At a recently held Ecosystm roundtable, in partnership with Qlik and 121Connects, Ecosystm Principal Advisor Manoj Chugh, moderated a conversation where Indian tech and data leaders discussed building trust in data strategies. They explored ways to automate data pipelines and improve governance to drive better decisions and business outcomes. Here are the key takeaways from the session.

Data isn’t just a byproduct anymore; it’s the lifeblood of modern businesses, fuelling informed decisions and strategic growth. But with vast amounts of data, the challenge isn’t just managing it; it’s building trust. AI, once a beacon of hope, is now at risk without a reliable data foundation. Ecosystm research reveals that a staggering 66% of Indian tech leaders doubt their organisation’s data quality, and the problem of data silos is exacerbating this trust crisis.
At the Leaders Roundtable in Mumbai, I had the opportunity to moderate a discussion among data and digital leaders on the critical components of building trust in data and leveraging it to drive business value. The consensus was that building trust requires a comprehensive strategy that addresses the complexities of data management and positions the organisation for future success. Here are the key strategies that are essential for achieving these goals.
1. Adopting a Unified Data Approach
Organisations are facing a growing wave of complex workloads and business initiatives. To manage this expansion, IT teams are turning to multi-cloud, SaaS, and hybrid environments. However, this diverse landscape introduces new challenges, such as data silos, security vulnerabilities, and difficulties in ensuring interoperability between systems.

A unified data strategy is crucial to overcome these challenges. By ensuring platform consistency, robust security, and seamless data integration, organisations can simplify data management, enhance security, and align with business goals – driving informed decisions, innovation, and long-term success.
Real-time data integration is essential for timely data availability, enabling organisations to make data-driven decisions quickly and effectively. By integrating data from various sources in real-time, businesses can gain valuable insights into their operations, identify trends, and respond to changing market conditions.
Organisations that are able to integrate their IT and operational technology (OT) systems find their data accuracy increasing. By combining IT’s digital data management expertise with OT’s real-time operational insights, organisations can ensure more accurate, timely, and actionable data. This integration enables continuous monitoring and analysis of operational data, leading to faster identification of errors, more precise decision-making, and optimised processes.
2. Enhancing Data Quality with Automation and Collaboration
As the volume and complexity of data continue to grow, ensuring high data quality is essential for organisations to make accurate decisions and to drive trust in data-driven solutions. Automated data quality tools are useful for cleansing and standardising data to eliminate errors and inconsistencies.
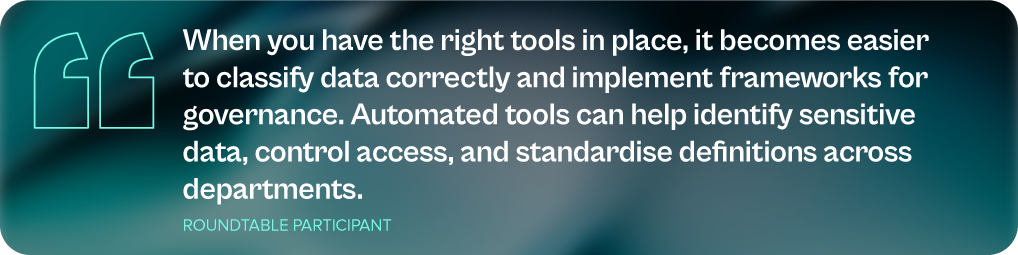
As mentioned earlier, integrating IT and OT systems can help organisations improve operational efficiency and resilience. By leveraging data-driven insights, businesses can identify bottlenecks, optimise workflows, and proactively address potential issues before they escalate. This can lead to cost savings, increased productivity, and improved customer satisfaction.
However, while automation technologies can help, organisations must also invest in training employees in data management, data visualisation, and data governance.
3. Modernising Data Infrastructure for Agility and Innovation
In today’s fast-paced business landscape, agility is paramount. Modernising data infrastructure is essential to remain competitive – the right digital infrastructure focuses on optimising costs, boosting capacity and agility, and maximising data leverage, all while safeguarding the organisation from cyber threats. This involves migrating data lakes and warehouses to cloud platforms and adopting advanced analytics tools. However, modernisation efforts must be aligned with specific business goals, such as enhancing customer experiences, optimising operations, or driving innovation. A well-modernised data environment not only improves agility but also lays the foundation for future innovations.

Technology leaders must assess whether their data architecture supports the organisation’s evolving data requirements, considering factors such as data flows, necessary management systems, processing operations, and AI applications. The ideal data architecture should be tailored to the organisation’s specific needs, considering current and future data demands, available skills, costs, and scalability.
4. Strengthening Data Governance with a Structured Approach
Data governance is crucial for establishing trust in data, and providing a framework to manage its quality, integrity, and security throughout its lifecycle. By setting clear policies and processes, organisations can build confidence in their data, support informed decision-making, and foster stakeholder trust.
