AI systems are creating huge amounts of data at a rapid rate. While this flood of information is extremely valuable, it is also difficult to analyse and understand. Organisations need to make sense of these large data sets to derive useful insights and make better decisions. Data visualisation plays a pivotal role in the interpretation of complex data, making it accessible, understandable, and actionable. Well-designed visualisation can translate complex, high-dimensional data into intuitive, visually appealing representations, helping stakeholders to understand patterns, trends, and anomalies that would otherwise be challenging to recognise.
There are some data visualisation methods that you are using already; and some that you definitely should master as data complexity increases and there is more demand from business teams for better data visualisation.
Download Common Data Visualisation Methods as a PDF
Add These to Your Data Visualisation Repertoire
There are additional visualisation tools that you should be using to tell a better data story. Each of these visualisation techniques serves specific purposes in data analysis, offering unique advantages for representing data insights.
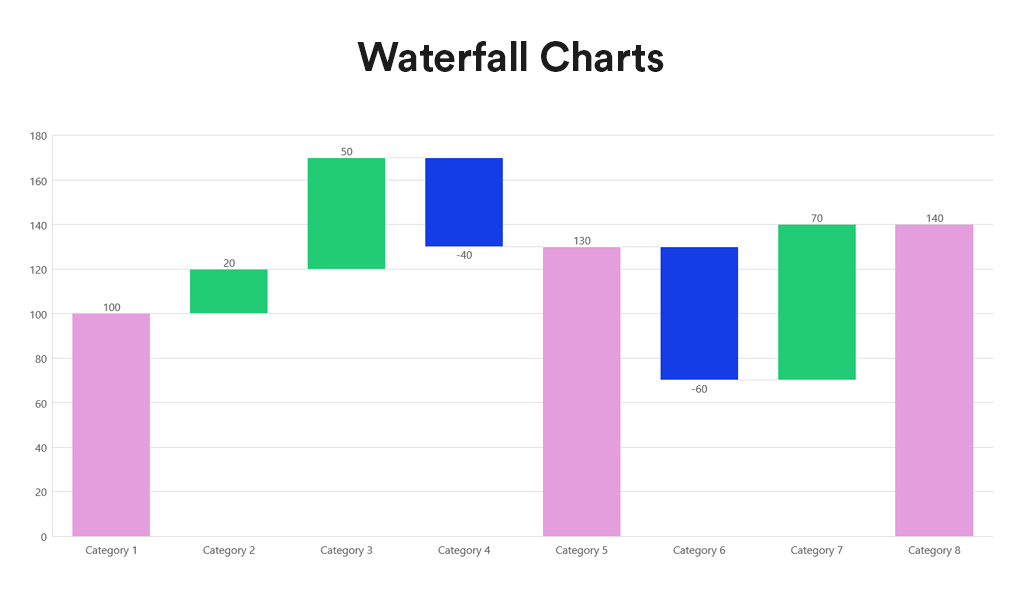
Waterfall charts depict the impact of intermediate positive and negative values on an initial value, often resulting in a final value. They are commonly employed in financial analysis to illustrate the contribution of various factors to a total, making them ideal for visualising step-by-step financial contributions or tracking the cumulative effect of sequentially introduced factors.
Advantages:
- Sequential Analysis. Ideal for understanding the cumulative effect of sequentially introduced positive or negative values.
- Financial Reporting. Commonly used for financial statements to break down the contributions of various elements to a net result, such as revenues, costs, and profits over time.
