The tech industry is experiencing a strategic convergence of AI, data management, and cybersecurity, driving a surge in major M&A activity. As enterprises tackle digital transformation, these three pillars are at the forefront, accelerating the race to acquire and integrate critical technologies.
Here are this year’s key consolidation moves, showcasing how leading tech companies are positioning themselves to capitalise on the rising demand for AI-driven solutions, robust data infrastructure, and enhanced cybersecurity.
AI Convergence: Architecting the Intelligent Enterprise
From customer service to supply chain management, AI is being deployed across the entire enterprise value chain. This widespread demand for AI solutions is creating a dynamic M&A market, with tech companies acquiring specialised AI capabilities.
IBM’s AI Power Play
IBM’s acquisitions of HashiCorp and DataStax mark a decisive step in its push to lead enterprise AI and hybrid cloud. The USD 6.4B HashiCorp deal that got finalised this year, brings Terraform, a top-tier infrastructure-as-code tool that streamlines multi-cloud deployments – key to integrating IBM’s Red Hat OpenShift and Watsonx AI. Embedding Terraform enhances automation, making hybrid cloud infrastructure more efficient and AI-ready.
The DataStax acquisition strengthens IBM’s AI data strategy. With AstraDB and Apache Cassandra, IBM gains scalable NoSQL solutions for AI workloads, while Langflow simplifies AI app development. Together, these moves position IBM as an end-to-end AI and cloud powerhouse, offering enterprises seamless automation, data management, and AI deployment at scale.
MongoDB’s RAG Focus
MongoDB’s USD 220M acquisition of Voyage AI signals a strategic push toward enhancing AI reliability. At the core of this move is retrieval-augmented generation (RAG), a technology that curbs AI hallucinations by grounding responses in accurate, relevant data.
By integrating Voyage AI into its Atlas cloud database, MongoDB is making AI applications more trustworthy and reducing the complexity of RAG implementations. Enterprises can now build AI-driven solutions directly within their database, streamlining development while improving accuracy. This move consolidates MongoDB’s role as a key player in enterprise AI, offering both scalable data management and built-in AI reliability.
Google’s 1B Bet on Anthropic
Google’s continued investment in Anthropic reinforces its commitment to foundation model innovation and the evolving GenAI landscape. More than a financial move, this signals Google’s intent to shape the future of AI by backing one of the field’s most promising players.
This investment aligns with a growing trend among cloud giants securing stakes in foundation model developers to drive AI advancements. By deepening ties with Anthropic, Google not only gains access to cutting-edge AI research but also strengthens its position in developing safe, scalable, and enterprise-ready AI. This solidifies Google’s long-term AI strategy, ensuring its leadership in GenAI while seamlessly integrating these capabilities into its cloud ecosystem.
ServiceNow’s AI Automation Expansion
ServiceNow’s USD 2.9B acquisition of Moveworks completed this year, marking a decisive push into AI-driven service desk automation. This goes beyond feature expansion – it redefines enterprise support operations by embedding intelligent automation into workflows, reducing resolution times, and enhancing employee productivity.
The acquisition reflects a growing shift: AI-powered service management is no longer optional but essential. Moveworks’ AI-driven capabilities – natural language understanding, machine learning, and automated issue resolution – will enable ServiceNow to deliver a smarter, more proactive support experience. Additionally, gaining Moveworks’ customer base strengthens ServiceNow’s market reach.
Data Acquisition Surge: Fuelling Digital Transformation
Data has transcended its role as a byproduct of operations, becoming the lifeblood that fuels digital transformation. This fundamental shift has triggered a surge in strategic acquisitions focused on enhancing data management and storage capabilities.
Lenovo Scaling Enterprise Storage
Lenovo’s USD 2B acquisition of Infinidat strengthens its position in enterprise storage as data demands surge. Infinidat’s AI-driven InfiniBox delivers high-performance, low-latency storage for AI, analytics, and HPC, while InfiniGuard ensures advanced data protection.
By integrating these technologies, Lenovo expands its hybrid cloud offerings, challenging Dell and NetApp while reinforcing its vision as a full-stack data infrastructure provider.
Databricks Streamlining Data Warehouse Migrations
Databricks’ USD 15B acquisition of BladeBridge accelerates data warehouse migrations with AI-driven automation, reducing manual effort and errors in migrating legacy platforms like Snowflake and Teradata. BladeBridge’s technology enhances Databricks’ SQL platform, simplifying the transition to modern data ecosystems.
This strengthens Databricks’ Data Intelligence Platform, boosting its appeal by enabling faster, more efficient enterprise data consolidation and supporting rapid adoption of data-driven initiatives.