A key component of data governance is data lineage – the ability to trace the history and transformation of data from its source to its final use. Understanding this journey helps organisations verify data accuracy and integrity, ensure compliance with regulatory requirements and internal policies, improve data quality by proactively addressing issues, and enhance decision-making through context and transparency.
A tiered data governance structure, with strategic oversight at the executive level and operational tasks managed by dedicated data governance councils, ensures that data governance aligns with broader organisational goals and is implemented effectively.
Are You Ready for the Future of AI?
The ultimate goal of your data management and discovery mechanisms is to ensure that you are advancing at pace with the industry. The analytics landscape is undergoing a profound transformation, promising to revolutionise how organisations interact with data. A key innovation, the data fabric, is enabling organisations to analyse unstructured data, where the true value often lies, resulting in cleaner and more reliable data models.
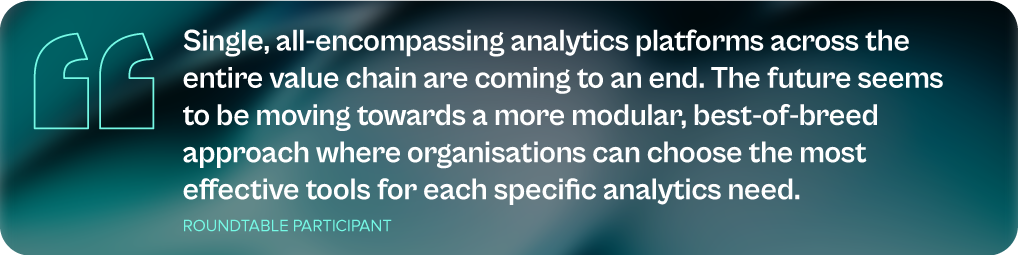
GenAI has emerged as another game-changer, empowering employees across the organisation to become citizen data scientists. This democratisation of data analytics allows for a broader range of insights and fosters a more data-driven culture. Organisations can leverage GenAI to automate tasks, generate new ideas, and uncover hidden patterns in their data.
The shift from traditional dashboards to real-time conversational tools is also reshaping how data insights are delivered and acted upon. These tools enable users to ask questions in natural language, receiving immediate and relevant answers based on the underlying data. This conversational approach makes data more accessible and actionable, empowering employees to make data-driven decisions at all levels of the organisation.
To fully capitalise on these advancements, organisations need to reassess their AI/ML strategies. By ensuring that their tech initiatives align with their broader business objectives and deliver tangible returns on investment, organisations can unlock the full potential of data-driven insights and gain a competitive edge. It is equally important to build trust in AI initiatives, through a strong data foundation. This involves ensuring data quality, accuracy, and consistency, as well as implementing robust data governance practices. A solid data foundation provides the necessary groundwork for AI and GenAI models to deliver reliable and valuable insights.
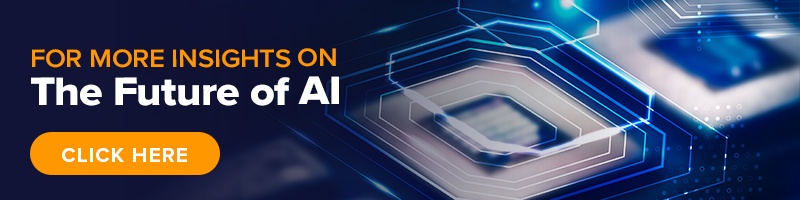
In my last Ecosystm Insight, I spoke about the importance of data architecture in defining the data flow, data management systems required, the data processing operations, and AI applications. Data Mesh and Data Fabric are both modern architectural approaches designed to address the complexities of managing and accessing data across a large organisation. While they share some commonalities, such as improving data accessibility and governance, they differ significantly in their methodologies and focal points.
Data Mesh
- Philosophy and Focus. Data Mesh is primarily focused on the organisational and architectural approach to decentralise data ownership and governance. It treats data as a product, emphasising the importance of domain-oriented decentralised data ownership and architecture. The core principles of Data Mesh include domain-oriented decentralised data ownership, data as a product, self-serve data infrastructure as a platform, and federated computational governance.
- Implementation. In a Data Mesh, data is managed and owned by domain-specific teams who are responsible for their data products from end to end. This includes ensuring data quality, accessibility, and security. The aim is to enable these teams to provide and consume data as products, improving agility and innovation.
- Use Cases. Data Mesh is particularly effective in large, complex organisations with many independent teams and departments. It’s beneficial when there’s a need for agility and rapid innovation within specific domains or when the centralisation of data management has become a bottleneck.
Data Fabric
- Philosophy and Focus. Data Fabric focuses on creating a unified, integrated layer of data and connectivity across an organisation. It leverages metadata, advanced analytics, and AI to improve data discovery, governance, and integration. Data Fabric aims to provide a comprehensive and coherent data environment that supports a wide range of data management tasks across various platforms and locations.
- Implementation. Data Fabric typically uses advanced tools to automate data discovery, governance, and integration tasks. It creates a seamless environment where data can be easily accessed and shared, regardless of where it resides or what format it is in. This approach relies heavily on metadata to enable intelligent and automated data management practices.