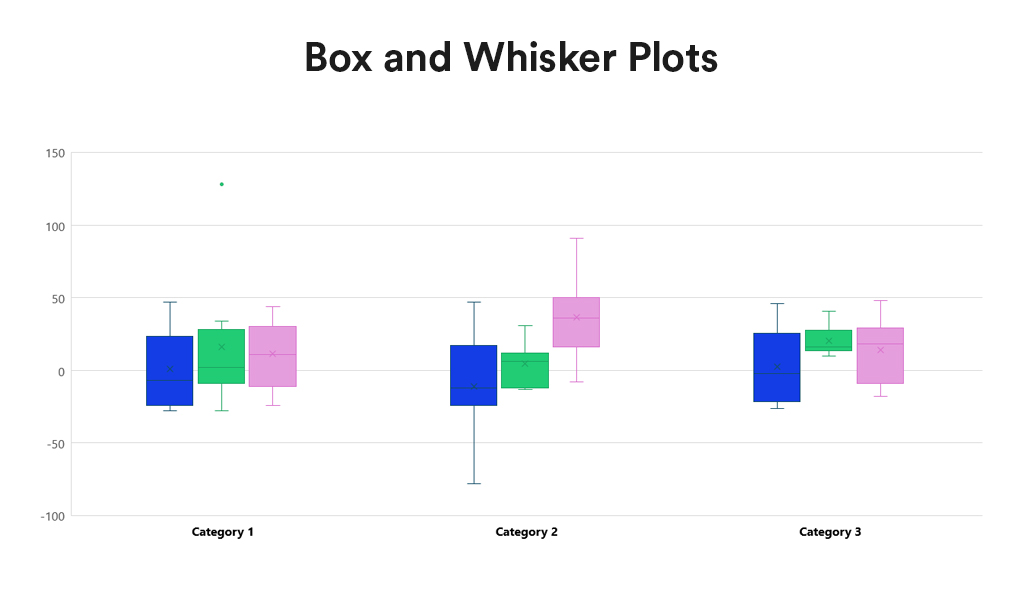
Box and Whisker Plots summarise data distribution using a five-number summary: minimum, first quartile (Q1), median, third quartile (Q3), and maximum. They are valuable for showcasing data sample variations without relying on specific statistical assumptions. Box and Whisker Plots excel in comparing distributions across multiple groups or datasets, providing a concise overview of various statistics.
Advantages:
- Distribution Clarity. Provide a clear view of the data distribution, including its central tendency, variability, and skewness.
- Outlier Identification. Easily identify outliers, offering insights into the spread and symmetry of the data.
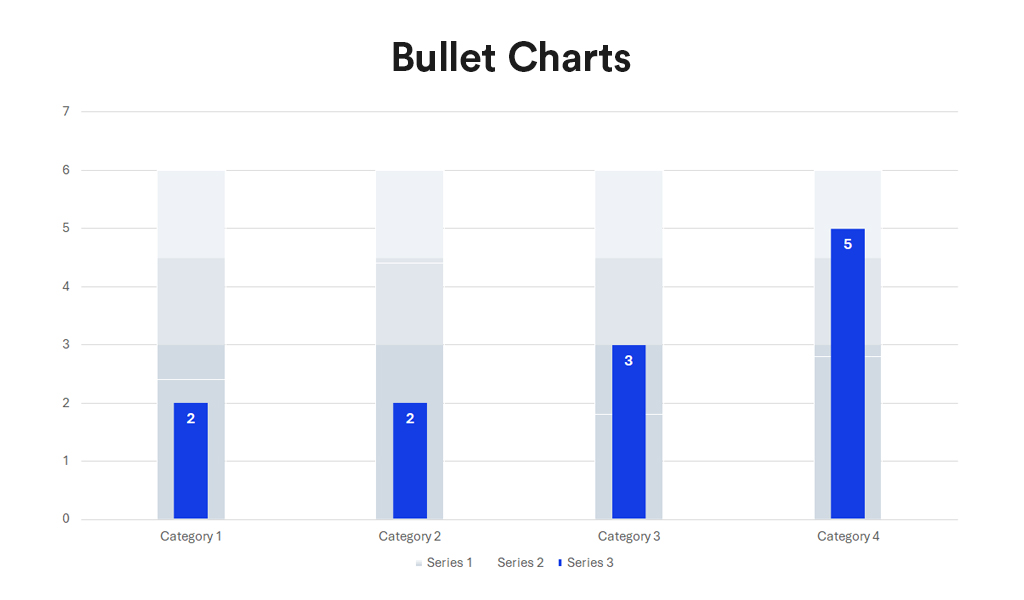
Bullet charts, a bar graph variant, serve as a replacement for dashboard gauges and meters. They showcase a primary measure alongside one or more other measures for context, such as a target or previous period’s performance, often incorporating qualitative ranges like poor, satisfactory, and good. Ideal for performance dashboards with limited space, bullet charts efficiently demonstrate progress towards goals.
