Innovation is a driving force behind new approaches, often occurring at the point of adoption rather than technology development. As public sector organisations increasingly focus on improving citizen services through technology, it is important to adopt a strategic approach that considers innovation as a complex journey of systemic and cultural transformation. This strategic approach should guide the integration of technology into citizen services.
Here is a comprehensive look at what public sector organisations should consider when integrating technology into citizen services.
Download ‘Future-Proofing Citizen Services: Technology Strategies for the Public Sector‘ as a PDF
1. Immediate View: Foundational Technologies
The immediate view focuses on deploying technologies that are widely adopted and essential for current digital service provision. These foundational technologies serve as the backbone for enhancing citizen services.
Foundational Technologies
Web 2.0. Establishing a solid online presence is usually the first step, as it is the broadest channel for reaching customers. Web 2.0 refers to the current state of the internet, encompassing dynamic content and interactive websites.
Mobile Applications. Given that mobile usage has surpassed desktop, a mobile-responsive platform or a dedicated mobile app is crucial. Mobile apps provide a more specialised and immersive user experience by utilising device-specific features like GPS, document scanning, and push notifications.
2. Second-Generation Enablers: Emerging Technologies
As organisations establish foundational technologies, they should look towards second-generation enablers. Although less mature, these technologies offer emerging digital opportunities, and can significantly enhance service differentiation.
Emerging Technologies
Interactive Voice Response (IVR) systems improve the efficiency and effectiveness of digital services by routing callers to self-service options and providing relevant information without human intervention. These systems operate outside typical government agency working hours, ensuring continuous accessibility. Additionally, IVRs generate valuable data for future Voice of the Customer programs, improving overall service quality and responsiveness.
Digital Wallets facilitate transactions by expediting fund transfers and enhancing transparency through meticulous transaction records. They streamline administrative tasks, simplify transactions, and encourage service usage and adoption.
AI-driven Virtual Agents or chatbots revolutionise customer interactions by providing 24/7 support. They offer prompt, efficient, and personalised services, enhancing customer satisfaction and trust. In resource-limited public sectors, virtual agents are cost-effective, optimising resource allocation and meeting growing service demands. Specialised virtual agents for specific sectors can further differentiate service providers.
3. Futuristic View: Ambitious Innovations
The futuristic view focuses on forward-looking technologies that address long-term roadblocks and offer transformative potential. These technologies are currently speculative but hold the promise of significantly reshaping the market.
Innovations
Subscription Management models enable public sector information services to be accessed in highly personalised ways, thereby enhancing citizen engagement. This model supports regulatory oversight by providing common data insights and improves the management of services, ultimately benefiting the public by ensuring more responsive and tailored information delivery.
AI concierge leverages advanced technologies like Natural Language Processing, Computer Vision, and Speech Technologies to provide personalised and proactive customer service. They redefine customer management, ensuring a seamless and tailored experience.
Immersive reality technologies, such as augmented and virtual reality (AR/VR) create captivating customer experiences by allowing interactions in virtual environments. These technologies establish a shared virtual environment, helping customers to engage with businesses and each other in new and immersive ways. As an emerging customer management tool, immersive reality can transform the dynamics of customer-business relationships, adding substantial value to the service experience.
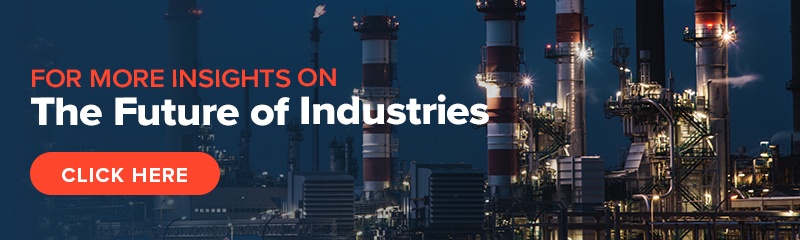
Data analysts play a vital role in today’s data-driven world, providing crucial insights that benefit decision-making processes. For those with a knack for numbers and a passion for uncovering patterns, a career as a data analyst can be both fulfilling and lucrative – it can also be a stepping stone towards other careers in data. While a data analyst focuses on data preparation and visualisation, an AI engineer specialises in creating AI solutions, a machine learning (ML) engineer concentrates on implementing ML models, and a data scientist combines elements of data analysis and ML to derive insights and predictions from data.
Tools, Skills, and Techniques of a Data Analyst
Excel Mastery. Unlocks a powerful toolbox for data manipulation and analysis. Essential skills include using a vast array of functions for calculations and data transformation. Pivot tables become your secret weapon for summarising and analysing large datasets, while charts and graphs bring your findings to life with visual clarity. Data validation ensures accuracy, and the Analysis ToolPak and Solver provide advanced functionalities for statistical analysis and complex problem-solving. Mastering Excel empowers you to transform raw data into actionable insights.
Advanced SQL. While basic skills handle simple queries, advanced users can go deeper with sorting, aggregation, and the art of JOINs to combine data from multiple tables. Common Table Expressions (CTEs) and subqueries become your allies for crafting complex queries, while aggregate functions summarise vast amounts of data. Window functions add another layer of power, allowing calculations within query results. Mastering Advanced SQL empowers you to extract hidden insights and manage data with unparalleled precision.
Data Visualisation. Crafts impactful data stories. These tools empower you to connect to various data sources, transform raw information into a usable format, and design interactive dashboards and reports. Filters and drilldowns allow users to explore your data from different angles, while calculated fields unlock deeper analysis. Parameters add a final touch of flexibility, letting viewers customise the report to their specific needs. With tools Tableau and Power BI, complex data becomes clear and engaging.
Essential Python. This powerful language excels at data analysis and automation. Libraries like NumPy and Pandas become your foundation for data manipulation and wrangling. Scikit-learn empowers you to build ML models, while SciPy and StatsModels provide a toolkit for in-depth statistical analysis. Python’s ability to interact with APIs and web scrape data expands its reach, and its automation capabilities streamline repetitive tasks. With Essential Python, you have the power to solve complex problems.
