Data analysts play a vital role in today’s data-driven world, providing crucial insights that benefit decision-making processes. For those with a knack for numbers and a passion for uncovering patterns, a career as a data analyst can be both fulfilling and lucrative – it can also be a stepping stone towards other careers in data. While a data analyst focuses on data preparation and visualisation, an AI engineer specialises in creating AI solutions, a machine learning (ML) engineer concentrates on implementing ML models, and a data scientist combines elements of data analysis and ML to derive insights and predictions from data.
Tools, Skills, and Techniques of a Data Analyst
Excel Mastery. Unlocks a powerful toolbox for data manipulation and analysis. Essential skills include using a vast array of functions for calculations and data transformation. Pivot tables become your secret weapon for summarising and analysing large datasets, while charts and graphs bring your findings to life with visual clarity. Data validation ensures accuracy, and the Analysis ToolPak and Solver provide advanced functionalities for statistical analysis and complex problem-solving. Mastering Excel empowers you to transform raw data into actionable insights.
Advanced SQL. While basic skills handle simple queries, advanced users can go deeper with sorting, aggregation, and the art of JOINs to combine data from multiple tables. Common Table Expressions (CTEs) and subqueries become your allies for crafting complex queries, while aggregate functions summarise vast amounts of data. Window functions add another layer of power, allowing calculations within query results. Mastering Advanced SQL empowers you to extract hidden insights and manage data with unparalleled precision.
Data Visualisation. Crafts impactful data stories. These tools empower you to connect to various data sources, transform raw information into a usable format, and design interactive dashboards and reports. Filters and drilldowns allow users to explore your data from different angles, while calculated fields unlock deeper analysis. Parameters add a final touch of flexibility, letting viewers customise the report to their specific needs. With tools Tableau and Power BI, complex data becomes clear and engaging.
Essential Python. This powerful language excels at data analysis and automation. Libraries like NumPy and Pandas become your foundation for data manipulation and wrangling. Scikit-learn empowers you to build ML models, while SciPy and StatsModels provide a toolkit for in-depth statistical analysis. Python’s ability to interact with APIs and web scrape data expands its reach, and its automation capabilities streamline repetitive tasks. With Essential Python, you have the power to solve complex problems.
Automating the Journey. Data analysts can be masters of efficiency, and their skills translate beautifully into AI. Scripting languages like Ansible and Terraform automate repetitive tasks. Imagine streamlining the process of training and deploying AI models – a skill that directly benefits the AI development pipeline. This proficiency in automation showcases the valuable foundation data analysts provide for building and maintaining AI systems.
Developing ML Expertise. Transitioning from data analysis to AI involves building on your existing skills to develop ML expertise. As a data analyst, you may start with basic predictive models. This knowledge is expanded in AI to include deep learning and advanced ML algorithms. Also, skills in statistical analysis and visualisation help in evaluating the performance of AI models.
Growing Your AI Skills
Becoming an AI engineer requires building on a data analysis foundation to focus on advanced skills such as:
- Deep Learning. Learning frameworks like TensorFlow and PyTorch to build and train neural networks.
- Natural Language Processing (NLP). Techniques for processing and analysing large amounts of natural language data.
- AI Ethics and Fairness. Understanding the ethical implications of AI and ensuring models are fair and unbiased.
- Big Data Technologies. Using tools like Hadoop and Spark for handling large-scale data is essential for AI applications.
The Evolution of a Data Analyst: Career Opportunities
Data analysis is a springboard to AI engineering. Businesses crave talent that bridges the data-AI gap. Your data analyst skills provide the foundation (understanding data sources and transformations) to excel in AI. As you master ML, you can progress to roles like:
- AI Engineer. Works on integrating AI solutions into products and services. They work with AI frameworks like TensorFlow and PyTorch, ensuring that AI models are incorporated into products and services in a fair and unbiased manner.
- ML Engineer. Focuses on designing and implementing ML models. They focus on preprocessing data, evaluating model performance, and collaborating with data scientists and engineers to bring models into production. They need strong programming skills and experience with big data tools and ML algorithms.
- Data Scientist. Bridges the gap between data analysis and AI, often involved in both data preparation and model development. They perform exploratory data analysis, develop predictive models, and collaborate with cross-functional teams to solve complex business problems. Their role requires a comprehensive understanding of both data analysis and ML, as well as strong programming and data visualisation skills.
Conclusion
Hone your data expertise and unlock a future in AI! Mastering in-demand skills like Excel, SQL, Python, and data visualisation tools will equip you to excel as a data analyst. Your data wrangling skills will be invaluable as you explore ML and advanced algorithms. Also, your existing BI knowledge translates seamlessly into building and evaluating AI models. Remember, the data landscape is constantly evolving, so continue to learn to stay at the forefront of this dynamic field. By combining your data skills with a passion for AI, you’ll be well-positioned to tackle complex challenges and shape the future of AI.
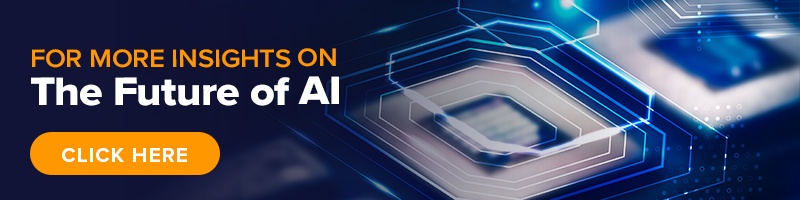
The data architecture outlines how data is managed in an organisation and is crucial for defining the data flow, data management systems required, the data processing operations, and AI applications. Data architects and engineers define data models and structures based on these requirements, supporting initiatives like data science. Before we delve into the right data architecture for your AI journey, let’s talk about the data management options. Technology leaders have the challenge of deciding on a data management system that takes into consideration factors such as current and future data needs, available skills, costs, and scalability. As data strategies become vital to business success, selecting the right data management system is crucial for enabling data-driven decisions and innovation.
Data Warehouse
A Data Warehouse is a centralised repository that stores vast amounts of data from diverse sources within an organisation. Its main function is to support reporting and data analysis, aiding businesses in making informed decisions. This concept encompasses both data storage and the consolidation and management of data from various sources to offer valuable business insights. Data Warehousing evolves alongside technological advancements, with trends like cloud-based solutions, real-time capabilities, and the integration of AI and machine learning for predictive analytics shaping its future.
Core Characteristics
- Integrated. It integrates data from multiple sources, ensuring consistent definitions and formats. This often includes data cleansing and transformation for analysis suitability.
- Subject-Oriented. Unlike operational databases, which prioritise transaction processing, it is structured around key business subjects like customers, products, and sales. This organisation facilitates complex queries and analysis.
- Non-Volatile. Data in a Data Warehouse is stable; once entered, it is not deleted. Historical data is retained for analysis, allowing for trend identification over time.
- Time-Variant. It retains historical data for trend analysis across various time periods. Each entry is time-stamped, enabling change tracking and trend analysis.
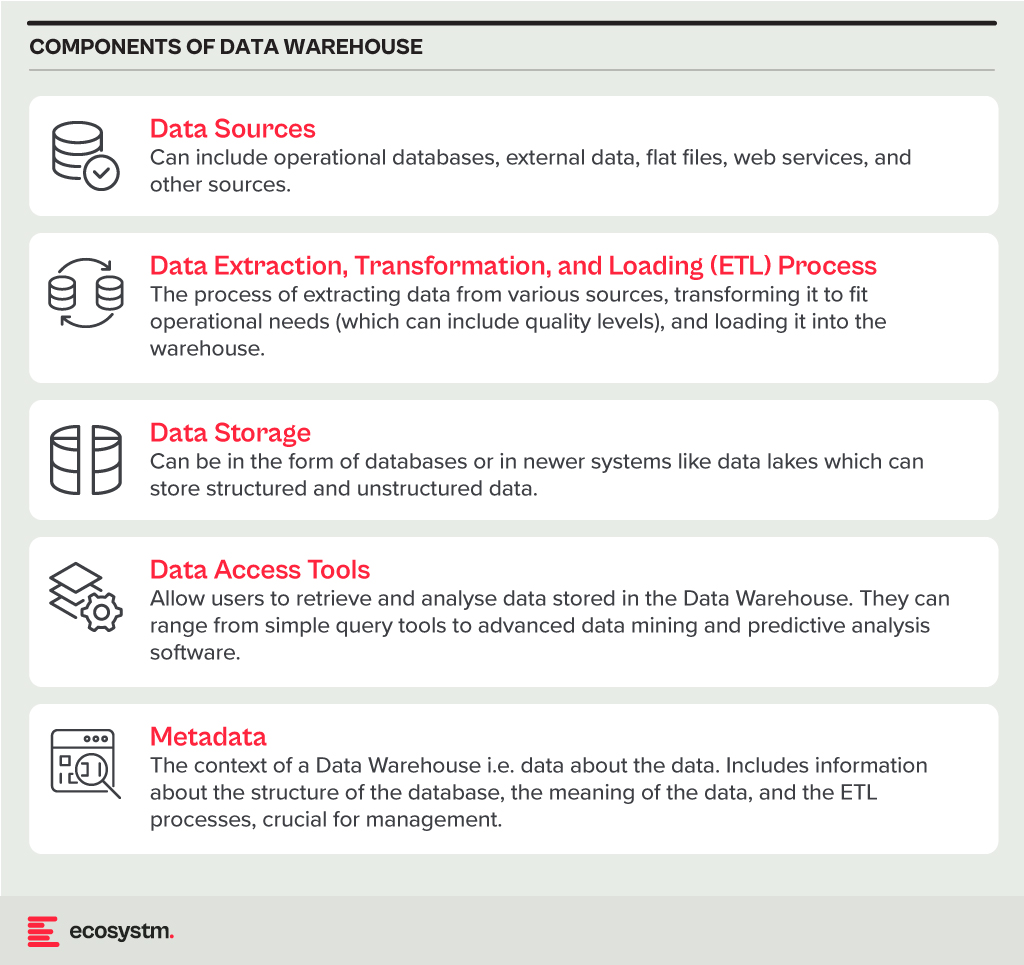
Benefits
- Better Decision Making. Data Warehouses consolidate data from multiple sources, offering a comprehensive business view for improved decision-making.
