In this Insight, guest author Anirban Mukherjee lists out the key challenges of AI adoption in traditional organisations – and how best to mitigate these challenges. “I am by no means suggesting that traditional companies avoid or delay adopting AI. That would be akin to asking a factory to keep using only steam as power, even as electrification came in during early 20th century! But organisations need to have a pragmatic strategy around what will undoubtedly be a big, but necessary, transition.”
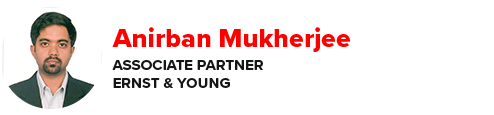
After years of evangelising digital adoption, I have more of a nuanced stance today – supporting a prudent strategy, especially where the organisation’s internal capabilities/technology maturity is in question. I still see many traditional organisations burning budgets in AI adoption programs with low success rates, simply because of poor choices driven by misplaced expectations. Without going into the obvious reasons for over-exuberance (media-hype, mis-selling, FOMO, irrational valuations – the list goes on), here are few patterns that can be detected in those organisations that have succeeded getting value – and gloriously so!
Data-driven decision-making is a cultural change. Most traditional organisations have a point person/role accountable for any important decision, whose “neck is on the line”. For these organisations to change over to trusting AI decisions (with its characteristic opacity, and stochastic nature of recommendations) is often a leap too far.
Work on your change management, but more crucially, strategically choose business/process decision points (aka use-cases) to acceptably AI-enable.
Technical choice of ML modeling needs business judgement too. The more flexible non-linear models that increase prediction accuracy, invariably suffer from lower interpretability – and may be a poor choice in many business contexts. Depending upon business data volumes and accuracy, model bias-variance tradeoffs need to be made. Assessing model accuracy and its thresholds (false-positive-false-negative trade-offs) are similarly nuanced. All this implies that organisation’s domain knowledge needs to merge well with data science design. A pragmatic approach would be to not try to be cutting-edge.
Look to use proven foundational model-platforms – such as those for NLP, visual analytics – for first use cases. Also note that not every problem needs AI; a lot can be sorted through traditional programming (“if-then automation”) and should be. The dirty secret of the industry is that the power of a lot of products marketed as “AI-powered” is mostly traditional logic, under the hood!
In getting results from AI, most often “better data trumps better models”. Practically, this means that organisations need to spend more on data engineering effort, than on data science effort. The CDO/CIO organisation needs to build the right balance of data competencies and tools.
Get the data readiness programs started – yesterday! While the focus of data scientists is often on training an AI model, deployment of the trained model online is a whole other level of technical challenge (particularly when it comes to IT-OT and real-time integrations).
It takes time to adopt AI in traditional organisations. Building up training data and model accuracy is a slow process. Organisational changes take time – and then you have to add considerations such as data standardisation; hygiene and integration programs; and the new attention required to build capabilities in AIOps, AI adoption and governance.
Typically plan for 3 years – monitor progress and steer every 6 months. Be ready to kill “zombie” projects along the way. Train the executive team – not to code, but to understand the technology’s capabilities and limitations. This will ensure better informed buyers/consumers and help drive adoption within the organisation.
I am by no means suggesting that traditional companies avoid or delay adopting AI. That would be akin to asking a factory to keep using only steam as power, even as electrification came in during early 20th century! But organisations need to have a pragmatic strategy around what will undoubtedly be a big, but necessary, transition.
These opinions are personal (and may change with time), but definitely informed through a decade of involvement in such journeys. It is not too early for any organisation to start – results are beginning to show for those who started earlier, and we know what they got right (and wrong).
I would love to hear your views, or even engage with you on your journey!
The views and opinions mentioned in the article are personal.
Anirban Mukherjee has more than 25 years of experience in operations excellence and technology consulting across the globe, having led transformations in Energy, Engineering, and Automotive majors. Over the last decade, he has focused on Smart Manufacturing/Industry 4.0 solutions that integrate cutting-edge digital into existing operations.
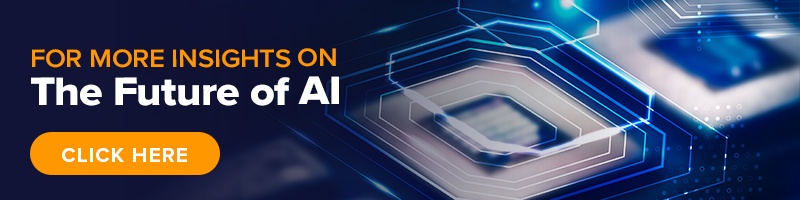
Last week I wrote about the need to remove hype from reality when it comes to AI. But what will ensure that your AI projects succeed?
It is quite obvious that success is determined by human aspects rather than technological factors. We have identified four key organisational actions that enable successful AI implementation at scale (Figure 1).
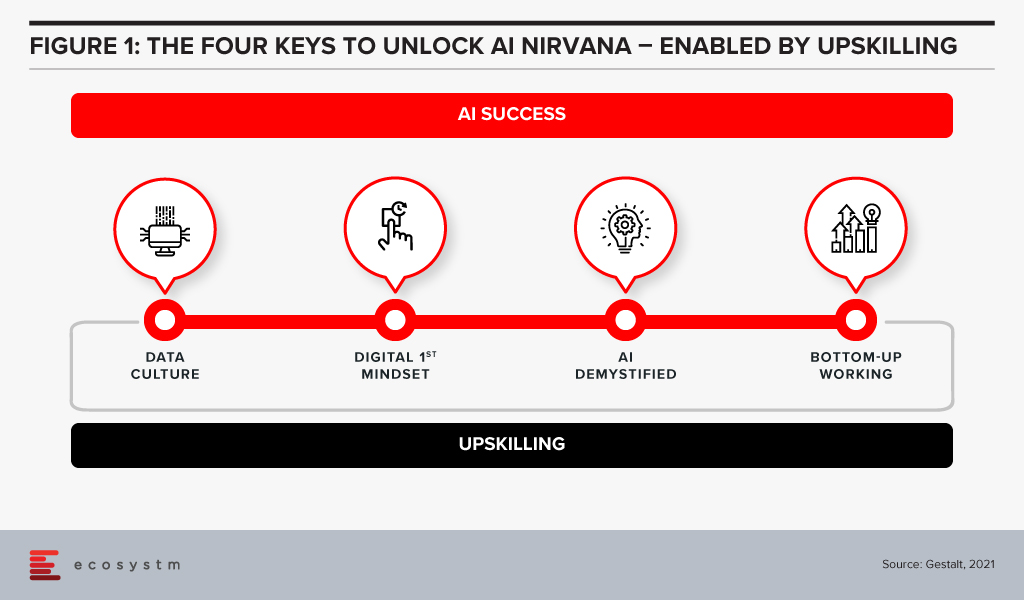
#1 Establish a Data Culture
The traditional focus for companies has been on ensuring access to good, clean data sets and the proper use of that data. Ecosystm research shows that only 28% of organisations focused on customer service, also focus on creating a data-driven organisational culture. But our experience has shown that culture is more critical than having the data. Does the organisation have a culture of using data to drive decisions? Does every level of the organisation understand and use data insights to do their day-to-day jobs? Is decision-making data-driven and decentralised, needing to be escalated only when there is ambiguity or need for strategic clarity? Do business teams push for new data sources when they are not able to get the insights they need?
