AI has become a business necessity today, catalysing innovation, efficiency, and growth by transforming extensive data into actionable insights, automating tasks, improving decision-making, boosting productivity, and enabling the creation of new products and services.
Generative AI stole the limelight in 2023 given its remarkable advancements and potential to automate various cognitive processes. However, now the real opportunity lies in leveraging this increased focus and attention to shine the AI lens on all business processes and capabilities. As organisations grasp the potential for productivity enhancements, accelerated operations, improved customer outcomes, and enhanced business performance, investment in AI capabilities is expected to surge.
In this eBook, Ecosystm VP Research Tim Sheedy and Vinod Bijlani and Aman Deep from HPE APAC share their insights on why it is crucial to establish tailored AI capabilities within the organisation.
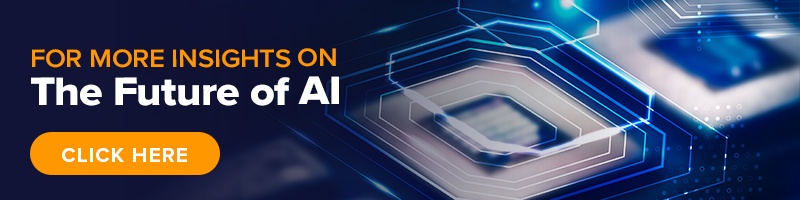
2024 will be another crucial year for tech leaders – through the continuing economic uncertainties, they will have to embrace transformative technologies and keep an eye on market disruptors such as infrastructure providers and AI startups. Ecosystm analysts outline the key considerations for leaders shaping their organisations’ tech landscape in 2024.
Navigating Market Dynamics

Continuing Economic Uncertainties. Organisations will focus on ongoing projects and consider expanding initiatives in the latter part of the year.
Popularity of Generative AI. This will be the time to go beyond the novelty factor and assess practical business outcomes, allied costs, and change management.
Infrastructure Market Disruption. Keeping an eye out for advancements and disruptions in the market (likely to originate from the semiconductor sector) will define vendor conversations.
Need for New Tech Skills. Generative AI will influence multiple tech roles, including AIOps and IT Architecture. Retaining talent will depend on upskilling and reskilling.
Increased Focus on Governance. Tech vendors are guide tech leaders on how to implement safeguards for data usage, sharing, and cybersecurity.
5 Key Considerations for Tech Leaders
#1 Accelerate and Adapt: Streamline IT with a DevOps Culture
Over the next 12-18 months, advancements in AI, machine learning, automation, and cloud-native technologies will be vital in leveraging scalability and efficiency. Modernisation is imperative to boost responsiveness, efficiency, and competitiveness in today’s dynamic business landscape.
The continued pace of disruption demands that organisations modernise their applications portfolios with agility and purpose. Legacy systems constrained by technical debt drag down velocity, impairing the ability to deliver new innovative offerings and experiences customers have grown to expect.
Prioritising modernisation initiatives that align with key value drivers is critical. Technology leaders should empower development teams to move beyond outdated constraints and swiftly deploy enhanced applications, microservices, and platforms.

#2 Empowering Tomorrow: Spring Clean Your Tech Legacy for New Leaders
Modernising legacy systems is a strategic and inter-generational shift that goes beyond simple technical upgrades. It requires transformation through the process of decomposing and replatforming systems – developed by previous generations – into contemporary services and signifies a fundamental realignment of your business with the evolving digital landscape of the 21st century.
The essence of this modernisation effort is multifaceted. It not only facilitates the integration of advanced technologies but also significantly enhances business agility and drives innovation. It is an approach that prepares your organisation for impending skill gaps, particularly as the older workforce begins to retire over the next decade. Additionally, it provides a valuable opportunity to thoroughly document, reevaluate, and improve business processes. This ensures that operations are not only efficient but also aligned with current market demands, contemporary regulatory standards, and the changing expectations of customers.

#3 Employee Retention: Consider the Strategic Role of Skills Acquisition
The agile, resilient organisation needs to be able to respond at pace to any threat or opportunity it faces. Some of this ability to respond will be related to technology platforms and architectures, but it will be the skills of employees that will dictate the pace of reform. While employee attrition rates will continue to decline in 2024 – but it will be driven by skills acquisition, not location of work.
Organisations who offer ongoing staff training – recognising that their business needs new skills to become a 21st century organisation – are the ones who will see increasing rates of employee retention and happier employees. They will also be the ones who offer better customer experiences, driven by motivated employees who are committed to their personal success, knowing that the organisation values their performance and achievements.

#4 Next-Gen IT Operations: Explore Gen AI for Incident Avoidance and Predictive Analysis
The integration of Generative AI in IT Operations signifies a transformative shift from the automation of basic tasks, to advanced functions like incident avoidance and predictive analysis. Initially automating routine tasks, Generative AI has evolved to proactively avoiding incidents by analysing historical data and current metrics. This shift from proactive to reactive management will be crucial for maintaining uninterrupted business operations and enhancing application reliability.
Predictive analysis provides insight into system performance and user interaction patterns, empowering IT teams to optimise applications pre-emptively, enhancing efficiency and user experience. This also helps organisations meet sustainability goals through accurate capacity planning and resource allocation, also ensuring effective scaling of business applications to meet demands.

