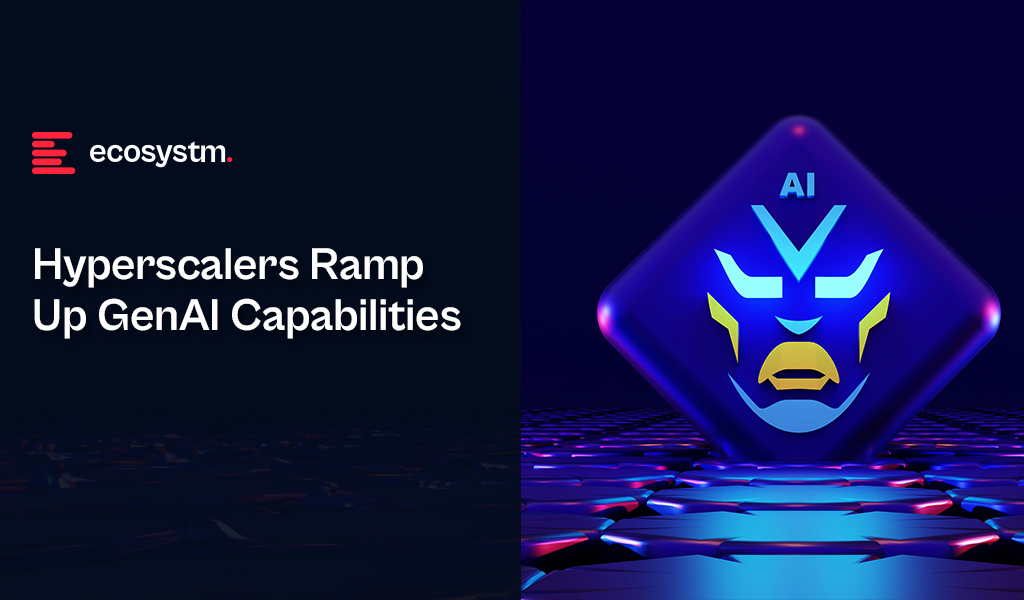
When OpenAI released ChatGPT, it became obvious – and very fast – that we were entering a new era of AI. Every tech company scrambled to release a comparable service or to infuse their products with some form of GenAI. Microsoft, piggybacking on its investment in OpenAI was the fastest to market with impressive text and image generation for the mainstream. Copilot is now embedded across its software, including Microsoft 365, Teams, GitHub, and Dynamics to supercharge the productivity of developers and knowledge workers. However, the race is on – AWS and Google are actively developing their own GenAI capabilities.
AWS Catches Up as Enterprise Gains Importance
Without a consumer-facing AI assistant, AWS was less visible during the early stages of the GenAI boom. They have since rectified this with a USD 4B investment into Anthropic, the makers of Claude. This partnership will benefit both Amazon and Anthropic, bringing the Claude 3 family of models to enterprise customers, hosted on AWS infrastructure.
As GenAI quickly emerges from shadow IT to an enterprise-grade tool, AWS is catching up by capitalising on their position as cloud leader. Many organisations view AWS as a strategic partner, already housing their data, powering critical applications, and providing an environment that developers are accustomed to. The ability to augment models with private data already residing in AWS data repositories will make it an attractive GenAI partner.
AWS has announced the general availability of Amazon Q, their suite of GenAI tools aimed at developers and businesses. Amazon Q Developer expands on what was launched as Code Whisperer last year. It helps developers accelerate the process of building, testing, and troubleshooting code, allowing them to focus on higher-value work. The tool, which can directly integrate with a developer’s chosen IDE, uses NLP to develop new functions, modernise legacy code, write security tests, and explain code.
Amazon Q Business is an AI assistant that can safely ingest an organisation’s internal data and connect with popular applications, such as Amazon S3, Salesforce, Microsoft Exchange, Slack, ServiceNow, and Jira. Access controls can be implemented to ensure data is only shared with authorised users. It leverages AWS’s visualisation tool, QuickSight, to summarise findings. It also integrates directly with applications like Slack, allowing users to query it directly.