Automating the Journey. Data analysts can be masters of efficiency, and their skills translate beautifully into AI. Scripting languages like Ansible and Terraform automate repetitive tasks. Imagine streamlining the process of training and deploying AI models – a skill that directly benefits the AI development pipeline. This proficiency in automation showcases the valuable foundation data analysts provide for building and maintaining AI systems.
Developing ML Expertise. Transitioning from data analysis to AI involves building on your existing skills to develop ML expertise. As a data analyst, you may start with basic predictive models. This knowledge is expanded in AI to include deep learning and advanced ML algorithms. Also, skills in statistical analysis and visualisation help in evaluating the performance of AI models.
Growing Your AI Skills
Becoming an AI engineer requires building on a data analysis foundation to focus on advanced skills such as:
- Deep Learning. Learning frameworks like TensorFlow and PyTorch to build and train neural networks.
- Natural Language Processing (NLP). Techniques for processing and analysing large amounts of natural language data.
- AI Ethics and Fairness. Understanding the ethical implications of AI and ensuring models are fair and unbiased.
- Big Data Technologies. Using tools like Hadoop and Spark for handling large-scale data is essential for AI applications.
The Evolution of a Data Analyst: Career Opportunities
Data analysis is a springboard to AI engineering. Businesses crave talent that bridges the data-AI gap. Your data analyst skills provide the foundation (understanding data sources and transformations) to excel in AI. As you master ML, you can progress to roles like:
- AI Engineer. Works on integrating AI solutions into products and services. They work with AI frameworks like TensorFlow and PyTorch, ensuring that AI models are incorporated into products and services in a fair and unbiased manner.
- ML Engineer. Focuses on designing and implementing ML models. They focus on preprocessing data, evaluating model performance, and collaborating with data scientists and engineers to bring models into production. They need strong programming skills and experience with big data tools and ML algorithms.
- Data Scientist. Bridges the gap between data analysis and AI, often involved in both data preparation and model development. They perform exploratory data analysis, develop predictive models, and collaborate with cross-functional teams to solve complex business problems. Their role requires a comprehensive understanding of both data analysis and ML, as well as strong programming and data visualisation skills.
Conclusion
Hone your data expertise and unlock a future in AI! Mastering in-demand skills like Excel, SQL, Python, and data visualisation tools will equip you to excel as a data analyst. Your data wrangling skills will be invaluable as you explore ML and advanced algorithms. Also, your existing BI knowledge translates seamlessly into building and evaluating AI models. Remember, the data landscape is constantly evolving, so continue to learn to stay at the forefront of this dynamic field. By combining your data skills with a passion for AI, you’ll be well-positioned to tackle complex challenges and shape the future of AI.
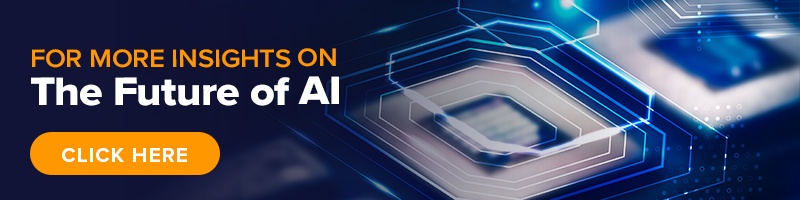
AI tools have become a game-changer for the technology industry, enhancing developer productivity and software quality. Leveraging advanced machine learning models and natural language processing, these tools offer a wide range of capabilities, from code completion to generating entire blocks of code, significantly reducing the cognitive load on developers. AI-powered tools not only accelerate the coding process but also ensure higher code quality and consistency, aligning seamlessly with modern development practices. Organisations are reaping the benefits of these tools, which have transformed the software development lifecycle.
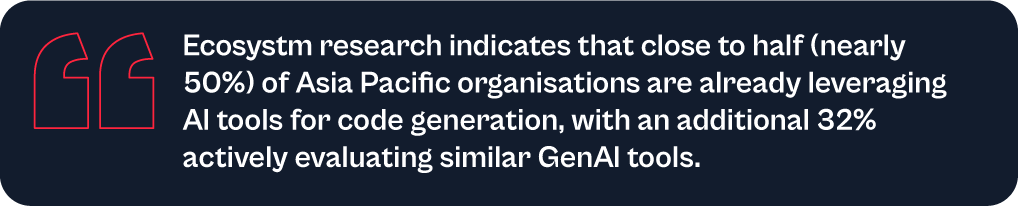
Impact on Developer Productivity
AI tools are becoming an indispensable part of software development owing to their:
- Speed and Efficiency. AI-powered tools provide real-time code suggestions, which dramatically reduces the time developers spend writing boilerplate code and debugging. For example, Tabnine can suggest complete blocks of code based on the comments or a partial code snippet, which accelerates the development process.
- Quality and Accuracy. By analysing vast datasets of code, AI tools can offer not only syntactically correct but also contextually appropriate code suggestions. This capability reduces bugs and improves the overall quality of the software.
- Learning and Collaboration. AI tools also serve as learning aids for developers by exposing them to new or better coding practices and patterns. Novice developers, in particular, can benefit from real-time feedback and examples, accelerating their professional growth. These tools can also help maintain consistency in coding standards across teams, fostering better collaboration.
Advantages of Using AI Tools in Development
- Reduced Time to Market. Faster coding and debugging directly contribute to shorter development cycles, enabling organisations to launch products faster. This reduction in time to market is crucial in today’s competitive business environment where speed often translates to a significant market advantage.
- Cost Efficiency. While there is an upfront cost in integrating these AI tools, the overall return on investment (ROI) is enhanced through the reduced need for extensive manual code reviews, decreased dependency on large development teams, and lower maintenance costs due to improved code quality.
- Scalability and Adaptability. AI tools learn and adapt over time, becoming more efficient and aligned with specific team or project needs. This adaptability ensures that the tools remain effective as the complexity of projects increases or as new technologies emerge.