#5 Expanding Possibilities: Incorporate AI Startups into Your Portfolio
While many of the AI startups have been around for over five years, this will be the year they come into your consciousness and emerge as legitimate solutions providers to your organisation. And it comes at a difficult time for you!
Most tech leaders are looking to reduce technical debt – looking to consolidate their suppliers and simplify their tech architecture. Considering AI startups will mean a shift back to more rather than fewer tech suppliers; a different sourcing strategy; more focus on integration and ongoing management of the solutions; and a more complex tech architecture.
To meet business requirements will mean that business cases will need to be watertight – often the value will need to be delivered before a contract has been signed.

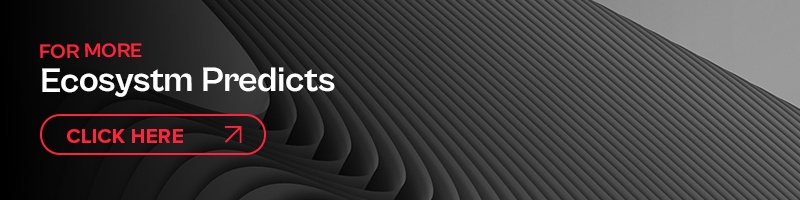
Hewlett Packard Enterprise (HPE) has entered into a definitive agreement to acquire Juniper Networks for USD 40 per share, totaling an equity value of about USD 14 Billion. This strategic move is aimed to enhance HPE’s portfolio by focusing on higher-growth solutions and reinforcing their high-margin networking business. HPE expects to double their networking business, positioning the combined entity as a leader in networking solutions. With the growing demand for secure, unified technology driven by AI and hybrid cloud trends, HPE aims to offer comprehensive, disruptive solutions that connect, protect, and analyse data from edge to cloud.
This would also be the organisation’s largest deal since becoming an independent company in 2015. The acquisition is expected to be completed by late 2024 or early 2025.
Ecosystm analysts Darian Bird and Richard Wilkins provide their insights on the HPE acquisition and its implications for the tech market.
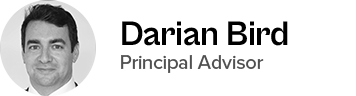
Converging Networking and Security
One of the big drawcards for HPE is Juniper’s Mist AI. The networking vendors have been racing to catch up – both in capabilities and in marketing. The acquisition though will give HPE a leadership position in network visibility and manageability. With GreenLake and soon Mist AI, HPE will have a solid AIOps story across the entire infrastructure.
HPE has been working steadily towards becoming a player in the converged networking-security space. They integrated Silver Peak well to make a name for themselves in SD-WAN and last year acquiring Axis Security gave them the Zero Trust Network Access (ZTNA), Secure Web Gateway (SWG), and Cloud Access Security Broker (CASB) modules in the Secure Service Edge (SSE) stack. Bringing all of this to the market with Juniper’s networking prowess positions HPE as a formidable player, especially as the Secure Access Service Edge (SASE) market gains momentum.
As the market shifts towards converged SASE, there will only be more interest in the SD-WAN and SSE vendors. In just over one year, Cato Networks and Netskope have raised funds, Check Point acquired Perimeter 81, and Versa Networks has made noises about an IPO. The networking and security players are all figuring out how they can deliver a single-vendor SASE.
Although HPE’s strategic initiatives signal a robust market position, potential challenges arise from the overlap between Aruba and Juniper. However, the distinct focus on the edge and data center, respectively, may help alleviate these concerns. The acquisition also marks HPE’s foray into the telecom space, leveraging its earlier acquisition of Athonet and establishing a significant presence among service providers. This expansion enhances HPE’s overall market influence, posing a challenge to the long-standing dominance of Cisco.
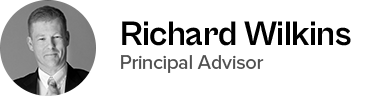
The strategic acquisition of Juniper Networks by HPE can make a transformative leap in AIOps and Software-Defined Networking (SDN). There is a potential for this to establish a new benchmark in IT management.
AI in IT Operations Transformation
The integration of Mist’s AI-driven wireless solutions and HPE’s SDN is a paradigm shift in IT operations management and will help organisations transition from a reactive to a predictive and proactive model. Mist’s predictive analytics, coupled with HPE’s SDN capabilities, empower networks to dynamically adjust to user demands and environmental changes, ensuring optimal performance and user experience. Marvis, Mist’s Virtual Network Assistant (VNA), adds conversational troubleshooting capabilities, enhancing HPE’s network solutions. The integration envisions an IT ecosystem where Juniper’s AI augments HPE’s InfoSight, providing deeper insights into network behaviour, preemptive security measures, and more autonomous IT operations.
Transforming Cloud and Edge Computing
The incorporation of Juniper’s AI into HPE’s cloud and edge computing solutions promises a significant improvement in data processing and management. AI-driven load balancing and resource allocation mechanisms will significantly enhance multi-cloud environment efficiency, ensuring robust and seamless cloud services, particularly vital in IoT applications where real-time data processing is critical. This integration not only optimises cloud operations but also has the potential to align with HPE’s commitment to sustainability, showcasing how AI advancements can contribute to energy conservation.
