3D maps and subsurface model, created by the Singapore Land Authority, will help in managing infrastructure and assets.
The Smart City Initiative promotes sustainability with innovative systems like automated pneumatic waste collection and investments in water management and energy-efficient solutions.
Malaysia’s Holistic Smart City Approach
With aspirations to become a Smart Nation by 2040 (outlined in their Fourth National Physical Plan – NPP4), Malaysia is making strides.
Five pilot cities, including Kuala Lumpur and Johor Bahru, are testing the waters by integrating advanced technologies to modernise infrastructure.
Pilots embrace sustainability, with projects like Gamuda Cove showcasing smart technologies for intelligent traffic management and centralised security within eco-friendly developments.
Malaysia’s Smart Cities go beyond infrastructure, adopting international standards like the WELL Building Standard to enhance resident health, well-being, and productivity. The Ministry of Housing and Local Government, collaborating with PLANMalaysia and the Department of Standards Malaysia, has established clear indicators for Smart City development.
Indonesia’s Green Smart City Ambitions
Eyeing carbon neutrality by 2060, Indonesia is pushing its Smart City initiatives.
Their National Long-Term Development Plan prioritises economic growth and improved quality of life through digital infrastructure and innovative public services.
The goal is 100 smart cities that integrate green technology and sustainable infrastructure, reflecting their climate commitment.
Leaving behind congested Jakarta, Indonesia is building Nusantara, the world’s first “smart forest city“. Spanning 250,000 hectares, Nusantara will boast high-capacity infrastructure, high-speed internet, and cutting-edge technology to support the archipelago’s activities.
Thailand’s Smart City Boom
Thailand’s national agenda goes big on smart cities.
They aim for 105 smart cities by 2027, with a focus on transportation, environment, and safety.
Key projects include:
- USD 37 billion smart city in Huai Yai with business centres and housing for 350,000.
- A 5G-powered smart city in Ban Chang for enhanced environmental and traffic management.
- USD $40 billion investment to create a smart regional financial centre across Chonburi, Rayong, and Chachoengsao.
Philippines Fights Urban Challenges with Smart Solutions
By 2050, population in cities is expected to soar to nearly 102 million – twice the current figure.
A glimmer of optimism emerges with the rise of smart city solutions championed by local governments (LGUs).
Rapid urbanisation burdens the Philippines with escalating waste. By 2025, daily waste production could reach a staggering 28,000 tonnes. Smart waste management solutions are being implemented to optimise collection and reduce fuel consumption.
Smart city developer Iveda is injecting innovation. Their ambitious USD 5 million project brings AI-powered technology to cities like Cebu, Bacolod, Iloilo, and Davao. The focus: leverage technology to modernise airports, roads, and sidewalks, paving the way for a more sustainable and efficient urban future.
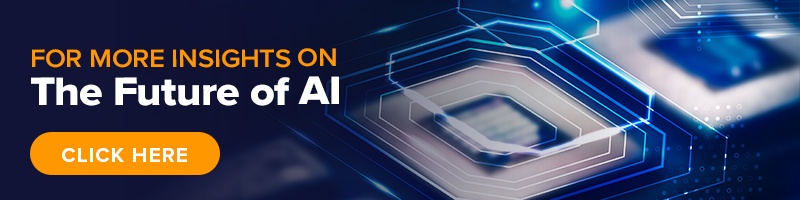
AI tools have become a game-changer for the technology industry, enhancing developer productivity and software quality. Leveraging advanced machine learning models and natural language processing, these tools offer a wide range of capabilities, from code completion to generating entire blocks of code, significantly reducing the cognitive load on developers. AI-powered tools not only accelerate the coding process but also ensure higher code quality and consistency, aligning seamlessly with modern development practices. Organisations are reaping the benefits of these tools, which have transformed the software development lifecycle.
