Ecosystm research reveals a stark reality: 75% of technology leaders in Financial Services anticipate data breaches.
Given the sector’s regulatory environment, data breaches carry substantial financial implications, emphasising the critical importance of giving precedence to cybersecurity. This is compelling a fresh cyber strategy focused on early threat detection and reduction of attack impact.
Read on to find out how tech leaders are building a culture of cyber-resilience, re-evaluating their cyber policies, and adopting technologies that keep them one step ahead of their adversaries.
Download ‘Cyber-Resilience in Finance: People, Policy & Technology’ as a PDF
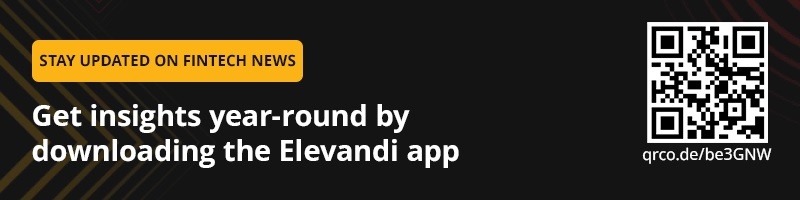
The impact of AI on Customer Experience (CX) has been profound and continues to expand. AI allows a a range of advantages, including improved operational efficiency, cost savings, and enhanced experiences for both customers and employees.
AI-powered solutions have the capability to analyse vast volumes of customer data in real-time, providing organisations with invaluable insights into individual preferences and behaviour. When executed effectively, the ability to capture, analyse, and leverage customer data at scale gives organisations significant competitive edge. Most importantly, AI unlocks opportunities for innovation.
Read on to discover the transformative impact of AI on customer experiences.
Click here to download ‘Customer Experience Redefined: The Role of AI’ as a PDF
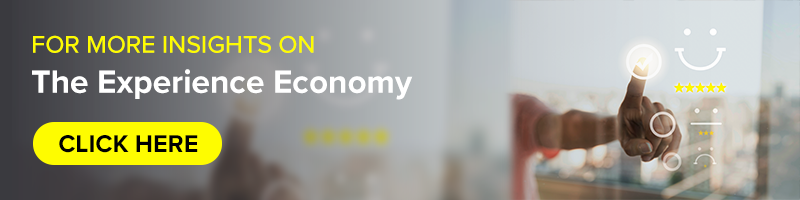
Generative AI has stolen the limelight in 2023 from nearly every other technology – and for good reason. The advances made by Generative AI providers have been incredible, with many human “thinking” processes now in line to be automated.
But before we had Generative AI, there was the run-of-the-mill “traditional AI”. However, despite the traditional tag, these capabilities have a long way to run within your organisation. In fact, they are often easier to implement, have less risk (and more predictability) and are easier to generate business cases for. Traditional AI systems are often already embedded in many applications, systems, and processes, and can easily be purchased as-a-service from many providers.
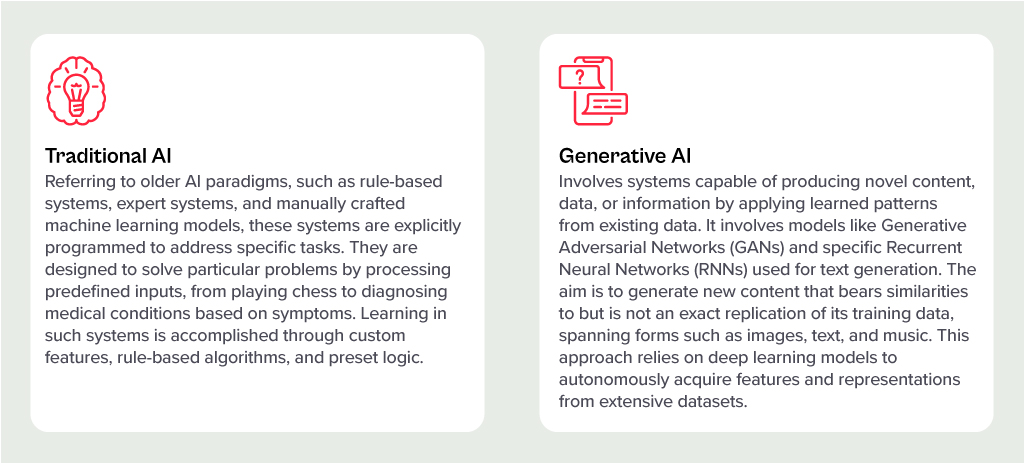
Unlocking the Potential of AI Across Industries
Many organisations around the world are exploring AI solutions today, and the opportunities for improvement are significant:
- Manufacturers are designing, developing and testing in digital environments, relying on AI to predict product responses to stress and environments. In the future, Generative AI will be called upon to suggest improvements.