Without this kind of culture, it may be possible to implement individual pieces of automation in a specific area or process, applying brute force to see it through. In order to transform the business and truly extract the power of AI, we advise organisations to build a culture of data-driven decision-making first. That organisational mindset, will make you capable implementing AI at scale. Focusing on changing the organisational culture will deliver greater returns than trying to implement piecemeal AI projects – even in the short to mid-term.
#2 Ingrain a Digital-First Mindset
Assuming a firm has passed the data culture hurdle, it needs to consider whether it has adopted a digital-first mindset. AI is one of many technologies that impact businesses, along with AR/VR, IoT, 5G, cloud and Blockchain to name a few. Today’s environment requires firms to be capable of utilising a variety of these technologies – often together – and possessing a workforce capable of using these digital tools.
A workforce with the digital-first mindset looks for a digital solution to problems wherever appropriate. They have a good understanding of digital technologies relevant to their space and understand key digital methodologies – such as Customer 360 to deliver a truly superior customer experience or Agile methodologies to successfully manage AI at scale.
AI needs business managers at the operational levels to work with IT or AI tech teams to pinpoint processes that are right for AI. They need to make an estimation based on historical data of what specific problems require an AI solution. This is enabled by the digital-first mindset.
#3 Demystify AI
The next step is to get business leaders, functional leaders, and business operational teams – not just those who work with AI – to acquire a basic understanding of AI.
They do not need to learn the intricacies of programming or how to create neural networks or anything nearly as technical in nature. However, all levels from the leadership down should have a solid understanding of what AI can do, the basics of how it works, how the process of training data results in improved outcomes and so on. They need to understand the continuous learning nature of AI solutions, getting better over time. While AI tools may recommend an answer, human insight is often needed to make a correct decision off this recommendation.

#4 Drive Implementation Bottom-Up
AI projects need alignment, objectives, strategy – and leadership and executive buy-in. But a very important aspect of an AI-driven organisation that is able to build scalable AI, is letting projects run bottom up.
As an example, a reputed Life Sciences company embarked on a multi-year AI project to improve productivity. They wanted to use NLP, Discovery, Cognitive Assist and ML to augment clinical proficiency of doctors and expected significant benefits in drug discovery and clinical trials by leveraging the immense dataset that was built over the last 20 years.
The company ran this like any other transformation project, with a central program management team taking the lead with the help of an AI Centre of Competency. These two teams developed a compelling business case, and identified initial pilots aligned with the long-term objectives of the program. However, after 18 months, they had very few tangible outcomes. Everyone including doctors, research scientists, technicians, and administrators, who participated in the program had their own interpretation of what AI was not able to do.
Discussion revealed that the doctors and researchers felt that they were training AI to replace themselves. Seeing a tool trying to mimic the same access and understanding of numerous documents baffled them at best. They were not ready to work with AI programs step-by-step to help AI tools learn and discover new insights.
At this point, we suggested approaching the project bottom-up – wherein the participating teams would decide specific projects to take up. This developed a culture where teams collaborated as well as competed with each other, to find new ways to use AI. Employees were shown a roadmap of how their jobs would be enhanced by offloading routine decisions to AI. They were shown that AI tools augment the employees’ cognitive capabilities and made them more effective.
The team working on critical trials found these tools extremely useful and were able to collaborate with other organisations specialising in similar trials. They created the metadata and used ML algorithms to discover new insights. Working bottom-up led to a very successful AI deployment.
We have seen time and again that while leadership may set the strategy and objectives, it is best to let the teams work bottom-up to come up with the projects to implement.
#5 Invest in Upskilling
The four “keys” are important to build an AI-powered, future-proof enterprise. They are all human related – and when they come together to work as a winning formula is when organisations invest in upskilling. Upskilling is the common glue and each factor requires specific kinds of upskilling (Figure 2).
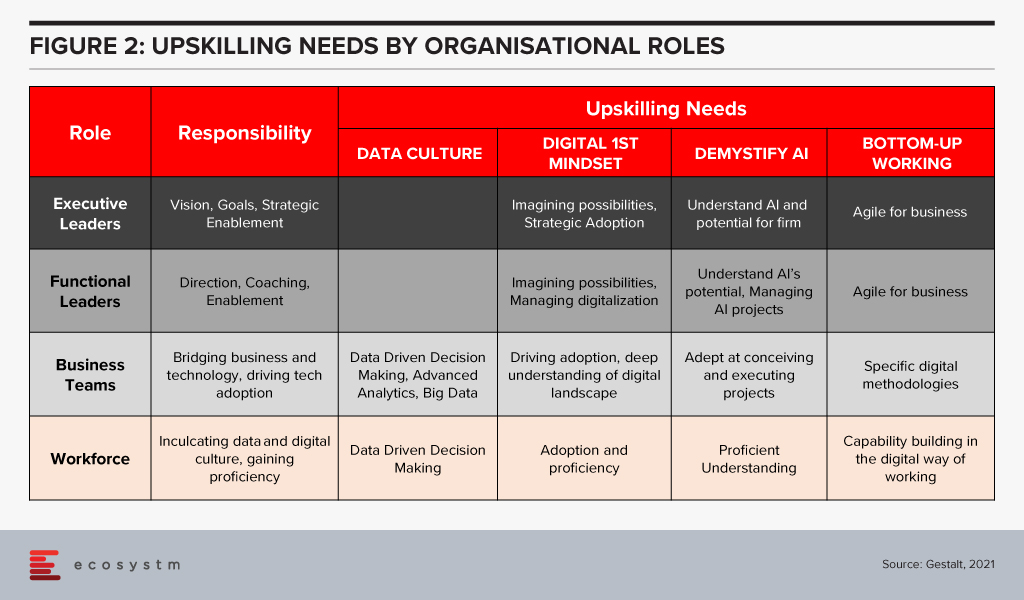
Upskilling needs vary by organisational level and the key being addressed. The bottom line is that upskilling is a universal requirement for driving AI at scale, successfully. And many organisations are realising it fast – Bosch and DBS Bank are some of the notable examples.