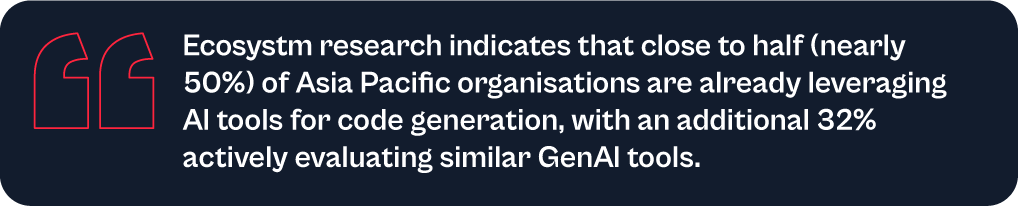
Impact on Developer Productivity
AI tools are becoming an indispensable part of software development owing to their:
- Speed and Efficiency. AI-powered tools provide real-time code suggestions, which dramatically reduces the time developers spend writing boilerplate code and debugging. For example, Tabnine can suggest complete blocks of code based on the comments or a partial code snippet, which accelerates the development process.
- Quality and Accuracy. By analysing vast datasets of code, AI tools can offer not only syntactically correct but also contextually appropriate code suggestions. This capability reduces bugs and improves the overall quality of the software.
- Learning and Collaboration. AI tools also serve as learning aids for developers by exposing them to new or better coding practices and patterns. Novice developers, in particular, can benefit from real-time feedback and examples, accelerating their professional growth. These tools can also help maintain consistency in coding standards across teams, fostering better collaboration.
Advantages of Using AI Tools in Development
- Reduced Time to Market. Faster coding and debugging directly contribute to shorter development cycles, enabling organisations to launch products faster. This reduction in time to market is crucial in today’s competitive business environment where speed often translates to a significant market advantage.
- Cost Efficiency. While there is an upfront cost in integrating these AI tools, the overall return on investment (ROI) is enhanced through the reduced need for extensive manual code reviews, decreased dependency on large development teams, and lower maintenance costs due to improved code quality.
- Scalability and Adaptability. AI tools learn and adapt over time, becoming more efficient and aligned with specific team or project needs. This adaptability ensures that the tools remain effective as the complexity of projects increases or as new technologies emerge.
Deployment Models
