Earlier in the year, Microsoft unveiled its vision for Copilot, a digital companion that aims to provide a unified user experience across Bing, Edge, Microsoft 365, and Windows. This vision includes a consistent user experience. The rollout began with Windows in September and expanded to Microsoft 365 Copilot for enterprise customers this month.
Many organisations across Asia Pacific will soon face the question on whether to invest in Microsoft 365 Copilot – despite its current limitations in supporting all regional languages. Copilot is currently supported in English (US, GB, AU, CA, IN), Japanese, and Chinese Simplified. Microsoft plans to support more languages such as Arabic, Chinese Traditional, Korean and Thai over the first half of 2024. There are still several languages used across Asia Pacific that will not be supported until at least the second half of 2024 or later.
Access to Microsoft 365 Copilot comes with certain prerequisites. Organisations need to have either a Microsoft 365 E3 or E5 license and an Azure Active Directory account. F3 licenses do not currently have access to 365 Copilot. For E3 license holders the cost per user for adding Copilot would nearly double – so it is a significant extra spend and will need to deliver measurable and tangible benefits and a strong business case. It is doubtful whether most organisations will be able to justify this extra spend.
However, Copilot has the potential to significantly enhance the productivity of knowledge workers, saving them many hours each week, with hundreds of use cases already emerging for different industries and user profiles. Microsoft is offering a plethora of information on how to best adopt, deploy, and use Copilot. The key focus when building a business case should revolve around how knowledge workers will use this extra time.
Maximising Copilot Integration: Steps to Drive Adoption and Enhance Productivity
Identifying use cases, building the business proposal, and securing funding for Copilot is only half the battle. Driving the change and ensuring all relevant employees use the new processes will be significantly harder. Consider how employees currently use their productivity tools compared to 15 years ago, with many still relying on the same features and capabilities in their Office suites as they did in earlier versions. In cases where new features were embraced, it typically occurred because knowledge workers didn’t have to make any additional efforts to incorporate them, such as the auto-type ahead functions in email or the seamless integration of Teams calls.
The ability of your organisation to seamlessly integrate Copilot into daily workflows, optimising productivity and efficiency while harnessing AI-generated data and insights for decision-making will be of paramount importance. It will be equally important to be watchful to mitigate potential risks associated with an over-reliance on AI without sufficient oversight.
Implementing Copilot will require some essential steps:
- Training and onboarding. Provide comprehensive training to employees on how to use Copilot’s features within Microsoft 365 applications.
- Integration into daily tasks. Encourage employees to use Copilot for drafting emails, documents, and generating meeting notes to familiarise them with its capabilities.
- Customisation. Tailor Copilot’s settings and suggestions to align with company-specific needs and workflows.
- Automation. Create bots, templates, integrations, and other automation functions for multiple use cases. For example, when users first log onto their PC, they could get a summary of missed emails, chats – without the need to request it.
- Feedback loop. Implement a feedback mechanism to monitor how Copilot is used and to make adjustments based on user experiences.
- Evaluating effectiveness. Gauge how Copilot’s features are enhancing productivity regularly and adjust usage strategies accordingly. Focus on the increased productivity – what knowledge workers now achieve with the time made available by Copilot.
Changing the behaviours of knowledge workers can be challenging – particularly for basic processes that they have been using for years or even decades. Knowledge of use cases and opportunities for Copilot will not just filter across the organisation. Implementing formal training and educational programs and backing them up with refresher courses is important to ensure compliance and productivity gains.
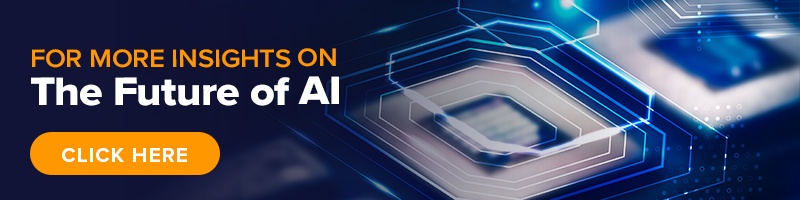