How much is your organisation invested in upskilling for AI implementation at scale? Share your stories in the comment box below.
Written with contributions from Ravi Pattamatta and Ratnesh Prasad
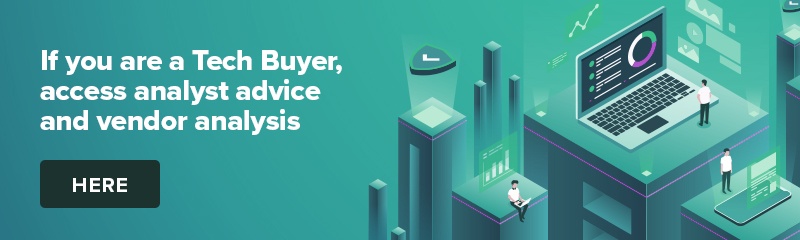
SAS announced that it has acquired Boemska, a provider of low-code development tools and analytics workload management software. The small, privately held company is UK-based with an R&D centre in Serbia. The acquisition will be integrated into SAS Viya, its cloud-native platform, which includes containerised analytics and machine learning offerings. Terms of the deal have not been disclosed.
A SAS silver partner, Boemska has wins in Health, Finance, and Travel. Most of its reference clients are based in Europe in addition to a small number in the US and South Africa. Boemska has two primary software offerings – Enterprise Session Monitor (ESM) and AppFactory. Additionally, it delivers cloud migration, performance diagnostics, and application development services.
Boemska Capabilities
Boemska ESM provides visibility into performance and cost management of analytics workloads. The product enables self-service root cause analysis for developers, monitoring and batch schedule optimisation for administrators, and departmental cost allocation of cloud resources. ESM manages SAS, R, and Python workloads and is compatible with workload management platforms from the likes of IBM and BMC. Boemska shipped an updated version of ESM in 2020 to improve the UI and ensure support for SAS Viya. At the time, it announced that its development team had doubled in the preceding 12 months, suggesting a trajectory of growth.
AppFactory is a low-code development platform for data scientists and data engineers using SAS, which generates JavaScript for front-end developers along with data transport, authentication, and exception handling. SAS emphasises the portability of apps that can be created and run on mobile and IoT devices. Examples provided include machine learning and event alerts in healthcare wearables, video-based defect identification in Manufacturing, and drone-based asset monitoring in Utilities. Boemska states that its low-code offering seeks to bridge the “last mile of analytics” by putting insights into the hands of decision-makers.
SAS Focuses on Cloud-Native Analytics and AI
SAS launched Viya 4.0 in mid-2020, a major step in its vision to become a provider of cloud-native analytics and machine learning solutions. The platform includes offerings, such as Visual Analytics, Visual Statistics, Visual Machine Learning, and Visual Data Science packaged in containers and orchestrated by Kubernetes. Microsoft Azure has become its preferred cloud partner, assisting in developing SAS Cloud, hosted from data centres in the US, Brazil, Australia, and newly launched facilities in Germany and the UK. Viya managed services are also available from Azure regions. AWS and Google Cloud are expected to make the leap to Viya 4.0 from version 3.5 soon. As part of its cloud-native strategy, SAS now offers three tiers for software updates – bi-annual, monthly, or immediately after release.
Ecosystm Comment
The major overhaul of SAS Viya is part of the vendor’s USD 1B investment into AI over three years from 2019-2021. The platform includes a heavy emphasis on NLP, machine learning, and computer vision. The integration of Boemska’s low-code development offering into Viya will allow SAS clients to extract greater value from AI by quickly embedding it in mobile and enterprise applications. The converging trends of citizen developers and data literacy suggest SAS has selected the right path for the future.
Download Ecosystm Predicts: The Top 5 AI & Automation Trends for 2021
Download Ecosystm’s complimentary report detailing the top 5 AI trends in 2021 and what innovations they bring to the table that IT leaders should monitor. Create your free account to access all the Ecosystm Predictions for 2021, and many other reports, on the Ecosystm platform
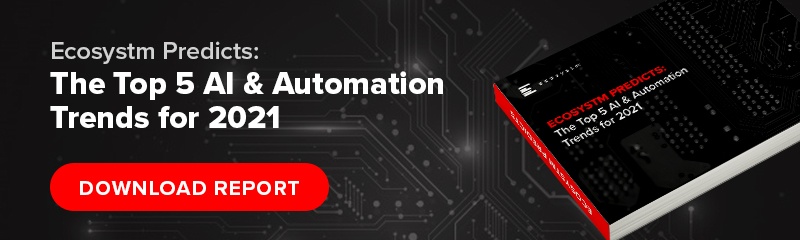
Organisations are finding that the ways to do work and conduct business are evolving rapidly. It is evident that we cannot use the perspectives from the past as a guide to the future. As a consequence both leaders and employees are discovering and adapting both their work and their expectations from it. In general, while job security concerns still command a big mindshare, the simpler productivity measures are evolving to more nuanced wellness measures. This puts demands on the CHRO and the leadership team to think about company, customer and people strategy as one holistic way of working and doing business.
Organisations will have to re-think their people and technology to evolve their Future of Work policies and strategise their Future of Talent. There are multiple dimensions that will require attention.
Hybrid is Becoming Mainstream
It is clear that hybrid workplaces are here to stay. Ecosystm research finds that in 2021 BFSI organisations will use more collaboration tools and platforms, and virtual meetings (Figure 1). Nearly 40% expect more employees to work from home, but only about a quarter of organisations are looking to reduce their physical workspaces. Organisations will give more choice to employees in the location of their work – and employees will choose to work from where they are more productive. The Hybrid model will be more mainstream than it has been in the last few months.
