Ecosystm research shows that cybersecurity is the most discussed technology at the Board and Management level, driven by the increasing sophistication of cyber threats and the rapid adoption of AI. While AI enhances security, it also introduces new vulnerabilities. As organisations face an evolving threat landscape, they are adopting a more holistic approach to cybersecurity, covering prevention, detection, response, and recovery.
In 2025, cybersecurity leaders will continue to navigate a complex mix of technological advancements, regulatory pressures, and changing business needs. To stay ahead, organisations will prioritise robust security solutions, skilled professionals, and strategic partnerships.
Ecosystm analysts Darian Bird, Sash Mukherjee, and Simona Dimovski present the key cybersecurity trends for 2025.
Click here to download ‘Securing the AI Frontier: Top 5 Cyber Trends for 2025’ as a PDF
1. Cybersecurity Will Be a Critical Differentiator in Corporate Strategy
The convergence of geopolitical instability, cyber weaponisation, and an interconnected digital economy will make cybersecurity a cornerstone of corporate strategy. State-sponsored cyberattacks targeting critical infrastructure, supply chains, and sensitive data have turned cyber warfare into an operational reality, forcing businesses to prioritise security.
Regulatory pressures are driving this shift, mandating breach reporting, data sovereignty, and significant penalties, while international cybersecurity norms compel companies to align with evolving standards to remain competitive.
The stakes are high. Stakeholders now see cybersecurity as a proxy for trust and resilience, scrutinising both internal measures and ecosystem vulnerabilities.
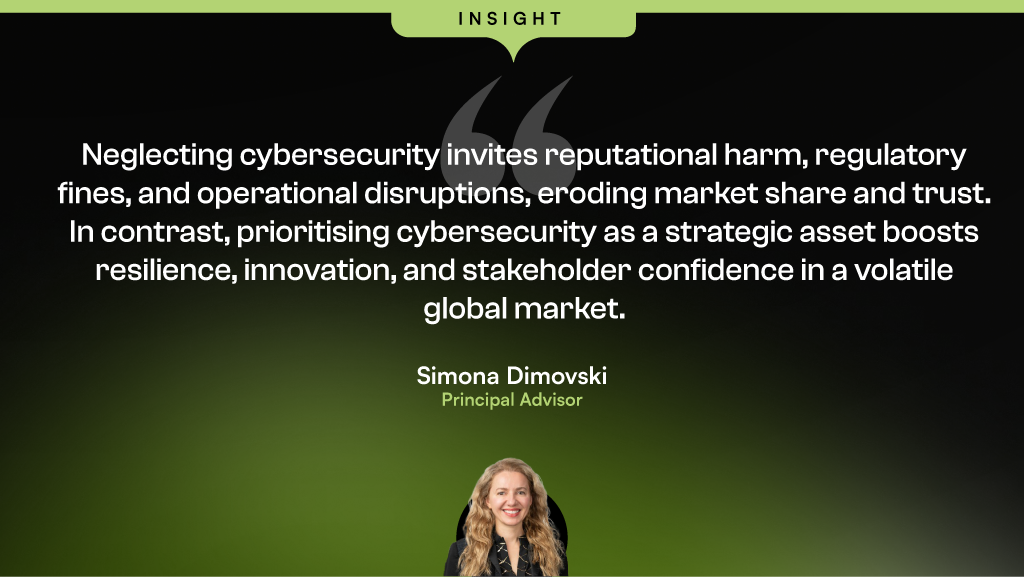
2. Zero Trust Architectures Will Anchor AI-Driven Environments
The future of cybersecurity lies in never trusting, always verifying – especially where AI is involved.
In 2025, the rise of AI-driven systems will make Zero Trust architectures vital for cybersecurity. Unlike traditional networks with implicit trust, AI environments demand stricter scrutiny due to their reliance on sensitive data, autonomous decisions, and interconnected systems. The growing threat of adversarial attacks – data poisoning, model inversion, and algorithmic manipulation – highlights the urgency of continuous verification.
Global forces are driving this shift. Regulatory mandates like the EU’s DORA, the US Cybersecurity Executive Order, and the NIST Zero Trust framework call for robust safeguards for critical systems. These measures align with the growing reliance on AI in high-stakes sectors like Finance, Healthcare, and National Security.
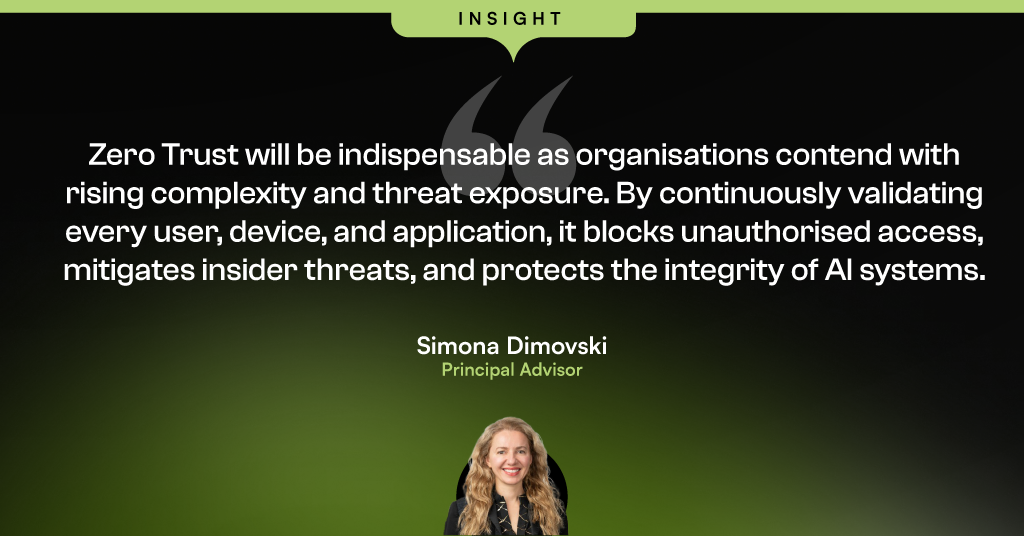
3. Organisations Will Proactively Focus on AI Governance & Data Privacy
Organisations are caught between excitement and uncertainty regarding AI. While the benefits are immense, businesses struggle with the complexities of governing AI. The EU AI Act looms large, pushing global organisations to brace for stricter regulations, while a rise in shadow IT sees business units bypassing traditional IT to deploy AI independently.
In this environment of regulatory ambiguity and organisational flux, CISOs and CIOs will prioritise data privacy and governance, proactively securing organisations with strong data frameworks and advanced security solutions to stay ahead of emerging regulations.
Recognising that AI will be multi-modal, multi-vendor, and hybrid, organisations will invest in model orchestration and integration platforms to simplify management and ensure smoother compliance.