Advantages:
- Compactness. Offer a compact and straightforward way to monitor performance against a target.
- Efficiency. More efficient than gauges and meters in dashboard design, as they take up less space and can display more information, making them ideal for comparing multiple measures.
Conclusion
Each data visualisation type has its unique strengths, making it better suited for certain types of data and analysis than others. The key to effective data visualisation lies in matching the visualisation type to your data’s specific needs, considering the story you want, to tell or the insights you aim to glean. Choosing the right data representation helps you to make informed decisions that enhance your data analysis and communication efforts.
Incorporating Waterfall Charts, Box and Whisker Plots, and Bullet Charts into the data visualisation toolkit allows for a broader range of insights to be derived from your data. From analysing financial data, comparing distributions, to tracking performance metrics, these additional types of visualisation can communicate complex data stories clearly and effectively. As with all data visualisation, the key is to choose the type that best matches the organisation’s data story, making it accessible and understandable to the audience.
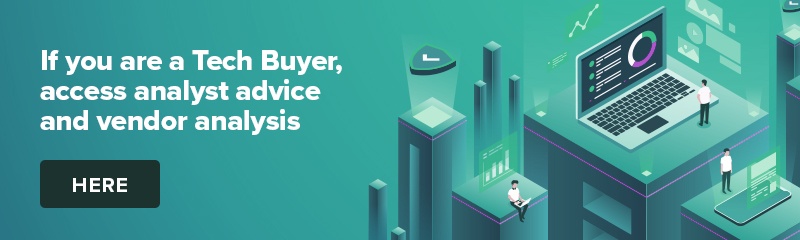
AI has become a business necessity today, catalysing innovation, efficiency, and growth by transforming extensive data into actionable insights, automating tasks, improving decision-making, boosting productivity, and enabling the creation of new products and services.
Generative AI stole the limelight in 2023 given its remarkable advancements and potential to automate various cognitive processes. However, now the real opportunity lies in leveraging this increased focus and attention to shine the AI lens on all business processes and capabilities. As organisations grasp the potential for productivity enhancements, accelerated operations, improved customer outcomes, and enhanced business performance, investment in AI capabilities is expected to surge.
In this eBook, Ecosystm VP Research Tim Sheedy and Vinod Bijlani and Aman Deep from HPE APAC share their insights on why it is crucial to establish tailored AI capabilities within the organisation.
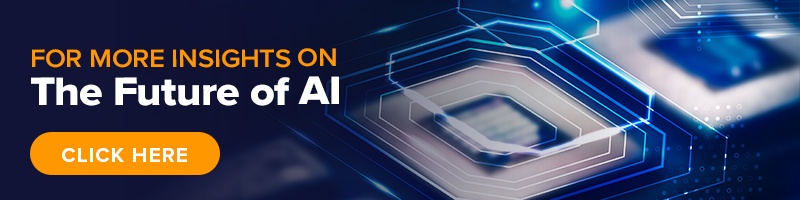
Fintechs have carved out a niche both in their customer-centric approach and in crafting solutions for underserved communities without access to traditional financial services. Irrespective of their objectives, there is an immense reliance on innovation for lower-cost, personalised, and more convenient services.
However, a staggering 75% of venture-backed fintech startups fail to scale and grow – and this applies to fintechs as well.
Here are the 5 areas that fintechs need to focus on to succeed in a competitive market.
Download ‘Building a Successful Fintech Business’ as a PDF
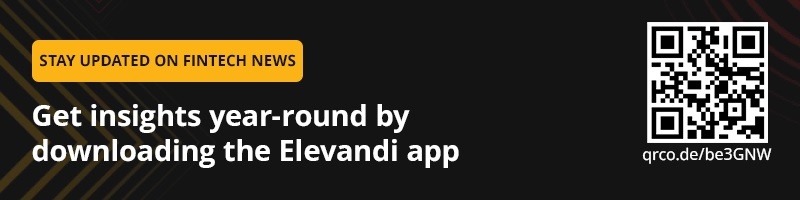
Data & AI initiatives are firmly at the core of any organisation’s tech-led transformation efforts. Businesses today realise the value of real-time data insights to deliver the agility that is required to succeed in today’s competitive, and often volatile, market.