Deployment Models
The choice between SaaS and on-premises deployment models involves a trade-off between control, cost, and flexibility. Organisations need to consider their specific requirements, including the level of control desired over the infrastructure, sensitivity of the data, compliance needs, and available IT resources. A thorough assessment will guide the decision, ensuring that the deployment model chosen aligns with the organisation’s operational objectives and strategic goals.
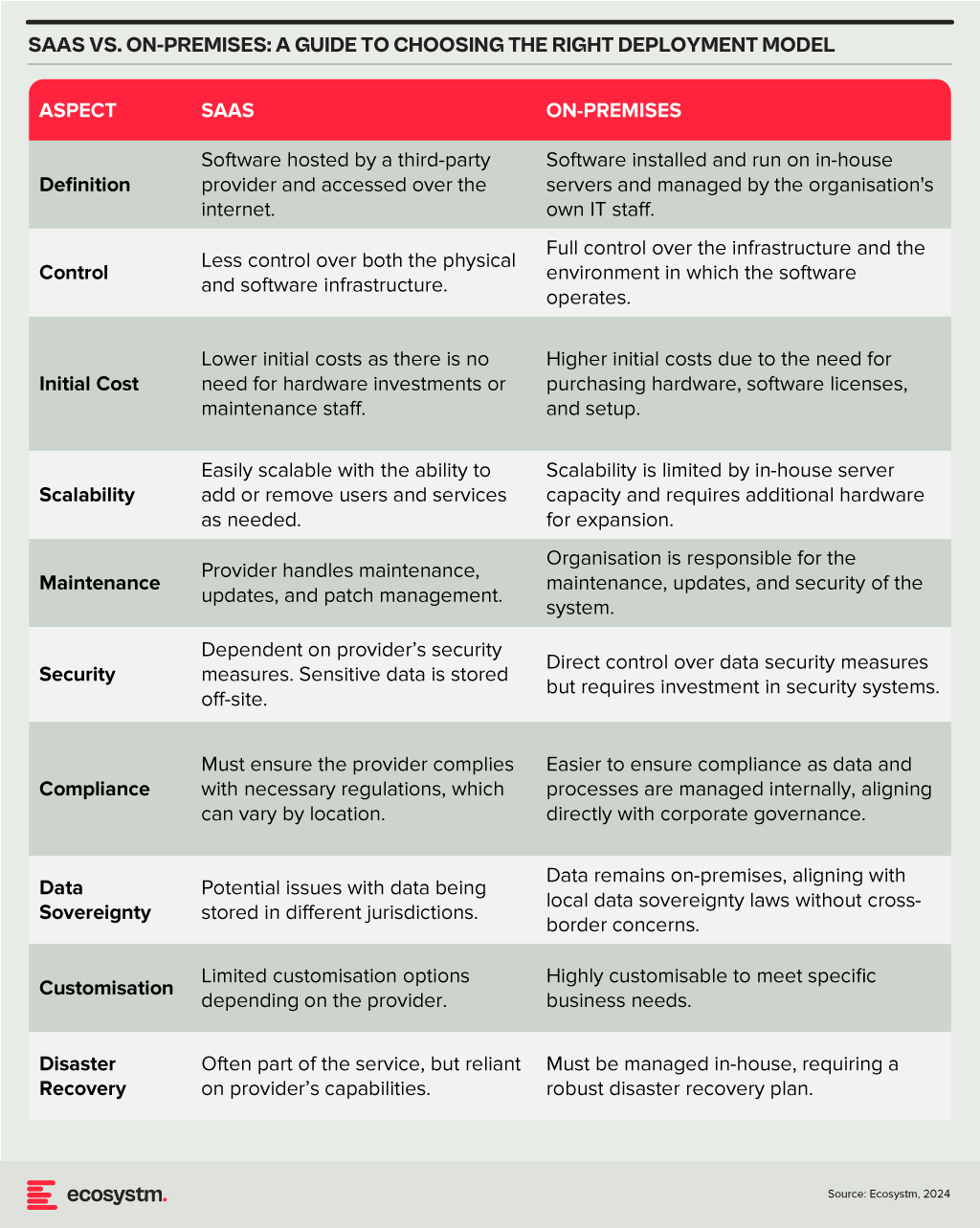
Technology teams must consider challenges such as the reliability of generated code, the potential for generating biased or insecure code, and the dependency on external APIs or services. Proper oversight, regular evaluations, and a balanced integration of AI tools with human oversight are recommended to mitigate these risks.
A Roadmap for AI Integration
The strategic integration of AI tools in software development offers a significant opportunity for companies to achieve a competitive edge. By starting with pilot projects, organisations can assess the impact and utility of AI within specific teams. Encouraging continuous training in AI advancements empowers developers to leverage these tools effectively. Regular audits ensure that AI-generated code adheres to security standards and company policies, while feedback mechanisms facilitate the refinement of tool usage and address any emerging issues.
Technology teams have the opportunity to not only boost operational efficiency but also cultivate a culture of innovation and continuous improvement in their software development practices. As AI technology matures, even more sophisticated tools are expected to emerge, further propelling developer capabilities and software development to new heights.
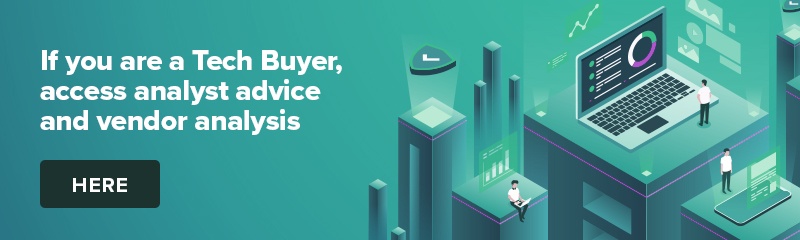
When OpenAI released ChatGPT, it became obvious – and very fast – that we were entering a new era of AI. Every tech company scrambled to release a comparable service or to infuse their products with some form of GenAI. Microsoft, piggybacking on its investment in OpenAI was the fastest to market with impressive text and image generation for the mainstream. Copilot is now embedded across its software, including Microsoft 365, Teams, GitHub, and Dynamics to supercharge the productivity of developers and knowledge workers. However, the race is on – AWS and Google are actively developing their own GenAI capabilities.