- Retailers are using AI to monitor customer behaviours and predict next steps. Algorithms are being used to drive the best outcome for the customer and the retailer, based on previous behaviours and trained outcomes.
- Transport and logistics businesses are using AI to minimise fuel usage and driver expenses while maximising delivery loads. Smart route planning and scheduling is ensuring timely deliveries while reducing costs and saving on vehicle maintenance.
- Warehouses are enhancing the safety of their environments and efficiently moving goods with AI. Through a combination of video analytics, connected IoT devices, and logistical software, they are maximising the potential of their limited space.
- Public infrastructure providers (such as shopping centres, public transport providers etc) are using AI to monitor public safety. Video analytics and sensors is helping safety and security teams take public safety beyond traditional human monitoring.
AI Impacts Multiple Roles
Even within the organisation, different lines of business expect different outcomes for AI implementations.
- IT teams are monitoring infrastructure, applications, and transactions – to better understand root-cause analysis and predict upcoming failures – using AI. In fact, AIOps, one of the fastest-growing areas of AI, yields substantial productivity gains for tech teams and boosts reliability for both customers and employees.
- Finance teams are leveraging AI to understand customer payment patterns and automate the issuance of invoices and reminders, a capability increasingly being integrated into modern finance systems.
- Sales teams are using AI to discover the best prospects to target and what offers they are most likely to respond to.
- Contact centres are monitoring calls, automating suggestions, summarising records, and scheduling follow-up actions through conversational AI. This is allowing to get agents up to speed in a shorter period, ensuring greater customer satisfaction and increased brand loyalty.
Transitioning from Low-Risk to AI-Infused Growth
These are just a tiny selection of the opportunities for AI. And few of these need testing or business cases – many of these capabilities are available out-of-the-box or out of the cloud. They don’t need deep analysis by risk, legal, or cybersecurity teams. They just need a champion to make the call and switch them on.
One potential downside of Generative AI is that it is drawing unwarranted attention to well-established, low-risk AI applications. Many of these do not require much time from data scientists – and if they do, the challenge is often finding the data and creating the algorithm. Humans can typically understand the logic and rules that the models create – unlike Generative AI, where the outcome cannot be reverse-engineered.
The opportunity today is to take advantage of the attention that LLMs and other Generative AI engines are getting to incorporate AI into every conceivable aspect of a business. When organisations understand the opportunities for productivity improvements, speed enhancement, better customer outcomes and improved business performance, the spend on AI capabilities will skyrocket. Ecosystm estimates that for most organisations, AI spend will be less than 5% of their total tech spend in 2024 – but it is likely to grow to over 20% within the next 4-5 years.
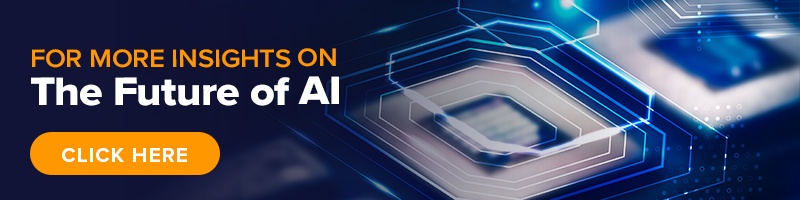
It seems for many employees, the benefits of working from home or even adopting a hybrid model are a thing of the past. Employees are returning to the grind of long commutes and losing hours in transit. What is driving this shift in sentiment? CEOs, who once rooted for remote work, have undergone a change of heart – many say that remote work hampers their ability to innovate.
That may not be the real reason, however. There is a good chance that the CEO and/or other managers feel they have lost control or visibility over their employees. Returning to a more traditional management approach, where everyone is within direct sight, might seem like a simpler solution.
The Myths of Workplace Innovation
I find it ironic that organisations say they want employees to come into the office because they cannot innovate at the same rate. What the last few years have demonstrated – and quite conclusively – is that employees can innovate wherever they are, if they are driven to it and have the right tools. So, organisations need to evaluate whether they have innovated on and evolved their hybrid and remote work solutions effectively, to continue to support hybrid work – and innovation.