But organisations continue to struggle with their data & AI initiatives for a variety of reasons. Organisations in ASEAN report some common challenges in implementing successful data & AI initiatives.
Here are 5 insights to build scalable AI.
- Data Access a Key Stumbling Block. Many organisations find that they no longer need to rely on centralised data repositories.
- Organisations Need Data Creativity. A true data-first organisation derives value from their data & AI investments across the entire organisation, cross-leveraging data.
- Governance Not Built into Organisational Psyche. A data-first organisation needs all employees to have a data-driven mindset. This can only be driven by clear guidelines that are laid out early on and adhered to by data generators, managers, and consumers.
- Lack of End-to-End Data Lifecycle Management. It is critical to have observability, intelligence, and automation built into the entire data lifecycle.
- Democratisation of Data & AI Should Be the Goal. The true value of data & AI solutions will be fully realised when the people who benefit from the solutions are the ones managing the solutions and running the queries that will help them deliver better value to the business.
Read below to find out more.
Download 5 Insights to Help Organisations Build Scalable AI – An ASEAN View as a PDF
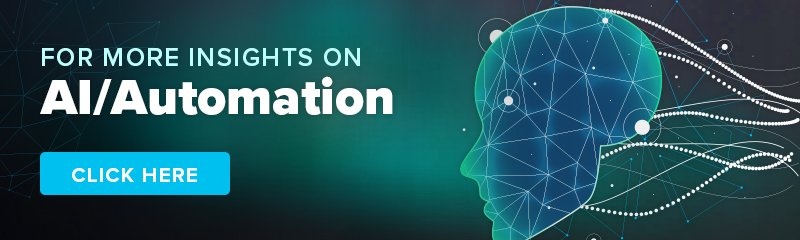
In the rush towards digital transformation, individual lines of business in organisations, have built up collections of unconnected systems, each generating a diversity of data. While these systems are suitable for rapidly launching services and are aimed at solving individual challenges, digital enterprises will need to take a platform approach to unlock the full value of the data they generate.
Data-driven enterprises can increase revenue and shift to higher margin offerings through personalisation tools, such as recommendation engines and dynamic pricing. Cost cutting can be achieved with predictive maintenance that relies on streaming sensor data integrated with external data sources. Increasingly, advanced organisations will monetise their integrated data by providing insights as a service.
Digital enterprises face new challenges – growing complexity, data explosion, and skills gap.
Here are 5 ways in which IT teams can mitigate these challenges.
- Data & AI projects must focus on data access. When the organisation can unify data and transmit it securely wherever it needs to, it will be ready to begin developing applications that utilise machine learning, deep learning, and AI.
- Transformation requires a hybrid cloud platform. Hybrid cloud provides the ability to place each workload in an environment that makes the most sense for the business, while still reaping the benefits of a unified platform.
- Application modernisation unlocks future value. The importance of delivering better experiences to internal and external stakeholders has not gone down; new experiences need modern applications.
- Data management needs to be unified and automated. Digital transformation initiatives result in ever-expanding technology estates and growing volumes of data that cannot be managed with manual processes.
- Cyber strategy should be Zero Trust – backed by the right technologies. Organisations have to build Digital Trust with privacy, protection, and compliance at the core. The Zero Trust strategy should be backed by automated identity governance, robust access and management policies, and least privilege.
Read below to find out more.
Download The Future of Business: 5 Ways IT Teams Can Help Unlock the Value of Data as a PDF
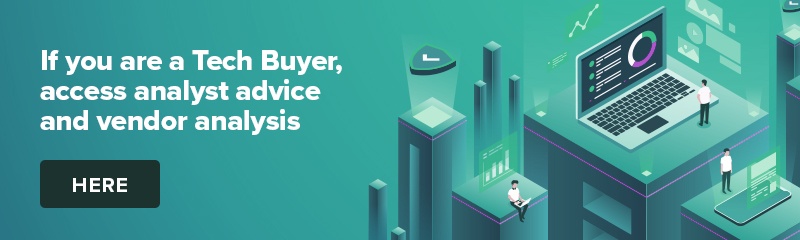
November has seen uncertainties in the technology market with news of layoffs and hiring freezes from big names in the industry – Meta, Amazon, Salesforce, and Apple to name a few. These have impacted thousands of people globally, leaving tech talent with one common question, ‘What next?’