The choice between SaaS and on-premises deployment models involves a trade-off between control, cost, and flexibility. Organisations need to consider their specific requirements, including the level of control desired over the infrastructure, sensitivity of the data, compliance needs, and available IT resources. A thorough assessment will guide the decision, ensuring that the deployment model chosen aligns with the organisation’s operational objectives and strategic goals.
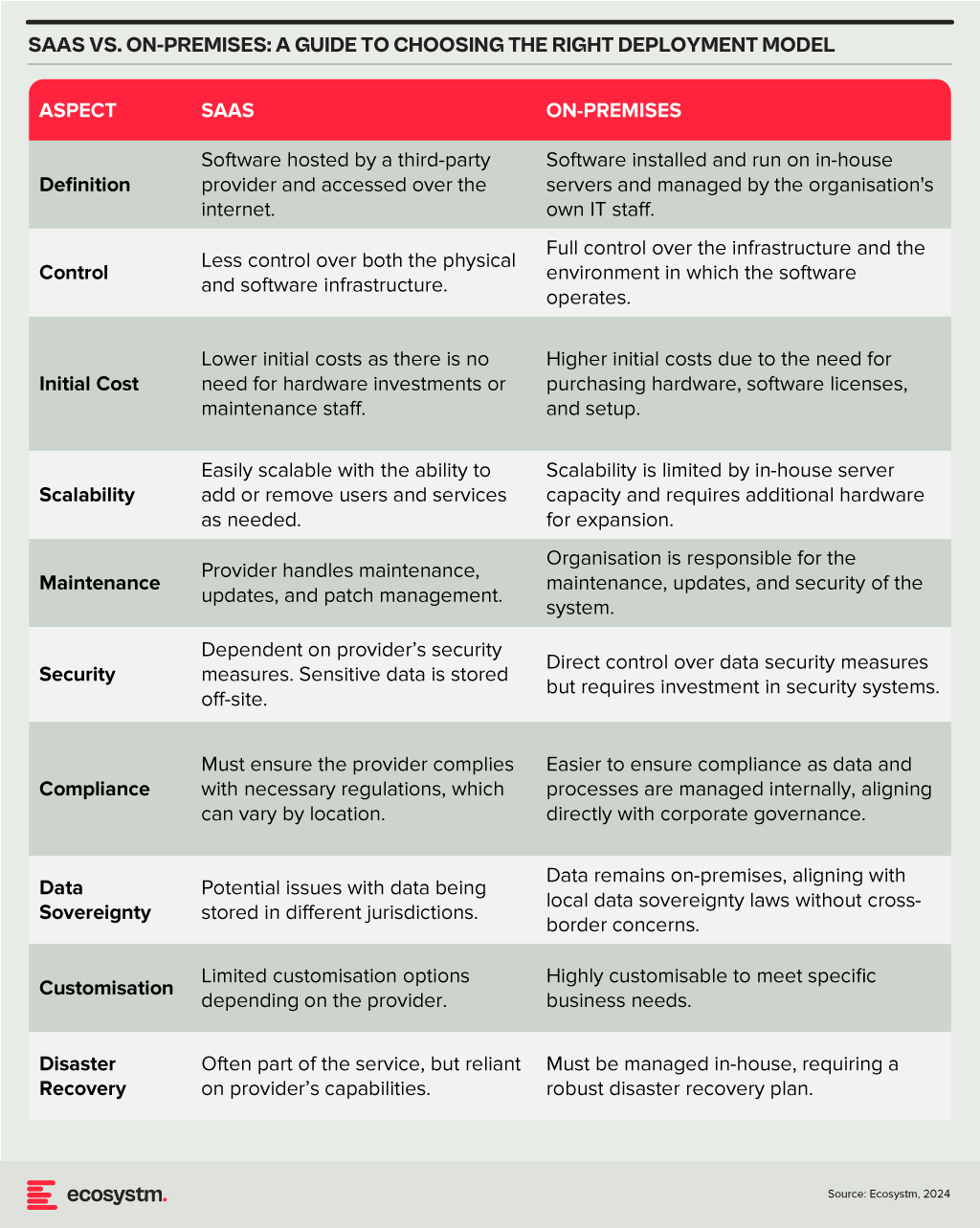
Technology teams must consider challenges such as the reliability of generated code, the potential for generating biased or insecure code, and the dependency on external APIs or services. Proper oversight, regular evaluations, and a balanced integration of AI tools with human oversight are recommended to mitigate these risks.
A Roadmap for AI Integration
The strategic integration of AI tools in software development offers a significant opportunity for companies to achieve a competitive edge. By starting with pilot projects, organisations can assess the impact and utility of AI within specific teams. Encouraging continuous training in AI advancements empowers developers to leverage these tools effectively. Regular audits ensure that AI-generated code adheres to security standards and company policies, while feedback mechanisms facilitate the refinement of tool usage and address any emerging issues.
Technology teams have the opportunity to not only boost operational efficiency but also cultivate a culture of innovation and continuous improvement in their software development practices. As AI technology matures, even more sophisticated tools are expected to emerge, further propelling developer capabilities and software development to new heights.
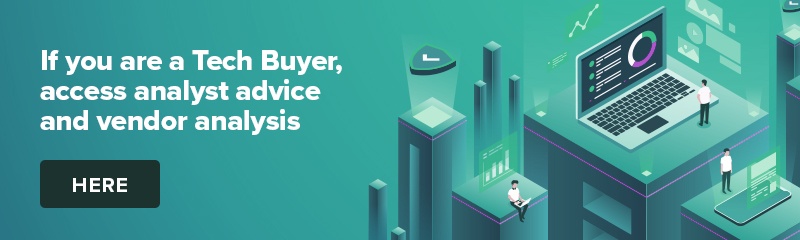
Southeast Asia’s massive workforce – 3rd largest globally – faces a critical upskilling gap, especially with the rise of AI. While AI adoption promises a USD 1 trillion GDP boost by 2030, unlocking this potential requires a future-proof workforce equipped with AI expertise.