What is confusing about this stance that many organisations are taking, is that when an organisation has multiple offices, they are effectively a hybrid business – they have had people working from different locations, but have never felt the need to get all their staff together for 3-5 days every week for organisation-wide innovation that is suddenly so important today.
The CEO of a tech research firm once said – the office used to be considered the place to get together to use the tools we need to innovate; but the reality is that the office is just one of the tools that businesses have, to drive their organisation forward. Ironically, this same CEO has recently called everyone back into the office 3 days a week!
Is Remote Work the Next Step in Employee Rights?
It has become clear that remote and hybrid work is the next step in employees getting greater rights. Many organisations fought against the five-day work weeks, claiming they wouldn’t make as much money as they did when employees worked whenever they were told. They fought against the 40-hour work week (in France some fought against the 35-hour work week!) They fight against the introduction of new public holidays, against increases to the minimum wages, against paid parental leave.
Some industries, companies, unions, and countries are looking to (or already have) formalised hybrid and remote work in their policies and regulations. More unions and businesses will do this – and employees will have choice.
People will have the option to work for an employer who wants their employees to come into the office – or work for someone else. And this will depend on preferences and working styles – some employees enjoy the time spent away from home and like the social nature of office environments. But many also like the extra time, money, and flexibility that remote work allows.
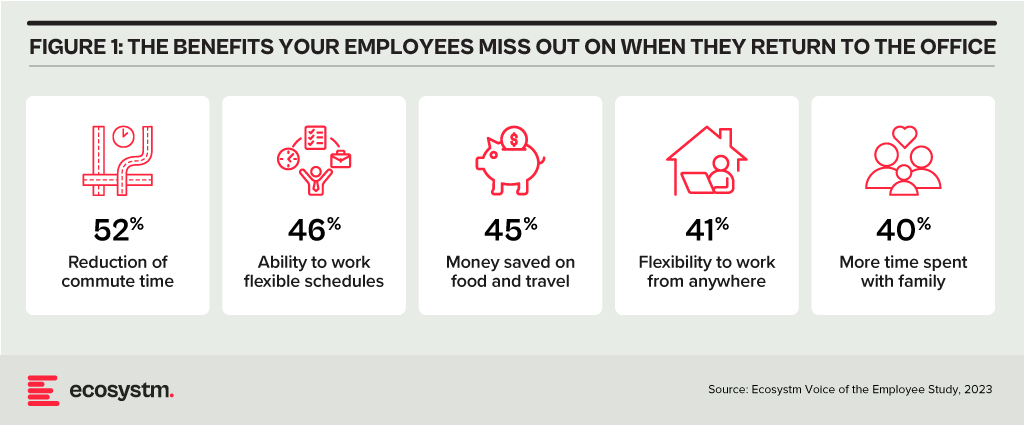
There might be many reasons why leadership teams would want employees to come into the office – and establishing and maintaining a common corporate culture would be a leading reason. But what they need to do is stop pretending it is about “innovation”. Innovation is possible while working remotely, as it is when working from separate offices or even different floors within the same building.
Evolving Employee Experience & Collaboration Needs
Organisations today face a challenge – and it is not the inability to innovate in a hybrid work environment! It is in their ability to deliver the employee experience that their employees want. This is more challenging now because there are more preferences, options, and technologies available. But it is established that organisations need to continue to evolve their employee experience.