- Enhanced Data Quality. The ETL process ensures clean and consistent data entry, crucial for accurate analysis.
- Historical Analysis. Storing historical data enables trend analysis over time, informing future strategies.
- Improved Efficiency. Data Warehouses enable swift access and analysis of relevant data, enhancing efficiency and productivity.
Challenges
- Complexity. Designing and implementing a Data Warehouse can be complex and time-consuming.
- Cost. The cost of hardware, software, and specialised personnel can be significant.
- Data Security. Storing large amounts of sensitive data in one place poses security risks, requiring robust security measures.
Data Lake
A Data Lake is a centralised repository for storing, processing, and securing large volumes of structured and unstructured data. Unlike traditional Data Warehouses, which are structured and optimised for analytics with predefined schemas, Data Lakes retain raw data in its native format. This flexibility in data usage and analysis makes them crucial in modern data architecture, particularly in the age of big data and cloud.
Core Characteristics
- Schema-on-Read Approach. This means the data structure is not defined until the data is read for analysis. This offers more flexible data storage compared to the schema-on-write approach of Data Warehouses.
- Support for Multiple Data Types. Data Lakes accommodate diverse data types, including structured (like databases), semi-structured (like JSON, XML files), unstructured (like text and multimedia files), and binary data.
- Scalability. Designed to handle vast amounts of data, Data Lakes can easily scale up or down based on storage needs and computational demands, making them ideal for big data applications.
- Versatility. Data Lakes support various data operations, including batch processing, real-time analytics, machine learning, and data visualisation, providing a versatile platform for data science and analytics.
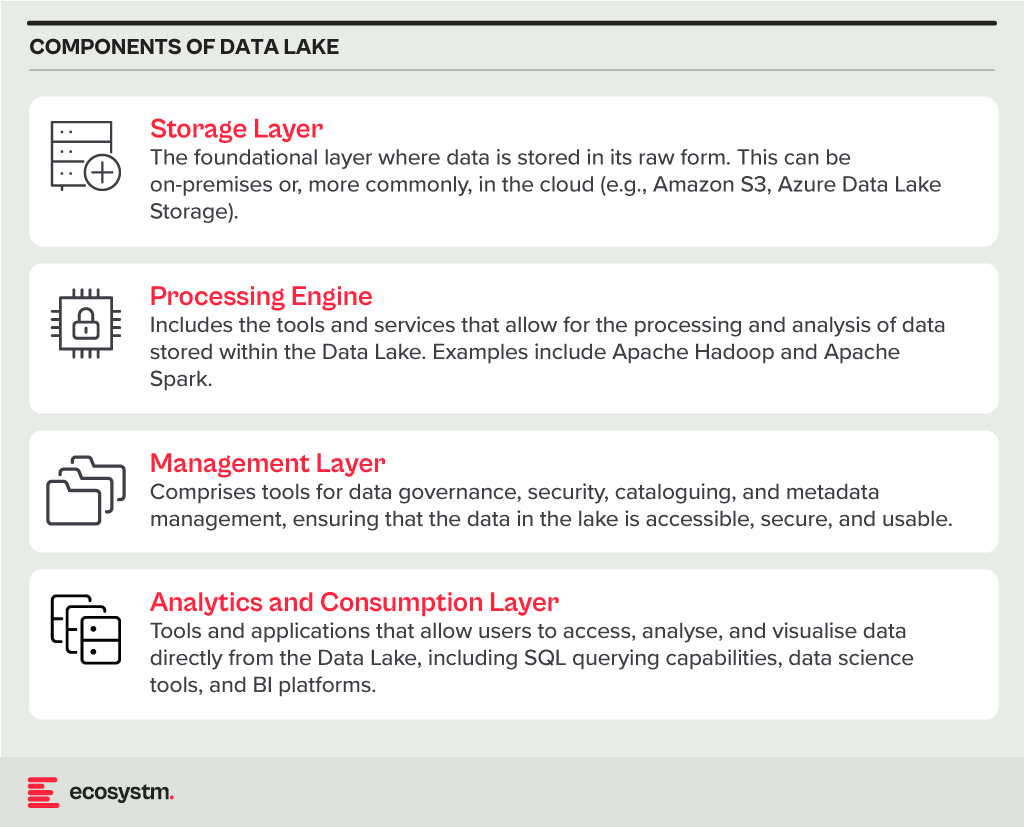
Benefits
- Flexibility. Data Lakes offer diverse storage formats and a schema-on-read approach for flexible analysis.
- Cost-Effectiveness. Cloud-hosted Data Lakes are cost-effective with scalable storage solutions.
- Advanced Analytics Capabilities. The raw, granular data in Data Lakes is ideal for advanced analytics, machine learning, and AI applications, providing deeper insights than traditional data warehouses.
Challenges
- Complexity and Management. Without proper management, a Data Lake can quickly become a “Data Swamp” where data is disorganised and unusable.
- Data Quality and Governance. Ensuring the quality and governance of data within a Data Lake can be challenging, requiring robust processes and tools.
- Security. Protecting sensitive data within a Data Lake is crucial, requiring comprehensive security measures.
Data Lakehouse
A Data Lakehouse is an innovative data management system that merges the strengths of Data Lakes and Data Warehouses. This hybrid approach strives to offer the adaptability and expansiveness of a Data Lake for housing extensive volumes of raw, unstructured data, while also providing the structured, refined data functionalities typical of a Data Warehouse. By bridging the gap between these two traditional data storage paradigms, Lakehouses enable more efficient data analytics, machine learning, and business intelligence operations across diverse data types and use cases.
Core Characteristics
- Unified Data Management. A Lakehouse streamlines data governance and security by managing both structured and unstructured data on one platform, reducing organizational data silos.
- Schema Flexibility. It supports schema-on-read and schema-on-write, allowing data to be stored and analysed flexibly. Data can be ingested in raw form and structured later or structured at ingestion.
- Scalability and Performance. Lakehouses scale storage and compute resources independently, handling large data volumes and complex analytics without performance compromise.
- Advanced Analytics and Machine Learning Integration. By providing direct access to both raw and processed data on a unified platform, Lakehouses facilitate advanced analytics, real-time analytics, and machine learning.