Companies are coming to terms with the fact that there is no single answer to operating in the new world. Experimentation and learnings are continuously captured to create the right workforce and workplace model that works best. Agility both in terms of being able to undersand the market as well as quickly adapt is becoming quite important. Thus being able to use different models and ways of working at the same time is the new norm.
Technology and Talent are Core
Talent and tech are the two core pillars that companies need to look at to be successful against their competition. It is becoming imperative to create synergy between the two to deliver a superior value proposition to customers. Companies that are able to bring the customer and employee experience journeys together will be able to create better value. HR tech stacks need to evolve to be more deliberate in the way they link the employee experience, customer experience, and the culture of the organisation. That’s how the Employee Value Proposition (EVP) comes to life on a day-to-day basis to the employers. With evolving work models, the tech stack is a key EVP pillar.
Governments will also need to partner with industry to make such talent available. Singapore is rolling out a new “Tech.Pass” to support the entry of up to 500 proven founders, leaders and experts from top tech companies into Singapore. Its an extension of the Tech@SG program launched in 2019, to provide fast-growing companies greater assurance and access to the talent they need. The EDB will administer the pass, supported by the Ministry of Manpower.
Attracting the Right Talent
Talent has always been difficult to find. Even with globalisation, significant investment of time and resources is needed to find and relocate talent to the right geography. In many instances this was not possible given the preferences of the candidates and/or the hiring managers. COVID-19 has changed this drastically. Remote working and distributed teams have become acceptable. With limitations on immigration and travel for work, there is a lot more openness to finding and hiring talent from outside the traditional talent pool.
However it is not as simple as it seems. The cost per applicant (CPA) – the cost to convert a job seeker to a job applicant – had been averaging US$11-12 throughout 2019 according to recruiting benchmark data from programmatic recruitment advertising provider, Appcast. But, the impact of COVID-19 saw the CPA reach US$19 in June – a 60% increase. I expect that finding right talent is going to be a “needle in a haystack” issue. But this is only one side of the coin – the other aspect is that the talent profile needed to be successful in roles that are all remote or hybrid is also significantly different from what it was before. Companies need to pay special attention to what kind of people they would like to hire in these new roles. Without this due consideration it is very likely that there would be difficulty in on-boarding and making these new hires successful within the organisation.
Automation Augmentation and Skills
The pace at which companies are choosing to automate or apply AI is increasing. This is changing the work patterns and job requirements for many roles within the industry. According to the BCG China AI study on the financial sector 23% of the roles will be replaced by AI by 2027. The roles that will not be replaced will need a higher degree of soft skills, critical thinking and creativity. However, automation is not the endgame. Firms that go ahead with automation without considering the implications on the business process, and the skills and roles it impacts will end up disrupting the business and customer experience. Firms will have to really design their customer journeys, their business processes along with roles and capabilities needed. Job redesign and reskilling will be key to ensuring a great customer experience
Analytics is Inadequate Without the Right Culture
Data-driven decision-making as well as modelling is known to add value to business. We have great examples of analytics and data modelling being used successfully in Attrition, Recruitment, Talent Analytics, Engagement and Employee Experience. The next evolution is already underway with advanced analytics, sentiment analysis, organisation network analysis and natural language processing (NLP) being used to draw better insights and make people strategies predictive. Being able to use effective data models to predict and and draw insights will be a key success factor for leadership teams. Data and bots do not drive engagement and alignment to purpose – leaders do. Working to promote transparency of data insights and decisions, for faster response, to champion diversity, and give everyone a voice through inclusion will lead to better co-creation, faster innovation and an overall market agility.
Creating a Synergy
We are seeing a number of resets to what we used to know, believe and think about the ways of working. It is a good time to rethink what we believe about the customer, business talent and tech. Just like customer experience is not just about good sales skills or customer service – the employee experience and role of Talent is also evolving rapidly. As companies experiment with work models, technology and work environment, there will a need to constantly recalibrate business models, job roles, job technology and skills. With this will come the challenge of melding the pieces together within the context of the entire business without falling into the trap of siloed thinking. Only by bringing together businesses processes, talent, capability evolution, culture and digital platforms together as one coherent ecosystem can firms create a winning formula to create a competitive edge.
Singapore FinTech Festival 2020: Talent Summit
For more insights, attend the Singapore FinTech Festival 2020: Infrastructure Summit which will cover topics on Founders success and failure stories, pandemic impact on founders and talent development, upskilling and reskilling for the future of work.
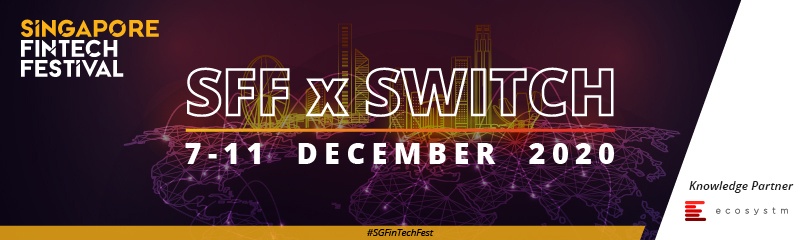
In 2020, much of the focus for organisations were on business continuity, and on empowering their employees to work remotely. Their primary focus in managing customer experience was on re-inventing their product and service delivery to their customers as regular modes were disrupted. As they emerge from the crisis, organisations will realise that it is not only their customer experience delivery models that have changed – but customer expectations have also evolved in the last few months. They are more open to digital interactions and in many cases the concept of brand loyalty has been diluted. This will change everything for organisations’ customer strategies. And digital technology will play a significant role as they continue to pivot to succeed in 2021 – across regions, industries and organisations.
Ecosystm Advisors Audrey William, Niloy Mukherjee and Tim Sheedy present the top 5 Ecosystm predictions for Customer Experience in 2021. This is a summary of the predictions – the full report (including the implications) is available to download for free on the Ecosystm platform.
The Top 5 Customer Experience Trends for 2021
- Customer Experience Will Go Truly Digital
COVID-19 made the few businesses that did not have an online presence acutely aware that they need one – yesterday! We have seen at least 4 years of digital growth squeezed into six months of 2020. And this is only the beginning. While in 2020, the focus was primarily on eCommerce and digital payments, there will now be a huge demand for new platforms to be able to interact digitally with the customer, not just to be able to sell something online.