Cybersecurity Consolidation: Fortifying the Digital Fortress
The escalating sophistication of cyber threats has transformed cybersecurity from a reactive measure to a strategic imperative. This has fuelled a surge in M&A aimed at building comprehensive and integrated security solutions.
Turn/River Capital’s Security Acquisition
Turn/River Capital’s USD 4.4 billion acquisition of SolarWinds underscores the enduring demand for robust IT service management and security software. This acquisition is a testament to the essential role SolarWinds plays in enterprise IT infrastructure, even in the face of past security breaches.
This is a bold investment, in the face of prior vulnerability and highlights a fundamental truth: the need for reliable security solutions outweighs even the most public of past failings. Investors are willing to make long term bets on companies that provide core security services.
Sophos Expanding Managed Detection & Response Capabilities
Sophos completed the acquisition of Secureworks for USD 859M significantly strengthens its managed detection and response (MDR) capabilities, positioning Sophos as a major player in the MDR market. This consolidation reflects the growing demand for comprehensive cybersecurity solutions that offer proactive threat detection and rapid incident response.
By integrating Secureworks’ XDR products, Sophos enhances its ability to provide end-to-end protection for its customers, addressing the evolving threat landscape with advanced security technologies.
Cisco’s Security Portfolio Expansion
Cisco completed the USD 28B acquisition of SnapAttack further expanding its security business, building upon its previous acquisition of Splunk. This move signifies Cisco’s commitment to creating a comprehensive security portfolio that can address the diverse needs of its enterprise customers.
By integrating SnapAttack’s threat detection capabilities, Cisco strengthens its ability to provide proactive threat intelligence and incident response, solidifying its position as a leading provider of security solutions.
Google’s Cloud Security Reinforcement
Google’s strategic acquisition of Wiz, a leading cloud security company, for USD 32B demonstrates its commitment to securing cloud-native environments. Wiz’s expertise in proactive threat detection and remediation will significantly enhance Google Cloud’s security offerings. This move is particularly crucial as organisations increasingly migrate their workloads to the cloud.
By integrating Wiz’s capabilities, Google aims to provide its customers with a robust security framework that can protect their cloud-based assets from sophisticated cyber threats. This acquisition positions Google as a stronger competitor in the cloud security market, reinforcing its commitment to enterprise-grade cybersecurity.
The Way Ahead
The M&A trends of 2025 underscore the critical role of AI, data, and security in shaping the technology landscape. Companies that prioritise these core areas will be best positioned for long-term success. Strategic acquisitions, when executed with foresight and agility, will serve as essential catalysts for navigating the complexities of the evolving digital world.
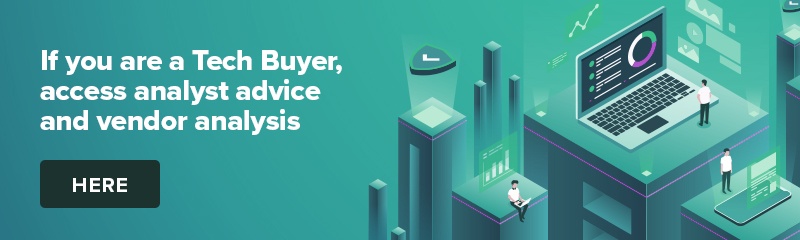
A lot has been written and spoken about DeepSeek since the release of their R1 model in January. Soon after, Alibaba, Mistral AI, and Ai2 released their own updated models, and we have seen Manus AI being touted as the next big thing to follow.
DeepSeek’s lower-cost approach to creating its model – using reinforcement learning, the mixture-of-experts architecture, multi-token prediction, group relative policy optimisation, and other innovations – has driven down the cost of LLM development. These methods are likely to be adopted by other models and are already being used today.
While the cost of AI is a challenge, it’s not the biggest for most organisations. In fact, few GenAI initiatives fail solely due to cost.
The reality is that many hurdles still stand in the way of organisations’ GenAI initiatives, which need to be addressed before even considering the business case – and the cost – of the GenAI model.
Real Barriers to GenAI
• Data. The lifeblood of any AI model is the data it’s fed. Clean, well-managed data yields great results, while dirty, incomplete data leads to poor outcomes. Even with RAG, the quality of input data dictates the quality of results. Many organisations I work with are still discovering what data they have – let alone cleaning and classifying it. Only a handful in Australia can confidently say their data is fully managed, governed, and AI-ready. This doesn’t mean GenAI initiatives must wait for perfect data, but it does explain why Agentic AI is set to boom – focusing on single applications and defined datasets.