In summary, HPE’s acquisition of Juniper Networks, and specifically the integration of the Mist AI platform, is a pivotal step towards an AI-driven, efficient, and predictive IT infrastructure. This can redefine the standards in AIOps and SDN, creating a future where IT systems are not only reactive but also intuitively adaptive to the evolving demands of the digital landscape.
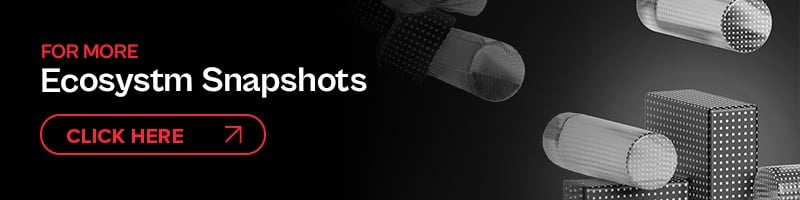
In 2024, business and technology leaders will leverage the opportunity presented by the attention being received by Generative AI engines to test and integrate AI comprehensively across the business. Many organisations will prioritise the alignment of their initial Generative AI initiatives with broader AI strategies, establishing distinct short-term and long-term goals for their AI investments.
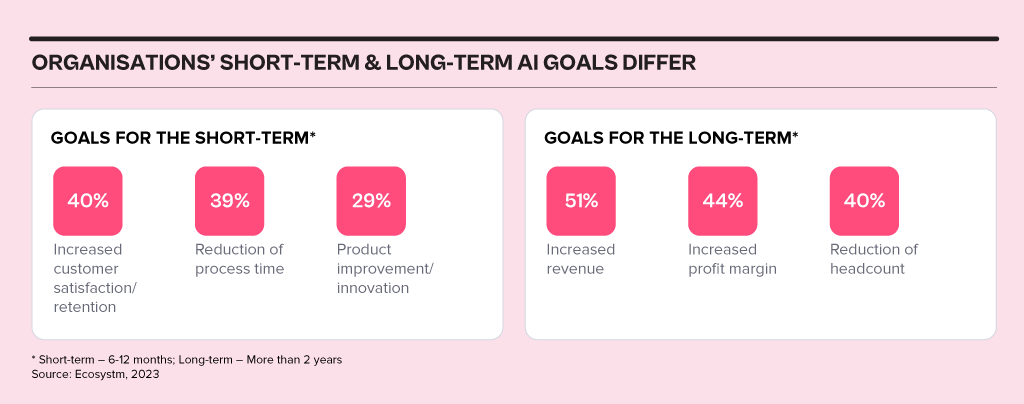
AI adoption will influence business processes, technology skills, and, in turn, reshape the product/service offerings of AI providers.
Ecosystm analysts Achim Granzen, Peter Carr, Richard Wilkins, Tim Sheedy, and Ullrich Loeffler present the top 5 AI trends in 2024.
Click here to download ‘Ecosystm Predicts: Top 5 AI Trends in 2024.
#1 By the End of 2024, Gen AI Will Become a ‘Hygiene Factor’ for Tech Providers
AI has widely been commended as the ‘game changer’ that will create and extend the divide between adopters and laggards and be the deciding factor for success and failure.
Cutting through the hype, strategic adoption of AI is still at a nascent stage and 2024 will be another year where companies identify use cases, experiment with POCs, and commit renewed efforts to get their data assets in order.
The biggest impact of AI will be derived from integrated AI capability in standard packaged software and products – and this will include Generative AI. We will see a plethora of product releases that seamlessly weave Generative AI into everyday tools generating new value through increased efficiency and user-friendliness.
Technology will be the first industry where AI becomes the deciding factor between success and failure; tech providers will be forced to deliver on their AI promises or be left behind.
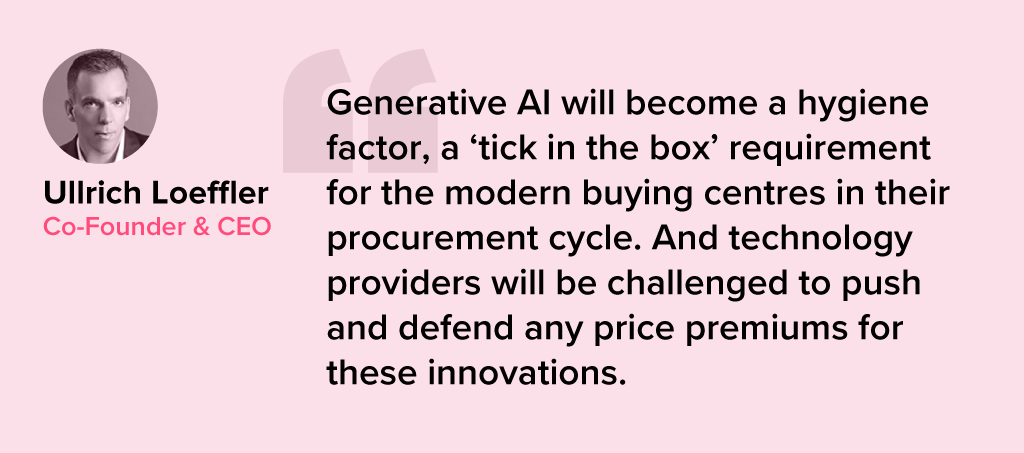
#2 Gen AI Will Disrupt the Role of IT Architects
Traditionally, IT has relied on three-tier architectures for applications, that faced limitations in scalability and real-time responsiveness. The emergence of microservices, containerisation, and serverless computing has paved the way for event-driven designs, a paradigm shift that decouples components and use events like user actions or data updates as triggers for actions across distributed services. This approach enhances agility, scalability, and flexibility in the system.