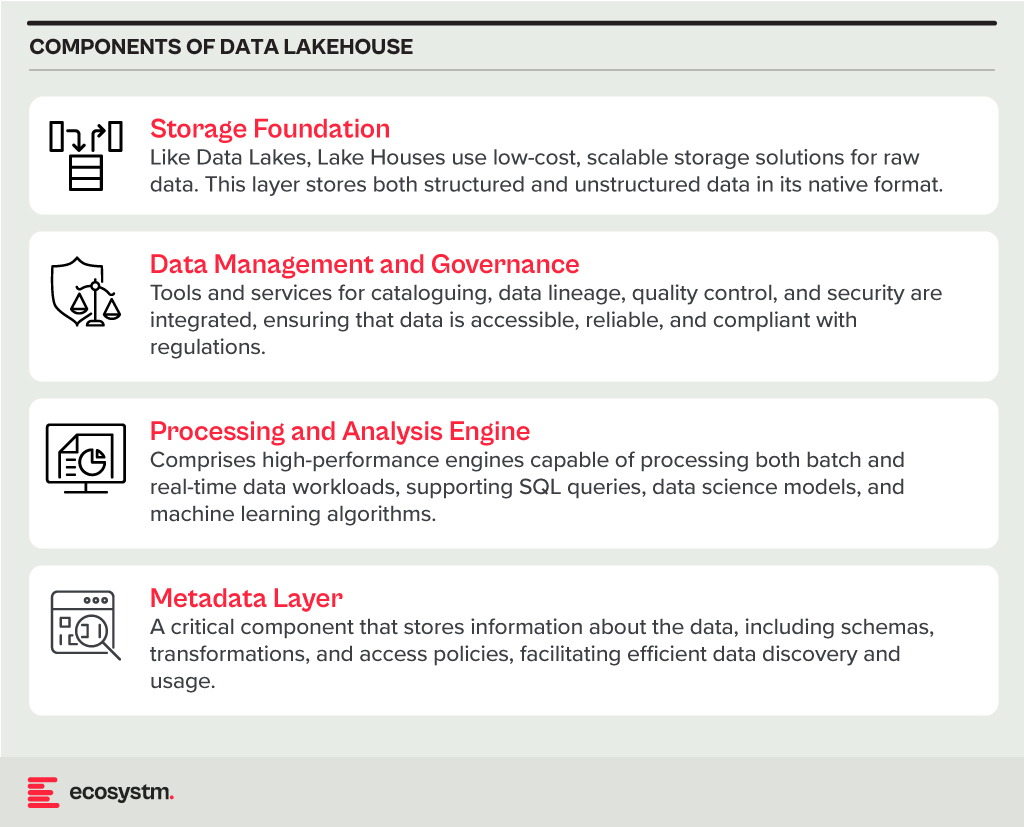
Benefits
- Versatility in Data Analysis. Lakehouses support diverse data analytics, spanning from traditional BI to advanced machine learning, all within one platform.
- Cost-Effective Scalability. The ability to scale storage and compute independently, often in a cloud environment, makes Lakehouses cost-effective for growing data needs.
- Improved Data Governance. Centralising data management enhances governance, security, and quality across all types of data.
Challenges
- Complexity in Implementation. Designing and implementing a Lakehouse architecture can be complex, requiring expertise in both Data Lakes and Data Warehouses.
- Data Consistency and Quality. Though crucial for reliable analytics, ensuring data consistency and quality across diverse data types and sources can be challenging.
- Governance and Security. Comprehensive data governance and security strategies are required to protect sensitive information and comply with regulations.
The choice between Data Warehouse, Data Lake, or Lakehouse systems is pivotal for businesses in harnessing the power of their data. Each option offers distinct advantages and challenges, requiring careful consideration of organisational needs and goals. By embracing the right data management system, organisations can pave the way for informed decision-making, operational efficiency, and innovation in the digital age.
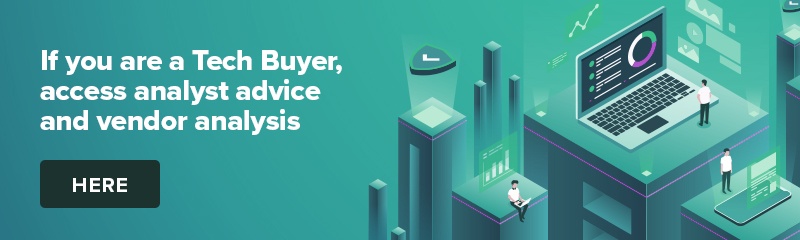
Historically, data scientists have been the linchpins in the world of AI and machine learning, responsible for everything from data collection and curation to model training and validation. However, as the field matures, we’re witnessing a significant shift towards specialisation, particularly in data engineering and the strategic role of Large Language Models (LLMs) in data curation and labelling. The integration of AI into applications is also reshaping the landscape of software development and application design.
The Growth of Embedded AI
AI is being embedded into applications to enhance user experience, optimise operations, and provide insights that were previously inaccessible. For example, natural language processing (NLP) models are being used to power conversational chatbots for customer service, while machine learning algorithms are analysing user behaviour to customise content feeds on social media platforms. These applications leverage AI to perform complex tasks, such as understanding user intent, predicting future actions, or automating decision-making processes, making AI integration a critical component of modern software development.
This shift towards AI-embedded applications is not only changing the nature of the products and services offered but is also transforming the roles of those who build them. Since the traditional developer may not possess extensive AI skills, the role of data scientists is evolving, moving away from data engineering tasks and increasingly towards direct involvement in development processes.
The Role of LLMs in Data Curation
The emergence of LLMs has introduced a novel approach to handling data curation and processing tasks traditionally performed by data scientists. LLMs, with their profound understanding of natural language and ability to generate human-like text, are increasingly being used to automate aspects of data labelling and curation. This not only speeds up the process but also allows data scientists to focus more on strategic tasks such as model architecture design and hyperparameter tuning.
The accuracy of AI models is directly tied to the quality of the data they’re trained on. Incorrectly labelled data or poorly curated datasets can lead to biased outcomes, mispredictions, and ultimately, the failure of AI projects. The role of data engineers and the use of advanced tools like LLMs in ensuring the integrity of data cannot be overstated.
The Impact on Traditional Developers
Traditional software developers have primarily focused on writing code, debugging, and software maintenance, with a clear emphasis on programming languages, algorithms, and software architecture. However, as applications become more AI-driven, there is a growing need for developers to understand and integrate AI models and algorithms into their applications. This requirement presents a challenge for developers who may not have specialised training in AI or data science. This is seeing an increasing demand for upskilling and cross-disciplinary collaboration to bridge the gap between traditional software development and AI integration.
Clear Role Differentiation: Data Engineering and Data Science
In response to this shift, the role of data scientists is expanding beyond the confines of traditional data engineering and data science, to include more direct involvement in the development of applications and the embedding of AI features and functions.
Data engineering has always been a foundational element of the data scientist’s role, and its importance has increased with the surge in data volume, variety, and velocity. Integrating LLMs into the data collection process represents a cutting-edge approach to automating the curation and labelling of data, streamlining the data management process, and significantly enhancing the efficiency of data utilisation for AI and ML projects.
Accurate data labelling and meticulous curation are paramount to developing models that are both reliable and unbiased. Errors in data labelling or poorly curated datasets can lead to models that make inaccurate predictions or, worse, perpetuate biases. The integration of LLMs into data engineering tasks is facilitating a transformation, freeing them from the burdens of manual data labelling and curation. This has led to a more specialised data scientist role that allocates more time and resources to areas that can create greater impact.
The Evolving Role of Data Scientists
Data scientists, with their deep understanding of AI models and algorithms, are increasingly working alongside developers to embed AI capabilities into applications. This collaboration is essential for ensuring that AI models are effectively integrated, optimised for performance, and aligned with the application’s objectives.
- Model Development and Innovation. With the groundwork of data preparation laid by LLMs, data scientists can focus on developing more sophisticated and accurate AI models, exploring new algorithms, and innovating in AI and ML technologies.
- Strategic Insights and Decision Making. Data scientists can spend more time analysing data and extracting valuable insights that can inform business strategies and decision-making processes.
- Cross-disciplinary Collaboration. This shift also enables data scientists to engage more deeply in interdisciplinary collaboration, working closely with other departments to ensure that AI and ML technologies are effectively integrated into broader business processes and objectives.
- AI Feature Design. Data scientists are playing a crucial role in designing AI-driven features of applications, ensuring that the use of AI adds tangible value to the user experience.
- Model Integration and Optimisation. Data scientists are also involved in integrating AI models into the application architecture, optimising them for efficiency and scalability, and ensuring that they perform effectively in production environments.
- Monitoring and Iteration. Once AI models are deployed, data scientists work on monitoring their performance, interpreting outcomes, and making necessary adjustments. This iterative process ensures that AI functionalities continue to meet user needs and adapt to changing data landscapes.
- Research and Continued Learning. Finally, the transformation allows data scientists to dedicate more time to research and continued learning, staying ahead of the rapidly evolving field of AI and ensuring that their skills and knowledge remain cutting-edge.
Conclusion
The integration of AI into applications is leading to a transformation in the roles within the software development ecosystem. As applications become increasingly AI-driven, the distinction between software development and AI model development is blurring. This convergence needs a more collaborative approach, where traditional developers gain AI literacy and data scientists take on more active roles in application development. The evolution of these roles highlights the interdisciplinary nature of building modern AI-embedded applications and underscores the importance of continuous learning and adaptation in the rapidly advancing field of AI.
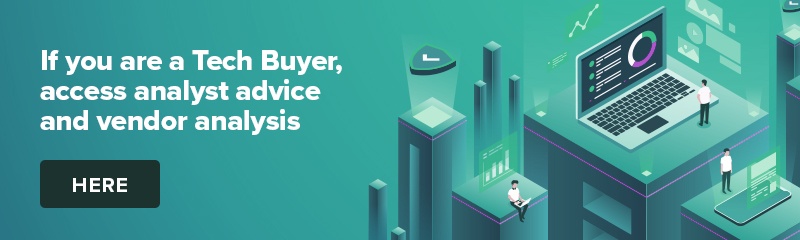
Healthcare delivery and healthtech have made significant strides; yet, the fundamental challenges in healthcare have remained largely unchanged for decades. The widespread acceptance and integration of digital solutions in recent years have supported healthcare providers’ primary goals of enhancing operational efficiency, better resource utilisation (with addressing skill shortages being a key driver), improving patient experience, and achieving better clinical outcomes. With governments pushing for advancements in healthcare outcomes at sustainable costs, the concept of value-based healthcare has gained traction across the industry.