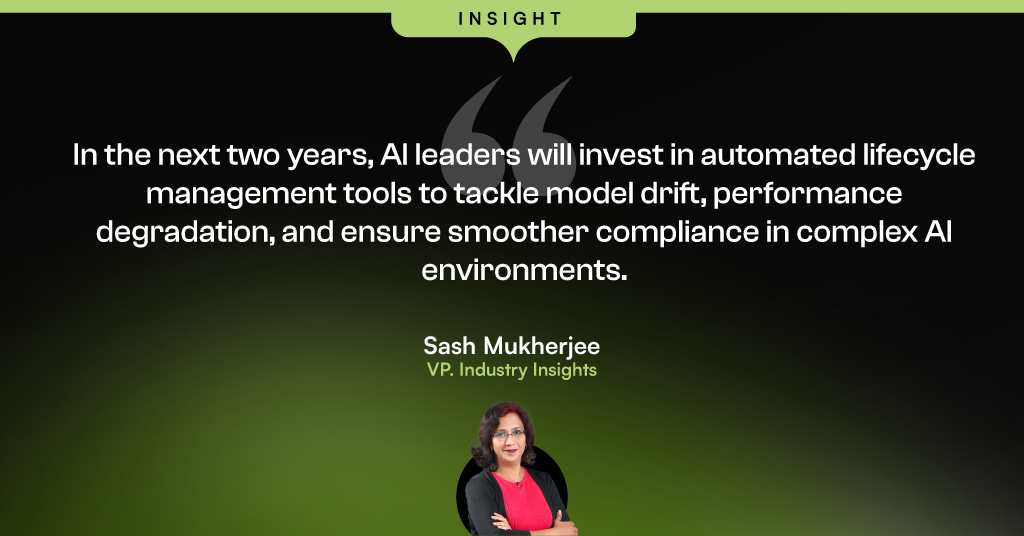
4. Network & Security Stacks Will Streamline Through Converged Platforms
This shift stems from the need for unified management, cost efficiency, and the recognition that standardisation enhances security posture.
Tech providers are racing to deliver comprehensive network and security platforms.
Recent M&A moves by HPE (Juniper), Palo Alto Networks (QRadar SaaS), Fortinet (Lacework), and LogRhythm (Exabeam) highlight this trend. Rising player Cato Networks is capitalising on mid-market demand for single-provider solutions, with many customers planning to consolidate vendors in their favour. Meanwhile, telecoms are expanding their SASE offerings to support organisations adapting to remote work and growing cloud adoption.
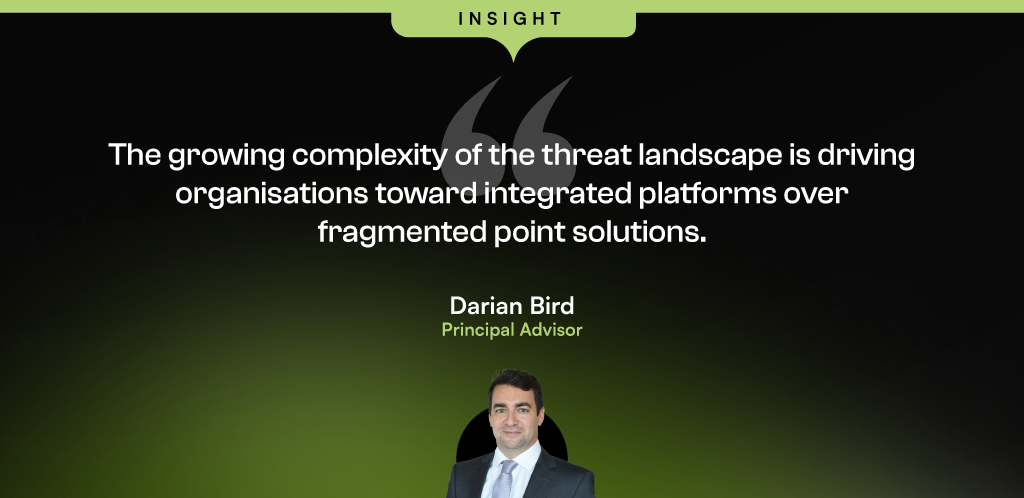
5. AI Will Be Widely Used to Combat AI-Powered Threats in Real-time
By 2025, the rise of AI-powered cyber threats will demand equally advanced AI-driven defences.
Threat actors are using AI to launch adaptive attacks like deepfake fraud, automated phishing, and adversarial machine learning, operating at a speed and scale beyond traditional defences.
Real-time AI solutions will be essential for detection and response.
Nation-state-backed advanced persistent threat (APT) groups and GenAI misuse are intensifying these challenges, exploiting vulnerabilities in critical infrastructure and supply chains. Mandatory reporting and threat intelligence sharing will strengthen AI defences, enabling real-time adaptation to emerging threats.
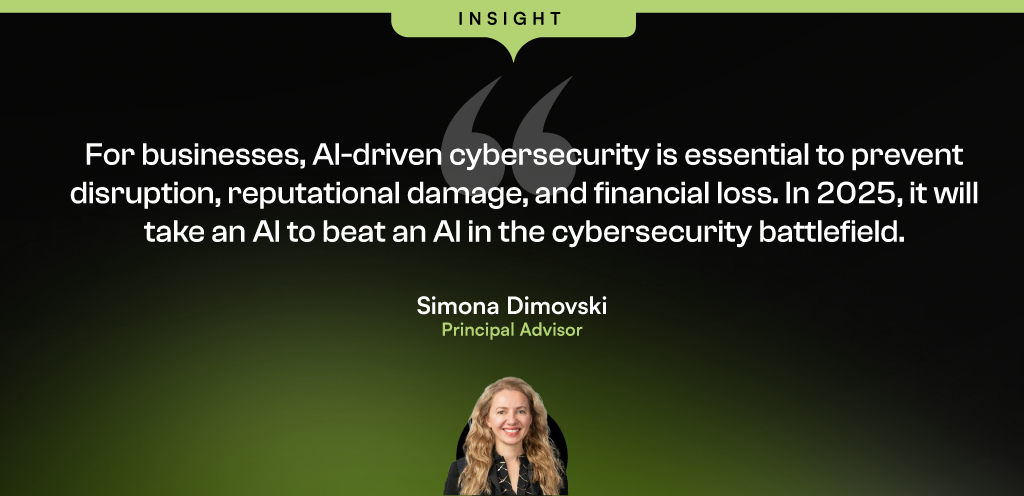
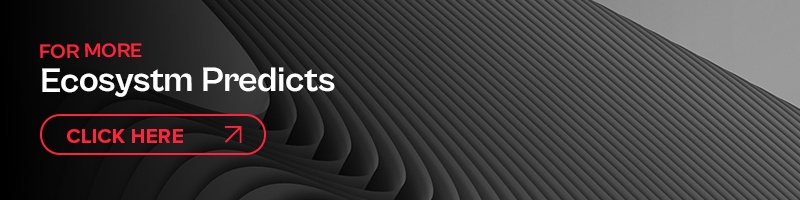
As AI evolves, the supporting infrastructure has become a crucial consideration for organisations and technology companies alike. AI demands massive processing power and efficient data handling, making high-performance computing clusters and advanced data management systems essential. Scalability, efficiency, security, and reliability are key to ensuring AI systems handle increasing demands and sensitive data responsibly.
Data centres must evolve to meet the increasing demands of AI and growing data requirements.
Equinix recently hosted technology analysts at their offices and data centre facilities in Singapore and Sydney to showcase how they are evolving to maintain their leadership in the colocation and interconnection space.
Equinix is expanding in Latin America, Africa, the Middle East, and Asia Pacific. In Asia Pacific, they recently opened data centres in Kuala Lumpur and Johor Bahru, with capacity additions in Mumbai, Sydney, Melbourne, Tokyo, and Seoul. Plans for the next 12 months include expanding in existing cities and entering new ones, such as Chennai and Jakarta.
Ecosystm analysts comment on Equinix’s growth potential and opportunities in Asia Pacific.