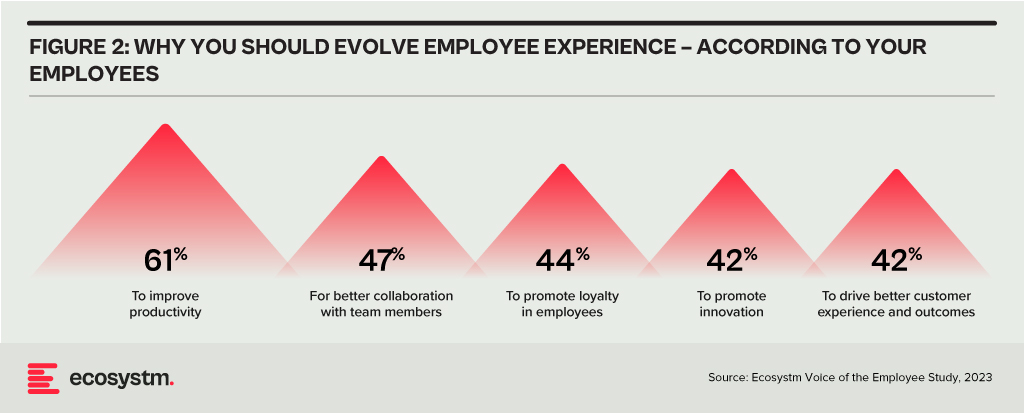
Technology does and will continue to play an important role in keeping our employees connected and productive. AI – such as Microsoft Copilot – will continue to improve our productivity. But the management needs to evolve with the technology. If the senior management feels that connecting people will help to solve the current growth challenges in the business, then it becomes the role of managers to better connect people – not just teams in offices, but virtual teams across the entire organisation.
Organisations that have focused their energies on connecting their employees better, regardless of their location (such as REA in Australia), find that productivity and innovation rates are better than when people are physically together. What do they do differently?
- Managers find their roles have moved from supporting individual employees to connecting employees
- Documentation of progress and challenges means that everyone knows where to focus their energies
- Managed virtual (and in-person) meetings mean that everyone has a voice and gets to contribute (not the loudest, most talkative or most senior person)
Remote and hybrid workers are often well-positioned to come up with new and innovative ideas. Senior management can encourage innovation and risk-taking by creating a safe environment for employees to share their ideas and by providing them with the resources they need to develop and implement their ideas. Sometimes these resources are in an office – but they don’t have to be. Manufacturers are quickly moving to complete digital development, prototyping, and testing of their new and improved products and services. Digital is often faster, better, and more innovative than physical – but employees need to be allowed to embrace these new platforms and tools to drive better organisational and customer outcomes.
What the pandemic has taught us is that people are good at solving problems; they are good at innovating irrespective of whether their managers are watching or not.
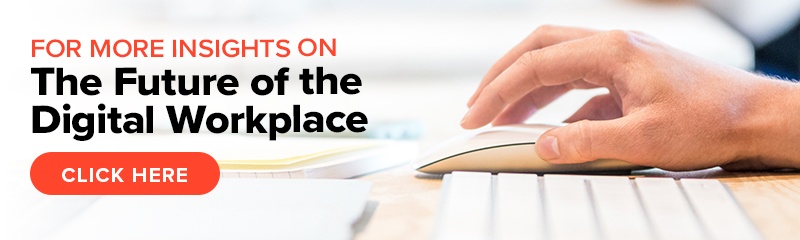
While there has been much speculation about AI being a potential negative force on humanity, what we do know today is that the accelerated use of AI WILL mean an accelerated use of energy. And if that energy source is not renewable, AI will have a meaningful negative impact on CO2 emissions and will accelerate climate change. Even if the energy is renewable, GPUs and CPUs generate significant heat – and if that heat is not captured and used effectively then it too will have a negative impact on warming local environments near data centres.
Balancing Speed and Energy Efficiency
While GPUs use significantly more energy than CPUs, they run many AI algorithms faster than CPUs – so use less energy overall. But the process needs to run – and these are additional processes. Data needs to be discovered, moved, stored, analysed, cleansed. In many cases, algorithms need to be recreated, tweaked and improved. And then that algorithm itself will kick off new digital processes that are often more processor and energy-intensive – as now organisations might have a unique process for every customer or many customer groups, requiring more decisioning and hence more digitally intensive.
The GPUs, servers, storage, cabling, cooling systems, racks, and buildings have to be constructed – often built from raw materials – and these raw materials need to be mined, transported and transformed. With the use of AI exploding at the moment, so is the demand for AI infrastructure – all of which has an impact on the resources of the planet and ultimately on climate change.
Sustainable Sourcing
Some organisations understand this already and are beginning to use sustainable sourcing for their technology services. However, it is not a top priority with Ecosystm research showing only 15% of organisations focus on sustainable procurement.