AWS Catches Up as Enterprise Gains Importance
Without a consumer-facing AI assistant, AWS was less visible during the early stages of the GenAI boom. They have since rectified this with a USD 4B investment into Anthropic, the makers of Claude. This partnership will benefit both Amazon and Anthropic, bringing the Claude 3 family of models to enterprise customers, hosted on AWS infrastructure.
As GenAI quickly emerges from shadow IT to an enterprise-grade tool, AWS is catching up by capitalising on their position as cloud leader. Many organisations view AWS as a strategic partner, already housing their data, powering critical applications, and providing an environment that developers are accustomed to. The ability to augment models with private data already residing in AWS data repositories will make it an attractive GenAI partner.
AWS has announced the general availability of Amazon Q, their suite of GenAI tools aimed at developers and businesses. Amazon Q Developer expands on what was launched as Code Whisperer last year. It helps developers accelerate the process of building, testing, and troubleshooting code, allowing them to focus on higher-value work. The tool, which can directly integrate with a developer’s chosen IDE, uses NLP to develop new functions, modernise legacy code, write security tests, and explain code.
Amazon Q Business is an AI assistant that can safely ingest an organisation’s internal data and connect with popular applications, such as Amazon S3, Salesforce, Microsoft Exchange, Slack, ServiceNow, and Jira. Access controls can be implemented to ensure data is only shared with authorised users. It leverages AWS’s visualisation tool, QuickSight, to summarise findings. It also integrates directly with applications like Slack, allowing users to query it directly.
Going a step further, Amazon Q Apps (in preview) allows employees to build their own lightweight GenAI apps using natural language. These employee-created apps can then be published to an enterprise’s app library for broader use. This no-code approach to development and deployment is part of a drive to use AI to increase productivity across lines of business.
AWS continues to expand on Bedrock, their managed service providing access to foundational models from companies like Mistral AI, Stability AI, Meta, and Anthropic. The service also allows customers to bring their own model in cases where they have already pre-trained their own LLM. Once a model is selected, organisations can extend its knowledge base using Retrieval-Augmented Generation (RAG) to privately access proprietary data. Models can also be refined over time to improve results and offer personalised experiences for users. Another feature, Agents for Amazon Bedrock, allows multi-step tasks to be performed by invoking APIs or searching knowledge bases.
To address AI safety concerns, Guardrails for Amazon Bedrock is now available to minimise harmful content generation and avoid negative outcomes for users and brands. Contentious topics can be filtered by varying thresholds, and Personally Identifiable Information (PII) can be masked. Enterprise-wide policies can be defined centrally and enforced across multiple Bedrock models.
Google Targeting Creators
Due to the potential impact on their core search business, Google took a measured approach to entering the GenAI field, compared to newer players like OpenAI and Perplexity. The useability of Google’s chatbot, Gemini, has improved significantly since its initial launch under the moniker Bard. Its image generator, however, was pulled earlier this year while it works out how to carefully tread the line between creativity and sensitivity. Based on recent demos though, it plans to target content creators with images (Imagen 3), video generation (Veo), and music (Lyria).
Like Microsoft, Google has seen that GenAI is a natural fit for collaboration and office productivity. Gemini can now assist the sidebar of Workspace apps, like Docs, Sheets, Slides, Drive, Gmail, and Meet. With Google Search already a critical productivity tool for most knowledge workers, it is determined to remain a leader in the GenAI era.
At their recent Cloud Next event, Google announced the Gemini Code Assist, a GenAI-powered development tool that is more robust than its previous offering. Using RAG, it can customise suggestions for developers by accessing an organisation’s private codebase. With a one-million-token large context window, it also has full codebase awareness making it possible to make extensive changes at once.
The Hardware Problem of AI
The demands that GenAI places on compute and memory have created a shortage of AI chips, causing the valuation of GPU giant, NVIDIA, to skyrocket into the trillions of dollars. Though the initial training is most hardware-intensive, its importance will only rise as organisations leverage proprietary data for custom model development. Inferencing is less compute-heavy for early use cases, such as text generation and coding, but will be dwarfed by the needs of image, video, and audio creation.
Realising compute and memory will be a bottleneck, the hyperscalers are looking to solve this constraint by innovating with new chip designs of their own. AWS has custom-built specialised chips – Trainium2 and Inferentia2 – to bring down costs compared to traditional compute instances. Similarly, Microsoft announced the Maia 100, which it developed in conjunction with OpenAI. Google also revealed its 6th-generation tensor processing unit (TPU), Trillium, with significant increase in power efficiency, high bandwidth memory capacity, and peak compute performance.
The Future of the GenAI Landscape
As enterprises gain experience with GenAI, they will look to partner with providers that they can trust. Challenges around data security, governance, lineage, model transparency, and hallucination management will all need to be resolved. Additionally, controlling compute costs will begin to matter as GenAI initiatives start to scale. Enterprises should explore a multi-provider approach and leverage specialised data management vendors to ensure a successful GenAI journey.
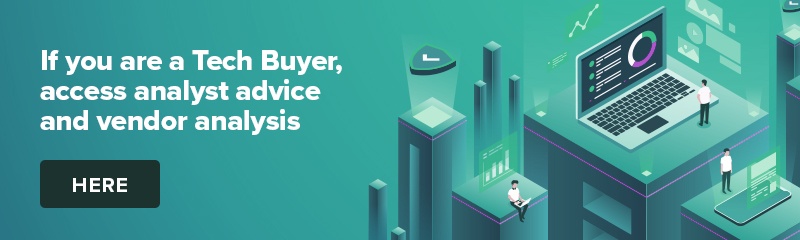
Historically, data scientists have been the linchpins in the world of AI and machine learning, responsible for everything from data collection and curation to model training and validation. However, as the field matures, we’re witnessing a significant shift towards specialisation, particularly in data engineering and the strategic role of Large Language Models (LLMs) in data curation and labelling. The integration of AI into applications is also reshaping the landscape of software development and application design.