Governments and technology providers are joining forces to build strong AI ecosystems, accelerating R&D and nurturing homegrown talent. It’s a tight race, but with focused investments, Southeast Asia can bridge the digital gap and turn its AI aspirations into reality.
Read on to find out how countries like Singapore, Thailand, Vietnam, and The Philippines are implementing comprehensive strategies to build AI literacy and expertise among their populations.
Download ‘Upskilling for the Future: Building AI Capabilities in Southeast Asia’ as a PDF
Big Tech Invests in AI Workforce
Southeast Asia’s tech scene heats up as Big Tech giants scramble for dominance in emerging tech adoption.
Microsoft is partnering with governments, nonprofits, and corporations across Indonesia, Malaysia, the Philippines, Thailand, and Vietnam to equip 2.5M people with AI skills by 2025. Additionally, the organisation will also train 100,000 Filipino women in AI and cybersecurity.
Singapore sets ambitious goal to triple its AI workforce by 2028. To achieve this, AWS will train 5,000 individuals annually in AI skills over the next three years.
NVIDIA has partnered with FPT Software to build an AI factory, while also championing AI education through Vietnamese schools and universities. In Malaysia, they have launched an AI sandbox to nurture 100 AI companies targeting USD 209M by 2030.
Singapore Aims to be a Global AI Hub
Singapore is doubling down on upskilling, global leadership, and building an AI-ready nation.
Singapore has launched its second National AI Strategy (NAIS 2.0) to solidify its global AI leadership. The aim is to triple the AI talent pool to 15,000, establish AI Centres of Excellence, and accelerate public sector AI adoption. The strategy focuses on developing AI “peaks of excellence” and empowering people and businesses to use AI confidently.
In keeping with this vision, the country’s 2024 budget is set to train workers who are over 40 on in-demand skills to prepare the workforce for AI. The country will also invest USD 27M to build AI expertise, by offering 100 AI scholarships for students and attracting experts from all over the globe to collaborate with the country.
Thailand Aims for AI Independence
Thailand’s ‘Ignite Thailand’ 2030 vision focuses on boosting innovation, R&D, and the tech workforce.
Thailand is launching the second phase of its National AI Strategy, with a USD 42M budget to develop an AI workforce and create a Thai Large Language Model (ThaiLLM). The plan aims to train 30,000 workers in sectors like tourism and finance, reducing reliance on foreign AI.
The Thai government is partnering with Microsoft to build a new data centre in Thailand, offering AI training for over 100,000 individuals and supporting the growing developer community.
Building a Digital Vietnam
Vietnam focuses on AI education, policy, and empowering women in tech.
Vietnam’s National Digital Transformation Programme aims to create a digital society by 2030, focusing on integrating AI into education and workforce training. It supports AI research through universities and looks to address challenges like addressing skill gaps, building digital infrastructure, and establishing comprehensive policies.
The Vietnamese government and UNDP launched Empower Her Tech, a digital skills initiative for female entrepreneurs, offering 10 online sessions on GenAI and no-code website creation tools.
The Philippines Gears Up for AI
The country focuses on investment, public-private partnerships, and building a tech-ready workforce.
With its strong STEM education and programming skills, the Philippines is well-positioned for an AI-driven market, allocating USD 30M for AI research and development.
The Philippine government is partnering with entities like IBPAP, Google, AWS, and Microsoft to train thousands in AI skills by 2025, offering both training and hands-on experience with cutting-edge technologies.
The strategy also funds AI research projects and partners with universities to expand AI education. Companies like KMC Teams will help establish and manage offshore AI teams, providing infrastructure and support.