Digital customer interactions with brands and products – through social media, online influencers, interactive AI-driven apps, online marketplaces and the like will accelerate dramatically in 2021. The organisations that will be successful will be the ones that are able to interact with their customers and connect with them at multiple touchpoints across the customer journey. Companies unable to do that will struggle.
- Digital Engagement Will Expand Beyond the Traditional Customer-focused Industries
One of the biggest changes in 2020 has been the increase in digital engagement by industries that have not traditionally had a strong eye on CX. This trend is likely to accelerate and be further enhanced in 2021.
Healthcare has traditionally been focused on improving clinical outcomes – and patient experience has been a byproduct of that focus. Many remote care initiatives have the core objective of keeping patients out of the already over-crowded healthcare provider organisations. These initiatives will now have a strong CX element to them. The need to disseminate information to citizens has also heightened expectations on how people want their healthcare organisations and Public Health to interact with them. The public sector will dramatically increase digital interactions with citizens, having been forced to look at digital solutions during the pandemic.
Other industries that have not had a traditional focus on CX will not be far behind. The Primary & Resources industries are showing an interest in Digital CX almost for the first time. Most of these businesses are looking to transform how they manage their supply chains from mine/farm to the end customer. Energy and Utilities and Manufacturing industries will also begin to benefit from a customer focus – primarily looking at technology – including 3D printing – to customise their products and services for better CX and a larger share of the market.
- Brands that Establish a Trusted Relationship Can Start Having Fun Again
Building trust was at the core of most businesses’ CX strategies in 2020 as they attempted to provide certainty in a world generally devoid of it. But in the struggle to build a trusted experience and brand, most businesses lost the “fun”. In fact, for many businesses, fun was off the agenda entirely. Soft drink brands, travel providers, clothing retailers and many other brands typically known for their fun or cheeky experiences moved the needle to “trust” and dialed it up to 11. But with a number of vaccines on the horizon, many CX professionals will look to return to pre-pandemic experiences, that look to delight and sometimes even surprise customers.
However, many companies will get this wrong. Customers will not be looking for just fun or just great experiences. Trust still needs to be at the core of the experience. Customers will not return to pre-pandemic thinking – not immediately anyway. You can create a fun experience only if you have earned their trust first. And trust is earned by not only providing easy and effective experiences, but by being authentic.
- Customer Data Platforms Will See Increased Adoption
Enterprises continue to struggle to have a single view of the customer. There is an immense interest in making better sense of data across every touchpoint – from mobile apps, websites, social media, in-store interactions and the calls to the contact centre – to be able to create deeper customer profiles. CRM systems have been the traditional repositories of customer data, helping build a sales pipeline, and providing Marketing teams with the information they need for lead generation and marketing campaigns. However, CRM systems have an incomplete view of the customer journey. They often collect and store the same data from limited touchpoints – getting richer insights and targeted action recommendations from the same datasets is not possible in today’s world. And organisations struggled to pivot their customer strategies during COVID-19. Data residing in silos was an obstacle to driving better customer experience.
We are living in an age where customer journeys and preferences are becoming complex to decipher. An API-based CDP can ingest data from any channel of interaction across multiple journeys and create unique and detailed customer profiles. A complete overhaul of how data can be segregated based on a more accurate and targeted profile of the customer from multiple sources will be the way forward in order to drive a more proactive CX engagement.
- Voice of the Customer Programs Will be Transformed
Designing surveys and Voice of Customer programs can be time-consuming and many organisations that have a routine of running these surveys use a fixed pattern for the data they collect and analyse. However, some organisations understand that just analysing results from a survey or CSAT score does not say much about what customers’ next plan of action will be. While it may give an idea of whether particular interactions were satisfactory, it gives no indication of whether they are likely to move to another brand; if they needed more assistance; if there was an opportunity to upsell or cross sell; or even what new products and services need to be introduced. Some customers will just tick the box as a way of closing off a feedback form or survey. Leading organisations realise that this may not be a good enough indication of a brand’s health.
Organisations will look beyond CSAT to other parameters and attributes. It is the time to pay greater attention to the Voice of the Customer – and old methods alone will not suffice. They want a 360-degree view of their customers’ opinions.
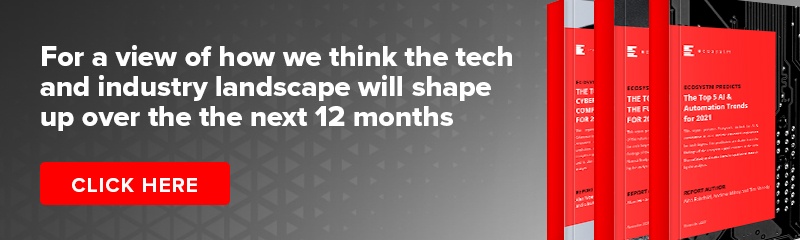
Artificial Intelligence (AI) is becoming embedded in financial services across consumer interactions and core business processes, including the use of chatbots and natural language processing (NLP) for KYC/AML risk assessment.
But what does AI mean for financial regulators? They are also consuming increasing amounts of data and are now using AI to gain new insights and inform policy decisions.
The efficiencies that AI offers can be harnessed in support of compliance within both financial regulation (RegTech) and financial supervision (SupTech). Authorities and regulated institutions have both turned to AI to help them manage the increased regulatory requirements that were put in place after the 2008 financial crisis. Ecosystm research finds that compliance is key to financial institutions (Figure 1).