- Use Cases. Data Fabric is ideal for organisations that need to manage large volumes of data across multiple systems and platforms. It is particularly useful for enhancing data accessibility, reducing integration complexity, and supporting data governance at scale. Data Fabric can benefit environments where there’s a need for real-time data access and analysis across diverse data sources.
Both approaches aim to overcome the challenges of data silos and improve data accessibility, but they do so through different methodologies and with different priorities.
Data Mesh and Data Fabric Vendors
The concepts of Data Mesh and Data Fabric are supported by various vendors, each offering tools and platforms designed to facilitate the implementation of these architectures. Here’s an overview of some key players in both spaces:
Data Mesh Vendors
Data Mesh is more of a conceptual approach than a product-specific solution, focusing on organisational structure and data decentralisation. However, several vendors offer tools and platforms that support the principles of Data Mesh, such as domain-driven design, product thinking for data, and self-serve data infrastructure:
- Thoughtworks. As the originator of the Data Mesh concept, Thoughtworks provides consultancy and implementation services to help organisations adopt Data Mesh principles.
- Starburst. Starburst offers a distributed SQL query engine (Starburst Galaxy) that allows querying data across various sources, aligning with the Data Mesh principle of domain-oriented, decentralised data ownership.
- Databricks. Databricks provides a unified analytics platform that supports collaborative data science and analytics, which can be leveraged to build domain-oriented data products in a Data Mesh architecture.
- Snowflake. With its Data Cloud, Snowflake facilitates data sharing and collaboration across organisational boundaries, supporting the Data Mesh approach to data product thinking.
- Collibra. Collibra provides a data intelligence cloud that offers data governance, cataloguing, and privacy management tools essential for the Data Mesh approach. By enabling better data discovery, quality, and policy management, Collibra supports the governance aspect of Data Mesh.
Data Fabric Vendors
Data Fabric solutions often come as more integrated products or platforms, focusing on data integration, management, and governance across a diverse set of systems and environments:
- Informatica. The Informatica Intelligent Data Management Cloud includes features for data integration, quality, governance, and metadata management that are core to a Data Fabric strategy.
- Talend. Talend provides data integration and integrity solutions with strong capabilities in real-time data collection and governance, supporting the automated and comprehensive approach of Data Fabric.
- IBM. IBM’s watsonx.data is a fully integrated data and AI platform that automates the lifecycle of data across multiple clouds and systems, embodying the Data Fabric approach to making data easily accessible and governed.
- TIBCO. TIBCO offers a range of products, including TIBCO Data Virtualization and TIBCO EBX, that support the creation of a Data Fabric by enabling comprehensive data management, integration, and governance.
- NetApp. NetApp has a suite of cloud data services that provide a simple and consistent way to integrate and deliver data across cloud and on-premises environments. NetApp’s Data Fabric is designed to enhance data control, protection, and freedom.
The choice of vendor or tool for either Data Mesh or Data Fabric should be guided by the specific needs, existing technology stack, and strategic goals of the organisation. Many vendors provide a range of capabilities that can support different aspects of both architectures, and the best solution often involves a combination of tools and platforms. Additionally, the technology landscape is rapidly evolving, so it’s wise to stay updated on the latest offerings and how they align with the organisation’s data strategy.
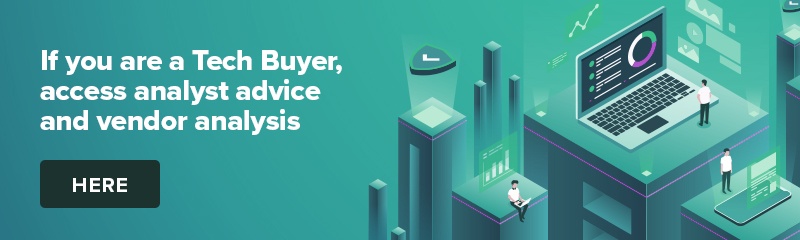
The data architecture outlines how data is managed in an organisation and is crucial for defining the data flow, data management systems required, the data processing operations, and AI applications. Data architects and engineers define data models and structures based on these requirements, supporting initiatives like data science. Before we delve into the right data architecture for your AI journey, let’s talk about the data management options. Technology leaders have the challenge of deciding on a data management system that takes into consideration factors such as current and future data needs, available skills, costs, and scalability. As data strategies become vital to business success, selecting the right data management system is crucial for enabling data-driven decisions and innovation.
Data Warehouse
A Data Warehouse is a centralised repository that stores vast amounts of data from diverse sources within an organisation. Its main function is to support reporting and data analysis, aiding businesses in making informed decisions. This concept encompasses both data storage and the consolidation and management of data from various sources to offer valuable business insights. Data Warehousing evolves alongside technological advancements, with trends like cloud-based solutions, real-time capabilities, and the integration of AI and machine learning for predictive analytics shaping its future.
Core Characteristics
- Integrated. It integrates data from multiple sources, ensuring consistent definitions and formats. This often includes data cleansing and transformation for analysis suitability.