Small Details, Big Impact
TIM SHEEDY. The tour of the new Equinix data centre in Sydney revealed the complexity of modern facilities. For instance, the liquid cooling system, essential for new Nvidia chipsets, includes backup cold water tanks for redundancy. Every system and process is designed with built-in redundancy.
As power needs grow, so do operational and capital costs. The diesel generators at the data centre, comparable to a small power plant, are supported by multiple fuel suppliers from several regions in Sydney to ensure reliability during disasters.
Security is critical, with some areas surrounded by concrete walls extending from the ceiling to the floor, even restricting access to Equinix staff.
By focusing on these details, Equinix enables customers to quickly set up and manage their environments through a self-service portal, delivering a cloud-like experience for on-premises solutions.
Equinix’s Commitment to the Environment
ACHIM GRANZEN. Compute-intensive AI applications challenge data centres’ “100% green energy” pledges, prompting providers to seek additional green measures. Equinix addresses this through sustainable design and green energy investments, including liquid cooling and improved traditional cooling. In Singapore, one of Equinix’s top 3 hubs, the company partnered with the government and Sembcorp to procure solar power from panels on public buildings. This improves Equinix’s power mix and supports Singapore’s renewable energy sector.
TIM SHEEDY Building and operating data centres sustainably is challenging. While the basics – real estate, cooling, and communications – remain, adding proximity to clients, affordability, and 100% renewable energy complicates matters. In Australia, reliant on a mixed-energy grid, Equinix has secured 151 MW of renewable energy from Victoria’s Golden Plains Wind Farm, aiming for 100% renewable by 2029.
Equinix leads with AIA-rated data centres that operate in warmer conditions, reducing cooling needs and boosting energy efficiency. Focusing on efficient buildings, sustainable water management, and a circular economy, Equinix aims for climate neutrality by 2030, demonstrating strong environmental responsibility.
Equinix’s Private AI Value Proposition
ACHIM GRANZEN. Most AI efforts, especially GenAI, have occurred in the public cloud, but there’s rising demand for Private AI due to concerns about data availability, privacy, governance, cost, and location. Technology providers in a position to offer alternative AI stacks (usually built on top of a GPU-as-a-service model) to the hyperscalers find themselves in high interest. Equinix, in partnership with providers such as Nvidia, offers Private AI solutions on a global turnkey AI infrastructure. These solutions are ideal for industries with large-scale operations and connectivity challenges, such as Manufacturing, or those slow to adopt public cloud.
SASH MUKHERJEE. Equinix’s Private AI value proposition will appeal to many organisations, especially as discussions on AI cost efficiency and ROI evolve. AI unites IT and business teams, and Equinix understands the need for conversations at multiple levels. Infrastructure leaders focus on data strategy capacity planning; CISOs on networking and security; business lines on application performance, and the C-suite on revenue, risk, and cost considerations. Each has a stake in the AI strategy. For success, Equinix must reshape its go-to-market message to be industry-specific (that’s how AI conversations are shaping) and reskill its salesforce for broader conversations beyond infrastructure.
Equinix’s Growth Potential
ACHIM GRANZEN. In Southeast Asia, Malaysia and Indonesia provide growth opportunities for Equinix. Indonesia holds massive potential as a digital-savvy G20 country. In Malaysia, the company’s data centres can play a vital part in the ongoing Mydigital initiative, having a presence in the country before the hyperscalers. Also, the proximity of the Johor Bahru data centre to Singapore opens additional business opportunities.
TIM SHEEDY. Equinix is evolving beyond being just a data centre real estate provider. By developing their own platforms and services, along with partner-provided solutions, they enable customers to optimise application placement, manage smaller points of presence, enhance cloud interconnectivity, move data closer to hyperscalers for backup and performance, and provide multi-cloud networking. Composable services – such as cloud routers, load balancers, internet access, bare metal, virtual machines, and virtual routing and forwarding – allow seamless integration with partner solutions.
Equinix’s focus over the last 12 months on automating and simplifying the data centre management and interconnection services is certainly paying dividends, and revenue is expected to grow above tech market growth rates.
Banks, insurers, and other financial services organisations in Asia Pacific have plenty of tech challenges and opportunities including cybersecurity and data privacy management; adapting to tech and customer demands, AI and ML integration; use of big data for personalisation; and regulatory compliance across business functions and transformation journeys.
Modernisation Projects are Back on the Table
An emerging tech challenge lies in modernising, replacing, or retiring legacy platforms and systems. Many banks still rely on outdated core systems, hindering agility, innovation, and personalised customer experiences. Migrating to modern, cloud-based systems presents challenges due to complexity, cost, and potential disruptions. Insurers are evaluating key platforms amid evolving customer needs and business models; ERP and HCM systems are up for renewal; data warehouses are transforming for the AI era; even CRM and other CX platforms are being modernised as older customer data stores and models become obsolete.
For the past five years, many financial services organisations in the region have sidelined large legacy modernisation projects, opting instead to make incremental transformations around their core systems. However, it is becoming critical for them to take action to secure their long-term survival and success.
Benefits of legacy modernisation include:
- Improved operational efficiency and agility
- Enhanced customer experience and satisfaction
- Increased innovation and competitive advantage
- Reduced security risks and compliance costs
- Preparation for future technologies
However, legacy modernisation and migration initiatives carry significant risks. For instance, TSB faced a USD 62M fine due to a failed mainframe migration, resulting in severe disruptions to branch operations and core banking functions like telephone, online, and mobile banking. The migration failure led to 225,492 complaints between 2018 and 2019, affecting all 550 branches and required TSB to pay more than USD 25M to customers through a redress program.
Modernisation Options
- Rip and Replace. Replacing the entire legacy system with a modern, cloud-based solution. While offering a clean slate and faster time to value, it’s expensive, disruptive, and carries migration risks.
- Refactoring. Rewriting key components of the legacy system with modern languages and architectures. It’s less disruptive than rip-and-replace but requires skilled developers and can still be time-consuming.
- Encapsulation. Wrapping the legacy system with a modern API layer, allowing integration with newer applications and tools. It’s quicker and cheaper than other options but doesn’t fully address underlying limitations.
- Microservices-based Modernisation. Breaking down the legacy system into smaller, independent services that can be individually modernised over time. It offers flexibility and agility but requires careful planning and execution.
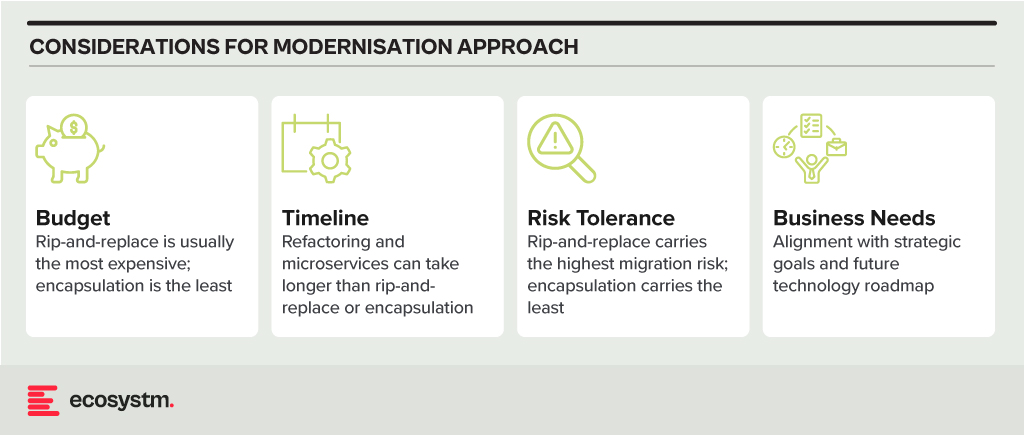
Financial Systems on the Block for Legacy Modernisation
Data Analytics Platforms. Harnessing customer data for insights and targeted offerings is vital. Legacy data warehouses often struggle with real-time data processing and advanced analytics.