Technology-driven Disruption
Healthcare saw significant disruptions four years ago, and while we will continue to feel the impact for the next decade, one positive outcome was witnessing the industry’s ability to transform amid such immense pressure. I am definitely not suggesting another healthcare calamity! But disruptions can have a positive impact – and I believe that technology will continue to disrupt healthcare at pace. Recently, my colleague Tim Sheedy shared his thoughts on how 2024 is poised to become the year of the AI startup, highlighting innovative options that organisations should consider in their AI journeys. AI startups and innovators hold the potential to further the “good disruption” that will transform healthcare.
Of course, there are challenges associated, including concerns on ethical and privacy-related issues, the reliability of technology – particularly while scaling – and on professional liability. However, the industry cannot overlook the substantial number of innovative startups that are using AI technologies to address some of the most pressing challenges in the healthcare industry.
Why Now?
AI is not new to healthcare. Many would cite the development of MYCIN – an early AI program aimed at identifying treatments for blood infections – as the first known example. It did kindle interest in research in AI and even during the 1980s and 1990s, AI brought about early healthcare breakthroughs, including faster data collection and processing, enhanced precision in surgical procedures, and research and mapping of diseases.
Now, healthcare is at an AI inflection point due to a convergence of three significant factors.
- Advanced AI. AI algorithms and capabilities have become more sophisticated, enabling them to handle complex healthcare data and tasks with greater accuracy and efficiency.
- Demand for Accessible Healthcare. Healthcare systems globally are striving for better care amid resource constraints, turning to AI for efficiency, cost reduction, and broader access.
- Consumer Demand. As people seek greater control over their health, personalised care has become essential. AI can analyse vast patient data to identify health risks and customise care plans, promoting preventative healthcare.
Promising Health AI Startups
As innovative startups continue to emerge in healthcare, we’re particularly keeping an eye on those poised to revolutionise diagnostics, care delivery, and wellness management. Here are some examples.
DIAGNOSTICS
- Claritas HealthTech has created advanced image enhancement software to address challenges in interpreting unclear medical images, improving image clarity and precision. A cloud-based platform with AI diagnostic tools uses their image enhancement technology to achieve greater predictive accuracy.
- Ibex offers Galen, a clinical-grade, multi-tissue platform to detect and grade cancers, that integrate with third-party digital pathology software solutions, scanning platforms, and laboratory information systems.
- MEDICAL IP is focused on advancing medical imaging analysis through AI and 3D technologies (such as 3D printing, CAD/CAM, AR/VR) to streamline medical processes, minimising time and costs while enhancing patient comfort.
- Verge Genomics is a biopharmaceutical startup employing systems biology to expedite the development of life-saving treatments for neurodegenerative diseases. By leveraging patient genomes, gene expression, and epigenomics, the platform identifies new therapeutic gene targets, forecasts effective medications, and categorises patient groups for enhanced clinical efficacy.
- X-Zell focuses on advanced cytology, diagnosing diseases through single atypical cells or clusters. Their plug-and-play solution detects, visualises, and digitises these phenomena in minimally invasive body fluids. With no complex specimen preparation required, it slashes the average sample-to-diagnosis time from 48 hours to under 4 hours.
CARE DELIVERY
- Abridge specialises in automating clinical notes and medical discussions for physicians, converting patient-clinician conversations into structured clinical notes in real time, powered by GenAI. It integrates seamlessly with EMRs such as Epic.
- Waltz Health offers AI-driven marketplaces aimed at reducing costs and innovative consumer tools to facilitate informed care decisions. Tailored for payers, pharmacies, and consumers, they introduce a fresh approach to pricing and reimbursing prescriptions that allows consumers to purchase medication at the most competitive rates, improving accessibility.
- Acorai offers a non-invasive intracardiac pressure monitoring device for heart failure management, aimed at reducing hospitalisations and readmissions. The technology can analyse acoustics, vibratory, and waveform data using ML to monitor intracardiac pressures.
WELLNESS MANAGEMENT
- Anya offers AI-driven support for women navigating life stages such as fertility, pregnancy, parenthood, and menopause. For eg. it provides support during the critical first 1,001 days of the parental journey, with personalised advice, tracking of developmental milestones, and connections with healthcare professionals.
- Dacadoo’s digital health engagement platform aims to motivate users to adopt healthier lifestyles through gamification, social connectivity, and personalised feedback. By analysing user health data, AI algorithms provide tailored insights, goal-setting suggestions, and challenges.
Conclusion
There is no question that innovative startups can solve many challenges for the healthcare industry. But startups flourish because of a supportive ecosystem. The health innovation ecosystem needs to be a dynamic network of stakeholders committed to transforming the industry and health outcomes – and this includes healthcare providers, researchers, tech companies, startups, policymakers, and patients. Together we can achieve the longstanding promise of accessible, cost-effective, and patient-centric healthcare.
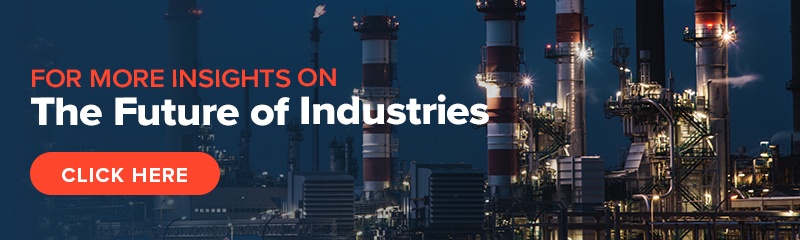
Banks, insurers, and other financial services organisations in Asia Pacific have plenty of tech challenges and opportunities including cybersecurity and data privacy management; adapting to tech and customer demands, AI and ML integration; use of big data for personalisation; and regulatory compliance across business functions and transformation journeys.
Modernisation Projects are Back on the Table
An emerging tech challenge lies in modernising, replacing, or retiring legacy platforms and systems. Many banks still rely on outdated core systems, hindering agility, innovation, and personalised customer experiences. Migrating to modern, cloud-based systems presents challenges due to complexity, cost, and potential disruptions. Insurers are evaluating key platforms amid evolving customer needs and business models; ERP and HCM systems are up for renewal; data warehouses are transforming for the AI era; even CRM and other CX platforms are being modernised as older customer data stores and models become obsolete.
For the past five years, many financial services organisations in the region have sidelined large legacy modernisation projects, opting instead to make incremental transformations around their core systems. However, it is becoming critical for them to take action to secure their long-term survival and success.
Benefits of legacy modernisation include:
- Improved operational efficiency and agility
- Enhanced customer experience and satisfaction
- Increased innovation and competitive advantage
- Reduced security risks and compliance costs
- Preparation for future technologies
However, legacy modernisation and migration initiatives carry significant risks. For instance, TSB faced a USD 62M fine due to a failed mainframe migration, resulting in severe disruptions to branch operations and core banking functions like telephone, online, and mobile banking. The migration failure led to 225,492 complaints between 2018 and 2019, affecting all 550 branches and required TSB to pay more than USD 25M to customers through a redress program.
Modernisation Options
- Rip and Replace. Replacing the entire legacy system with a modern, cloud-based solution. While offering a clean slate and faster time to value, it’s expensive, disruptive, and carries migration risks.
- Refactoring. Rewriting key components of the legacy system with modern languages and architectures. It’s less disruptive than rip-and-replace but requires skilled developers and can still be time-consuming.
- Encapsulation. Wrapping the legacy system with a modern API layer, allowing integration with newer applications and tools. It’s quicker and cheaper than other options but doesn’t fully address underlying limitations.
- Microservices-based Modernisation. Breaking down the legacy system into smaller, independent services that can be individually modernised over time. It offers flexibility and agility but requires careful planning and execution.