Going a step further, Amazon Q Apps (in preview) allows employees to build their own lightweight GenAI apps using natural language. These employee-created apps can then be published to an enterprise’s app library for broader use. This no-code approach to development and deployment is part of a drive to use AI to increase productivity across lines of business.
AWS continues to expand on Bedrock, their managed service providing access to foundational models from companies like Mistral AI, Stability AI, Meta, and Anthropic. The service also allows customers to bring their own model in cases where they have already pre-trained their own LLM. Once a model is selected, organisations can extend its knowledge base using Retrieval-Augmented Generation (RAG) to privately access proprietary data. Models can also be refined over time to improve results and offer personalised experiences for users. Another feature, Agents for Amazon Bedrock, allows multi-step tasks to be performed by invoking APIs or searching knowledge bases.
To address AI safety concerns, Guardrails for Amazon Bedrock is now available to minimise harmful content generation and avoid negative outcomes for users and brands. Contentious topics can be filtered by varying thresholds, and Personally Identifiable Information (PII) can be masked. Enterprise-wide policies can be defined centrally and enforced across multiple Bedrock models.
Google Targeting Creators
Due to the potential impact on their core search business, Google took a measured approach to entering the GenAI field, compared to newer players like OpenAI and Perplexity. The useability of Google’s chatbot, Gemini, has improved significantly since its initial launch under the moniker Bard. Its image generator, however, was pulled earlier this year while it works out how to carefully tread the line between creativity and sensitivity. Based on recent demos though, it plans to target content creators with images (Imagen 3), video generation (Veo), and music (Lyria).
Like Microsoft, Google has seen that GenAI is a natural fit for collaboration and office productivity. Gemini can now assist the sidebar of Workspace apps, like Docs, Sheets, Slides, Drive, Gmail, and Meet. With Google Search already a critical productivity tool for most knowledge workers, it is determined to remain a leader in the GenAI era.
At their recent Cloud Next event, Google announced the Gemini Code Assist, a GenAI-powered development tool that is more robust than its previous offering. Using RAG, it can customise suggestions for developers by accessing an organisation’s private codebase. With a one-million-token large context window, it also has full codebase awareness making it possible to make extensive changes at once.
The Hardware Problem of AI
The demands that GenAI places on compute and memory have created a shortage of AI chips, causing the valuation of GPU giant, NVIDIA, to skyrocket into the trillions of dollars. Though the initial training is most hardware-intensive, its importance will only rise as organisations leverage proprietary data for custom model development. Inferencing is less compute-heavy for early use cases, such as text generation and coding, but will be dwarfed by the needs of image, video, and audio creation.
Realising compute and memory will be a bottleneck, the hyperscalers are looking to solve this constraint by innovating with new chip designs of their own. AWS has custom-built specialised chips – Trainium2 and Inferentia2 – to bring down costs compared to traditional compute instances. Similarly, Microsoft announced the Maia 100, which it developed in conjunction with OpenAI. Google also revealed its 6th-generation tensor processing unit (TPU), Trillium, with significant increase in power efficiency, high bandwidth memory capacity, and peak compute performance.
The Future of the GenAI Landscape
As enterprises gain experience with GenAI, they will look to partner with providers that they can trust. Challenges around data security, governance, lineage, model transparency, and hallucination management will all need to be resolved. Additionally, controlling compute costs will begin to matter as GenAI initiatives start to scale. Enterprises should explore a multi-provider approach and leverage specialised data management vendors to ensure a successful GenAI journey.
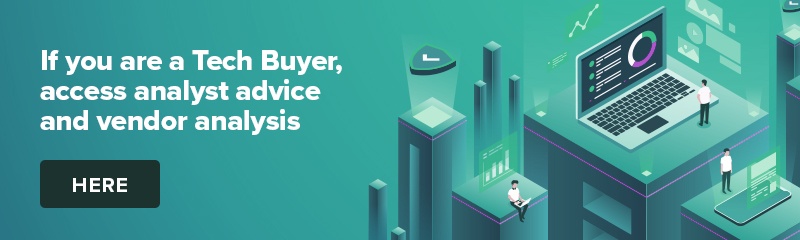