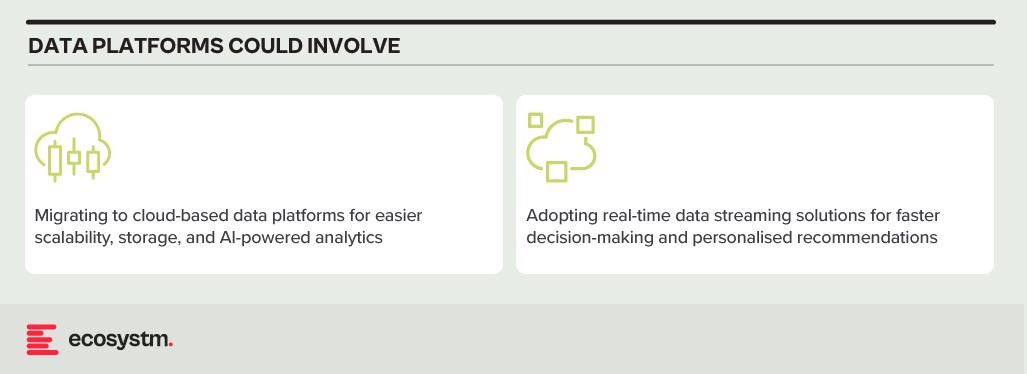
CRM Systems. Effective customer interactions require integrated CRM platforms. Outdated systems might hinder communication, personalisation, and cross-selling opportunities.
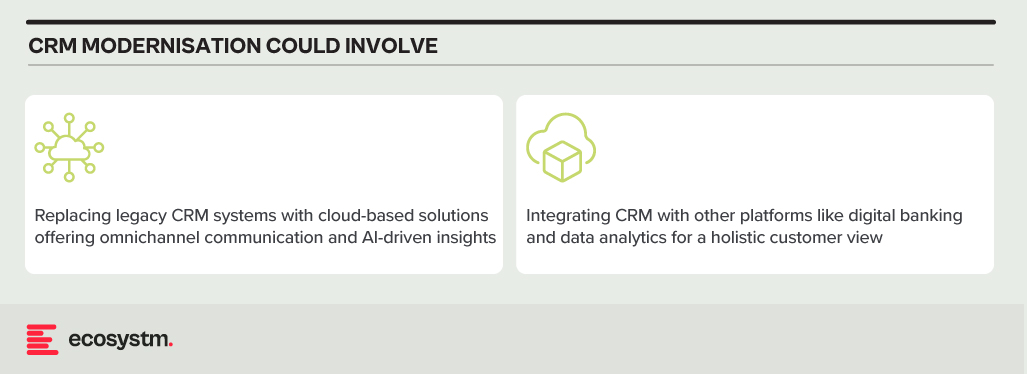
Payment Processing Systems. Legacy systems might lack support for real-time secure transactions, mobile payments, and cross-border transactions.
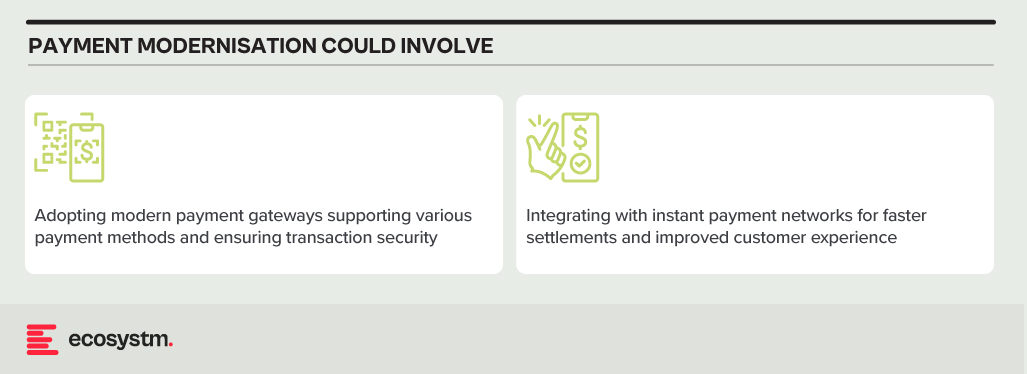
Core Banking Systems (CBS). The central nervous system of any bank, handling account management, transactions, and loan processing. Many Asia Pacific banks rely on aging, monolithic CBS with limited digital capabilities.
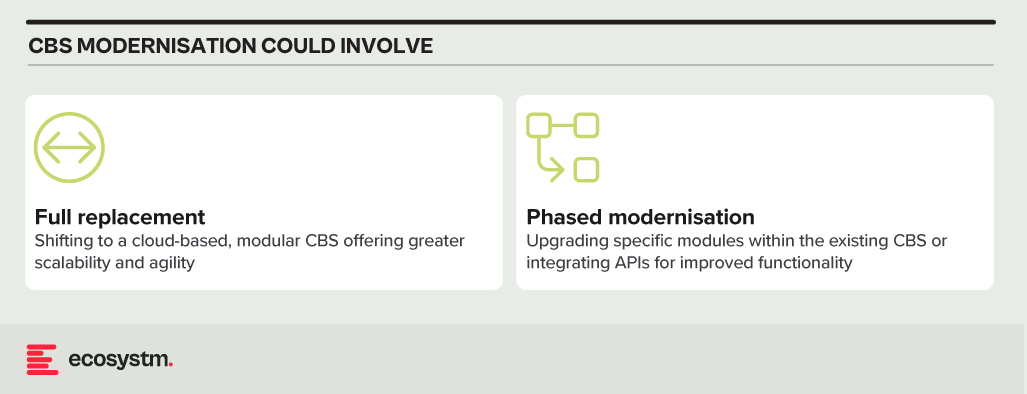
Digital Banking Platforms. While several Asia Pacific banks provide basic online banking, genuine digital transformation requires mobile-first apps with features such as instant payments, personalised financial management tools, and seamless third-party service integration.
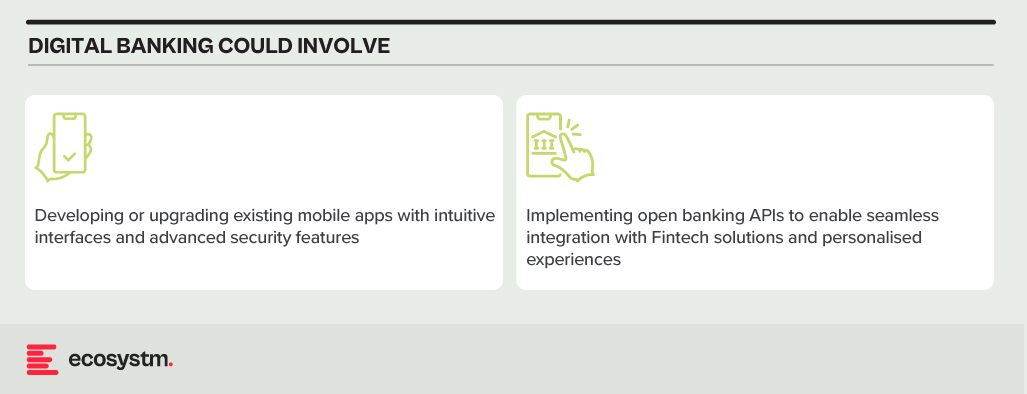
Modernising Technical Approaches and Architectures
Numerous technical factors need to be addressed during modernisation, with decisions needing to be made upfront. Questions around data migration, testing and QA, change management, data security and development methodology (agile, waterfall or hybrid) need consideration.