- Subject-Oriented. Unlike operational databases, which prioritise transaction processing, it is structured around key business subjects like customers, products, and sales. This organisation facilitates complex queries and analysis.
- Non-Volatile. Data in a Data Warehouse is stable; once entered, it is not deleted. Historical data is retained for analysis, allowing for trend identification over time.
- Time-Variant. It retains historical data for trend analysis across various time periods. Each entry is time-stamped, enabling change tracking and trend analysis.
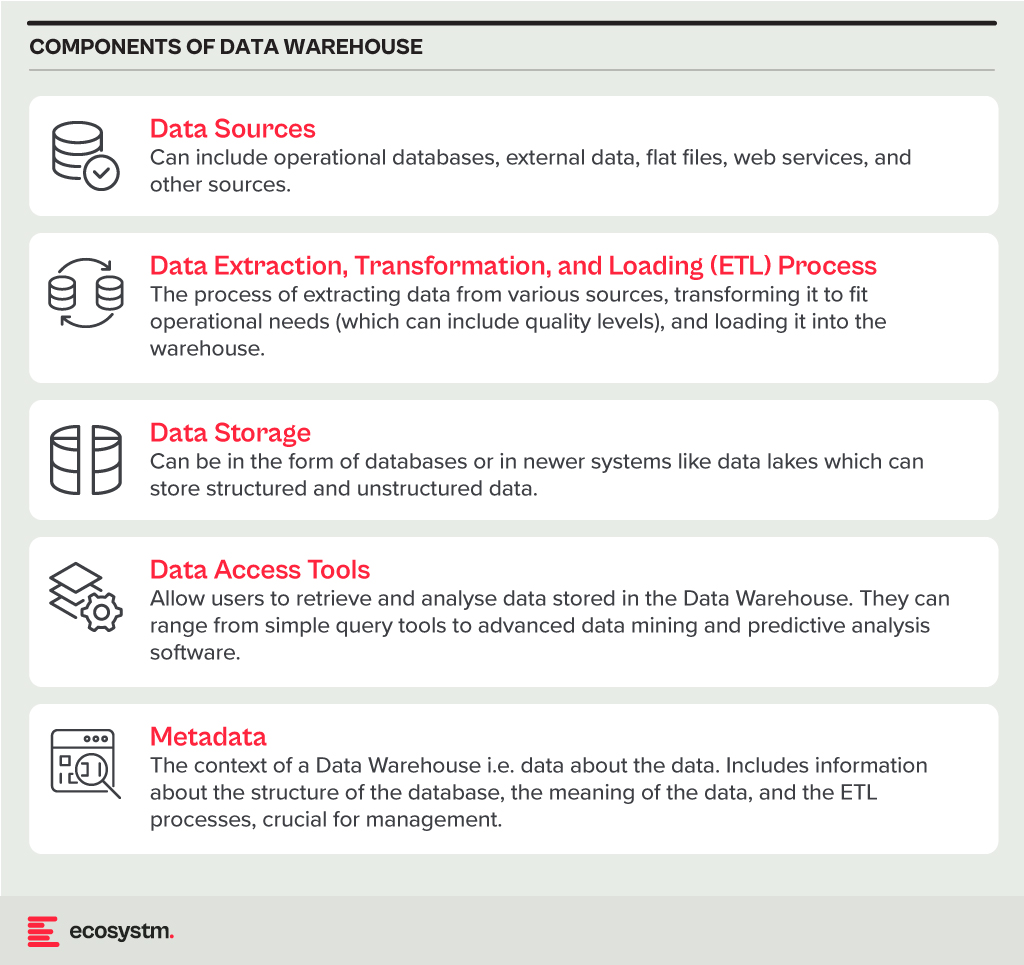
Benefits
- Better Decision Making. Data Warehouses consolidate data from multiple sources, offering a comprehensive business view for improved decision-making.
- Enhanced Data Quality. The ETL process ensures clean and consistent data entry, crucial for accurate analysis.
- Historical Analysis. Storing historical data enables trend analysis over time, informing future strategies.
- Improved Efficiency. Data Warehouses enable swift access and analysis of relevant data, enhancing efficiency and productivity.
Challenges
- Complexity. Designing and implementing a Data Warehouse can be complex and time-consuming.
- Cost. The cost of hardware, software, and specialised personnel can be significant.
- Data Security. Storing large amounts of sensitive data in one place poses security risks, requiring robust security measures.
Data Lake
A Data Lake is a centralised repository for storing, processing, and securing large volumes of structured and unstructured data. Unlike traditional Data Warehouses, which are structured and optimised for analytics with predefined schemas, Data Lakes retain raw data in its native format. This flexibility in data usage and analysis makes them crucial in modern data architecture, particularly in the age of big data and cloud.
Core Characteristics
- Schema-on-Read Approach. This means the data structure is not defined until the data is read for analysis. This offers more flexible data storage compared to the schema-on-write approach of Data Warehouses.