The shift towards event-driven designs and advanced architectural patterns presents a compelling challenge for IT Architects, as traditionally their role revolved around designing, planning and overseeing complex systems.
Generative AI is progressively demonstrating capabilities in architectural design through pattern recognition, predictive analytics, and automated decision-making.
With the adoption of Generative AI, the role of an IT Architect will change into a symbiotic relationship where human expertise collaborates with AI insights.
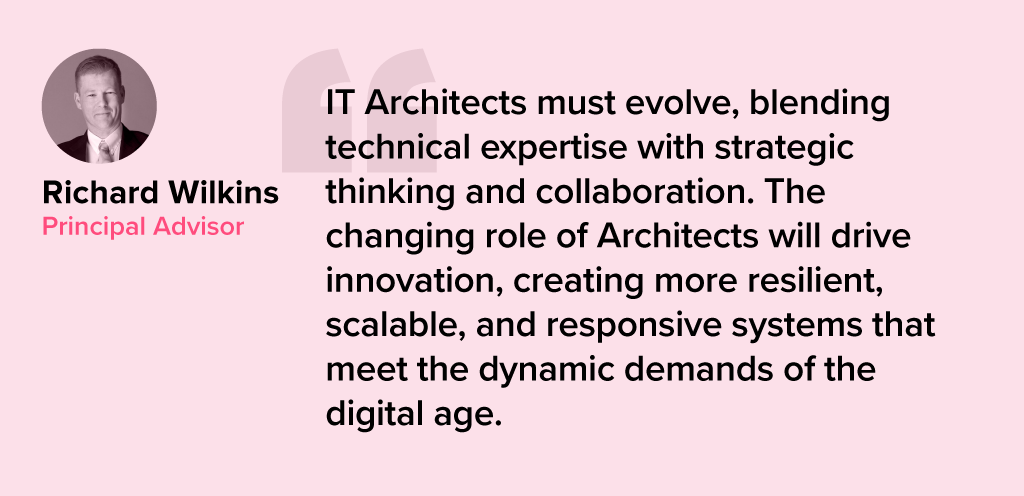
#3 Gen AI Adoption Will be Confined to Specific Use Cases
A little over a year ago, a new era in AI began with the initial release of OpenAI’s ChatGPT. Since then, many organisations have launched Generative AI pilots.
In its second-year enterprises will start adoption – but in strictly defined and limited use cases. Examples such as Microsoft Copilot demonstrate an early adopter route. While productivity increases for individuals can be significant, its enterprise impact is unclear (at this time).
But there are impactful use cases in enterprise knowledge and document management. Organisations across industries have decades (or even a century) of information, including digitised documents and staff expertise. That treasure trove of information can be made accessible through cognitive search and semantic answering, driven by Generative AI.
Generative AI will provide organisations with a way to access, distill, and create value out of that data – a task that may well be impossible to achieve in any other way.
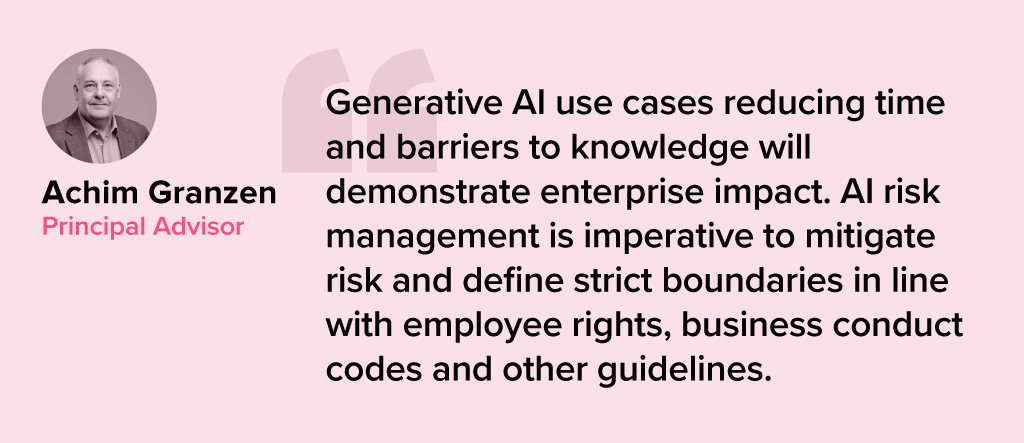
#4 Gen AI Will Get Press Inches; ‘Traditional’ AI Will Do the Hard Work
While the use cases for Generative AI will continue to expand, the deployment models and architectures for enterprise Generative AI do not add up – yet.