The Growth of Embedded AI
AI is being embedded into applications to enhance user experience, optimise operations, and provide insights that were previously inaccessible. For example, natural language processing (NLP) models are being used to power conversational chatbots for customer service, while machine learning algorithms are analysing user behaviour to customise content feeds on social media platforms. These applications leverage AI to perform complex tasks, such as understanding user intent, predicting future actions, or automating decision-making processes, making AI integration a critical component of modern software development.
This shift towards AI-embedded applications is not only changing the nature of the products and services offered but is also transforming the roles of those who build them. Since the traditional developer may not possess extensive AI skills, the role of data scientists is evolving, moving away from data engineering tasks and increasingly towards direct involvement in development processes.
The Role of LLMs in Data Curation
The emergence of LLMs has introduced a novel approach to handling data curation and processing tasks traditionally performed by data scientists. LLMs, with their profound understanding of natural language and ability to generate human-like text, are increasingly being used to automate aspects of data labelling and curation. This not only speeds up the process but also allows data scientists to focus more on strategic tasks such as model architecture design and hyperparameter tuning.
The accuracy of AI models is directly tied to the quality of the data they’re trained on. Incorrectly labelled data or poorly curated datasets can lead to biased outcomes, mispredictions, and ultimately, the failure of AI projects. The role of data engineers and the use of advanced tools like LLMs in ensuring the integrity of data cannot be overstated.
The Impact on Traditional Developers
Traditional software developers have primarily focused on writing code, debugging, and software maintenance, with a clear emphasis on programming languages, algorithms, and software architecture. However, as applications become more AI-driven, there is a growing need for developers to understand and integrate AI models and algorithms into their applications. This requirement presents a challenge for developers who may not have specialised training in AI or data science. This is seeing an increasing demand for upskilling and cross-disciplinary collaboration to bridge the gap between traditional software development and AI integration.
Clear Role Differentiation: Data Engineering and Data Science
In response to this shift, the role of data scientists is expanding beyond the confines of traditional data engineering and data science, to include more direct involvement in the development of applications and the embedding of AI features and functions.
Data engineering has always been a foundational element of the data scientist’s role, and its importance has increased with the surge in data volume, variety, and velocity. Integrating LLMs into the data collection process represents a cutting-edge approach to automating the curation and labelling of data, streamlining the data management process, and significantly enhancing the efficiency of data utilisation for AI and ML projects.
Accurate data labelling and meticulous curation are paramount to developing models that are both reliable and unbiased. Errors in data labelling or poorly curated datasets can lead to models that make inaccurate predictions or, worse, perpetuate biases. The integration of LLMs into data engineering tasks is facilitating a transformation, freeing them from the burdens of manual data labelling and curation. This has led to a more specialised data scientist role that allocates more time and resources to areas that can create greater impact.
The Evolving Role of Data Scientists
Data scientists, with their deep understanding of AI models and algorithms, are increasingly working alongside developers to embed AI capabilities into applications. This collaboration is essential for ensuring that AI models are effectively integrated, optimised for performance, and aligned with the application’s objectives.
- Model Development and Innovation. With the groundwork of data preparation laid by LLMs, data scientists can focus on developing more sophisticated and accurate AI models, exploring new algorithms, and innovating in AI and ML technologies.
- Strategic Insights and Decision Making. Data scientists can spend more time analysing data and extracting valuable insights that can inform business strategies and decision-making processes.
- Cross-disciplinary Collaboration. This shift also enables data scientists to engage more deeply in interdisciplinary collaboration, working closely with other departments to ensure that AI and ML technologies are effectively integrated into broader business processes and objectives.
- AI Feature Design. Data scientists are playing a crucial role in designing AI-driven features of applications, ensuring that the use of AI adds tangible value to the user experience.
- Model Integration and Optimisation. Data scientists are also involved in integrating AI models into the application architecture, optimising them for efficiency and scalability, and ensuring that they perform effectively in production environments.
- Monitoring and Iteration. Once AI models are deployed, data scientists work on monitoring their performance, interpreting outcomes, and making necessary adjustments. This iterative process ensures that AI functionalities continue to meet user needs and adapt to changing data landscapes.
- Research and Continued Learning. Finally, the transformation allows data scientists to dedicate more time to research and continued learning, staying ahead of the rapidly evolving field of AI and ensuring that their skills and knowledge remain cutting-edge.
Conclusion
The integration of AI into applications is leading to a transformation in the roles within the software development ecosystem. As applications become increasingly AI-driven, the distinction between software development and AI model development is blurring. This convergence needs a more collaborative approach, where traditional developers gain AI literacy and data scientists take on more active roles in application development. The evolution of these roles highlights the interdisciplinary nature of building modern AI-embedded applications and underscores the importance of continuous learning and adaptation in the rapidly advancing field of AI.
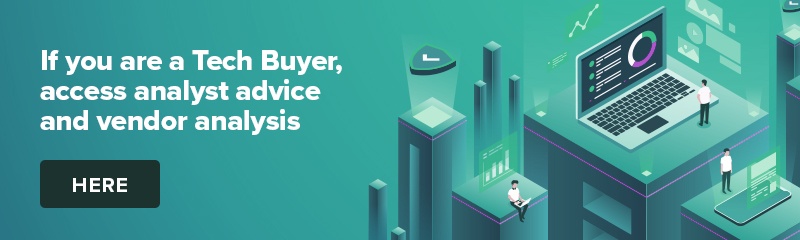
As AI evolves rapidly, the emergence of GenAI technologies such as GPT models has sparked a novel and critical role: prompt engineering. This specialised function is becoming indispensable in optimising the interaction between humans and AI, serving as a bridge that translates human intentions into prompts that guide AI to produce desired outcomes. In this Ecosystm Insight, I will explore the importance of prompt engineering, highlighting its significance, responsibilities, and the impact it has on harnessing AI’s full potential.