- Support for Multiple Data Types. Data Lakes accommodate diverse data types, including structured (like databases), semi-structured (like JSON, XML files), unstructured (like text and multimedia files), and binary data.
- Scalability. Designed to handle vast amounts of data, Data Lakes can easily scale up or down based on storage needs and computational demands, making them ideal for big data applications.
- Versatility. Data Lakes support various data operations, including batch processing, real-time analytics, machine learning, and data visualisation, providing a versatile platform for data science and analytics.
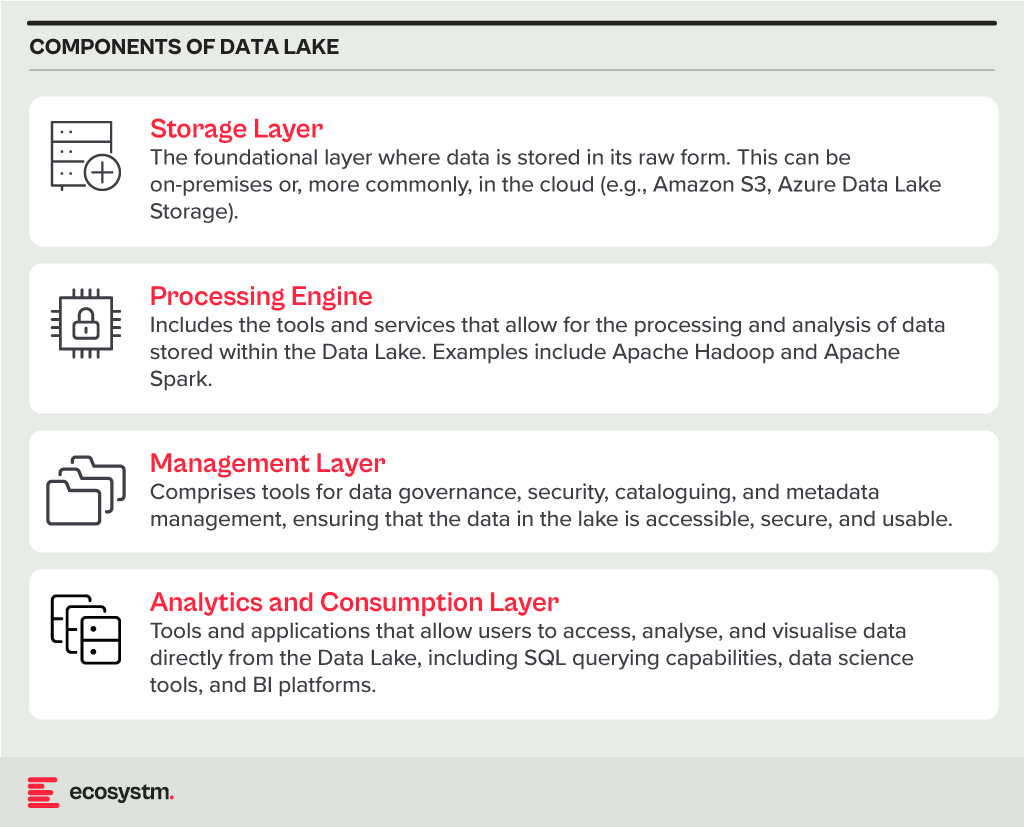
Benefits
- Flexibility. Data Lakes offer diverse storage formats and a schema-on-read approach for flexible analysis.
- Cost-Effectiveness. Cloud-hosted Data Lakes are cost-effective with scalable storage solutions.
- Advanced Analytics Capabilities. The raw, granular data in Data Lakes is ideal for advanced analytics, machine learning, and AI applications, providing deeper insights than traditional data warehouses.
Challenges
- Complexity and Management. Without proper management, a Data Lake can quickly become a “Data Swamp” where data is disorganised and unusable.
- Data Quality and Governance. Ensuring the quality and governance of data within a Data Lake can be challenging, requiring robust processes and tools.
- Security. Protecting sensitive data within a Data Lake is crucial, requiring comprehensive security measures.
Data Lakehouse
A Data Lakehouse is an innovative data management system that merges the strengths of Data Lakes and Data Warehouses. This hybrid approach strives to offer the adaptability and expansiveness of a Data Lake for housing extensive volumes of raw, unstructured data, while also providing the structured, refined data functionalities typical of a Data Warehouse. By bridging the gap between these two traditional data storage paradigms, Lakehouses enable more efficient data analytics, machine learning, and business intelligence operations across diverse data types and use cases.
Core Characteristics
- Unified Data Management. A Lakehouse streamlines data governance and security by managing both structured and unstructured data on one platform, reducing organizational data silos.
- Schema Flexibility. It supports schema-on-read and schema-on-write, allowing data to be stored and analysed flexibly. Data can be ingested in raw form and structured later or structured at ingestion.
- Scalability and Performance. Lakehouses scale storage and compute resources independently, handling large data volumes and complex analytics without performance compromise.
- Advanced Analytics and Machine Learning Integration. By providing direct access to both raw and processed data on a unified platform, Lakehouses facilitate advanced analytics, real-time analytics, and machine learning.
