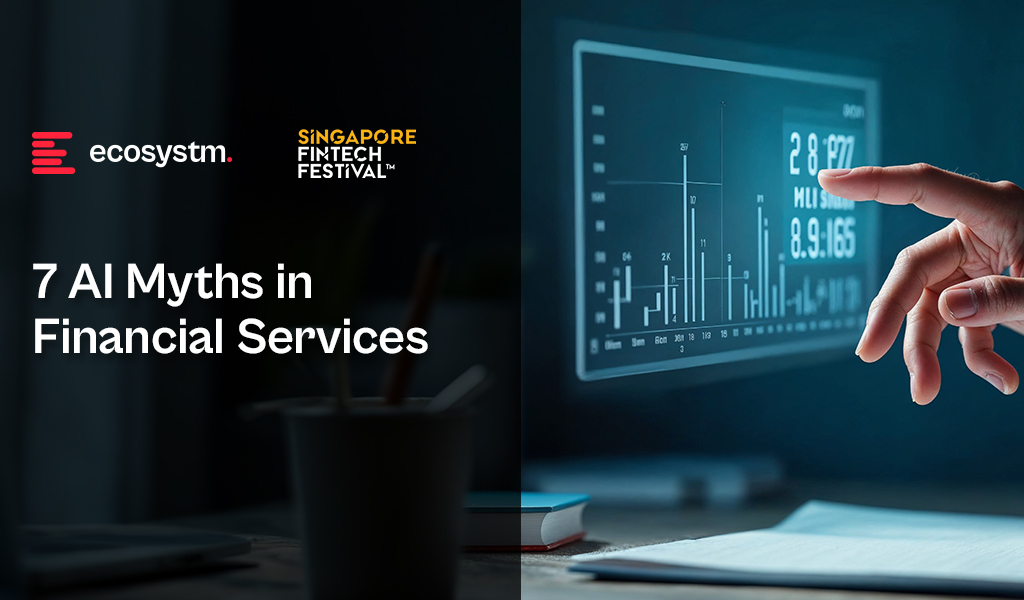
Large organisations in the banking and financial services industry have come a long way over the past two decades in cutting costs, restructuring IT systems and redefining customer relationship management. And, as if that was not enough, they now face the challenge of having to adapt to ongoing global technological shifts or the challenge of having to “do something with AI” without being AI-ready in terms of strategy, skills and culture.
Most organisations in the industry have started approaching AI implementation in a conventional way, based on how they have historically managed IT initiatives. Their first attempts at experimenting with AI have led to rapid conclusions forming seven common myths. However, as experience with AI grows, these myths are gradually being debunked. Let us put these myths to a reality check.
1. We can rely solely on external tech companies
Even in a highly regulated industry like banking and financial services, internal processes and data management practices can vary significantly from one institution to another. Experience shows that while external providers – many of whom lack direct industry experience – can offer solutions tailored to the more obvious use cases and provide customisation, they fall short when it comes to identifying less apparent opportunities and driving fundamental changes in workflows. No one understands an institution’s data better than its own employees. Therefore, a key success factor in AI implementation is active internal ownership, involving employees directly rather than delegating the task entirely to external parties. While technology providers are essential partners, organisations must also cultivate their own internal understanding of AI to ensure successful implementation.
2. AI is here to be applied to single use cases
In the early stages of experimenting with AI, many financial institutions treated it as a side project, focusing on developing minimum viable products and solving isolated problems to explore what worked and what didn’t. Given their inherently risk-averse nature, organisations often approached AI cautiously, addressing one use case at a time to avoid disrupting their broader IT landscape or core business. However, with AI’s potential for deep transformation, the financial services industry has an opportunity not only to address inefficiencies caused by manual, time-consuming tasks but also to question how data is created, captured, and used from the outset. This requires an ecosystem of visionary minds in the industry who join forces and see beyond deal generation.
3. We can staff AI projects with our highly motivated junior employees and let our senior staff focus on what they do best – managing the business
Financial institutions that still view AI as a side hustle, secondary to their day-to-day operations, often assign junior employees to handle AI implementation. However, this can be a mistake. AI projects involve numerous small yet critical decisions, and team members need the authority and experience to make informed judgments that align with the organisation’s goals. Also, resistance to change often comes from those who were not involved in shaping or developing the initiative. Experience shows that project teams with a balanced mix of seniority and diversity in perspectives tend to deliver the best results, ensuring both strategic insight and operational engagement.
4. AI projects do not pay off
Compared to conventional IT projects, the business cases for AI implementation – especially when limited to solving a few specific use cases – often do not pay off over a period of two to three years. Traditional IT projects can usually be executed with minimal involvement of subject matter experts, and their costs are easier to estimate based on reference projects. In contrast, AI projects are highly experimental, requiring multiple iterations, significant involvement from experts, and often lacking comparable reference projects. When AI solutions address only small parts of a process, the benefits may not be immediately apparent. However, if AI is viewed as part of a long-term transformational journey, gradually integrating into all areas of the organisation and unlocking new business opportunities over the next five to ten years, the true value of AI becomes clear. A conventional business case model cannot fully capture this long-term payoff.
5. We are on track with AI if we have several initiatives ongoing
Many financial institutions have begun their AI journey by launching multiple, often unrelated, use case-based projects. The large number of initiatives can give top management a false sense of progress, as if they are fully engaged in AI. However, investors and project teams often ask key questions: Where are these initiatives leading? How do they contribute? What is the AI vision and strategy, and how does it align with the business strategy? If these answers remain unclear, it’s difficult to claim that the organisation is truly on track with AI. To ensure that AI initiatives are truly impactful and aligned with business objectives, organisations must have a clear AI vision and strategy – and not rely on number of initiatives to measure progress.
6. AI implementation projects always exceed their deadlines
AI solutions in the banking and financial services industry are rarely off-the-shelf products. In cases of customisation or in-house development, particularly when multiple model-building iterations and user tests are required, project delays of three to nine months can occur. This is largely because organisations want to avoid rolling out solutions that do not perform reliably. The goal is to ensure that users have a positive experience with AI and embrace the change. Over time, as an organisation becomes more familiar with AI implementation, the process will become faster.
7. We upskill our people by giving them access to AI training
Learning by doing has always been and will remain the most effective way to learn, especially with technology. Research has shown that 90% of knowledge acquired in training is forgotten after a week if it is not applied. For organisations, the best way to digitally upskill employees is to involve them in AI implementation projects, even if it’s just a few hours per week. To evaluate their AI readiness or engagement, organisations could develop new KPIs, such as the average number of hours an employee actively engages in AI implementation or the percentage of employees serving as subject matter experts in AI projects.
Which of these myths have you believed, and where do you already see changes?
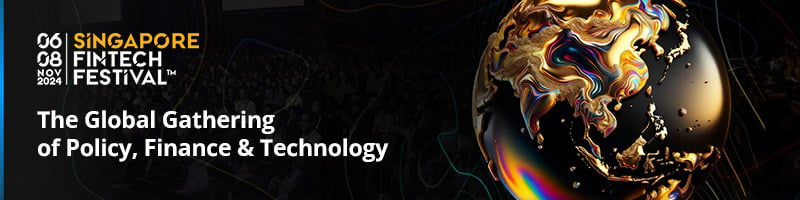