Understanding Prompt Engineering
Prompt engineering is an interdisciplinary role that combines elements of linguistics, psychology, computer science, and creative writing. It involves crafting inputs (prompts) that are specifically designed to elicit the most accurate, relevant, and contextually appropriate responses from AI models. This process requires a nuanced understanding of how different models process information, as well as creativity and strategic thinking to manipulate these inputs for optimal results.
As GenAI applications become more integrated across sectors – ranging from creative industries to technical fields – the ability to effectively communicate with AI systems has become a cornerstone of leveraging AI capabilities. Prompt engineers play a crucial role in this scenario, refining the way we interact with AI to enhance productivity, foster innovation, and create solutions that were previously unimaginable.
The Art and Science of Crafting Prompts
Prompt engineering is as much an art as it is a science. It demands a balance between technical understanding of AI models and the creative flair to engage these models in producing novel content. A well-crafted prompt can be the difference between an AI generating generic, irrelevant content and producing work that is insightful, innovative, and tailored to specific needs.
Key responsibilities in prompt engineering include:
- Prompt Optimisation. Fine-tuning prompts to achieve the highest quality output from AI models. This involves understanding the intricacies of model behaviour and leveraging this knowledge to guide the AI towards desired responses.
- Performance Testing and Iteration. Continuously evaluating the effectiveness of different prompts through systematic testing, analysing outcomes, and refining strategies based on empirical data.
- Cross-Functional Collaboration. Engaging with a diverse team of professionals, including data scientists, AI researchers, and domain experts, to ensure that prompts are aligned with project goals and leverage domain-specific knowledge effectively.
- Documentation and Knowledge Sharing. Developing comprehensive guidelines, best practices, and training materials to standardise prompt engineering methodologies within an organisation, facilitating knowledge transfer and consistency in AI interactions.
The Strategic Importance of Prompt Engineering
Effective prompt engineering can significantly enhance the efficiency and outcomes of AI projects. By reducing the need for extensive trial and error, prompt engineers help streamline the development process, saving time and resources. Moreover, their work is vital in mitigating biases and errors in AI-generated content, contributing to the development of responsible and ethical AI solutions.
As AI technologies continue to advance, the role of the prompt engineer will evolve, incorporating new insights from research and practice. The ability to dynamically interact with AI, guiding its creative and analytical processes through precisely engineered prompts, will be a key differentiator in the success of AI applications across industries.
Want to Hire a Prompt Engineer?
Here is a sample job description for a prompt engineer if you think that your organisation will benefit from the role.
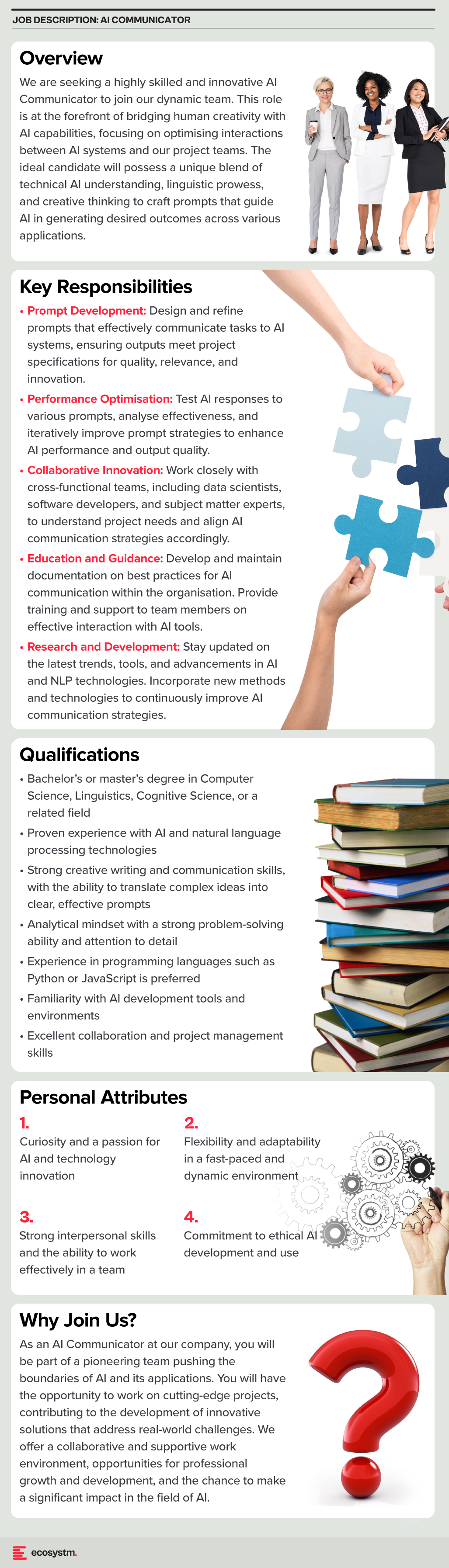
Conclusion
Prompt engineering represents a crucial evolution in the field of AI, addressing the gap between human intention and machine-generated output. As we continue to explore the boundaries of what AI can achieve, the demand for skilled prompt engineers – who can navigate the complex interplay between technology and human language – will grow. Their work not only enhances the practical applications of AI but also pushes the frontier of human-machine collaboration, making them indispensable in the modern AI ecosystem.