Running Generative AI in organisations’ data centres is costly and using public models for all but the most obvious use cases is too risky. Most organisations opt for a “small target” strategy, implementing Generative AI in isolated use cases within specific processes, teams, or functions. Justifying investment in hardware, software, and services for an internal AI platform is challenging when the payback for each AI initiative is not substantial.
“Traditional AI/ML” will remain the workhorse, with a significant rise in use cases and deployments. Organisations are used to investing for AI by individual use cases. Managing process change and training is also more straightforward with traditional AI, as the changes are implemented in a system or platform, eliminating the need to retrain multiple knowledge workers.
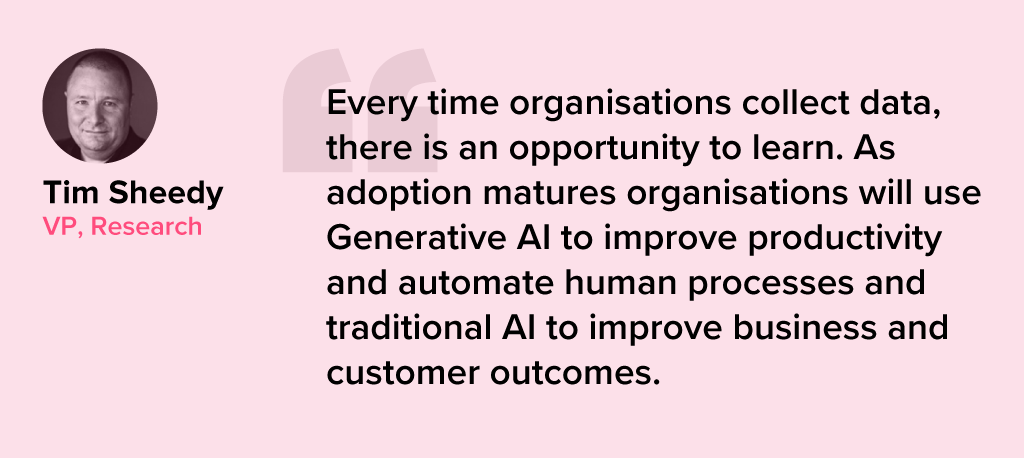
#5 AI Will Pioneer a 21st Century BPM Renaissance
As we near the 25-year milestone of the 21st century, it becomes clear that many businesses are still operating with 20th-century practices and philosophies.
AI, however, represents more than a technological breakthrough; it offers a new perspective on how businesses operate and is akin to a modern interpretation of Business Process Management (BPM). This development carries substantial consequences for digital transformation strategies. To fully exploit the potential of AI, organisations need to commit to an extensive and ongoing process spanning the collection, organisation, and expansion of data, to integrating these insights at an application and workflow level.
The role of AI will transcend technological innovation, becoming a driving force for substantial business transformation. Sectors that specialise in workflow, data management, and organisational transformation are poised to see the most growth in 2024 because of this shift.
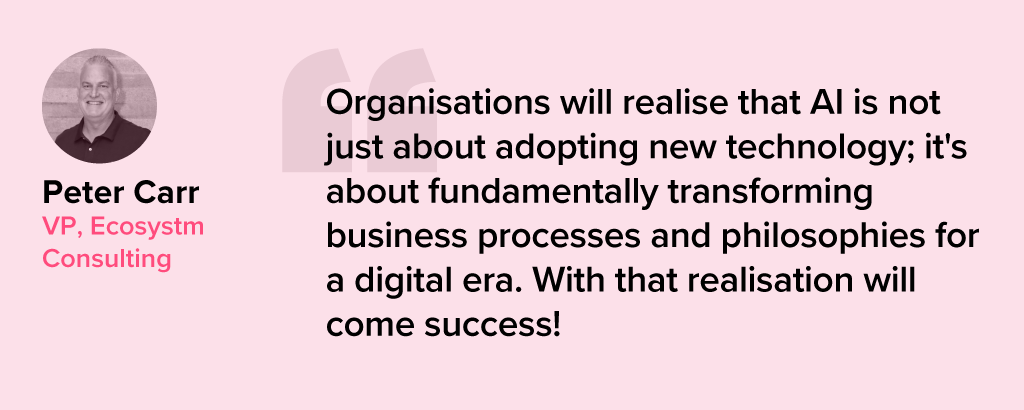
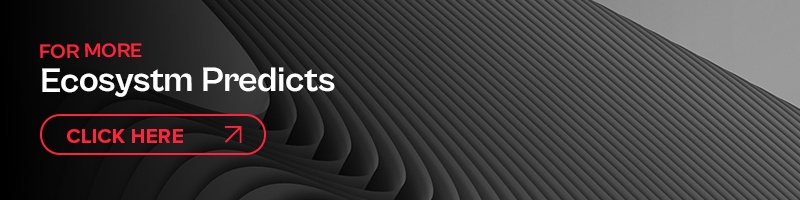
Setting and achieving Sustainability goals is complex in BFSI. To be truly sustainable, organisations need to:
- Reduce internal energy consumption and carbon footprint
- Fund the transition to decarbonisation in high emission industries
- Introduce “green” customer products and services
- Monitor carbon data for financed emissions
Data and AI have the potential to assist in achieving these objectives, provided they are used effectively. Here is how.