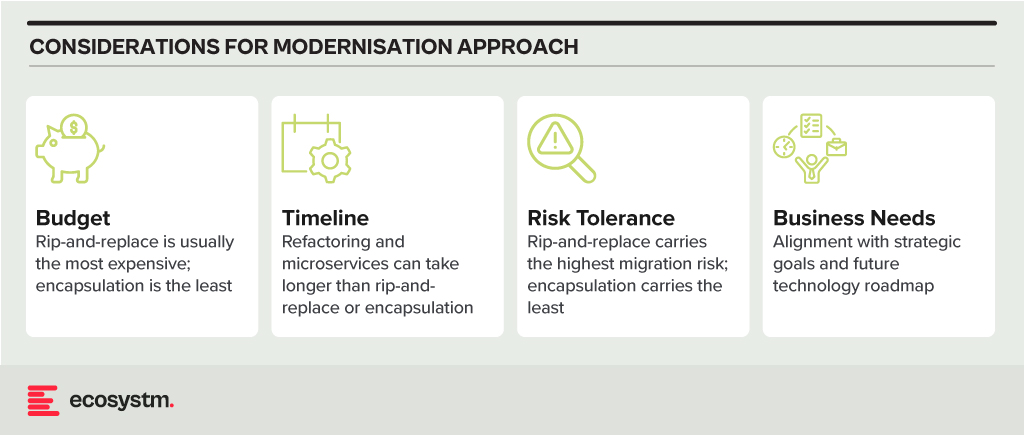
Financial Systems on the Block for Legacy Modernisation
Data Analytics Platforms. Harnessing customer data for insights and targeted offerings is vital. Legacy data warehouses often struggle with real-time data processing and advanced analytics.
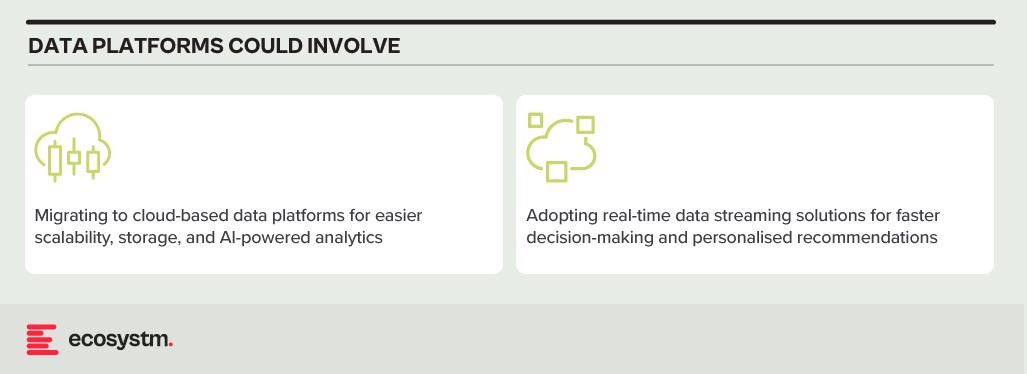
CRM Systems. Effective customer interactions require integrated CRM platforms. Outdated systems might hinder communication, personalisation, and cross-selling opportunities.
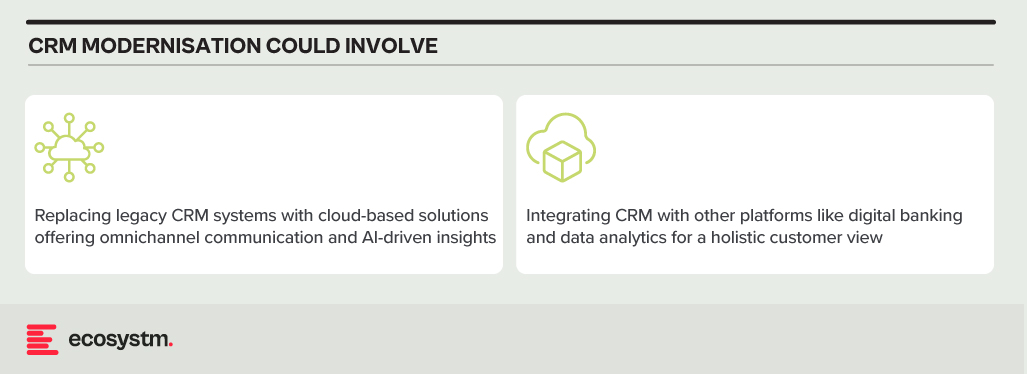
Payment Processing Systems. Legacy systems might lack support for real-time secure transactions, mobile payments, and cross-border transactions.
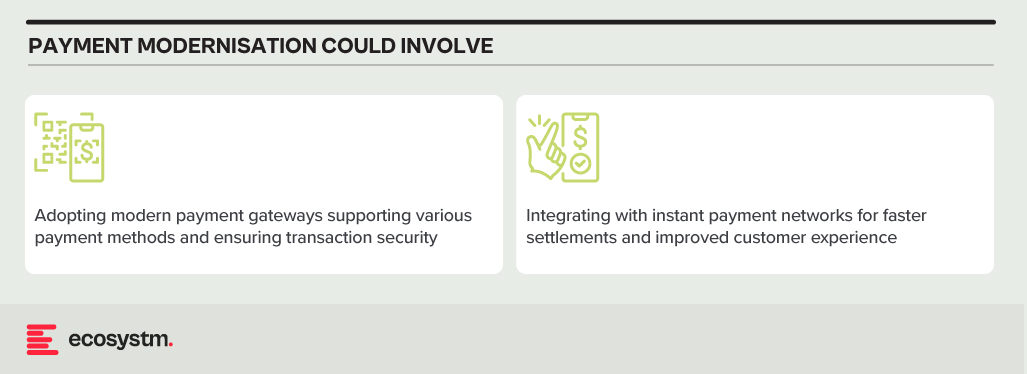
Core Banking Systems (CBS). The central nervous system of any bank, handling account management, transactions, and loan processing. Many Asia Pacific banks rely on aging, monolithic CBS with limited digital capabilities.
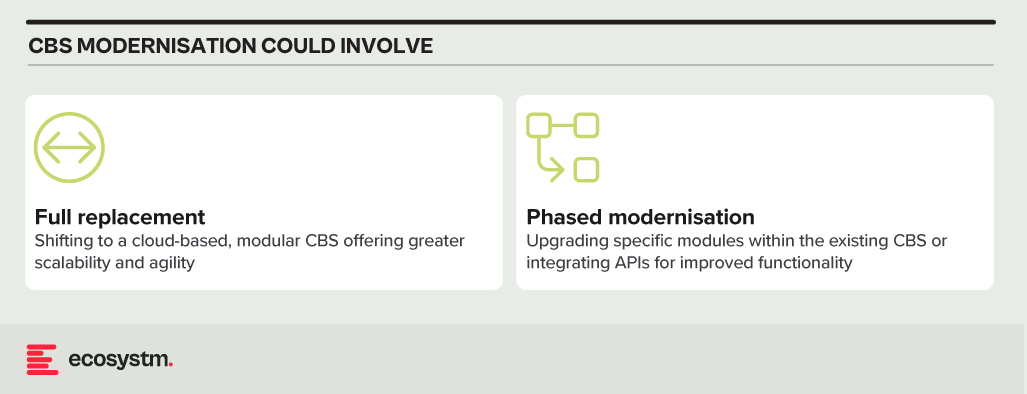
Digital Banking Platforms. While several Asia Pacific banks provide basic online banking, genuine digital transformation requires mobile-first apps with features such as instant payments, personalised financial management tools, and seamless third-party service integration.
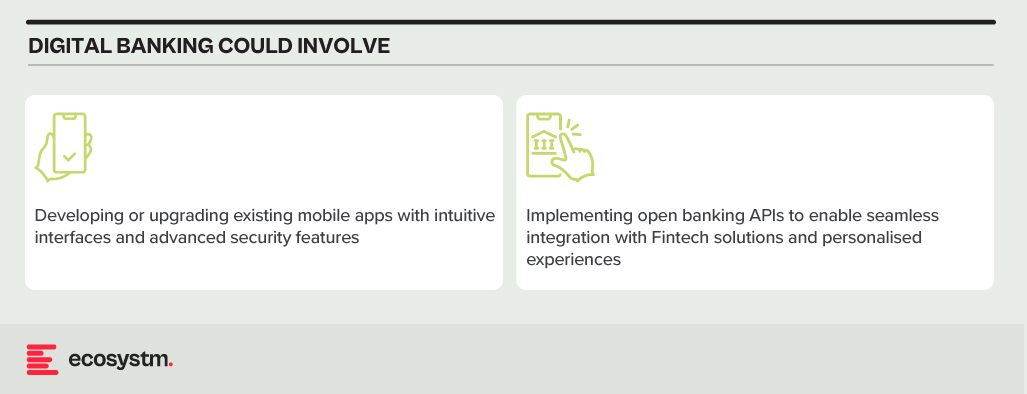
Modernising Technical Approaches and Architectures
Numerous technical factors need to be addressed during modernisation, with decisions needing to be made upfront. Questions around data migration, testing and QA, change management, data security and development methodology (agile, waterfall or hybrid) need consideration.
Best practices in legacy migration have taught some lessons.
Adopt a data fabric platform. Many organisations find that centralising all data into a single warehouse or platform rarely justifies the time and effort invested. Businesses continually generate new data, adding sources, and updating systems. Managing data where it resides might seem complex initially. However, in the mid to longer term, this approach offers clearer benefits as it reduces the likelihood of data discrepancies, obsolescence, and governance challenges.