Best practices in legacy migration have taught some lessons.
Adopt a data fabric platform. Many organisations find that centralising all data into a single warehouse or platform rarely justifies the time and effort invested. Businesses continually generate new data, adding sources, and updating systems. Managing data where it resides might seem complex initially. However, in the mid to longer term, this approach offers clearer benefits as it reduces the likelihood of data discrepancies, obsolescence, and governance challenges.
Focus modernisation on the customer metrics and journeys that matter. Legacy modernisation need not be an all-or-nothing initiative. While systems like mainframes may require complete replacement, even some mainframe-based software can be partially modernised to enable services for external applications and processes. Assess the potential of modernising components of existing systems rather than opting for a complete overhaul of legacy applications.
Embrace the cloud and SaaS. With the growing network of hyperscaler cloud locations and data centres, there’s likely to be a solution that enables organisations to operate in the cloud while meeting data residency requirements. Even if not available now, it could align with the timeline of a multi-year legacy modernisation project. Whenever feasible, prioritise SaaS over cloud-hosted applications to streamline management, reduce overhead, and mitigate risk.
Build for customisation for local and regional needs. Many legacy applications are highly customised, leading to inflexibility, high management costs, and complexity in integration. Today, software providers advocate minimising configuration and customisation, opting for “out-of-the-box” solutions with room for localisation. The operations in different countries may require reconfiguration due to varying regulations and competitive pressures. Architecting applications to isolate these configurations simplifies system management, facilitating continuous improvement as new services are introduced by platform providers or ISV partners.
Explore the opportunity for emerging technologies. Emerging technologies, notably AI, can significantly enhance the speed and value of new systems. In the near future, AI will automate much of the work in data migration and systems integration, reducing the need for human involvement. When humans are required, low-code or no-code tools can expedite development. Private 5G services may eliminate the need for new network builds in branches or offices. AIOps and Observability can improve system uptime at lower costs. Considering these capabilities in platform decisions and understanding the ecosystem of partners and providers can accelerate modernisation journeys and deliver value faster.
Don’t Let Analysis Paralysis Slow Down Your Journey!
Yes, there are a lot of decisions that need to be made; and yes, there is much at stake if things go wrong! However, there’s a greater risk in not taking action. Maintaining a laser-focus on the customer and business outcomes that need to be achieved will help align many decisions. Keeping the customer experience as the guiding light ensures organisations are always moving in the right direction.
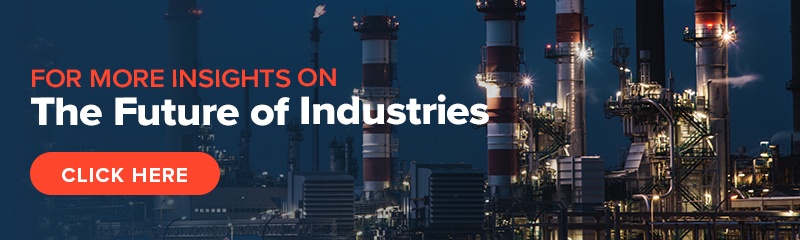
Over the past year, many organisations have explored Generative AI and LLMs, with some successfully identifying, piloting, and integrating suitable use cases. As business leaders push tech teams to implement additional use cases, the repercussions on their roles will become more pronounced. Embracing GenAI will require a mindset reorientation, and tech leaders will see substantial impact across various ‘traditional’ domains.
AIOps and GenAI Synergy: Shaping the Future of IT Operations
When discussing AIOps adoption, there are commonly two responses: “Show me what you’ve got” or “We already have a team of Data Scientists building models”. The former usually demonstrates executive sponsorship without a specific business case, resulting in a lukewarm response to many pre-built AIOps solutions due to their lack of a defined business problem. On the other hand, organisations with dedicated Data Scientist teams face a different challenge. While these teams can create impressive models, they often face pushback from the business as the solutions may not often address operational or business needs. The challenge arises from Data Scientists’ limited understanding of the data, hindering the development of use cases that effectively align with business needs.
The most effective approach lies in adopting an AIOps Framework. Incorporating GenAI into AIOps frameworks can enhance their effectiveness, enabling improved automation, intelligent decision-making, and streamlined operational processes within IT operations.
This allows active business involvement in defining and validating use-cases, while enabling Data Scientists to focus on model building. It bridges the gap between technical expertise and business requirements, ensuring AIOps initiatives are influenced by the capabilities of GenAI, address specific operational challenges and resonate with the organisation’s goals.
The Next Frontier of IT Infrastructure
Many companies adopting GenAI are openly evaluating public cloud-based solutions like ChatGPT or Microsoft Copilot against on-premises alternatives, grappling with the trade-offs between scalability and convenience versus control and data security.
Cloud-based GenAI offers easy access to computing resources without substantial upfront investments. However, companies face challenges in relinquishing control over training data, potentially leading to inaccurate results or “AI hallucinations,” and concerns about exposing confidential data. On-premises GenAI solutions provide greater control, customisation, and enhanced data security, ensuring data privacy, but require significant hardware investments due to unexpectedly high GPU demands during both the training and inferencing stages of AI models.
Hardware companies are focusing on innovating and enhancing their offerings to meet the increasing demands of GenAI. The evolution and availability of powerful and scalable GPU-centric hardware solutions are essential for organisations to effectively adopt on-premises deployments, enabling them to access the necessary computational resources to fully unleash the potential of GenAI. Collaboration between hardware development and AI innovation is crucial for maximising the benefits of GenAI and ensuring that the hardware infrastructure can adequately support the computational demands required for widespread adoption across diverse industries. Innovations in hardware architecture, such as neuromorphic computing and quantum computing, hold promise in addressing the complex computing requirements of advanced AI models.
The synchronisation between hardware innovation and GenAI demands will require technology leaders to re-skill themselves on what they have done for years – infrastructure management.
The Rise of Event-Driven Designs in IT Architecture
IT leaders traditionally relied on three-tier architectures – presentation for user interface, application for logic and processing, and data for storage. Despite their structured approach, these architectures often lacked scalability and real-time responsiveness. The advent of microservices, containerisation, and serverless computing facilitated event-driven designs, enabling dynamic responses to real-time events, and enhancing agility and scalability. Event-driven designs, are a paradigm shift away from traditional approaches, decoupling components and using events as a central communication mechanism. User actions, system notifications, or data updates trigger actions across distributed services, adding flexibility to the system.
However, adopting event-driven designs presents challenges, particularly in higher transaction-driven workloads where the speed of serverless function calls can significantly impact architectural design. While serverless computing offers scalability and flexibility, the latency introduced by initiating and executing serverless functions may pose challenges for systems that demand rapid, real-time responses. Increasing reliance on event-driven architectures underscores the need for advancements in hardware and compute power. Transitioning from legacy architectures can also be complex and may require a phased approach, with cultural shifts demanding adjustments and comprehensive training initiatives.