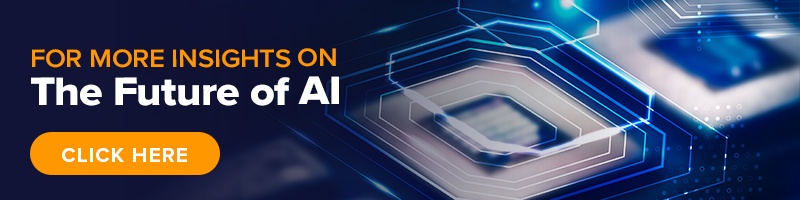
To mitigate the challenges of cloud vendor lock-in, 44% of organisations in Thailand will prioritise data centre consolidation and infrastructure modernisation in 2024.
Explore the key trends impacting Thailand’s technology adoption. Keep an eye out for more data-backed insights on tech markets across Southeast Asia.
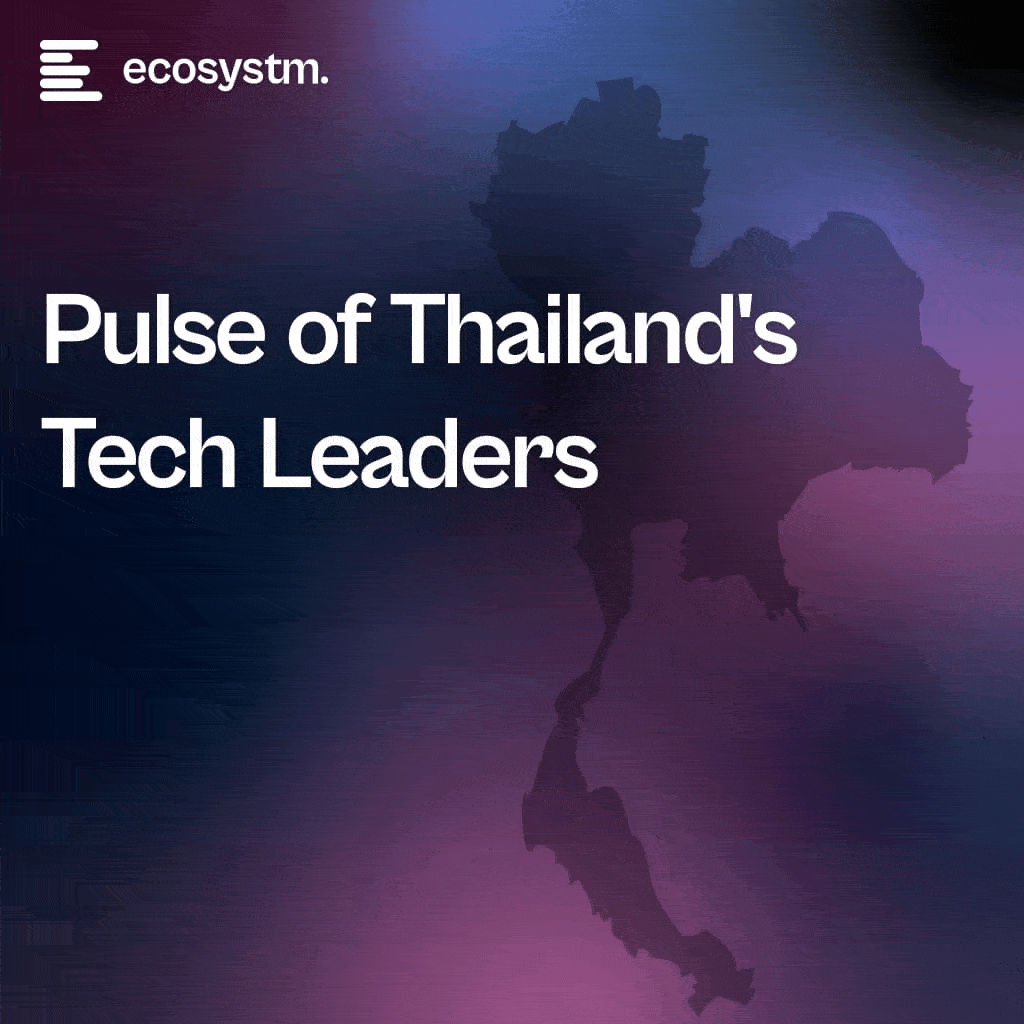
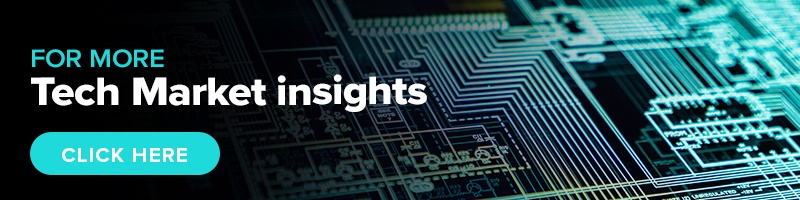
ASEAN, poised to become the world’s 4th largest economy by 2030, is experiencing a digital boom. With an estimated 125,000 new internet users joining daily, it is the fastest-growing digital market globally. These users are not just browsing, but are actively engaged in data-intensive activities like gaming, eCommerce, and mobile business. As a result, monthly data usage is projected to soar from 9.2 GB per user in 2020 to 28.9 GB per user by 2025, according to the World Economic Forum. Businesses and governments are further fuelling this transformation by embracing Cloud, AI, and digitisation.
Investments in data centre capacity across Southeast Asia are estimated to grow at a staggering pace to meet this growing demand for data. While large hyperscale facilities are currently handling much of the data needs, edge computing – a distributed model placing data centres closer to users – is fast becoming crucial in supporting tomorrow’s low-latency applications and services.
The Big & the Small: The Evolving Data Centre Landscape
As technology pushes boundaries with applications like augmented reality, telesurgery, and autonomous vehicles, the demand for ultra-low latency response times is skyrocketing. Consider driverless cars, which generate a staggering 5 TB of data per hour and rely heavily on real-time processing for split-second decisions. This is where edge data centres come in. Unlike hyperscale data centres, edge data centres are strategically positioned closer to users and devices, minimising data travel distances and enabling near-instantaneous responses; and are typically smaller with a capacity ranging from 500 KW to 2 MW. In comparison, large data centres have a capacity of more than 80MW.