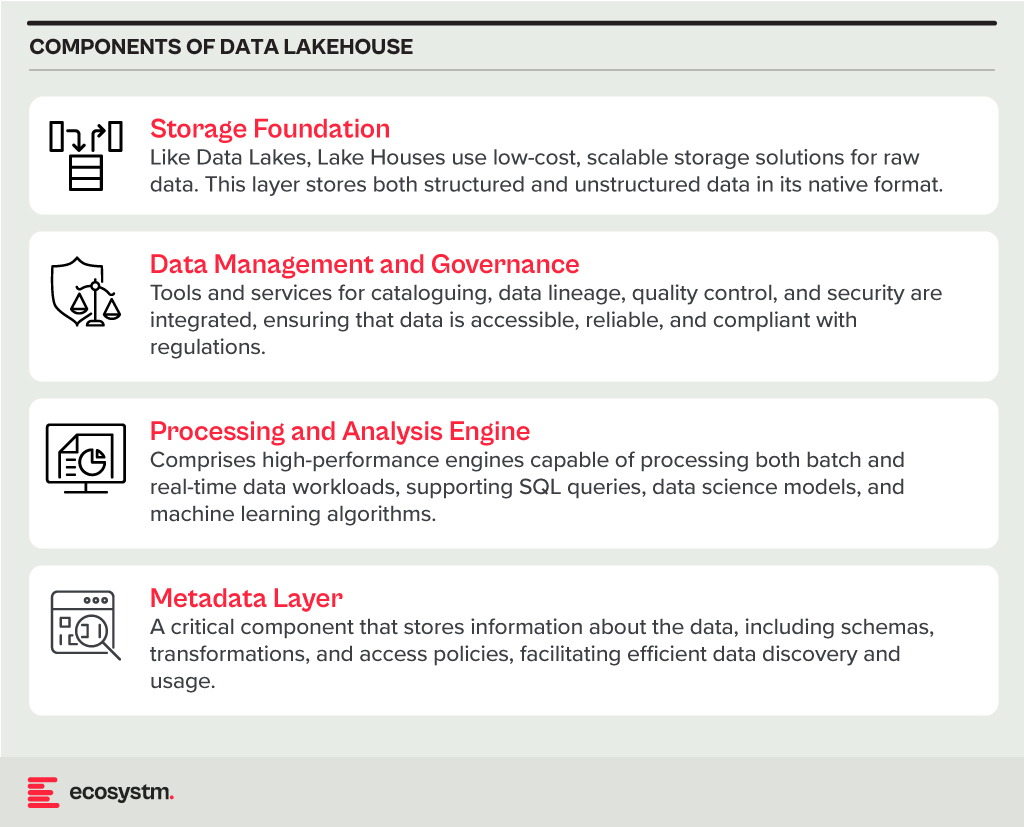
Benefits
- Versatility in Data Analysis. Lakehouses support diverse data analytics, spanning from traditional BI to advanced machine learning, all within one platform.
- Cost-Effective Scalability. The ability to scale storage and compute independently, often in a cloud environment, makes Lakehouses cost-effective for growing data needs.
- Improved Data Governance. Centralising data management enhances governance, security, and quality across all types of data.
Challenges
- Complexity in Implementation. Designing and implementing a Lakehouse architecture can be complex, requiring expertise in both Data Lakes and Data Warehouses.
- Data Consistency and Quality. Though crucial for reliable analytics, ensuring data consistency and quality across diverse data types and sources can be challenging.
- Governance and Security. Comprehensive data governance and security strategies are required to protect sensitive information and comply with regulations.
The choice between Data Warehouse, Data Lake, or Lakehouse systems is pivotal for businesses in harnessing the power of their data. Each option offers distinct advantages and challenges, requiring careful consideration of organisational needs and goals. By embracing the right data management system, organisations can pave the way for informed decision-making, operational efficiency, and innovation in the digital age.
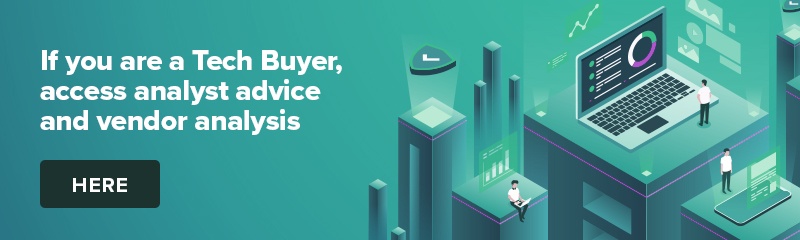
Ecosystm, supported by their partner Zurich Insurance, conducted an invitation-only Executive ThinkTank at the Point Zero Forum in Zurich, earlier this year. A select group of regulators, investors, and senior executives from financial institutions from across the globe came together to share their insights and experiences on the critical role data is playing in a digital economy, and the concrete actions that governments and businesses can take to allow a free flow of data that will help create a global data economy.
Here are the key takeaways from the ThinkTank.