Download ‘Driving Sustainability with Data and AI in Financial Services’ as a PDF
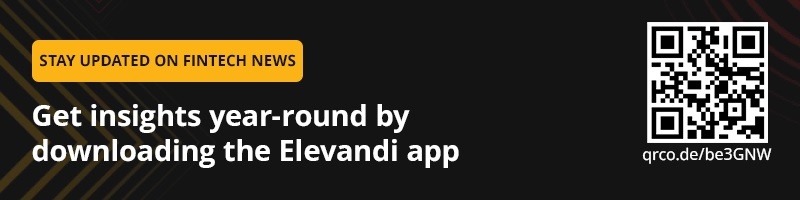
Leading Banking and Financial Services organisations play a crucial role in financing sustainability transition. They have the infrastructure and resources to kickstart their own sustainability journey. But beyond that, they also have a greater role in building a sustainable value chain.
This extends to helping the traditional economy to transition; green investments to promote organisations with the right intentions; and empowering their customers to make environmentally-friendly choices.
As a technology leader in BFSI, you are an integral part of your organisation’s sustainability journey. Here are 5 ways in which BFSI tech leaders can support their organisations to turn sustainability intentions into reality.
Align tech with business goals and strategy. Think like a business leader and understand larger goals beyond technology deployments to empower your team.
View reporting as more than a checklist. You are in an ideal position to demonstrate the value of data insights beyond reporting mandates to the leadership team – link them to larger business outcomes.
Build intelligence into your facilities and assets. Consider investing in an intelligent enterprise asset management solution to automate asset and infrastructure management, remotely monitor and manage asset operations, and achieve sustainable business outcomes.
Automate your infrastructure allocation. You are increasingly using FinOps tools and other predictive analytics dashboards for cost and resource optimisation – extend the use for greater energy efficiency.
Understand your organisation’s unique sustainability journey. Seek independent opinion from third parties to empower your organisation to take the first step in the sustainability strategy, derive insights from data assets, and create market differentiation.
Read on to find more.
Download 5 Sustainability Actions for BFSI Tech Leaders as a PDF
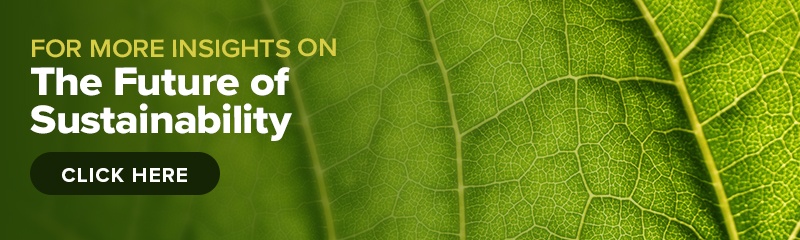
The Internet of Things (IoT) solutions require data integration capabilities to help business leaders solve real problems. Ecosystm research finds that the problem is that more than half of all organisations are finding integration a key challenge – right behind security (Figure 1). So, chances are, you are facing similar challenges.
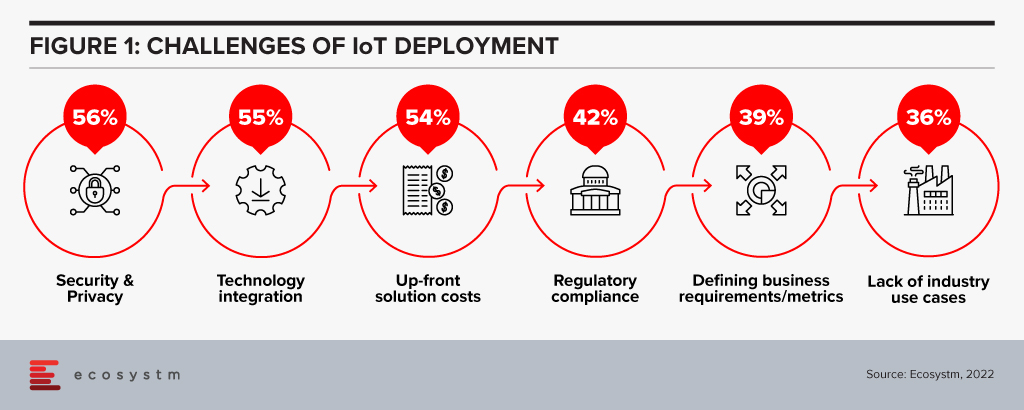
This should not be taken as a criticism of IoT; just a wake-up call for all those seeking to implement what has long been test-lab technology into an enterprise environment. I love absolutely everything about IoT. IT is an essential technology. Contemporary sensor technologies are at the core of everything. It’s just that there are a lot of organisations not doing it right.
Like many technologists, I was hooked on IoT since I first sat in a Las Vegas AWS re: invent conference breakout session in 2015 and learned about MQTT protocols applied to any little thing, and how I could re-order laundry detergent or beer with an AWS button, that clumsy precursor to Alexa.
Parts of that presentation have stayed with me to this day. Predict and act. What business doesn’t want to be able to do that better? I can still see the room. I still have those notes. And I’m still working to help others embrace the full potential of this must-have enterprise capability.