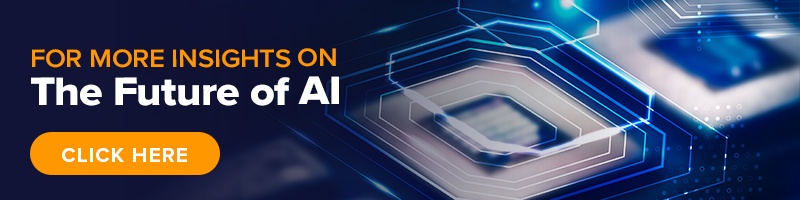
AI has become a business necessity today, catalysing innovation, efficiency, and growth by transforming extensive data into actionable insights, automating tasks, improving decision-making, boosting productivity, and enabling the creation of new products and services.
Generative AI stole the limelight in 2023 given its remarkable advancements and potential to automate various cognitive processes. However, now the real opportunity lies in leveraging this increased focus and attention to shine the AI lens on all business processes and capabilities. As organisations grasp the potential for productivity enhancements, accelerated operations, improved customer outcomes, and enhanced business performance, investment in AI capabilities is expected to surge.
In this eBook, Ecosystm VP Research Tim Sheedy and Vinod Bijlani and Aman Deep from HPE APAC share their insights on why it is crucial to establish tailored AI capabilities within the organisation.
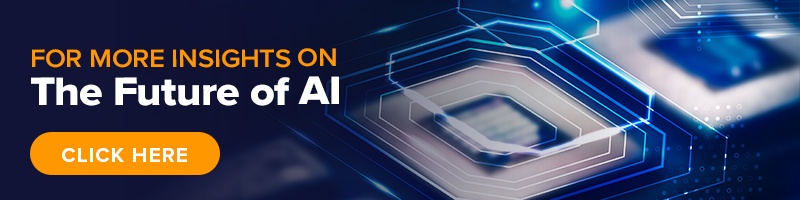
In my last Ecosystm Insights, I spoke about the implications of the shift from Predictive AI to Generative AI on ROI considerations of AI deployments. However, from my discussions with colleagues and technology leaders it became clear that there is a need to define and distinguish between Predictive AI and Generative AI better.
Predictive AI analyses historical data to predict future outcomes, crucial for informed decision-making and strategic planning. Generative AI unlocks new avenues for innovation by creating novel data and content. Organisations need both – Predictive AI for enhancing operational efficiencies and forecasting capabilities and Generative AI to drive innovation; create new products, services, and experiences; and solve complex problems in unprecedented ways.
This guide aims to demystify these categories, providing clarity on their differences, applications, and examples of the algorithms they use.
Predictive AI: Forecasting the Future
Predictive AI is extensively used in fields such as finance, marketing, healthcare and more. The core idea is to identify patterns or trends in data that can inform future decisions. Predictive AI relies on statistical, machine learning, and deep learning models to forecast outcomes.
Key Algorithms in Predictive AI
- Regression Analysis. Linear and logistic regression are foundational tools for predicting a continuous or categorical outcome based on one or more predictor variables.
- Decision Trees. These models use a tree-like graph of decisions and their possible consequences, including chance event outcomes, resource costs and utility.
- Random Forest (RF). An ensemble learning method that operates by constructing a multitude of decision trees at training time to improve predictive accuracy and control over-fitting.
- Gradient Boosting Machines (GBM). Another ensemble technique that builds models sequentially, each new model correcting errors made by the previous ones, used for both regression and classification tasks.
- Support Vector Machines (SVM). A supervised machine learning model that uses classification algorithms for two-group classification problems.
Generative AI: Creating New Data
Generative AI, on the other hand, focuses on generating new data that is similar but not identical to the data it has been trained on. This can include anything from images, text, and videos to synthetic data for training other AI models. GenAI is particularly known for its ability to innovate, create, and simulate in ways that predictive AI cannot.
Key Algorithms in Generative AI
- Generative Adversarial Networks (GANs). Comprising two networks – a generator and a discriminator – GANs are trained to generate new data with the same statistics as the training set.
- Variational Autoencoders (VAEs). These are generative algorithms that use neural networks for encoding inputs into a latent space representation, then reconstructing the input data based on this representation.
- Transformer Models. Originally designed for natural language processing (NLP) tasks, transformers can be adapted for generative purposes, as seen in models like GPT (Generative Pre-trained Transformer), which can generate coherent and contextually relevant text based on a given prompt.
Comparing Predictive and Generative AI
The fundamental difference between the two lies in their primary objectives: Predictive AI aims to forecast future outcomes based on past data, while Generative AI aims to create new, original data that mimics the input data in some form.
The differences become clearer when we look at these examples.
Predictive AI Examples
- Supply Chain Management. Analyses historical supply chain data to forecast demand, manage inventory levels, reduces costs and improve delivery times.
- Healthcare. Analysing patient records to predict disease outbreaks or the likelihood of a disease in individual patients.
- Predictive Maintenance. Analyse historical and real-time data and preemptively identifies system failures or network issues, enhancing infrastructure reliability and operational efficiency.
- Finance. Using historical stock prices and indicators to predict future market trends.
Generative AI Examples
- Content Creation. Generating realistic images or art from textual descriptions using GANs.
- Text Generation. Creating coherent and contextually relevant articles, stories, or conversational responses using transformer models like GPT-3.
- Chatbots and Virtual Assistants. Advanced GenAI models are enhancing chatbots and virtual assistants, making them more realistic.
- Automated Code Generation. By the use of natural language descriptions to generate programming code and scripts, to significantly speed up software development processes.
Conclusion
Organisations that exclusively focus on Generative AI may find themselves at the forefront of innovation, by leveraging its ability to create new content, simulate scenarios, and generate original data. However, solely relying on Generative AI without integrating Predictive AI’s capabilities may limit an organisation’s ability to make data-driven decisions and forecasts based on historical data. This could lead to missed opportunities to optimise operations, mitigate risks, and accurately plan for future trends and demands. Predictive AI’s strength lies in analysing past and present data to inform strategic decision-making, crucial for long-term sustainability and operational efficiency.
It is essential for companies to adopt a dual-strategy approach in their AI efforts. Together, these AI paradigms can significantly amplify an organisation’s ability to adapt, innovate, and compete in rapidly changing markets.