The shift to event-driven designs challenges IT Architects, whose traditional roles involved designing, planning, and overseeing complex systems. With Gen AI and automation enhancing design tasks, Architects will need to transition to more strategic and visionary roles. Gen AI showcases capabilities in pattern recognition, predictive analytics, and automated decision-making, promoting a symbiotic relationship with human expertise. This evolution doesn’t replace Architects but signifies a shift toward collaboration with AI-driven insights.
IT Architects need to evolve their skill set, blending technical expertise with strategic thinking and collaboration. This changing role will drive innovation, creating resilient, scalable, and responsive systems to meet the dynamic demands of the digital age.
Whether your organisation is evaluating or implementing GenAI, the need to upskill your tech team remains imperative. The evolution of AI technologies has disrupted the tech industry, impacting people in tech. Now is the opportune moment to acquire new skills and adapt tech roles to leverage the potential of GenAI rather than being disrupted by it.
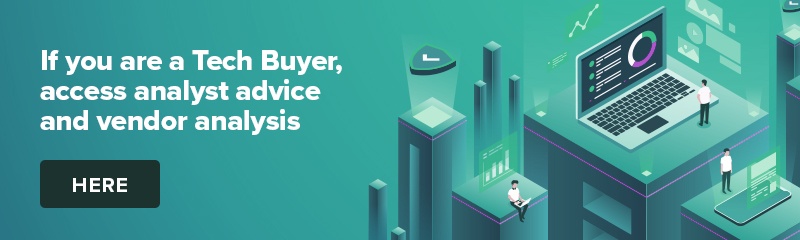
“AI Guardrails” are often used as a method to not only get AI programs on track, but also as a way to accelerate AI investments. Projects and programs that fall within the guardrails should be easy to approve, govern, and manage – whereas those outside of the guardrails require further review by a governance team or approval body. The concept of guardrails is familiar to many tech businesses and are often applied in areas such as cybersecurity, digital initiatives, data analytics, governance, and management.
While guidance on implementing guardrails is common, organisations often leave the task of defining their specifics, including their components and functionalities, to their AI and data teams. To assist with this, Ecosystm has surveyed some leading AI users among our customers to get their insights on the guardrails that can provide added value.
Data Security, Governance, and Bias
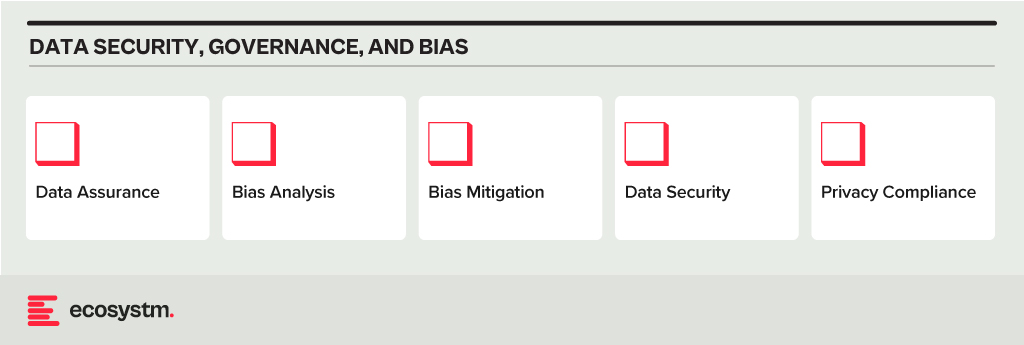
- Data Assurance. Has the organisation implemented robust data collection and processing procedures to ensure data accuracy, completeness, and relevance for the purpose of the AI model? This includes addressing issues like missing values, inconsistencies, and outliers.
- Bias Analysis. Does the organisation analyse training data for potential biases – demographic, cultural and so on – that could lead to unfair or discriminatory outputs?
- Bias Mitigation. Is the organisation implementing techniques like debiasing algorithms and diverse data augmentation to mitigate bias in model training?
- Data Security. Does the organisation use strong data security measures to protect sensitive information used in training and running AI models?
- Privacy Compliance. Is the AI opportunity compliant with relevant data privacy regulations (country and industry-specific as well as international standards) when collecting, storing, and utilising data?
Model Development and Explainability
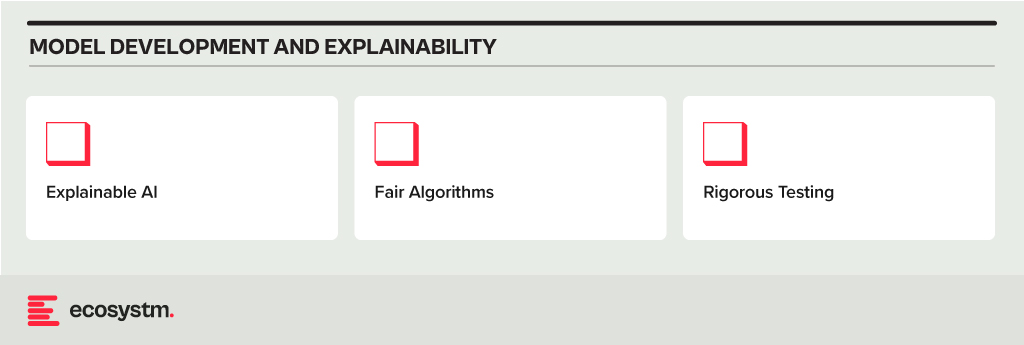
- Explainable AI. Does the model use explainable AI (XAI) techniques to understand and explain how AI models reach their decisions, fostering trust and transparency?
- Fair Algorithms. Are algorithms and models designed with fairness in mind, considering factors like equal opportunity and non-discrimination?
- Rigorous Testing. Does the organisation conduct thorough testing and validation of AI models before deployment, ensuring they perform as intended, are robust to unexpected inputs, and avoid generating harmful outputs?
AI Deployment and Monitoring
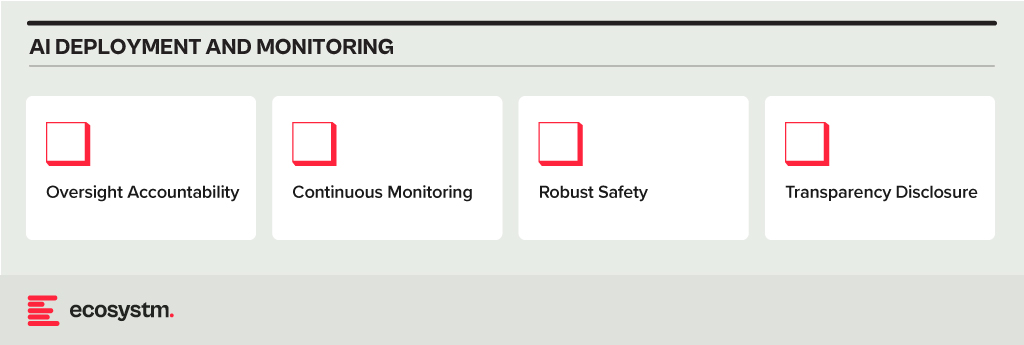
- Oversight Accountability. Has the organisation established clear roles and responsibilities for human oversight throughout the AI lifecycle, ensuring human control over critical decisions and mitigation of potential harm?
- Continuous Monitoring. Are there mechanisms to continuously monitor AI systems for performance, bias drift, and unintended consequences, addressing any issues promptly?