• Infrastructure. Not every business can or will move data to the public cloud – many still require on-premises infrastructure optimised for AI. Some companies are building their own environments, but this often adds significant complexity. To address this, system manufacturers are offering easy-to-manage, pre-built private cloud AI solutions that reduce the effort of in-house AI infrastructure development. However, adoption will take time, and some solutions will need to be scaled down in cost and capacity to be viable for smaller enterprises in Asia Pacific.
• Process Change. AI algorithms are designed to improve business outcomes – whether by increasing profitability, reducing customer churn, streamlining processes, cutting costs, or enhancing insights. However, once an algorithm is implemented, changes will be required. These can range from minor contact centre adjustments to major warehouse overhauls. Change is challenging – especially when pre-coded ERP or CRM processes need modification, which can take years. Companies like ServiceNow and SS&C Blue Prism are simplifying AI-driven process changes, but these updates still require documentation and training.
• AI Skills. While IT teams are actively upskilling in data, analytics, development, security, and governance, AI opportunities are often identified by business units outside of IT. Organisations must improve their “AI Quotient” – a core understanding of AI’s benefits, opportunities, and best applications. Broad upskilling across leadership and the wider business will accelerate AI adoption and increase the success rate of AI pilots, ensuring the right people guide investments from the start.
• AI Governance. Trust is the key to long-term AI adoption and success. Being able to use AI to do the “right things” for customers, employees, and the organisation will ultimately drive the success of GenAI initiatives. Many AI pilots fail due to user distrust – whether in the quality of the initial data or in AI-driven outcomes they perceive as unethical for certain stakeholders. For example, an AI model that pushes customers toward higher-priced products or services, regardless of their actual needs, may yield short-term financial gains but will ultimately lose to ethical competitors who prioritise customer trust and satisfaction. Some AI providers, like IBM and Microsoft, are prioritising AI ethics by offering tools and platforms that embed ethical principles into AI operations, ensuring long-term success for customers who adopt responsible AI practices.
GenAI and Agentic AI initiatives are far from becoming standard business practice. Given the current economic and political uncertainty, many organisations will limit unbudgeted spending until markets stabilise. However, technology and business leaders should proactively address the key barriers slowing AI adoption within their organisations. As more AI platforms adopt the innovations that helped DeepSeek reduce model development costs, the economic hurdles to GenAI will become easier to overcome.
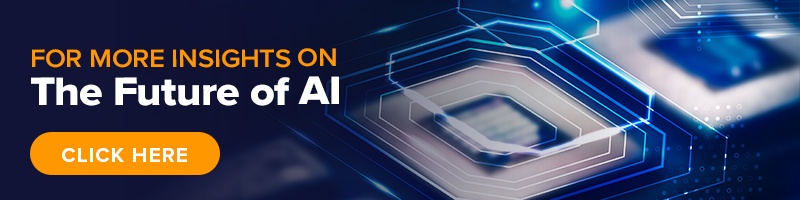
When OpenAI released ChatGPT, it became obvious – and very fast – that we were entering a new era of AI. Every tech company scrambled to release a comparable service or to infuse their products with some form of GenAI. Microsoft, piggybacking on its investment in OpenAI was the fastest to market with impressive text and image generation for the mainstream. Copilot is now embedded across its software, including Microsoft 365, Teams, GitHub, and Dynamics to supercharge the productivity of developers and knowledge workers. However, the race is on – AWS and Google are actively developing their own GenAI capabilities.
AWS Catches Up as Enterprise Gains Importance
Without a consumer-facing AI assistant, AWS was less visible during the early stages of the GenAI boom. They have since rectified this with a USD 4B investment into Anthropic, the makers of Claude. This partnership will benefit both Amazon and Anthropic, bringing the Claude 3 family of models to enterprise customers, hosted on AWS infrastructure.
As GenAI quickly emerges from shadow IT to an enterprise-grade tool, AWS is catching up by capitalising on their position as cloud leader. Many organisations view AWS as a strategic partner, already housing their data, powering critical applications, and providing an environment that developers are accustomed to. The ability to augment models with private data already residing in AWS data repositories will make it an attractive GenAI partner.
AWS has announced the general availability of Amazon Q, their suite of GenAI tools aimed at developers and businesses. Amazon Q Developer expands on what was launched as Code Whisperer last year. It helps developers accelerate the process of building, testing, and troubleshooting code, allowing them to focus on higher-value work. The tool, which can directly integrate with a developer’s chosen IDE, uses NLP to develop new functions, modernise legacy code, write security tests, and explain code.