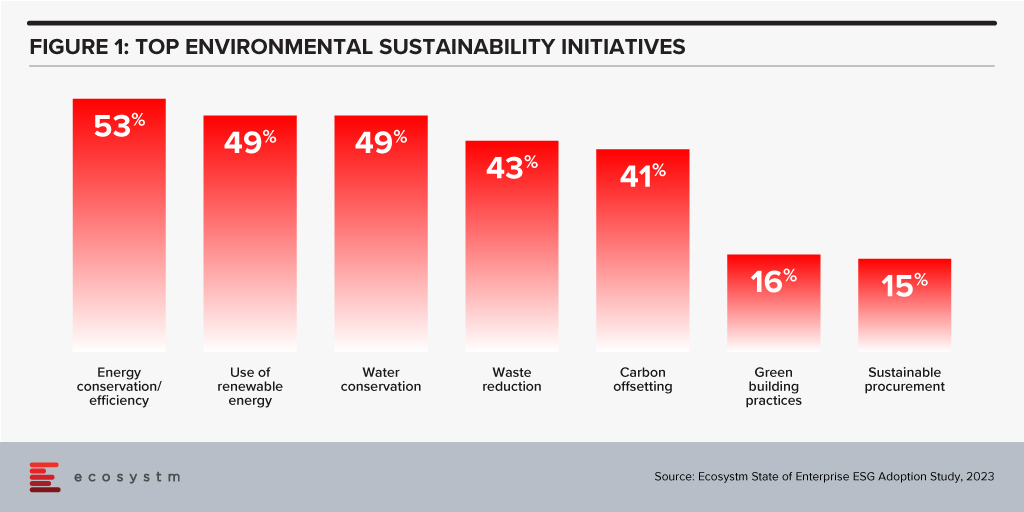
Technology Providers Can Help
Leading technology providers are introducing initiatives that make it easier for organisations to procure sustainable IT solutions. The recently announced HPE GreenLake for Large Language Models will be based in a data centre built and run by Qscale in Canada that is not only sustainably built and sourced, but sits on a grid supplying 99.5% renewable electricity – and waste (warm) air from the data centre and cooling systems is funneled to nearby greenhouses that grow berries. I find the concept remarkable and this is one of the most impressive sustainable data centre stories to date.
The focus on sustainability needs to be universal – across all cloud and AI providers. AI usage IS exploding – and we are just at the tip of the iceberg today. It will continue to grow as it becomes easier to use and deploy, more readily available, and more relevant across all industries and organisations. But we are at a stage of climate warming where we cannot increase our greenhouse gas emissions – and offsetting these emissions just passes the buck.
We need more companies like HPE and Qscale to build this Sustainable Future – and we need to be thinking the same way in our own data centres and putting pressure on our own AI and overall technology value chain to think more sustainably and act in the interests of the planet and future generations. Cloud providers – like AWS – are committed to the NetZero goal (by 2040 in their case) – but this is meaningless if our requirement for computing capacity increases a hundred-fold in that period. Our businesses and our tech partners need to act today. It is time for organisations to demand it from their tech providers to influence change in the industry.
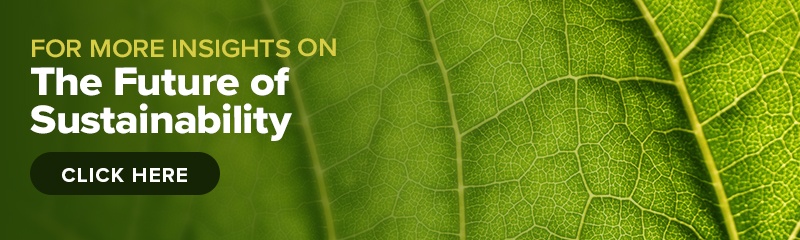
Technology has been reshaping the Real Estate industry landscape. Advancements in manufacturing technologies, digital tools, AI & analytics, and IoT – coupled with customer and employee expectations – are revolutionising how properties are built, bought, sold, managed, and experienced.
The evolution of RealTech and PropTech has a far-reaching impact on the industry, streamlining processes, improving customer experiences, and driving innovation across the entire sector.
Read on to find out how technology impacts the entire value chain; the key drivers of Real Estate evolution; the strong influence of “smart consumers”; and what Ecosystm VP Industry Insights Sash Mukherjee thinks where the industry is headed.