Focus modernisation on the customer metrics and journeys that matter. Legacy modernisation need not be an all-or-nothing initiative. While systems like mainframes may require complete replacement, even some mainframe-based software can be partially modernised to enable services for external applications and processes. Assess the potential of modernising components of existing systems rather than opting for a complete overhaul of legacy applications.
Embrace the cloud and SaaS. With the growing network of hyperscaler cloud locations and data centres, there’s likely to be a solution that enables organisations to operate in the cloud while meeting data residency requirements. Even if not available now, it could align with the timeline of a multi-year legacy modernisation project. Whenever feasible, prioritise SaaS over cloud-hosted applications to streamline management, reduce overhead, and mitigate risk.
Build for customisation for local and regional needs. Many legacy applications are highly customised, leading to inflexibility, high management costs, and complexity in integration. Today, software providers advocate minimising configuration and customisation, opting for “out-of-the-box” solutions with room for localisation. The operations in different countries may require reconfiguration due to varying regulations and competitive pressures. Architecting applications to isolate these configurations simplifies system management, facilitating continuous improvement as new services are introduced by platform providers or ISV partners.
Explore the opportunity for emerging technologies. Emerging technologies, notably AI, can significantly enhance the speed and value of new systems. In the near future, AI will automate much of the work in data migration and systems integration, reducing the need for human involvement. When humans are required, low-code or no-code tools can expedite development. Private 5G services may eliminate the need for new network builds in branches or offices. AIOps and Observability can improve system uptime at lower costs. Considering these capabilities in platform decisions and understanding the ecosystem of partners and providers can accelerate modernisation journeys and deliver value faster.
Don’t Let Analysis Paralysis Slow Down Your Journey!
Yes, there are a lot of decisions that need to be made; and yes, there is much at stake if things go wrong! However, there’s a greater risk in not taking action. Maintaining a laser-focus on the customer and business outcomes that need to be achieved will help align many decisions. Keeping the customer experience as the guiding light ensures organisations are always moving in the right direction.
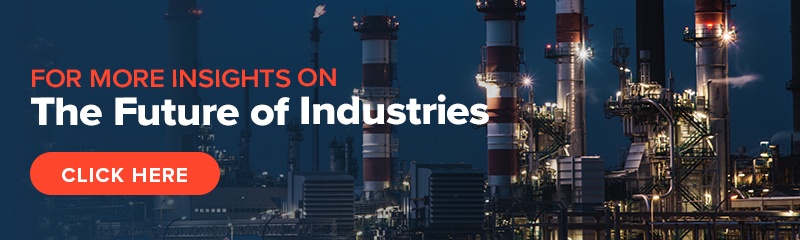
2024 and 2025 are looking good for IT services providers – particularly in Asia Pacific. All types of providers – from IT consultants to managed services VARs and systems integrators – will benefit from a few converging events.
However, amidst increasing demand, service providers are also challenged with cost control measures imposed in organisations – and this is heightened by the challenge of finding and retaining their best people as competition for skills intensifies. Providers that service mid-market clients might find it hard to compete and grow without significant process automation to compensate for the higher employee costs.
Why Organisations are Opting for IT Service
- Organisations are seeking further cost reductions. Managed services providers will see more opportunities to take cost and complexity out of organisation’s IT functions. The focus in 2024 will be less on “managing” services and more on “transforming” them using ML, AI, and automation to reduce cost and improve value.
- Big app upgrades are back on the agenda. SAP is going above and beyond to incentivise their customers and partners to migrate their on-premises and hyperscale hosted instances to true cloud ERP. Initiatives such as Rise with SAP have been further expanded and improved to accelerate the transition. Salesforce customers are also looking to streamline their deployments while also taking advantage of the new AI and data capabilities. But many of these projects will still be complex and time-consuming.
- Cloud deployments are getting more complex. For many organisations, the simple cloud migrations are done. This is the stage of replatforming, retiring, and refactoring applications to take advantage of public and hybrid cloud capabilities. These are not simple lift and shift – or switch to SaaS – engagements.
- AI will drive a greater need for process improvement and transformation. This will happen along with associated change management and training programs. While it is still early days for GenAI, before the end of 2024, many organisations will move beyond experimentation to department or enterprise wide GenAI initiatives.
- Increasing cybersecurity and data governance demands will prolong the security skill shortage. More organisations will turn to managed security services providers and cybersecurity consultants to help them develop their strategy and response to the rising threat levels.
Choosing the Right Cost Model for IT Services
Buyers of IT services must implement strict cost-control measures and consider various approaches to align costs with business and customer outcomes, including different cost models:
Fixed-Price Contracts. These contracts set a firm price for the entire project or specific deliverables. Ideal when project scope is clear, they offer budget certainty upfront but demand detailed specifications, potentially leading to higher initial quotes due to the provider assuming more risk.
Time and Materials (T&M) Contracts with Caps. Payment is based on actual time and materials used, with negotiated caps to prevent budget overruns. Combining flexibility with cost predictability, this model offers some control over total expenses.
Performance-Based Pricing. Fees are tied to service provider performance, incentivising achievement of specific KPIs or milestones. This aligns provider interests with client goals, potentially resulting in cost savings and improved service quality.
Retainer Agreements with Scope Limits. Recurring fees are paid for ongoing services, with defined limits on work scope or hours within a given period. This arrangement ensures resource availability while containing expenses, particularly suitable for ongoing support services.
Other Strategies for Cost Efficiency and Effective Management
Technology leaders should also consider implementing some of the following strategies:
Phased Payments. Structuring payments in phases, tied to the completion of project milestones, helps manage cash flow and provides a financial incentive for the service provider to meet deadlines and deliverables. It also allows for regular financial reviews and adjustments if the project scope changes.
Cost Transparency and Itemisation. Detailed billing that itemises the costs of labour, materials, and other expenses provides transparency to verify charges, track spending against the budget, and identify areas for potential savings.
Volume Discounts and Negotiated Rates. Negotiating volume discounts or preferential rates for long-term or large-scale engagements, makes providers to offer reduced rates for a commitment to a certain volume of work or an extended contract duration.
Utilisation of Shared Services or Cloud Solutions. Opting for shared or cloud-based solutions where feasible, offers economies of scale and reduces the need for expensive, dedicated infrastructure and resources.
Regular Review and Adjustment. Conducting regular reviews of the services and expenses with the provider to ensure alignment with the budget and objectives, prepares organisations to adjust the scope, renegotiate terms, or implement cost-saving measures as needed.
Exit Strategy. Planning an exit strategy that include provisions for contract termination, transition services, protects an organisation in case the partnership needs to be dissolved.
Conclusion
Many businesses swing between insourcing and outsourcing technology capabilities – with the recent trend moving towards insourcing development and outsourcing infrastructure to the public cloud. But 2024 will see demand for all types of IT services across nearly every geography and industry. Tech services providers can bring significant value to your business – but improved management, monitoring, and governance will ensure that this value is delivered at a fair cost.
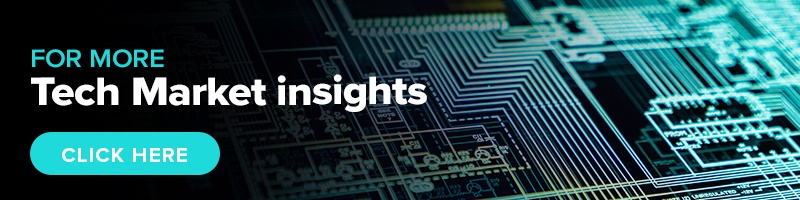
In 2024, business and technology leaders will leverage the opportunity presented by the attention being received by Generative AI engines to test and integrate AI comprehensively across the business. Many organisations will prioritise the alignment of their initial Generative AI initiatives with broader AI strategies, establishing distinct short-term and long-term goals for their AI investments.
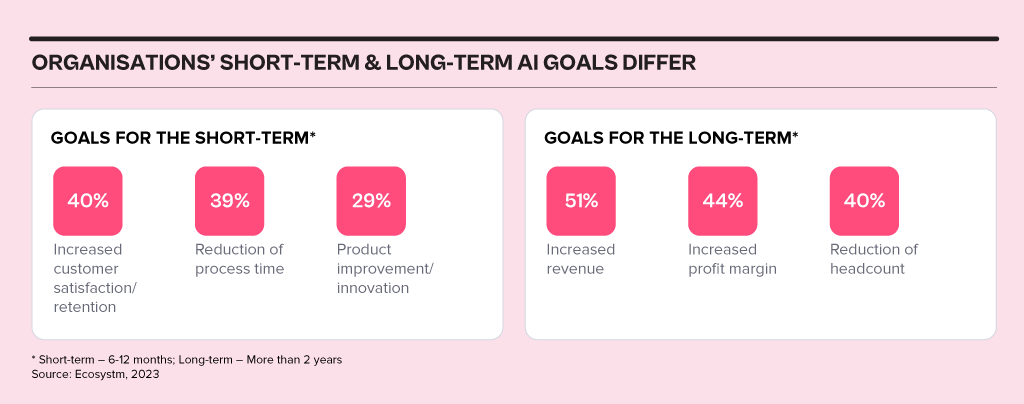
AI adoption will influence business processes, technology skills, and, in turn, reshape the product/service offerings of AI providers.