- Bilateral Agreements for Transparency. Trade agreements play an important role in developing standards that ensure transparency across objective criteria. This builds the foundation for cross-border privacy and data protection measures, in alignment with local legislations.
- Building Trust is Crucial. Privacy and private data are defined differently across countries. One of the first steps is to establish common standards for opening up the APIs. This starts with building trust in common data platforms and establishing some standards and interoperability arrangements.
- Consumers Can Influence Cross-Border Data Exchange. Organisations should continue to actively lobby to change regulator perspectives on data exchange. But, the real impact will be created when consumers come into the conversation – as they are the ones who will miss out on access to global and uniform services due to restrictions in cross-country data sharing.
Read below to find out more.
Click here to download “Opportunities Created by Cross Border Data Flows” as a PDF
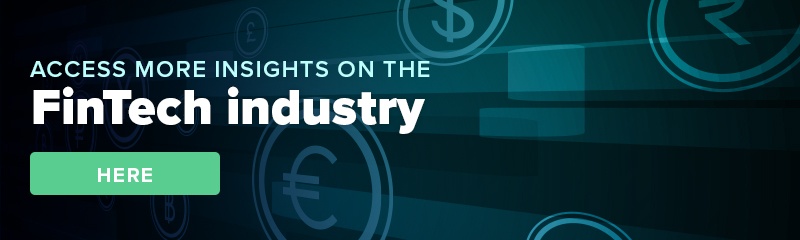
Organisations have had to transform and innovate to survive over the last two years. However, now when they look at their competitors, they see that everyone has innovated at about the same pace. The 7-year innovation cycle is history in today’s world – organisations need the right strategy and technologies to bring the time to market for innovations down to 1-2 years.
As they continue to innovate to stay ahead of the competition, here are 5 things organisations in India should keep in mind:
- The drivers of innovation will shift rapidly and industry trends need to be monitored continually to adapt to these shifts.
- Their biggest challenge in deploying Data & AI solutions will be identification of the right data for the right purpose – this will require a robust data architecture.
- While customer experience gives them immediate and tangible benefits, employee experience is almost equally – if not more – important.
- Cloud investments have helped build distributed enterprises – but streamlining investments needs a lot of focus now.
- There is a misalignment between organisations’ overall awareness of growing cyber threats and risks and their responses to them. A new cyber approach is urgently needed.
More insights into the India tech market are below.
Click here to download The Future of the Digital Enterprise – Southeast Asia as a PDF

Earlier this month, I had the privilege of attending Oracle’s Executive Leadership Forum, to mark the launch of the Oracle Cloud Singapore Region. Oracle now has 34 cloud regions worldwide across 17 countries and intends to expand their footprint further to 44 regions by the end of 2022. They are clearly aiming for rapid expansion across the globe, leveraging their customers’ need to migrate to the cloud. The new Singapore region aims to support the growing demand for enterprise cloud services in Southeast Asia, as organisations continue to focus on business and digital transformation for recovery and future success.
Here are my key takeaways from the session:
#1 Enabling the Digital Futures
The theme for the session revolved around Digital Futures. Ecosystm research shows that 77% of enterprises in Southeast Asia are looking at technology to pivot, shift, change and adapt for the Digital Futures. Organisations are re-evaluating and accelerating the use of digital technology for back-end and customer workloads, as well as product development and innovation. Real-time data access lies at the backbone of these technologies. This means that Digital & IT Teams must build the right and scalable infrastructure to empower a digital, data-driven organisation. However, being truly data-driven requires seamless data access, irrespective of where they are generated or stored, to unlock the full value of the data and deliver the insights needed. Oracle Cloud is focused on empowering this data-led economy through data sovereignty, lower latency, and resiliency.
The Oracle Cloud Singapore Region brings to Southeast Asia an integrated suite of applications and the Oracle Cloud Infrastructure (OCI) platform that aims to help run native applications, migrate, and modernise them onto cloud. There has been a growing interest in hybrid cloud in the region, especially in large enterprises. Oracle’s offering will give companies the flexibility to run their workloads on their cloud and/or on premises. With the disruption that the pandemic has caused, it is likely that Oracle customers will increasingly use the local region for backup and recovery of their on-premises workloads.
#2 Partnering for Success
Oracle has a strong partner ecosystem of collaboration platforms, consulting and advisory firms and co-location providers, that will help them consolidate their global position. To begin with they rely on third-party co-location providers such as Equinix and Digital Realty for many of their data centres. While Oracle will clearly benefit from these partnerships, the benefit that they can bring to their partners is their ability to build a data fabric – the architecture and services. Organisations are looking to build a digital core and layer data and AI solutions on top of the core; Oracle’s ability to handle complex data structures will be important to their tech partners and their route to market.
#3 Customers Benefiting from Oracle’s Core Strengths
The session included some customer engagement stories, that highlight Oracle’s unique strengths in the enterprise market. One of Oracle’s key clients in the region, Beyonics – a precision manufacturing company for the Healthcare, Automotive and Technology sectors – spoke about how Oracle supported them in their migration and expansion of ERP platform from 7 to 22 modules onto the cloud. Hakan Yaren, CIO, APL Logistics says, “We have been hosting our data lake initiative on OCI and the data lake has helped us consolidate all these complex data points into one source of truth where we can further analyse it”.