Download ‘The Future of Real Estate’ as a PDF
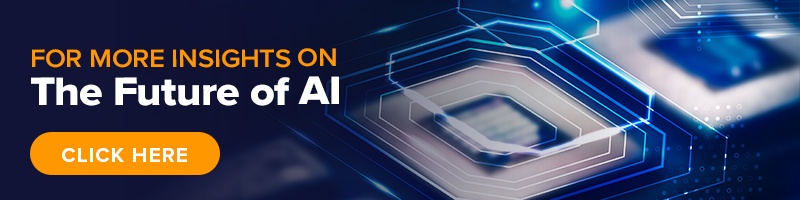
Technology is reshaping the Public Sector worldwide, optimising operations, improving citizen services, and fostering data-driven decision-making. Government agencies are also embracing innovation for effective governance in this digital era.
Public sector organisations worldwide recognise the need for swift and agile interventions. With citizen expectations resembling those of commercial customers, public sector organisations face mounting pressure to break down the barriers to provide seamless service experiences.
Read on to find out how public sector organisations in countries such as Australia, Vietnam, the Philippines, South Korea, and Singapore are innovating to stay ahead of the curve; and what Ecosystm VP Consulting, Peter Carr sees as the Future of Public Sector.
Click here to Download ‘The Future of the Public Sector’ as a PDF
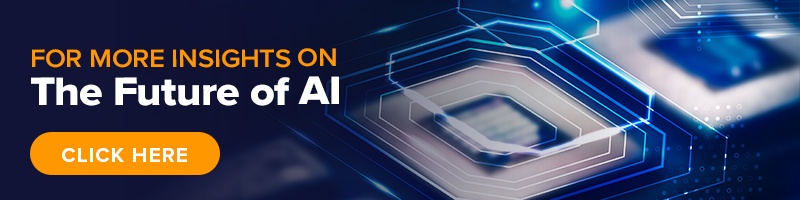
I have spent many years analysing the mobile and end-user computing markets. Going all the way back to 1995 where I was part of a Desktop PC research team, to running the European wireless and mobile comms practice, to my time at 3 Mobile in Australia and many years after, helping clients with their end-user computing strategies. From the birth of mobile data services (GPRS, WAP, and so on to 3G, 4G and 5G), from simple phones to powerful foldable devices, from desktop computers to a complex array of mobile computing devices to meet the many and varied employee needs. I am always looking for the “next big thing” – and there have been some significant milestones – Palm devices, Blackberries, the iPhone, Android, foldables, wearables, smaller, thinner, faster, more powerful laptops.
But over the past few years, innovation in this space has tailed off. Outside of the foldable space (which is already four years old), the major benefits of new devices are faster processors, brighter screens, and better cameras. I review a lot of great computers too (like many of the recent Surface devices) – and while they are continuously improving, not much has got my clients or me “excited” over the past few years (outside of some of the very cool accessibility initiatives).
The Force of AI
But this is all about to change. Devices are going to get smarter based on their data ecosystem, the cloud, and AI-specific local processing power. To be honest, this has been happening for some time – but most of the “magic” has been invisible to us. It happened when cameras took multiple shots and selected the best one; it happened when pixels were sharpened and images got brighter, better, and more attractive; it happened when digital assistants were called upon to answer questions and provide context.
Microsoft, among others, are about to make AI smarts more front and centre of the experience – Windows Copilot will add a smart assistant that can not only advise but execute on advice. It will help employees improve their focus and productivity, summarise documents and long chat threads, select music, distribute content to the right audience, and find connections. Added to Microsoft 365 Copilot it will help knowledge workers spend less time searching and reading – and more time doing and improving.
The greater integration of public and personal data with “intent insights” will also play out on our mobile devices. We are likely to see the emergence of the much-promised “integrated app”– one that can take on many of the tasks that we currently undertake across multiple applications, mobile websites, and sometimes even multiple devices. This will initially be through the use of public LLMs like Bard and ChatGPT, but as more custom, private models emerge they will serve very specific functions.
Focused AI Chips will Drive New Device Wars
In parallel to these developments, we expect the emergence of very specific AI processors that are paired to very specific AI capabilities. As local processing power becomes a necessity for some AI algorithms, the broad CPUs – and even the AI-focused ones (like Google’s Tensor Processor) – will need to be complemented by specific chips that serve specific AI functions. These chips will perform the processing more efficiently – preserving the battery and improving the user experience.
While this will be a longer-term trend, it is likely to significantly change the game for what can be achieved locally on a device – enabling capabilities that are not in the realm of imagination today. They will also spur a new wave of device competition and innovation – with a greater desire to be on the “latest and greatest” devices than we see today!