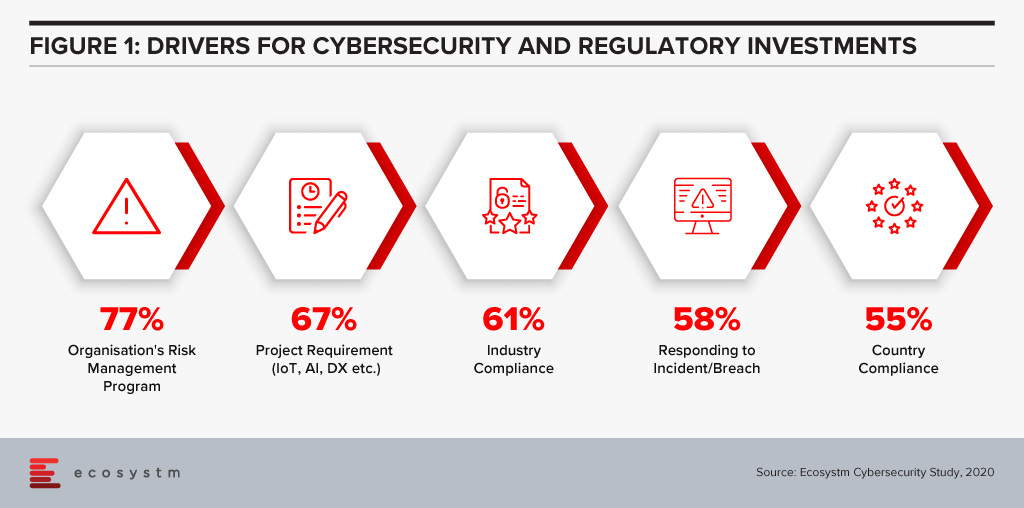
SupTech is maturing with more robust safeguards and frameworks, enabling the necessary advancements in technology implementation for AI and Machine Learning (ML) to be used for regulatory supervision. The Bank of England and the UK Financial Conduct Authority surveyed the industry in March 2019 to understand how and where AI and ML are being used, and their results indicated 80% of survey respondents were using ML. The most common application of SupTech is ML techniques, and more specifically NLP to create more efficient and effective supervisory processes.
Let us focus on the use of NLP, specifically on how it has been used by banking authorities for policy decision making during the COVID-19 crisis. AI has the potential to read and comprehend significant details from text. NLP, which is an important subset of AI, can be seen to have supported operations to stay updated with the compliance and regulatory policy shifts during this challenging period.
Use of NLP in Policy Making During COVID-19
The Financial Stability Board (FSB) coordinates at the international level, the work of national financial authorities and international standard-setting bodies in order to develop and promote the implementation of effective regulatory, supervisory and other financial sector policies. A recent FSB report delivered to G20 Finance Ministers and Central Bank Governors for their virtual meeting in October 2020 highlighted a number of AI use cases in national institutions.
We illustrate several use cases from their October report to show how NLP has been deployed specifically for the COVID-19 situation. These cases demonstrate AI aiding supervisory team in banks and in automating information extraction from regulatory documents using NLP.
De Nederlandsche Bank (DNB)
The DNB is developing an interactive reporting dashboard to provide insight for supervisors on COVID-19 related risks. The dashboard that is in development, enables supervisors to have different data views as needed (e.g. over time, by bank). Planned SupTech improvements include incorporating public COVID-19 information and/or analysing comment fields with text analysis.
Monetary Authority of Singapore (MAS)
MAS deployed automation tools using NLP to gather international news and stay abreast of COVID-19 related developments. MAS also used NLP to analyse consumer feedback on COVID-19 issues, and monitor vulnerabilities in the different customer and product segments. MAS also collected weekly data from regulated institutions to track the take-up of credit relief measures as the pandemic unfolded. Data aggregation and transformation were automated and visualised for monitoring.
US Federal Reserve Bank Board of Governors
One of the Federal Reserve Banks in the US is currently working on a project to develop an NLP tool used to analyse public websites of supervised regulated institutions to identify information on “work with your customer” programs, in response to the COVID-19 crisis.
Bank of England
The Bank developed a Policy Response Tracker using web scraping (targeted at the English versions of each authority/government website) and NLP for the extraction of key words, topics and actions taken in each jurisdiction. The tracker pulls information daily from the official COVID-19 response pages then runs it through specific criteria (e.g. user-defined keywords, metrics and risks) to sift and present a summary of the information to supervisors.
Market Implications
Even with its enhanced efficiencies, NLP in SupTech is still an aid to decision making and cannot replace the need for human judgement. NLP in policy decision is performing clearly defined information gathering tasks with greater efficiency and speed. But NLP cannot change the quality of the data provided, so data selection and choice are still critical to effective policy making.
For authorities, the use of SupTech could improve oversight, surveillance, and analytical capabilities. These efficiency gains and possible improvement in quality arising from automation of previously manual processes could be consideration for adoption.
Attention will be paid in 2021 to focusing on automation of processes using AI (Figure 2).
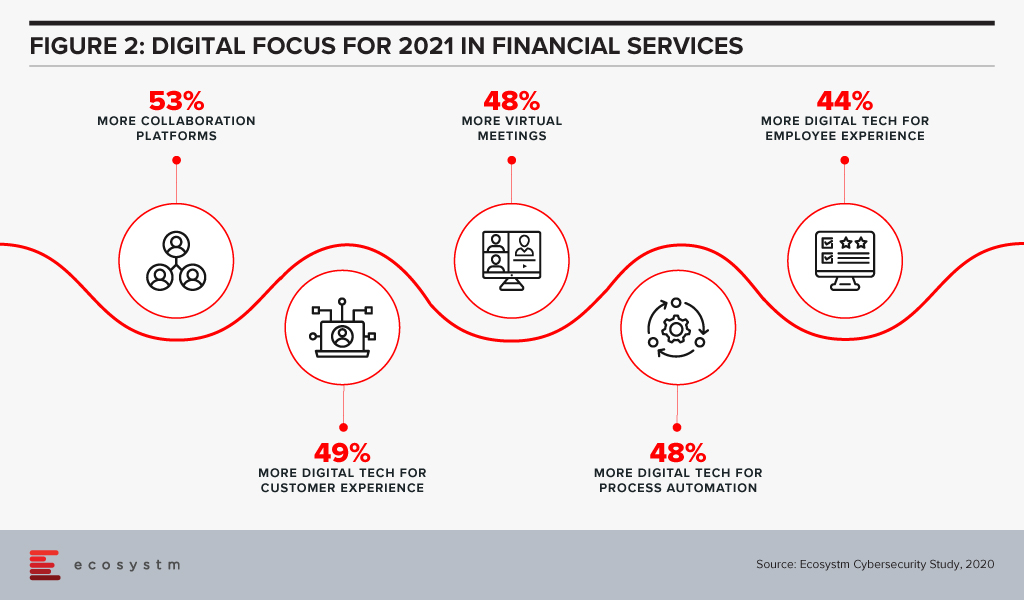
Based on a survey done by the FSB of its members (Figure 3), the majority of their respondents had a SupTech innovation or data strategy in place, with the use of such strategies growing significantly since 2016.