While edge data centres are gaining traction, cloud-based hyperscalers such as AWS, Microsoft Azure, and Google Cloud remain a dominant force in the Southeast Asian data centre landscape. These facilities require substantial capital investment – for instance, it took almost USD 1 billion to build Meta’s 150 MW hyperscale facility in Singapore – but offer immense processing power and scalability. While hyperscalers have the resources to build their own data centres in edge locations or emerging markets, they often opt for colocation facilities to familiarise themselves with local markets, build out operations, and take a “wait and see” approach before committing significant investments in the new market.
The growth of data centres in Southeast Asia – whether edge, cloud, hyperscale, or colocation – can be attributed to a range of factors. The region’s rapidly expanding digital economy and increasing internet penetration are the prime reasons behind the demand for data storage and processing capabilities. Additionally, stringent data sovereignty regulations in many Southeast Asian countries require the presence of local data centres to ensure compliance with data protection laws. Indonesia’s Personal Data Protection Law, for instance, allows personal data to be transferred outside of the country only where certain stringent security measures are fulfilled. Finally, the rising adoption of cloud services is also fuelling the need for onshore data centres to support cloud infrastructure and services.
Notable Regional Data Centre Hubs
Singapore. Singapore imposed a moratorium on new data centre developments between 2019 to 2022 due to concerns over energy consumption and sustainability. However, the city-state has recently relaxed this ban and announced a pilot scheme allowing companies to bid for permission to develop new facilities.