So, while the levels of device innovation have flattened, AI-driven software and chipset innovation will see current and future devices enable new levels of employee productivity and consumer capability. The focus in 2023 and beyond needs to be less on the hardware announcements and more on the platforms and tools. End-user computing strategies need to be refreshed with a new perspective around intent and intelligence. The persona-based strategies of the past have to be changed in a world where form factors and processing power are less relevant than outcomes and insights.
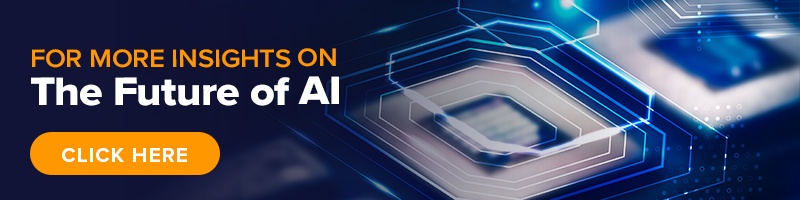
All growth must end eventually. But it is a brave person who will predict the end of growth for the public cloud hyperscalers. The hyperscaler cloud revenues have been growing at between 25-60% the past few years (off very different bases – and often including and counting different revenue streams). Even the current softening of economic spend we are seeing across many economies is only causing a slight slowdown.
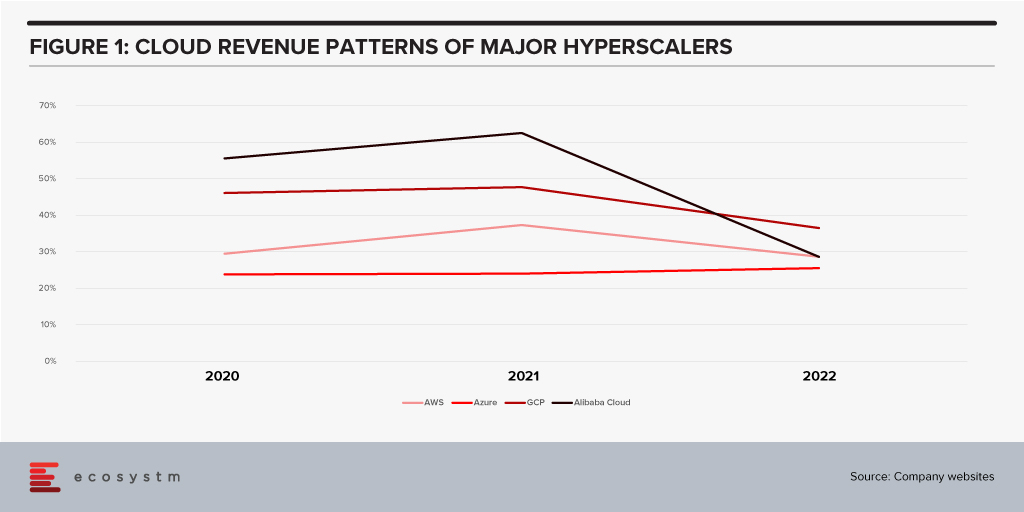
Looking forward, we expect growth in public cloud infrastructure and platform spend to continue to decline in 2024, but to accelerate in 2025 and 2026 as businesses take advantage of new cloud services and capabilities. However, the sheer size of the market means that we will see slower growth going forward – but we forecast 2026 to see the highest revenue growth of any year since public cloud services were founded.
The factors driving this growth include:
- Acceleration of digital intensity. As countries come out of their economic slowdowns and economic activity increases, so too will digital activity. And greater volumes of digital activity will require an increase in the capacity of cloud environments on which the applications and processes are hosted.
- Increased use of AI services. Businesses and AI service providers will need access to GPUs – and eventually, specialised AI chipsets – which will see cloud bills increase significantly. The extra data storage to drive the algorithms – and the increase in CPU required to deliver customised or personalised experiences that these algorithms will direct will also drive increased cloud usage.
- Further movement of applications from on-premises to cloud. Many organisations – particularly those in the Asia Pacific region – still have the majority of their applications and tech systems sitting in data centre environments. Over the next few years, more of these applications will move to hyperscalers.
- Edge applications moving to the cloud. As the public cloud giants improve their edge computing capabilities – in partnership with hardware providers, telcos, and a broader expansion of their own networks – there will be greater opportunity to move edge applications to public cloud environments.