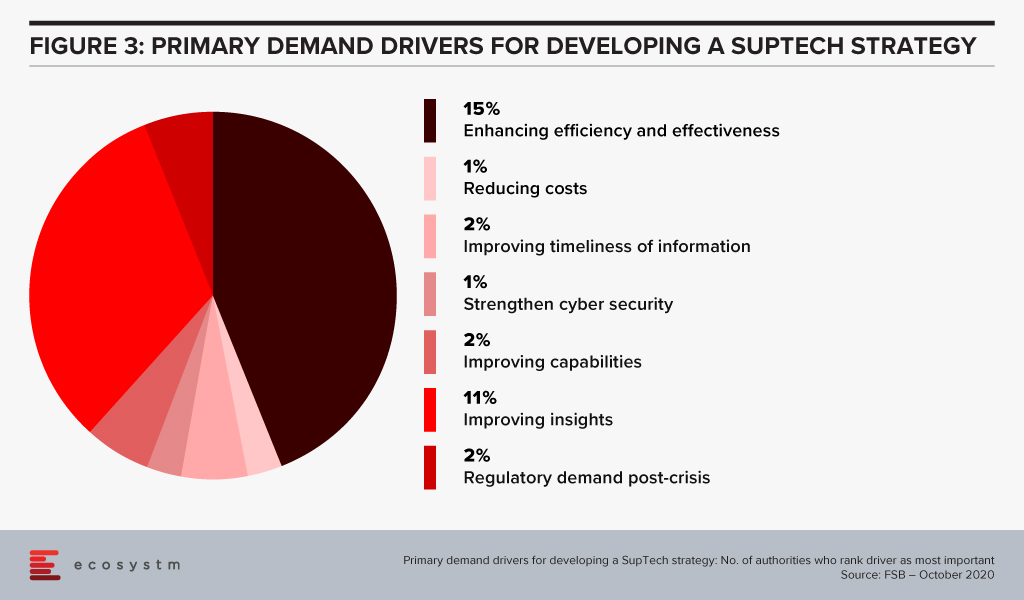
Summary
For more mainstream adoption, data standards and use of effective governance frameworks will be important. As seen from the FSB survey, SupTech applications are now used in reporting, data management and virtual assistance. But institutions still send the transaction data history in different reporting formats which results in a slower process of data analysing and data gathering. AI, using NLP, can help with this by streamlining data collection and data analytics. While time and cost savings are obvious benefits, the ability to identify key information (the proverbial needle in the haystack) can be a significant efficiency advantage.
Singapore FinTech Festival 2020: Infrastructure Summit
For more insights, attend the Singapore FinTech Festival 2020: Infrastructure Summit which will cover topics tied to creating infrastructure for a digital economy; and RegTech and SupTech policies to drive innovation and efficiencies in a co-Covid-19 world.
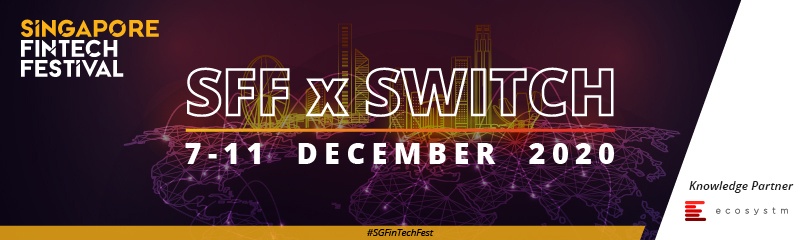
Technologies to automate IT systems and relieve over-stretched IT operations teams have been moving into the mainstream over the last few years. Several factors, driven by the digital era, have made this necessary. Firstly, digital transformation is creating ever-larger IT environments and volumes of data that cannot be managed by manual processes. These distributed systems are also becoming more complex, incorporating IoT, mobile, multi-cloud, containers, and APIs. Moreover, for digital businesses, the financial impact of an outage makes time to resolution critical. Identifying and remediating issues before they affect the user is now paramount. AIOps provides intelligence to the IT operations team that allows them to proactively resolve events before they become outages.
Augmenting IT Operations with AIOps
AIOps allows IT operations teams to not only ensure observability of their systems and reduce noise but to also understand how events are interacting together to affect performance and take corrective action quickly. The primary features of AIOps are:
- Noise reduction. AIOps ingests systems data, surfaces priority anomalies and correlates them together. This brings the number of incidents to investigate back down to a human level. Rackspace recently announced that AIOps helped it reduce alert noise by 99% during the initial stage of its rollout. Successful vendor references typically cite similar figures between 95-99%.
- Root cause analysis. Once priority events have been correlated, AIOps identifies a root cause to enable the operations team to focus its efforts on a resolution. This is a task that proves challenging to perform at speed for a human operator considering the complexity of today’s systems.
- Proactive response. A range of responses is available with AIOps, from directing issues to the appropriate people, to recommending actions that can be taken by operators directly in a collaboration tool, to rules-based workflows performed automatically, such as spinning up additional AWS EC2 instances.
- Learning. By evaluating past failures and successes, AIOps can learn over time which events are likely to become critical and how to respond to them. This brings us closer to the dream of NoOps, where operations are completely automated.
The Impact of COVID-19 on IT Operations
The Ecosystm Digital Priorities in the New Normal study launched this month, asks technology users about how their digital priorities have shifted during the pandemic. Despite pressure to shift to digital delivery, almost 40% of participants reported that their organisations cut headcount in the IT department (Figure 1). Furthermore, over one third had been forced to cut their employees’ salaries. As we have seen in previous crises, IT operations teams are being asked to do more with less and will need automation to bridge the gaps.
As we begin to move into the next phase of the COVID-19 reality and businesses continue to open, we will see many launch digital services that were conceived of during the crisis. One of the greatest challenges that IT departments face will be scalability as digital businesses grow. AIOps will be a go-to tool for IT operations to ensure uptime and improve user experience. It is likely that the next 12-18 months will be a watershed moment for AIOps.
NLP and the Democratisation of Data
Natural Language Processing (NLP) will be the next string in the bow of AIOps. While the ultimate goal of IT operations is to identify and remediate situations before they have an impact on the user, oftentimes it is the service desk that generates the initial barrage of alerts. AIOps equipped with NLP can extract relevant data from user tickets, correlate them with other system events and potentially even suggest a resolution to the user. Here, ChatOps can help to reduce the workload on the service desk and bring relevant events to the attention of the operations team faster. NLP will also help democratise IT operations data within the organisation. As they digitalise, lines of business (LoBs) besides IT will need access to system health and user experience data but business managers may not have the necessary technical skills to extract them. Chatbots that can return these metrics to non-technical users will begin to proliferate.