There is no doubt that IoT is the Cinderella of smart cities. Even digital twinning. Without it, there is no story. It is critical to contemporary organisations because of the real-time decision-making data it can provide into significant (Industry 4.0) infrastructure and service investments. That’s worth repeating. It is critical to supporting large scale capital investments and anyone who has been in IT for any length of time knows that vindicating the need for new IT investments to capital holders is the most elusive of business demands.
But it is also a bottom-up technology that requires a top-down business case – a challenge also faced by around 40% of organisations in the Ecosystm study – and a number of other architectural components to realise its full cost-benefit or capital growth potential. Let’s not quibble, IoT is fundamental to both operational and strategic data insights, but it is not the full story.
If IoT is the belle of the smart cities ball, then integration is the glass slipper that ties the whole story together. After four years as head of technology for a capital city deeply committed to the Smart City vision, if there was one area of IoT investment I was constantly wishing I had more of, it was integration. We were drowning in data but starved of the skills and technology to deliver true strategic insights outside of single-function domains.
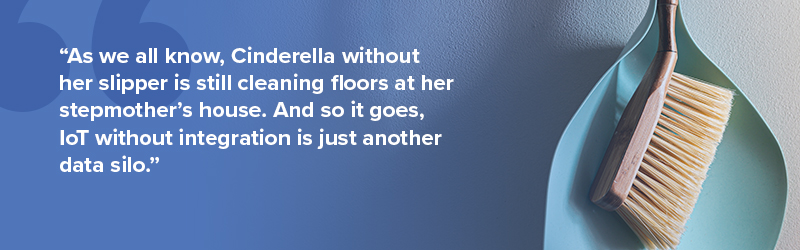
This reality in no way diminishes the value of IoT. Nor is it either a binary or chicken-and-egg question of whether to invest in IoT or integration. In fact, the symbiotic market potential for both IoT and integration solutions in asset-intensive businesses is not only huge but necessary.
IoT solutions are fundamental contemporary technologies that provide the opportunity for many businesses to do well in areas they would otherwise continue to do very poorly. They provide a foundation for digital enablement and a critical gateway to analytics for real-time and predictive decision making.
When applied strategically and at scale, IoT provides a magical technology capability. But the bottom line is that even magic technology can never carry the day when left to do the work of other solutions. If you have already plunged into IoT then chances are it has already become your next data silo. The question is now, what you are going to do about it?
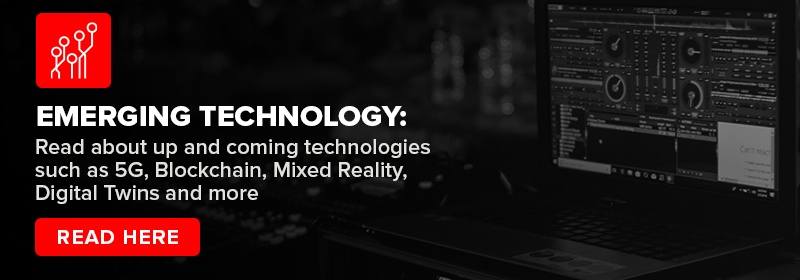
Organisations have found that it is not always desirable to send data to the cloud due to concerns about latency, connectivity, energy, privacy and security. So why not create learning processes at the Edge?
What challenges does IoT bring?
Sensors are now generating such an increasing volume of data that it is not practical that all of it be sent to the cloud for processing. From a data privacy perspective, some sensor data is sensitive and sending data and images to the cloud will be subject to privacy and security constraints.
Regardless of the speed of communications, there will always be a demand for more data from more sensors – along with more security checks and higher levels of encryption – causing the potential for communication bottlenecks.
As the network hardware itself consumes power, sending a constant stream of data to the cloud can be taxing for sensor devices. The lag caused by the roundtrip to the cloud can be prohibitive in applications that require real-time response inputs.
Machine learning (ML) at the Edge should be prioritised to leverage that constant flow of data and address the requirement for real-time responses based on that data. This should be aided by both new types of ML algorithms and by visual processing units (VPUs) being added to the network.
By leveraging ML on Edge networks in production facilities, for example, companies can look out for potential warning signs and do scheduled maintenance to avoid any nasty surprises. Remember many sensors are linked intrinsically to public safety concerns such as water processing, supply of gas or oil, and public transportation such as metros or trains.
Ecosystm research shows that deploying IoT has its set of challenges (Figure 1) – many of these challenges can be mitigated by processing data at the Edge.
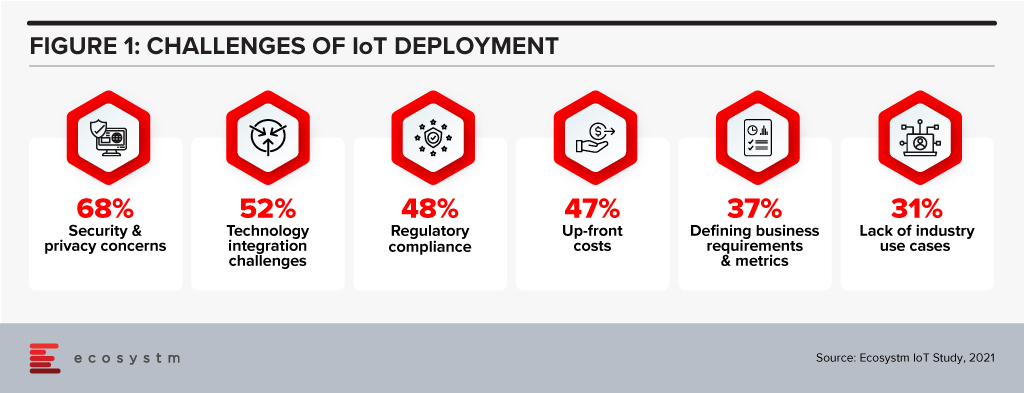
Predictive analytics is a fundamental value proposition for IoT, where responding faster to issues or taking action before issues occur, is key to a high return on investment. So, using edge computing for machine learning located within or close to the point of data gathering can in some cases be a more practical or socially beneficial approach.