Ecosystm analysts Achim Granzen, Peter Carr, Richard Wilkins, Tim Sheedy, and Ullrich Loeffler present the top 5 AI trends in 2024.
Click here to download ‘Ecosystm Predicts: Top 5 AI Trends in 2024.
#1 By the End of 2024, Gen AI Will Become a ‘Hygiene Factor’ for Tech Providers
AI has widely been commended as the ‘game changer’ that will create and extend the divide between adopters and laggards and be the deciding factor for success and failure.
Cutting through the hype, strategic adoption of AI is still at a nascent stage and 2024 will be another year where companies identify use cases, experiment with POCs, and commit renewed efforts to get their data assets in order.
The biggest impact of AI will be derived from integrated AI capability in standard packaged software and products – and this will include Generative AI. We will see a plethora of product releases that seamlessly weave Generative AI into everyday tools generating new value through increased efficiency and user-friendliness.
Technology will be the first industry where AI becomes the deciding factor between success and failure; tech providers will be forced to deliver on their AI promises or be left behind.
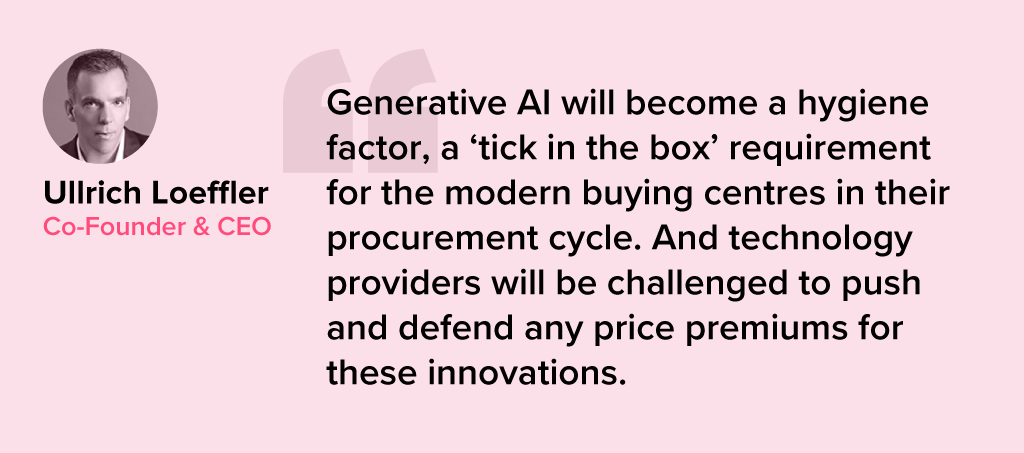
#2 Gen AI Will Disrupt the Role of IT Architects
Traditionally, IT has relied on three-tier architectures for applications, that faced limitations in scalability and real-time responsiveness. The emergence of microservices, containerisation, and serverless computing has paved the way for event-driven designs, a paradigm shift that decouples components and use events like user actions or data updates as triggers for actions across distributed services. This approach enhances agility, scalability, and flexibility in the system.
The shift towards event-driven designs and advanced architectural patterns presents a compelling challenge for IT Architects, as traditionally their role revolved around designing, planning and overseeing complex systems.
Generative AI is progressively demonstrating capabilities in architectural design through pattern recognition, predictive analytics, and automated decision-making.
With the adoption of Generative AI, the role of an IT Architect will change into a symbiotic relationship where human expertise collaborates with AI insights.
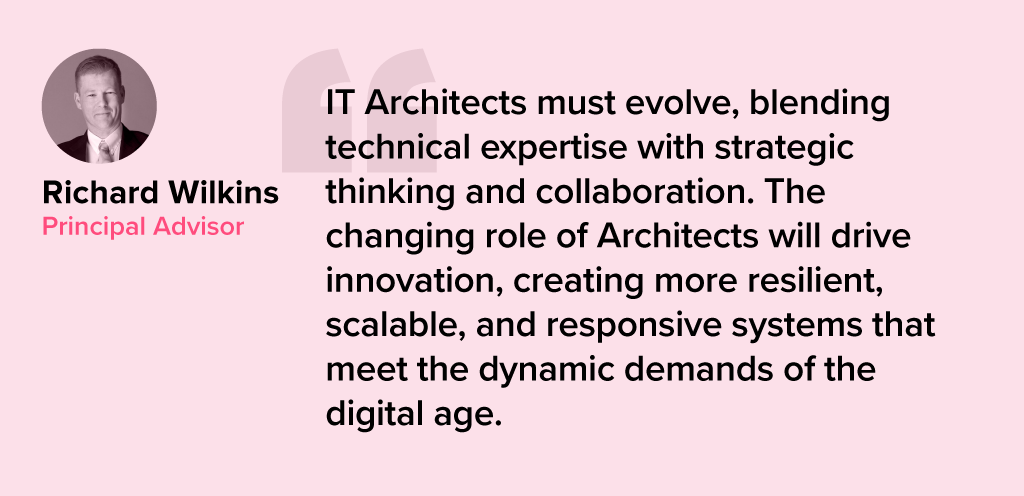
#3 Gen AI Adoption Will be Confined to Specific Use Cases
A little over a year ago, a new era in AI began with the initial release of OpenAI’s ChatGPT. Since then, many organisations have launched Generative AI pilots.
In its second-year enterprises will start adoption – but in strictly defined and limited use cases. Examples such as Microsoft Copilot demonstrate an early adopter route. While productivity increases for individuals can be significant, its enterprise impact is unclear (at this time).
But there are impactful use cases in enterprise knowledge and document management. Organisations across industries have decades (or even a century) of information, including digitised documents and staff expertise. That treasure trove of information can be made accessible through cognitive search and semantic answering, driven by Generative AI.
Generative AI will provide organisations with a way to access, distill, and create value out of that data – a task that may well be impossible to achieve in any other way.
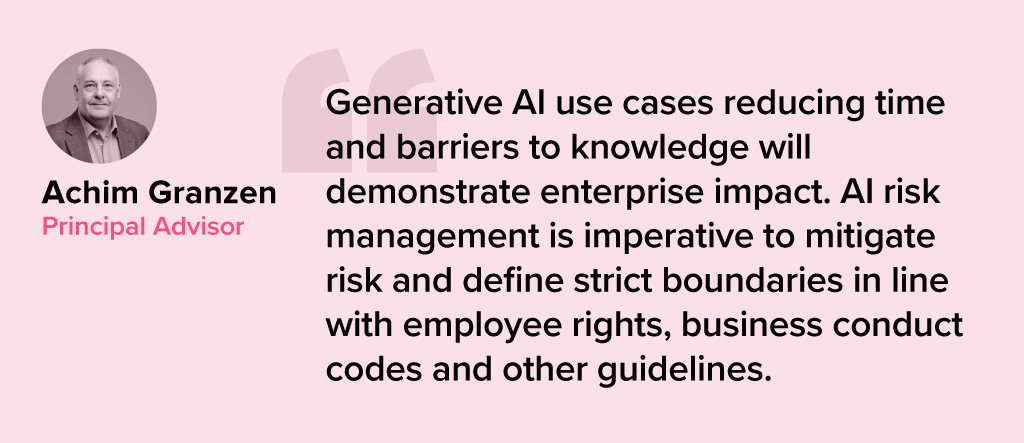
#4 Gen AI Will Get Press Inches; ‘Traditional’ AI Will Do the Hard Work
While the use cases for Generative AI will continue to expand, the deployment models and architectures for enterprise Generative AI do not add up – yet.
Running Generative AI in organisations’ data centres is costly and using public models for all but the most obvious use cases is too risky. Most organisations opt for a “small target” strategy, implementing Generative AI in isolated use cases within specific processes, teams, or functions. Justifying investment in hardware, software, and services for an internal AI platform is challenging when the payback for each AI initiative is not substantial.
“Traditional AI/ML” will remain the workhorse, with a significant rise in use cases and deployments. Organisations are used to investing for AI by individual use cases. Managing process change and training is also more straightforward with traditional AI, as the changes are implemented in a system or platform, eliminating the need to retrain multiple knowledge workers.