While the current situation and economic trends may seem grim, it is not all bad news for tech workers. It is true that people strategies in the sector may be impacted, but there are still plenty of opportunities for tech experts in the industry.
Here is what Ecosystm Analysts say about what’s next for technology workers.
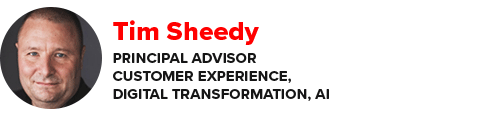
Today, we are seeing two quite conflicting signals in the market: Tech vendors are laying off staff; and IT teams in businesses are struggling to hire the people they need.
At Ecosystm, we still expect a healthy growth in tech spend in 2023 and 2024 regardless of economic conditions. Businesses will be increasing their spend on security and data governance to limit their exposure to cyber-attacks; they will spend on automation to help teams grow productivity with current or lower headcount; they will continue their cloud investments to simplify their technology architectures, increase resilience, and to drive business agility. Security, cloud, data management and analytics, automation, and digital developers will all continue to see employment opportunities.
If this is the case, then why are tech vendors laying off headcount?
The slowdown in the American economy is a big reason. Tech providers that are laying of staff are heavily exposed to the American market.
- Salesforce – 68% Americas
- Facebook – 44% North America
- Genesys – around 60% in North America
Much of the messaging that these providers are giving is it is not that business is performing poorly – it is that growth is slowing down from the fast pace that many were witnessing when digital strategies accelerated.
Some of these tech providers might also be using the opportunity to “trim the fat” from their business – using the opportunity to get rid of the 2-3% of staff or teams that are underperforming. Interestingly, many of the people that are being laid off are from in or around the sales organisation. In some cases, tech providers are trimming products or services from their business and associated product, marketing, and technical staff are also being laid off.
While the majority of the impact is being felt in North America, there are certainly some people being laid off in Asia Pacific too. Particularly in companies where the development is done in Asia (India, China, ASEAN, etc.), there will be some impact when products or services are discontinued.
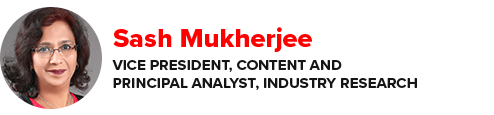
While it is not all bad news for tech talent, there is undoubtedly some nervousness. So this is what you should think about:
Change your immediate priorities. Ecosystm research found that 40% of digital/IT talent were looking to change employers in 2023. Nearly 60% of them were also thinking of changes in terms of where they live and their career.
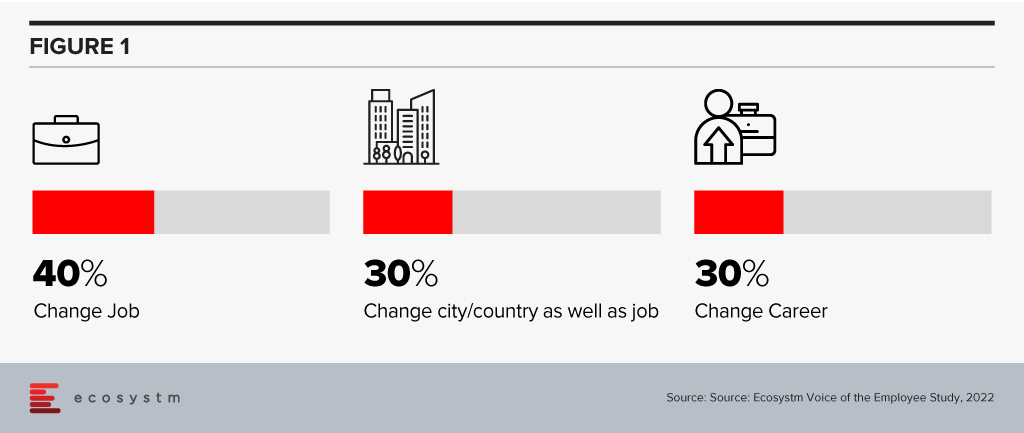
This may not be the right time to voluntarily change your job. Job profiles and industry requirements should guide your decision – by February 2023, a clearer image of the job market will emerge. Till then, upskill and get those certifications to stay relevant!