- Increasing number of ISVs hosting on these platforms. The move from on-premise to cloud will drive some growth in hyperscaler revenues and activities – but the ISVs born in the cloud will also drive significant growth. SaaS and PaaS are typically seeing growth above the rates of IaaS – but are also drivers of the growth of cloud infrastructure services.
- Improving cloud marketplaces. Continuing on the topic of ISV partners, as the cloud hyperscalers make it easier and faster to find, buy, and integrate new services from their cloud marketplace, the adoption of cloud infrastructure services will continue to grow.
- New cloud services. No one has a crystal ball, and few people know what is being developed by Microsoft, AWS, Google, and the other cloud providers. New services will exist in the next few years that aren’t even being considered today. Perhaps Quantum Computing will start to see real business adoption? But these new services will help to drive growth – even if “legacy” cloud service adoption slows down or services are retired.
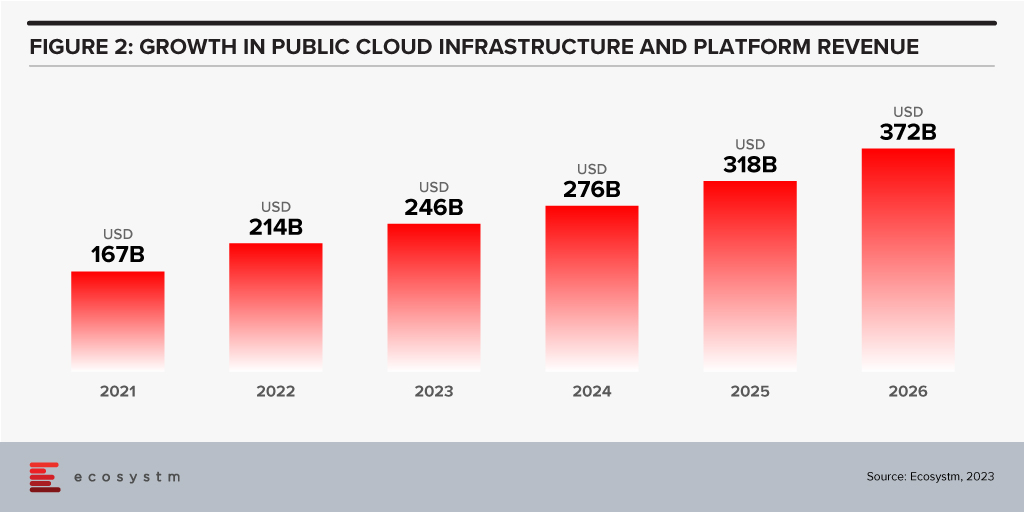
Hybrid Cloud Will Play an Important Role for Many Businesses
Growth in hyperscalers doesn’t mean that the hybrid cloud will disappear. Many organisations will hit a natural “ceiling” for their public cloud services. Regulations, proximity, cost, volumes of data, and “gravity” will see some applications remain in data centres. However, businesses will want to manage, secure, transform, and modernise these applications at the same rate and use the same tools as their public cloud environments. Therefore, hybrid and private cloud will remain important elements of the overall cloud market. Their success will be the ability to integrate with and support public cloud environments.
The future of cloud is big – but like all infrastructure and platforms, they are not a goal in themselves. It is what cloud is and will further enable businesses and customers which is exciting. As the rates of digitisation and digital intensity increase, the opportunities for the cloud infrastructure and platform providers will blossom. Sometimes they will be the driver of the growth, and other times they will just be supporting actors. But either way, in 2026 – 20 years after the birth of AWS – the growth in cloud services will be bigger than ever.
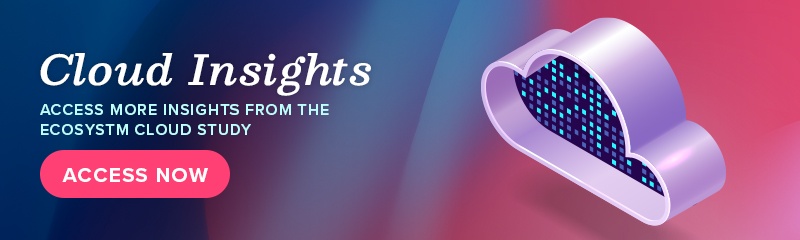