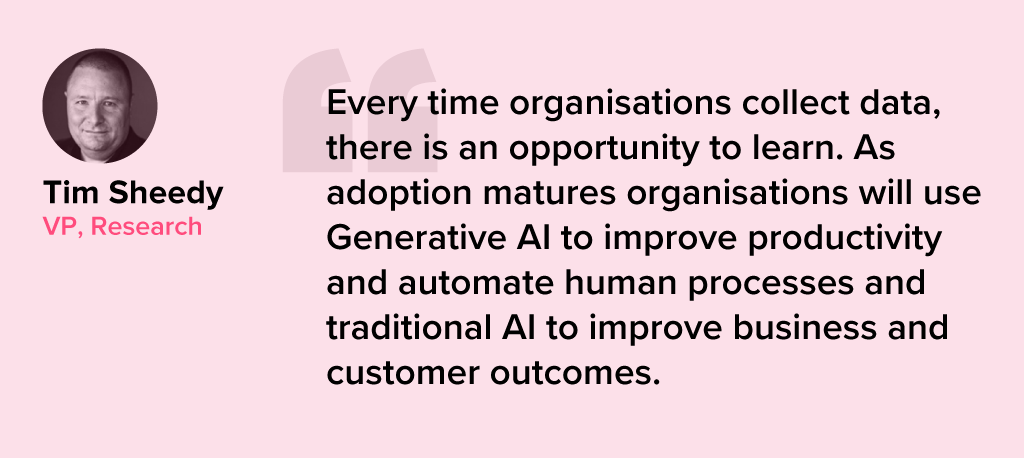
#5 AI Will Pioneer a 21st Century BPM Renaissance
As we near the 25-year milestone of the 21st century, it becomes clear that many businesses are still operating with 20th-century practices and philosophies.
AI, however, represents more than a technological breakthrough; it offers a new perspective on how businesses operate and is akin to a modern interpretation of Business Process Management (BPM). This development carries substantial consequences for digital transformation strategies. To fully exploit the potential of AI, organisations need to commit to an extensive and ongoing process spanning the collection, organisation, and expansion of data, to integrating these insights at an application and workflow level.
The role of AI will transcend technological innovation, becoming a driving force for substantial business transformation. Sectors that specialise in workflow, data management, and organisational transformation are poised to see the most growth in 2024 because of this shift.
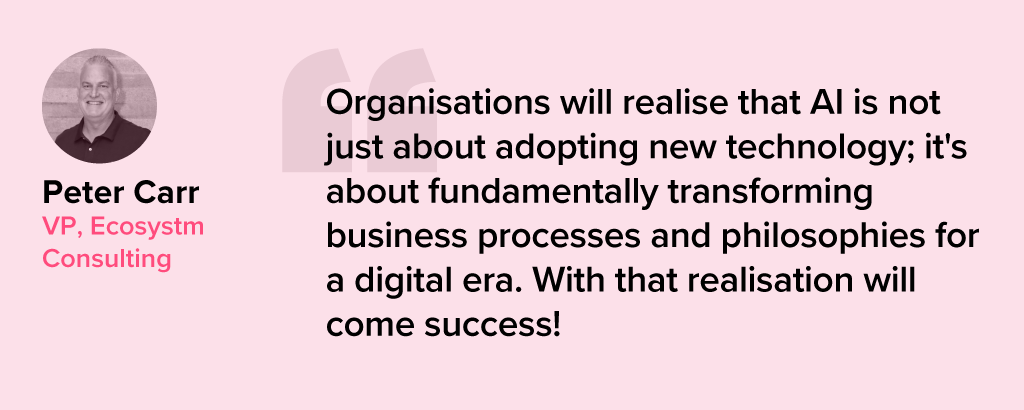
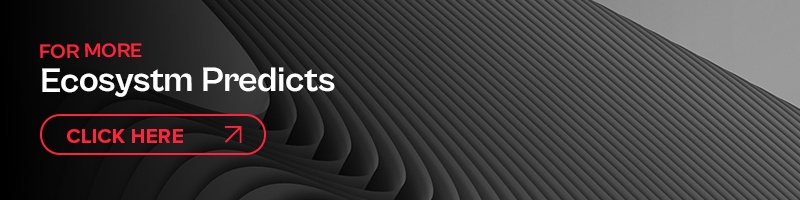
Ecosystm research reveals a stark reality: 75% of technology leaders in Financial Services anticipate data breaches.
Given the sector’s regulatory environment, data breaches carry substantial financial implications, emphasising the critical importance of giving precedence to cybersecurity. This is compelling a fresh cyber strategy focused on early threat detection and reduction of attack impact.
Read on to find out how tech leaders are building a culture of cyber-resilience, re-evaluating their cyber policies, and adopting technologies that keep them one step ahead of their adversaries.
Download ‘Cyber-Resilience in Finance: People, Policy & Technology’ as a PDF
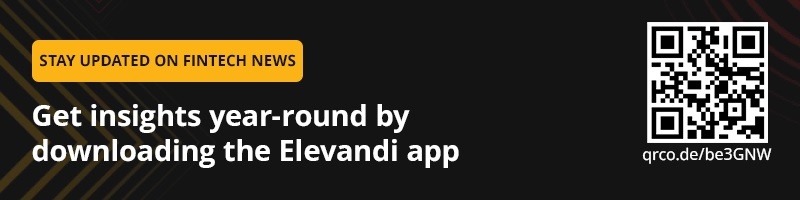
As organisations demand more industry-ready and localised services, global technology companies feel an active need to re-evaluate their capabilities and bridge gaps through partnerships and acquisitions. Ecosystm has been helping global technology companies evolve their partner strategies to remain relevant to the Asia Pacific market.
Our analysis of the tech acquisition landscape in Asia Pacific over the last three years shows that:
- While global tech providers are expanding their presence in Australia and Singapore, emerging markets in Southeast Asia are receiving a fair share of attention. 17% of tech acquisitions by global tech companies between 2020-22 have been in these markets.
- A quarter of the acquisitions were for product and platform capabilities. The rest were all done to bolster services portfolios – such as managed technology, business process, implementation & integration and consulting services.
- A large percentage of the acquisitions are to bolster industry capabilities through transformative technologies such as AI/ML, cloud and cybersecurity.
Here is the analysis of more than 100 acquisitions made by large global tech companies in Asia Pacific between 2020-2022.
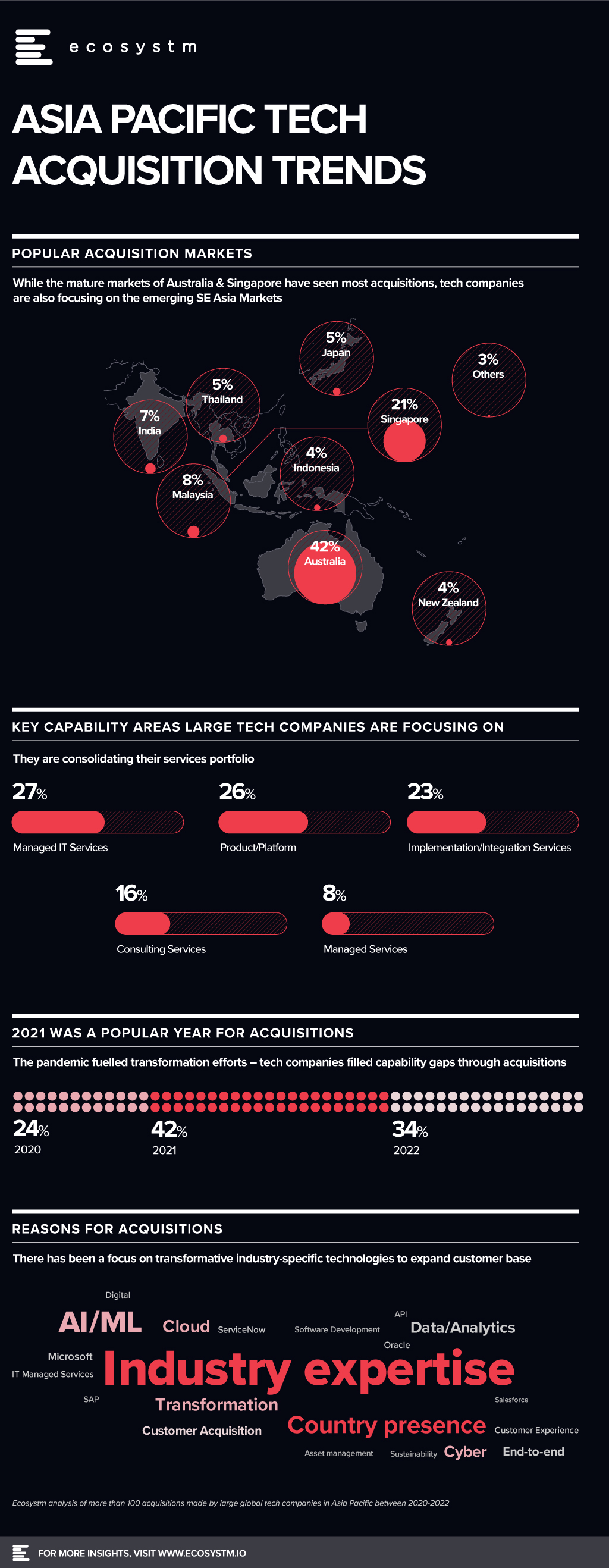
Download ‘Asia Pacific Tech Acquisition Trends’ as a PDF