Be prepared for contract roles. With a huge pool of highly skilled technologists on the hunt for new opportunities, smaller technology providers and start-ups have a cause to celebrate. They have faced the challenge of getting the right talent largely because of their inability to match the remunerations offered by large tech firms.
These companies may still not be able to match the benefits offered by the large tech firms – but they provide opportunities to expand your portfolio, industry expertise, and experience in emerging technologies. This will see a change in job profiles. It is expected that more contractual roles will open up for the technology industry. You will have more opportunities to explore the option of working on short-term assignments and consulting projects – sometimes on multiple projects and with multiple clients at the same time.
Think about switching sides. The fact remains that digital and technology upgrades continue to be organisational priorities, across all industries. As organisations continue on their digital journeys, they have an immense potential to address their skills gap now with the availability of highly skilled talent. In a recently conducted Ecosystm roundtable, CIOs reported that new graduates have been demanding salaries as high as USD 200,000 per annum! Even banks and consultancies – typically the top paying businesses – have been finding it hard to afford these skills! These industries may well benefit from the layoffs.
If you look at technology job listings, we see no signs of the demand abating!
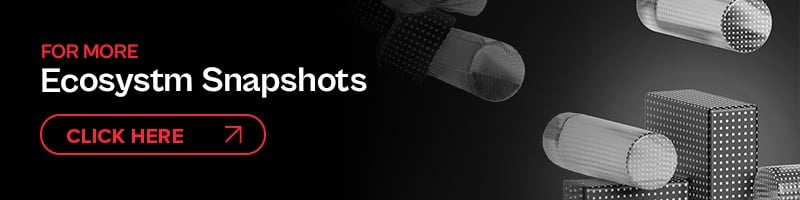
In recent years, businesses have faced significant disruptions. Organisations are challenged on multiple fronts – such as the continuing supply chain disruptions; an ongoing energy crisis that has led to a strong focus on sustainability; economic uncertainty; skills shortage; and increased competition from digitally native businesses. The challenge today is to build intelligent, data-driven, and agile businesses that can respond to the many changes that lie ahead.
Leading organisations are evaluating ways to empower the entire business with data, machine learning, automation, and AI to build agile, innovative, and customer-focused businesses.
Here are 7 steps that will help you deliver business value with data and AI:
- Understand the problems that need solutions. Before an organisation sets out on its data, automation, and AI journey, it is important to evaluate what it wants to achieve. This requires an engagement with the Tech/Data Teams to discuss the challenges it is trying to resolve.
- Map out a data strategy framework. Perhaps the most important part of this strategy are the data governance principles – or a new automated governance to enforce policies and rules automatically and consistently across data on any cloud.
- Industrialise data management & AI technologies. The cumulation of many smart, data-driven initiatives will ultimately see the need for a unified enterprise approach to data management, AI, and automation.
- Recognise the skills gap – and start closing it today. There is a real skills gap when it comes to the ability to identify and solve data-centric issues. Many businesses today turn to technology and business consultants and system integrators to help them solve the skills challenge.
- Re-start the data journey with a pilot. Real-world pilots help generate data and insights to build a business case to scale capabilities.
- Automate the outcomes. Modern applications have made it easier to automate actions based on insights. APIs let systems integrate with each other, share data, and trigger processes; and RPA helps businesses automate across applications and platforms.
- Learn and improve. Intelligent automation tools and adaptive AI/machine learning solutions exist today. What organisations need to do is to apply the learnings for continuous improvements.
Find more insights below.