In 2023, the Singapore Economic Development Board (EDB) and the Infocomm Media Development Authority (IMDA) provisionally awarded around 80 MW of new capacity to four data centre operators: Equinix, GDS, Microsoft, and a consortium of AirTrunk and ByteDance (TikTok’s parent company). Singapore boasts a formidable digital infrastructure with 100 data centres, 1,195 cloud service providers, and 22 network fabrics. Its robust network, supported by 24 submarine cables, has made it a global cloud connectivity leader, hosting major players like AWS, Azure, IBM Softlayer, and Google Cloud.
Aware of the high energy consumption of data centres, Singapore has taken a proactive stance towards green data centre practices. A collaborative effort between the IMDA, government agencies, and industries led to the development of a “Green Data Centre Standard“. This framework guides organisations in improving data centre energy efficiency, leveraging the established ISO 50001 standard with customisations for Singapore’s context. The standard defines key performance metrics for tracking progress and includes best practices for design and operation. By prioritising green data centres, Singapore strives to reconcile its digital ambitions with environmental responsibility, solidifying its position as a leading Asian data centre hub.
Malaysia. Initiatives like MyGovCloud and the Digital Economy Blueprint are driving Malaysia’s public sector towards cloud-based solutions, aiming for 80% use of cloud storage. Tenaga Nasional Berhad also established a “green lane” for data centres, solidifying Malaysia’s commitment to environmentally responsible solutions and streamlined operations. Some of the big companies already operating include NTT Data Centers, Bridge Data Centers and Equinix.
The district of Kulai in Johor has emerged as a hotspot for data centre activity, attracting major players like Nvidia and AirTrunk. Conditional approvals have been granted to industry giants like AWS, Microsoft, Google, and Telekom Malaysia to build hyperscale data centres, aimed at making the country a leading hub for cloud services in the region. AWS also announced a new AWS Region in the country that will meet the high demand for cloud services in Malaysia.
Indonesia. With over 200 million internet users, Indonesia boasts one of the world’s largest online populations. This expanding internet economy is leading to a spike in the demand for data centre services. The Indonesian government has also implemented policies, including tax incentives and a national data centre roadmap, to stimulate growth in this sector.
Microsoft, for instance, is set to open its first regional data centre in Thailand and has also announced plans to invest USD 1.7 billion in cloud and AI infrastructure in Indonesia. The government also plans to operate 40 MW of national data centres across West Java, Batam, East Kalimantan, and East Nusa Tenggara by 2026.
Thailand. Remote work and increasing online services have led to a data centre boom, with major industry players racing to meet Thailand’s soaring data demands.
In 2021, Singapore’s ST Telemedia Global Data Centres launched its first 20 MW hyperscale facility in Bangkok. Soon after, AWS announced a USD 5 billion investment plan to bolster its cloud capacity in Thailand and the region over the next 15 years. Heavyweights like TCC Technology Group, CAT Telecom, and True Internet Data Centre are also fortifying their data centre footprints to capitalise on this explosive growth. Microsoft is also set to open its first regional data centre in the country.
Conclusion
Southeast Asia’s booming data centre market presents a goldmine of opportunity for tech investment and innovation. However, navigating this lucrative landscape requires careful consideration of legal hurdles. Data protection regulations, cross-border data transfer restrictions, and local policies all pose challenges for investors. Beyond legal complexities, infrastructure development needs and investment considerations must also be addressed. Despite these challenges, the potential rewards for companies that can navigate them are substantial.
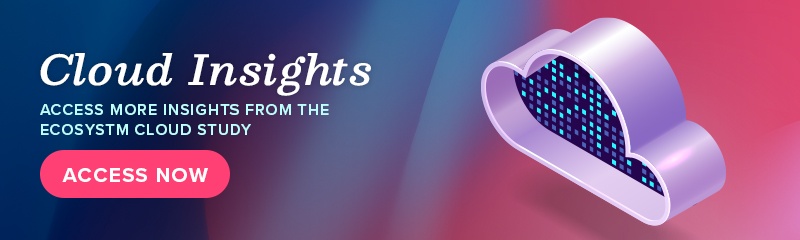