Amazon Q Business is an AI assistant that can safely ingest an organisation’s internal data and connect with popular applications, such as Amazon S3, Salesforce, Microsoft Exchange, Slack, ServiceNow, and Jira. Access controls can be implemented to ensure data is only shared with authorised users. It leverages AWS’s visualisation tool, QuickSight, to summarise findings. It also integrates directly with applications like Slack, allowing users to query it directly.
Going a step further, Amazon Q Apps (in preview) allows employees to build their own lightweight GenAI apps using natural language. These employee-created apps can then be published to an enterprise’s app library for broader use. This no-code approach to development and deployment is part of a drive to use AI to increase productivity across lines of business.
AWS continues to expand on Bedrock, their managed service providing access to foundational models from companies like Mistral AI, Stability AI, Meta, and Anthropic. The service also allows customers to bring their own model in cases where they have already pre-trained their own LLM. Once a model is selected, organisations can extend its knowledge base using Retrieval-Augmented Generation (RAG) to privately access proprietary data. Models can also be refined over time to improve results and offer personalised experiences for users. Another feature, Agents for Amazon Bedrock, allows multi-step tasks to be performed by invoking APIs or searching knowledge bases.
To address AI safety concerns, Guardrails for Amazon Bedrock is now available to minimise harmful content generation and avoid negative outcomes for users and brands. Contentious topics can be filtered by varying thresholds, and Personally Identifiable Information (PII) can be masked. Enterprise-wide policies can be defined centrally and enforced across multiple Bedrock models.
Google Targeting Creators
Due to the potential impact on their core search business, Google took a measured approach to entering the GenAI field, compared to newer players like OpenAI and Perplexity. The useability of Google’s chatbot, Gemini, has improved significantly since its initial launch under the moniker Bard. Its image generator, however, was pulled earlier this year while it works out how to carefully tread the line between creativity and sensitivity. Based on recent demos though, it plans to target content creators with images (Imagen 3), video generation (Veo), and music (Lyria).
Like Microsoft, Google has seen that GenAI is a natural fit for collaboration and office productivity. Gemini can now assist the sidebar of Workspace apps, like Docs, Sheets, Slides, Drive, Gmail, and Meet. With Google Search already a critical productivity tool for most knowledge workers, it is determined to remain a leader in the GenAI era.
At their recent Cloud Next event, Google announced the Gemini Code Assist, a GenAI-powered development tool that is more robust than its previous offering. Using RAG, it can customise suggestions for developers by accessing an organisation’s private codebase. With a one-million-token large context window, it also has full codebase awareness making it possible to make extensive changes at once.
The Hardware Problem of AI
The demands that GenAI places on compute and memory have created a shortage of AI chips, causing the valuation of GPU giant, NVIDIA, to skyrocket into the trillions of dollars. Though the initial training is most hardware-intensive, its importance will only rise as organisations leverage proprietary data for custom model development. Inferencing is less compute-heavy for early use cases, such as text generation and coding, but will be dwarfed by the needs of image, video, and audio creation.
Realising compute and memory will be a bottleneck, the hyperscalers are looking to solve this constraint by innovating with new chip designs of their own. AWS has custom-built specialised chips – Trainium2 and Inferentia2 – to bring down costs compared to traditional compute instances. Similarly, Microsoft announced the Maia 100, which it developed in conjunction with OpenAI. Google also revealed its 6th-generation tensor processing unit (TPU), Trillium, with significant increase in power efficiency, high bandwidth memory capacity, and peak compute performance.
The Future of the GenAI Landscape
As enterprises gain experience with GenAI, they will look to partner with providers that they can trust. Challenges around data security, governance, lineage, model transparency, and hallucination management will all need to be resolved. Additionally, controlling compute costs will begin to matter as GenAI initiatives start to scale. Enterprises should explore a multi-provider approach and leverage specialised data management vendors to ensure a successful GenAI journey.
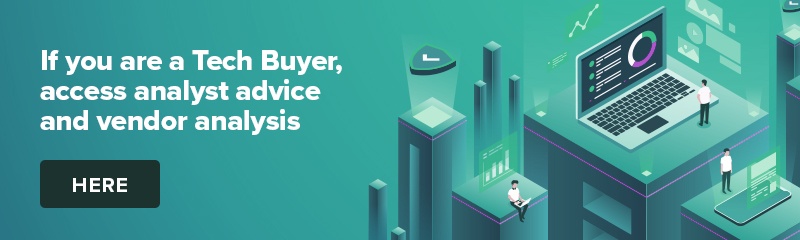