In IoT the role of an edge computer is to pre-process data and act before the data is passed on to the main server. This allows a faster, low latency response and minimal traffic between the cloud server processing and the Edge. However, a better understanding of the benefits of edge computing is required if it has to be beneficial for a number of outcomes.
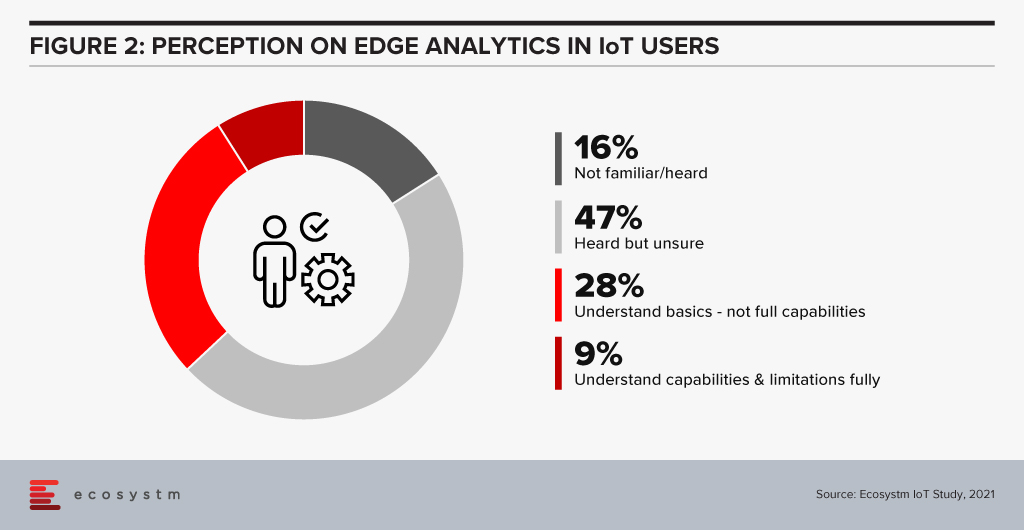

If we can get machine learning happening in the field, at the Edge, then we reduce the time lag and also create an extra trusted layer in unmanned production or automated utilities situations. This can create more trusted environments in terms of possible threats to public services.
What kind of examples of machine learning in the field can we see?
Healthcare
Health systems can improve hospital patient flow through machine learning (ML) at the Edge. ML offers predictive models to assist decision-makers with complex hospital patient flow information based on near real-time data.
For example, an academic medical centre created an ML pipeline that leveraged all its data – patient administration, EHR and clinical and claims data – to create learnings that could predict length of stay, emergency department (ED) arrival models, ED admissions, aggregate discharges, and total bed census. These predictive models proved effective as the medical centre reduced patient wait times and staff overtime and was able to demonstrate improved patient outcomes. And for a medical centre that use sensors to monitor patients and gather requests for medicine or assistance, Edge processing means keeping private healthcare data in-house rather than sending it off to cloud servers.
Retail
A retail store could use numerous cameras for self-checkout and inventory management and to monitor foot traffic. Such specific interaction details could slow down a network and can be replaced by an on-site Edge server with lower latency and a lower total cost. This is useful for standalone grocery pop-up sites such as in Sweden and Germany.
In Retail, k-nearest neighbours is often used in ML for abnormal activity analysis – this learning algorithm can also be used for visual pattern recognition used as part of retailers’ loss prevention tactics.
Summary
Working with the data locally on the Edge, creates reduced latency, reduced cloud usage and costs, independence from a network connection, more secure data, and increased data privacy.
Cloud and Edge computing that uses machine learning can together provide the best of both worlds: decentralised local storage, processing and reaction, and then uploading to the cloud, enabling additional insights, data backups (redundancy), and remote access.
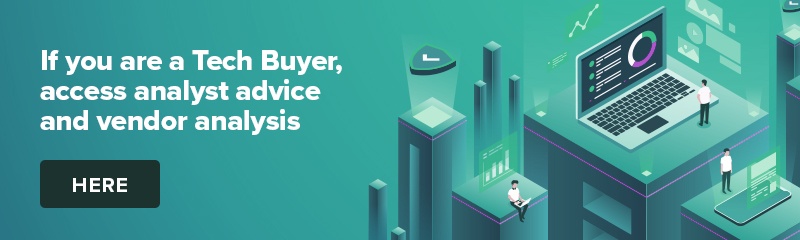