In both cases what was highlighted was that Oracle provided the platform with the right capacity and capabilities for their business growth. This demonstrates the strength of Oracle’s enterprise capabilities. They are perhaps the only tech vendor that can support enterprises equally for their database, workloads, and hardware requirements. As organisations look to transform and innovate, they will benefit from the strength of these enterprise-wide capabilities that can address multiple pain points of their digital journeys.
#4 Getting Front and Centre of the Start-up Ecosystem
One of the most exciting announcements for me was Oracle’s focus on the start-up ecosystem. They make a start with a commitment to offer 100 start-ups in Singapore USD 30,000 each, in Oracle Cloud credits over the next two years. This is good news for the country’s strong start-up community. It will be good to see Oracle build further on this support so that start-ups can also benefit from Oracles’ enterprise offerings. This will be a win-win for Oracle. The companies they support could be “soonicorns” – the unicorns of tomorrow; and Oracle will get the opportunity to grow their accounts as these companies grow. Given the momentum of the data economy, these start-ups can benefit tremendously from the core differentiators that OCI can bring to their data fabric design. While this is a good start, Oracle should continue to engage with the start-up community – not just in Singapore but across Southeast Asia.
#5 Commitment to Sustainability at the Core of the Digital Futures
Another area where Oracle is aligning themselves to the future is in their commitment to sustainability. Earlier this year they pledged to power their global operations with 100% renewable energy by 2025, with goals set for clean cloud, hardware recycling, waste reduction and responsible sourcing. As Jacqueline Poh, Managing Director, EDB Singapore pointed out, sustainability can no longer be an afterthought and must form part of the core growth strategy. Oracle has aligned themselves to the SG Green Plan that aims to achieve sustainability targets under the UN’s 2030 Sustainable Development Agenda.
Cloud infrastructure is going to be pivotal in shaping the future of the Digital Economy; but the ability to keep sustainability at its core will become a key differentiator. To quote Sir David Attenborough from his speech at COP26, “In my lifetime, I’ve witnessed a terrible decline. In yours, you could and should witness a wonderful recovery”
Conclusion
Oracle operates in a hyper competitive world – AWS, Microsoft and Google have emerged as the major hyperscalers over the last few years. With their global expansion plans and targeted offerings to help enterprises achieve their transformation goals, Oracle is positioned well to claim a larger share of the cloud market. Their strength lies in the enterprise market, and their cloud offerings should see them firmly entrenched in that segment. I hope however, that they will keep an equal focus on their commitment to the start-up ecosystem. Most of today’s hyperscalers have been successful in building scale by deeply entrenching themselves in the core innovation ecosystem – building on the ‘possibilities’ of the future rather than just on the ‘financial returns’ today.
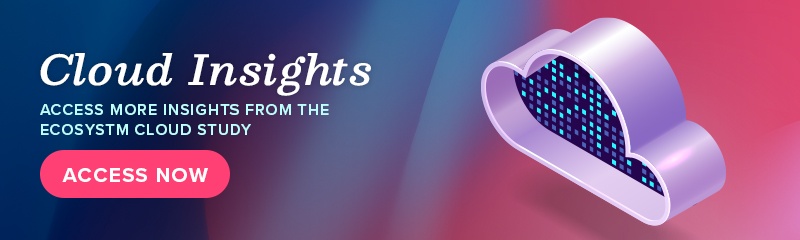
AI has become intrinsic to our personal lives – we are often completely unaware of technology’s influence on our daily lives. For enterprises too, tech solutions often come embedded with AI capabilities. Today, an organisation’s ability to automate processes and decisions is often dependent more on their desire and appetite for tech adoption, than the technology itself.
In 2022 the key focus for enterprises will be on being able to trust their Data & AI solutions. This will include trust in their IT infrastructure, architecture and AI services; and stretch to being able to participate in trusted data sharing models. Technology vendors will lead this discussion and showcase their solutions in the light of trust.
Read what Ecosystm analysts, Darian Bird, Niloy Mukherjee, Peter Carr and Tim Sheedy think will be the leading Data & AI trends in 2022.
Click here to download Ecosystm Predicts: The Top 5 Trends for Data & AI in 2022 as PDF
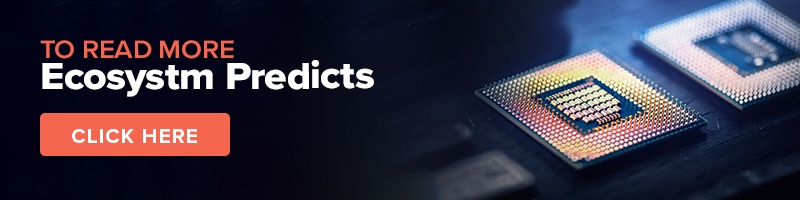