- Robust Safety. Can the organisation ensure AI systems are robust and safe, able to handle errors or unexpected situations without causing harm? This includes thorough testing and validation of AI models under diverse conditions before deployment.
- Transparency Disclosure. Is the organisation transparent with stakeholders about AI use, including its limitations, potential risks, and how decisions made by the system are reached?
Other AI Considerations
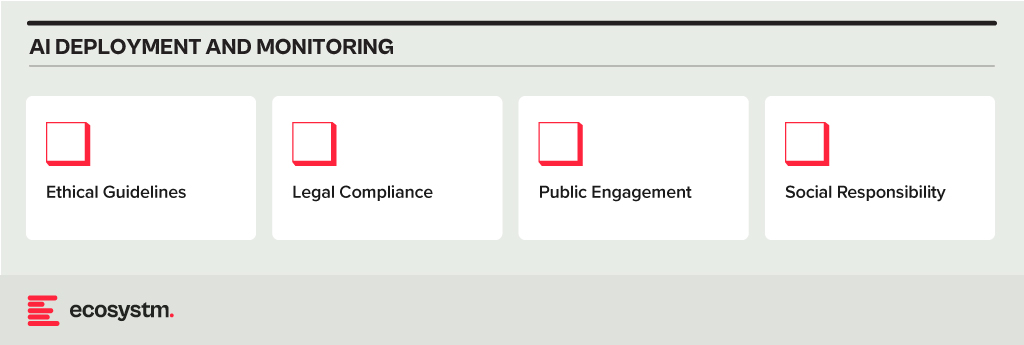
- Ethical Guidelines. Has the organisation developed and adhered to ethical principles for AI development and use, considering areas like privacy, fairness, accountability, and transparency?
- Legal Compliance. Has the organisation created mechanisms to stay updated on and compliant with relevant legal and regulatory frameworks governing AI development and deployment?
- Public Engagement. What mechanisms are there in place to encourage open discussion and engage with the public regarding the use of AI, addressing concerns and building trust?
- Social Responsibility. Has the organisation considered the environmental and social impact of AI systems, including energy consumption, ecological footprint, and potential societal consequences?
Implementing these guardrails requires a comprehensive approach that includes policy formulation, technical measures, and ongoing oversight. It might take a little longer to set up this capability, but in the mid to longer term, it will allow organisations to accelerate AI implementations and drive a culture of responsible AI use and deployment.
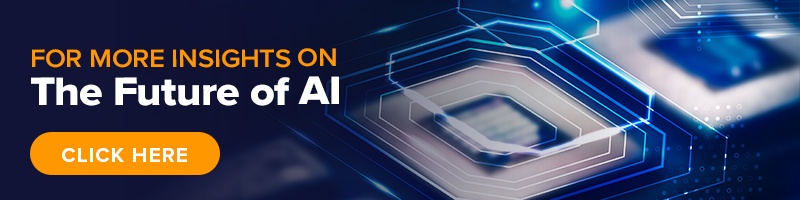
The challenge of AI is that it is hard to build a business case when the outcomes are inherently uncertain. Unlike a traditional process improvement procedure, there are few guarantees that AI will solve the problem it is meant to solve. Organisations that have been experimenting with AI for some time are aware of this, and have begun to formalise their Proof of Concept (PoC) process to make it easily repeatable by anyone in the organisation who has a use case for AI. PoCs can validate assumptions, demonstrate the feasibility of an idea, and rally stakeholders behind the project.
PoCs are particularly useful at a time when AI is experiencing both heightened visibility and increased scrutiny. Boards, senior management, risk, legal and cybersecurity professionals are all scrutinising AI initiatives more closely to ensure they do not put the organisation at risk of breaking laws and regulations or damaging customer or supplier relationships.
13 Steps to Building an AI PoC
Despite seeming to be lightweight and easy to implement, a good PoC is actually methodologically sound and consistent in its approach. To implement a PoC for AI initiatives, organisations need to:
- Clearly define the problem. Businesses need to understand and clearly articulate the problem they want AI to solve. Is it about improving customer service, automating manual processes, enhancing product recommendations, or predicting machinery failure?
- Set clear objectives. What will success look like for the PoC? Is it about demonstrating technical feasibility, showing business value, or both? Set tangible metrics to evaluate the success of the PoC.
- Limit the scope. PoCs should be time-bound and narrow in scope. Instead of trying to tackle a broad problem, focus on a specific use case or a subset of data.
- Choose the right data. AI is heavily dependent on data. For a PoC, select a representative dataset that’s large enough to provide meaningful results but manageable within the constraints of the PoC.
- Build a multidisciplinary team. Involve team members from IT, data science, business units, and other relevant stakeholders. Their combined perspectives will ensure both technical and business feasibility.
- Prioritise speed over perfection. Use available tools and platforms to expedite the development process. It’s more important to quickly test assumptions than to build a highly polished solution.
- Document assumptions and limitations. Clearly state any assumptions made during the PoC, as well as known limitations. This helps set expectations and can guide future work.
- Present results clearly. Once the PoC is complete, create a clear and concise report or presentation that showcases the results, methodologies, and potential implications for the business.
- Get feedback. Allow stakeholders to provide feedback on the PoC. This includes end-users, technical teams, and business leaders. Their insights will help refine the approach and guide future iterations.
- Plan for the next steps. What actions need to follow a successful PoC demonstration? This might involve a pilot project with a larger scope, integrating the AI solution into existing systems, or scaling the solution across the organisation.
- Assess costs and ROI. Evaluate the costs associated with scaling the solution and compare it with the anticipated ROI. This will be crucial for securing budget and support for further expansion.
- Continually learn and iterate. AI is an evolving field. Use the PoC as a learning experience and be prepared to continually iterate on your solutions as technologies and business needs evolve.
- Consider ethical and social implications. Ensure that the AI initiative respects privacy, reduces bias, and upholds the ethical standards of the organisation. This is critical for building trust and ensuring long-term success.
Customising AI for Your Business
The primary purpose of a PoC is to validate an idea quickly and with minimal risk. It should provide a clear path for decision-makers to either proceed with a more comprehensive implementation or to pivot and explore alternative solutions. It is important for the legal, risk and cybersecurity teams to be aware of the outcomes and support further implementation.
AI initiatives will inevitably drive significant productivity and customer experience improvements – but not every solution will be right for the business. At Ecosystm, we have come across organisations that have employed conversational AI in their contact centres to achieve entirely distinct results – so the AI experience of peers and competitors may not be relevant. A consistent PoC process that trains business and technology teams across the organisation and encourages experimentation at every possible opportunity, would be far more useful.
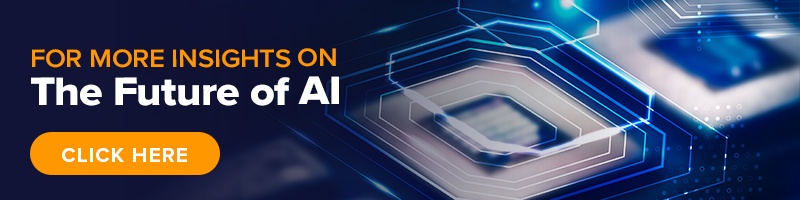
In my last Ecosystm Insight, I spoke about the 5 strategies that leading CX leaders follow to stay ahead of the curve. Data is at the core of these CX strategies. But a customer data breach can have an enormous financial and reputational impact on a brand.
Here are 12 essential steps to effective governance that will help you unlock the power of customer data.