Download The Future of Business: 7 Steps to Delivering Business Value with Data & AI as a PDF
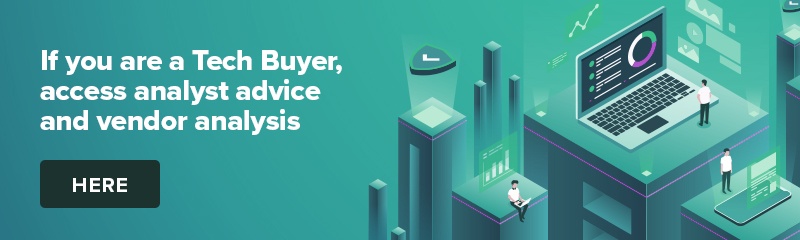
Verint has announced the intention to acquire Conversocial, a US-based social media management system provider for USD 50 million to integrate social messaging capabilities across Verint’s cloud platform. The deal is expected to be closed in Verint’s third quarter subject to customary closing conditions and regulatory clearances.
Verint has been expanding their digital engagement capabilities through acquisitions. In June, Verint expanded their Workforce Management (WFM) offerings to include AI-driven insights for better hiring decisions through the acquisition of HireIQ. To extend Verint’s omnichannel cloud Voice of the Customer (VoC) portfolio, Verint acquired Foresee. Verint is also building IVA capabilities and recently launched a low code version of their IVA solution to make it easier for brands to build the solution without the need for technical knowledge.
The Need to Enhance Digital Engagement
Using self-service and messaging as the first point of connection to engage with a brand is growing rapidly. It accelerated during the pandemic, and it is common now for individuals to engage with their financial institution, airlines, retail and others through social media. Now that customers are demanding it, brands are lifting their game and engaging with customers on the platform of their choices. This acquisition will allow Verint to deepen their digital engagement with customers across Marketing, Contact Centres and digital functions – it follows the pulse of today’s customers.
Digital discussions are accelerating and having a platform that can orchestrate as well as understand all the data from each digital and social messaging channel is important. Verint is taking the data discussion seriously and earlier this year they launched Engagement Data Management Solution (EDM). The ‘’data’’ piece is huge and cuts across functions – from back-office communications to gathering data across all channels and touchpoints. However, where this is going wrong for some enterprises is that all the data they collect sits in multiple repositories; in some instances the data has not been analysed for years! Managing the multiple social experiences, including data management and insights from these multiple sources, will be key to delivering proactive customer experience.
The data discussion is particularly significant for a vendor such as Verint – they are well known for their speech analytics and compliance management capabilities. These are all critical to managing multiple channels of conversation. They help agents to be accurate, efficient, and compliant; allow organisations to use asynchronous channels and social messaging and digital channels; immediately rectified errors through monitoring the data on the channels and so on. More importantly, they allow organisations to pick up points from conversations that can be passed on to Marketing to gauge the effectiveness of the messaging and campaigns. Organisations can ‘’identify and fix” problems by truly listening to customers.
Why Conversocial
If we look at the Conversocial customer stories, we realise how relevant their offerings are to industry requirements. They offer brands the ability to engage through an automated channel and chatbots. Whirlpool appears to have benefited by integrating channels to deliver better customer care as well as communicating with field engineers through WhatsApp. Another customer, Freshly – a meals delivery company – saw a spike in incoming queries at the start of the pandemic. They were able to use automation to ease of the load and say that 50% of the conversations were handled in-channel through automation without the need for human agent intervention. They were also able to use Facebook Messenger as a preferred contact channel and decreased their cost-per-contact.
This acquisition demonstrates how Verint is taking the digital and the data discussion seriously. CX Vendors that do not move fast in building end-to-end digital capabilities will find it hard to compete in a highly competitive CX market.
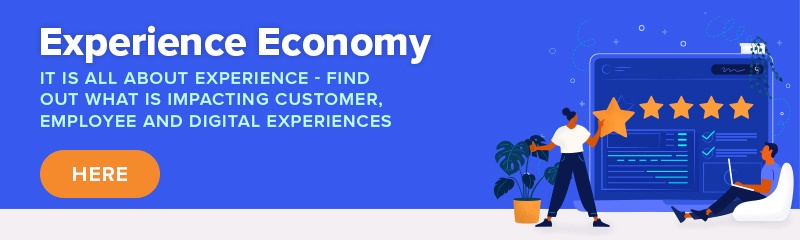