AIOps Recommendations
Most IT departments would have discovered the limitations of their current systems during the upheaval caused by recent lockdowns. Only about 7% of organisations in our study reported that they were well-prepared across all areas of IT, to handle the COVID-19 crisis. For those organisations that have yet to invest in AIOps, we recommend starting now but starting small. Develop a topology map to understand where you have reliable data sources that could be analysed by AIOps. Then select a domain by assessing the present level of observability and automation, IT skills gap, frequency of outages, and business criticality. As you add additional domains and the system learns, the value you realise from AIOps will grow.
The power of collaborative AIOps tools would have been undeniable as the COVID-19 crisis began and IT departments were forced to work in a distributed manner. When evaluating a system, carefully consider how it will integrate into your organisation’s preferred collaboration suite, whether it be the AIOps vendor’s proprietary situation tool or a third-party provider like Slack or Microsoft Teams. The ability for operations teams to collaborate effectively reduces time to resolution.
Artificial intelligence (AI) is perhaps the most electrifying and controversial of the so-called “disruptive” technologies. As AI becomes more sophisticated and the technology evolves, it will increasingly help to perform more complex tasks whether for personal or commercial use.
Today’s AI machines can replicate certain elements of intellectual ability and they are constantly striving to achieve more. This includes applications of autonomous vehicles, domestic and industrial robots, surveillance and security, automation, personal assistants, forecasting, data analysis and more.
The global Ecosystm AI study reveals top drivers of AI adoption. Organisations are trying to incorporate AI in their existing processes for better competitor analysis and insights, cost-effectiveness, deeper customer engagement to provide personalised service/product offerings, and for process redesign or automation.
AI supporting technologies
AI is driving important technologies and processes and driving better, faster and more accurate decisions which help processes run more effectively and efficiently. With AI insights, business strategies will be more information-driven, efficient and consistent.
There are certainly many benefits that organisations are deriving from AI. Ecosystm AI study reveals that organisations implementing AI are using it to drive various business solutions including billing management, supply chain optimisation, predictive maintenance, enhancing operations and more.
Below is a list of some hot technologies driven by AI.
Advanced Analytics
The proliferation of Big Data has led to the creation of massive data sets that can only be effectively analysed with AI tools and statistical models. AI can spot complex patterns in the data which is difficult for humans to understand. AI’s usefulness as an analytics tool is highly gaining importance in the use of predictive analytics and decision automation. Once sufficient data is available for use by AI, which is further filtered and processed thoroughly, the system can suggest actions or outcomes based on various parameters such as trends, patterns, historical information, frequency and more.
For example, the financial services industry is using advanced analytics to evaluate how customers earn, invest, spend and make financial decisions, which is useful for the organisations to customise their customers’ preference and offerings accordingly.
Natural Language Processing (NLP) and speech recognition
NLP involves the learning of languages by machine through the means of interaction between computers and unstructured speech/text. NLP requires massive processing power and complex algorithms to reinforce learning mechanisms. To help in NLP, AI generates models, which are further improved to create NLP and speech simulations. Nowadays, Natural language is being implemented and used in various conversational interfaces, such as those with bots, artificial learning agents that can generalise to new environments, and autonomous vehicles.
Cognitive processing
Otherwise known as semantic computing, refers to a digital processing that attempts to mimic the operation of the human brain. In general, semantics means the meaning and interpretation of words and sentence structure and how words relate to other words. So, how is semantic related and what is the semantic analysis used for in AI? Semantic technology processes the logical structure of sentences to identify the most relevant elements in the text to understand the topic. It is especially suited to the analysis of large unstructured datasets with high efficiency.
Vantagepoint has an artificial intelligence tool to improve their trading results. It has a patented tool which can forecast stocks, futures, commodities, Forex and ETFs and claims an accuracy of up to 86%. The tool can predict changes in market trend direction up to three days in advance thus enabling traders to get in and out of trades at optimal times with confidence.
Robotic Process Automation (RPA)
RPA has grown out of Business Process Automation (BPA) and refers to the use of AI to automate workflow and business processes. The advantages of RPA demonstrate it to be a solid tool in attaining higher quality output at lower costs which is much quicker than traditional methods. RPA can be used in IT support processes, back-office work, and workflow processes. The rules are programmed, and bots extract structured inputs from applications like Excel and enter them into other software such as CRM, SCM or accounting. A good example is the use of NLP to scan incoming emails and undertake the appropriate action, such as generating an invoice or flagging a complaint in an automated manner.
Machine Learning
Machine learning is an application of artificial intelligence (AI) which involves a combination of raw computing power and logic-based models to simulate the human learning process. Machine Learning is proving to be a successful approach to AI. When humans learn, they alter the way they relate information and the world, similarly when machines learn, they alter the data and form it into a piece of information.
An example, Image recognition is a popular application of machine learning in which images are fed into an algorithm, which attempts to recognise the contents of the image based on patterns. For instance, Yelp’s machine learning algorithms help the company’s human staff deal with tens of millions of photos to compile, categorise, and label the images more efficiently.
Chatbots and virtual assistants
Chatbots are robotic processes which simulate human conversation and automate functions. The technology is also used for so-called ‘virtual assistants’, which uses AI to interact with humans and aid with specific queries. They are increasingly being used to handle simple conversation and tasks in B2B and B2C environments. The addition of chatbots reduces human assistants and they can work throughout the clock. Chatbots and Virtual assistants improve with AI and can be trained to review conversations, past transactions and to draft a response based on context. If the user interacts with the bot through voice, then the chatbot requires a speech recognition engine.
Chatbots have been used in instant messaging (IM) applications and online interactive platforms. To exemplify, chatbots are deployed to assist online shoppers by answering noncomplex product questions, pricing, FAQ’s, order processing steps or forwarding information to human agents on complicated questions such as shipping delays or faults.
With AI technology evolving and improving so rapidly, many organisations are looking to use AI in their business, but there are still many questions to which adopters are seeking answers such as how to integrate AI into their existing systems, how to get access to data that will enable AI as well as the persistent technology concerns around cybersecurity and cost. The goal of many AI providers is to reach a stage where AI will support humans, control machines for us and automate repetitive tasks and processes.