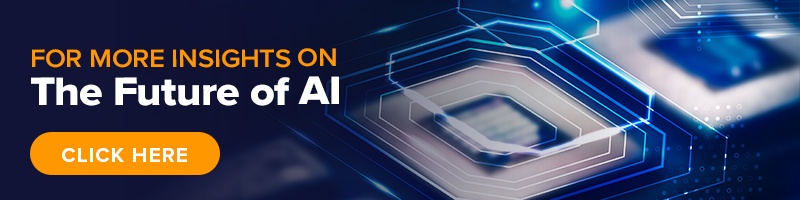
Google recently extended its Generative AI, Bard, to include coding in more than 20 programming languages, including C++, Go, Java, Javascript, and Python. The search giant has been eager to respond to last year’s launch of ChatGPT but as the trusted incumbent, it has naturally been hesitant to move too quickly. The tendency for large language models (LLMs) to produce controversial and erroneous outputs has the potential to tarnish established brands. Google Bard was released in March in the US and the UK as an LLM but lacked the coding ability of OpenAI’s ChatGPT and Microsoft’s Bing Chat.
Bard’s new features include code generation, optimisation, debugging, and explanation. Using natural language processing (NLP), users can explain their requirements to the AI and ask it to generate code that can then be exported to an integrated development environment (IDE) or executed directly in the browser with Google Colab. Similarly, users can request Bard to debug already existing code, explain code snippets, or optimise code to improve performance.
Google continues to refer to Bard as an experiment and highlights that as is the case with generated text, code produced by the AI may not function as expected. Regardless, the new functionality will be useful for both beginner and experienced developers. Those learning to code can use Generative AI to debug and explain their mistakes or write simple programs. More experienced developers can use the tool to perform lower-value work, such as commenting on code, or scaffolding to identify potential problems.
GitHub Copilot X to Face Competition
While the ability for Bard, Bing, and ChatGPT to generate code is one of their most important use cases, developers are now demanding AI directly in their IDEs.
In March, Microsoft made one of its most significant announcements of the year when it demonstrated GitHub Copilot X, which embeds GPT-4 in the development environment. Earlier this year, Microsoft invested $10 billion into OpenAI to add to the $1 billion from 2019, cementing the partnership between the two AI heavyweights. Among other benefits, this agreement makes Azure the exclusive cloud provider to OpenAI and provides Microsoft with the opportunity to enhance its software with AI co-pilots.
Currently, under technical preview, when Copilot X eventually launches, it will integrate into Visual Studio — Microsoft’s IDE. Presented as a sidebar or chat directly in the IDE, Copilot X will be able to generate, explain, and comment on code, debug, write unit tests, and identify vulnerabilities. The “Hey, GitHub” functionality will allow users to chat using voice, suitable for mobile users or more natural interaction on a desktop.
Not to be outdone by its cloud rivals, in April, AWS announced the general availability of what it describes as a real-time AI coding companion. Amazon CodeWhisperer, integrates with a range of IDEs, namely Visual Studio Code, IntelliJ IDEA, CLion, GoLand, WebStorm, Rider, PhpStorm, PyCharm, RubyMine, and DataGrip, or natively in AWS Cloud9 and AWS Lambda console. While the preview worked for Python, Java, JavaScript, TypeScript, and C#, the general release extends support for most languages. Amazon’s key differentiation is that it is available for free to individual users, while GitHub Copilot is currently subscription-based with exceptions only for teachers, students, and maintainers of open-source projects.
The Next Step: Generative AI in Security
The next battleground for Generative AI will be assisting overworked security analysts. Currently, some of the greatest challenges that Security Operations Centres (SOCs) face are being understaffed and overwhelmed with the number of alerts. Security vendors, such as IBM and Securonix, have already deployed automation to reduce alert noise and help analysts prioritise tasks to avoid responding to false threats.
Google recently introduced Sec-PaLM and Microsoft announced Security Copilot, bringing the power of Generative AI to the SOC. These tools will help analysts interact conversationally with their threat management systems and will explain alerts in natural language. How effective these tools will be is yet to be seen, considering hallucinations in security is far riskier than writing an essay with ChatGPT.
The Future of AI Code Generators
Although GitHub Copilot and Amazon CodeWhisperer had already launched with limited feature sets, it was the release of ChatGPT last year that ushered in a new era in AI code generation. There is now a race between the cloud hyperscalers to win over developers and to provide AI that supports other functions, such as security.
Despite fears that AI will replace humans, in their current state it is more likely that they will be used as tools to augment developers. Although AI and automated testing reduce the burden on the already stretched workforce, humans will continue to be in demand to ensure code is secure and satisfies requirements. A likely scenario is that with coding becoming simpler, rather than the number of developers shrinking, the volume and quality of code written will increase. AI will generate a new wave of citizen developers able to work on projects that would previously have been impossible to start. This may, in turn, increase demand for developers to build on these proofs-of-concept.
How the Generative AI landscape evolves over the next year will be interesting. In a recent interview, OpenAI’s founder, Sam Altman, explained that the non-profit model it initially pursued is not feasible, necessitating the launch of a capped-for-profit subsidiary. The company retains its values, however, focusing on advancing AI responsibly and transparently with public consultation. The appearance of Microsoft, Google, and AWS will undoubtedly change the market dynamics and may force OpenAI to at least reconsider its approach once again.
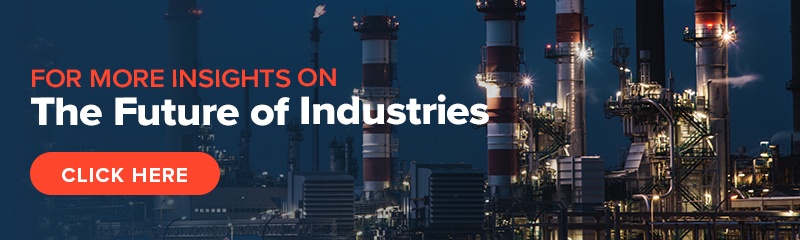