- Understand data protection laws and regulations
- Create a data governance framework
- Establish data privacy and security policies
- Implement data minimisation
- Ensure data accuracy
- Obtain explicit consent
- Mask, anonymise and pseudonymise data
- Implement strong access controls
- Train employees
- Conduct risk assessments and audits
- Develop a data breach response plan
- Monitor and review
Read on to find out more.
Download ‘A 12-Step Plan for Governance of Customer Data’ as a PDF
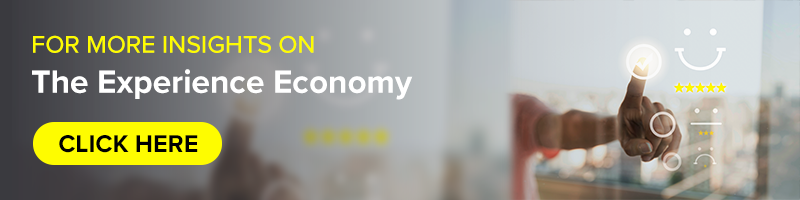
Ecosystm, supported by their partner Zurich Insurance, conducted an invitation-only Executive ThinkTank at the Point Zero Forum in Zurich, earlier this year. A select group of regulators, investors, and senior executives from financial institutions from across the globe came together to share their insights and experiences on the critical role data is playing in a digital economy, and the concrete actions that governments and businesses can take to allow a free flow of data that will help create a global data economy.
Here are the key takeaways from the ThinkTank.
- Bilateral Agreements for Transparency. Trade agreements play an important role in developing standards that ensure transparency across objective criteria. This builds the foundation for cross-border privacy and data protection measures, in alignment with local legislations.
- Building Trust is Crucial. Privacy and private data are defined differently across countries. One of the first steps is to establish common standards for opening up the APIs. This starts with building trust in common data platforms and establishing some standards and interoperability arrangements.
- Consumers Can Influence Cross-Border Data Exchange. Organisations should continue to actively lobby to change regulator perspectives on data exchange. But, the real impact will be created when consumers come into the conversation – as they are the ones who will miss out on access to global and uniform services due to restrictions in cross-country data sharing.
Read below to find out more.
Click here to download “Opportunities Created by Cross Border Data Flows” as a PDF
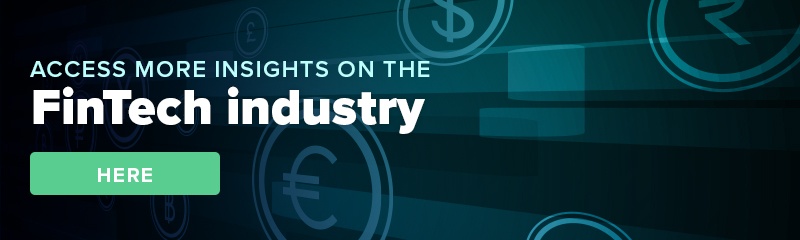
When non-organic (man-made) fabric was introduced into fashion, there were a number of harsh warnings about using polyester and man-made synthetic fibres, including their flammability.
In creating non-organic data sets, should we also be creating warnings on their use and flammability? Let’s look at why synthetic data is used in industries such as Financial Services, Automotive as well as for new product development in Manufacturing.
Synthetic Data Defined
Synthetic data can be defined as data that is artificially developed rather than being generated by actual interactions. It is often created with the help of algorithms and is used for a wide range of activities, including as test data for new products and tools, for model validation, and in AI model training. Synthetic data is a type of data augmentation which involves creating new and representative data.
Why is it used?
The main reasons why synthetic data is used instead of real data are cost, privacy, and testing. Let’s look at more specifics on this:
- Data privacy. When privacy requirements limit data availability or how it can be used. For example, in Financial Services where restrictions around data usage and customer privacy are particularly limiting, companies are starting to use synthetic data to help them identify and eliminate bias in how they treat customers – without contravening data privacy regulations.
- Data availability. When the data needed for testing a product does not exist or is not available to the testers. This is often the case for new releases.
- Data for testing. When training data is needed for machine learning algorithms. However, in many instances, such as in the case of autonomous vehicles, the data is expensive to generate in real life.
- Training across third parties using cloud. When moving private data to cloud infrastructures involves security and compliance risks. Moving synthetic versions of sensitive data to the cloud can enable organisations to share data sets with third parties for training across cloud infrastructures.
- Data cost. Producing synthetic data through a generative model is significantly more cost-effective and efficient than collecting real-world data. With synthetic data, it becomes cheaper and faster to produce new data once the generative model is set up.
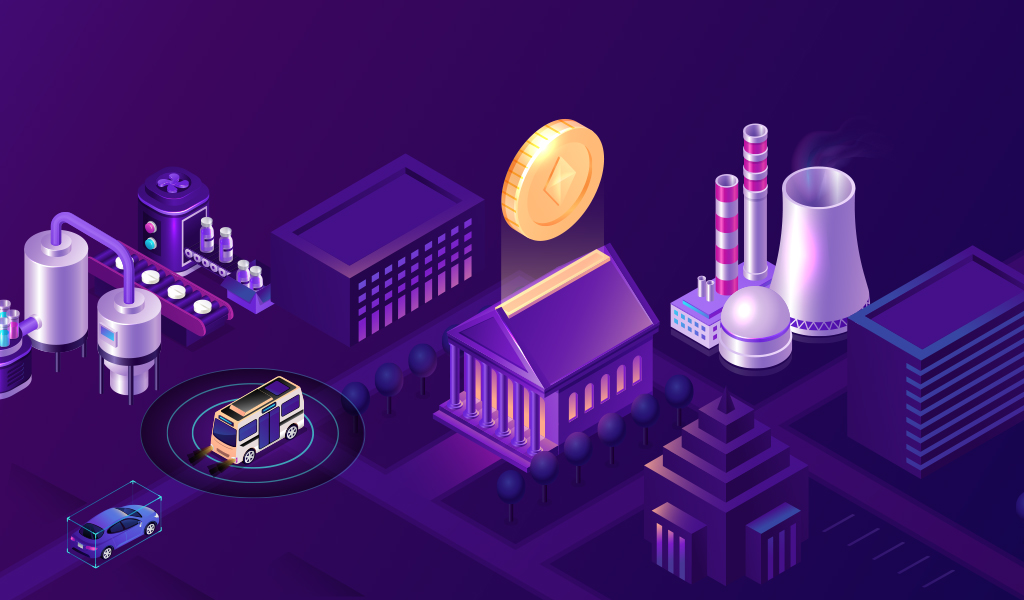
Why should it cause concern?
If real dataset contains biases, data augmented from it will contain biases, too. So, identification of optimal data augmentation strategy is important.
If the synthetic set doesn’t truly represent the original customer data set, it might contain the wrong buying signals regarding what customers are interested in or are inclined to buy.
Synthetic data also requires some form of output/quality control and internal regulation, specifically in highly regulated industries such as the Financial Services.
Creating incorrect synthetic data also can get a company in hot water with external regulators. For example, if a company created a product that harmed someone or didn’t work as advertised, it could lead to substantial financial penalties and, possibly, closer scrutiny in the future.
Conclusion
Synthetic data allows us to continue developing new and innovative products and solutions when the data necessary to do so wouldn’t otherwise be present or available due to volume, data sensitivity or user privacy challenges. Generating synthetic data comes with the flexibility to adjust its nature and environment as and when required in order to improve the performance of the model to create opportunities to check for outliers and extreme conditions.