The Manufacturing sector, traditionally defined by stable processes and infrastructure, is now facing a pivotal shift. Rapid technological advancements and shifting global market dynamics have rendered incremental improvements inadequate for long-term competitiveness and growth. To thrive, manufacturers must fundamentally reimagine their entire value chain.
By embracing intelligent systems, enhancing agility, and proactively shaping future-ready operations, organisations can navigate today’s industrial complexities and position themselves for sustained success.
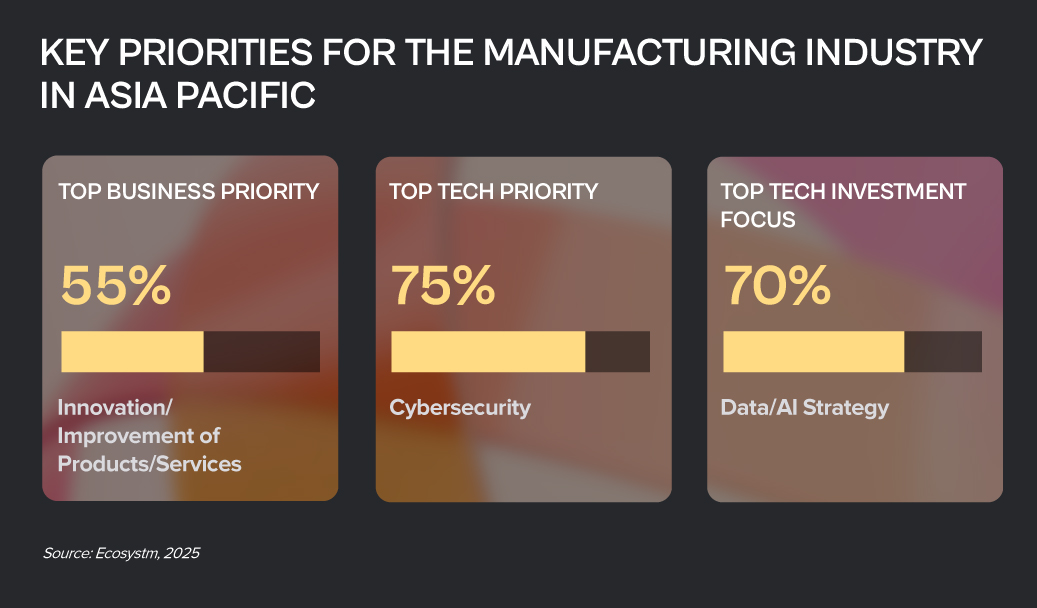
Here are recent examples of Manufacturing transformation in the Asia Pacific.
Click here to download “Future Forward: Reimagining Manufacturing” as a PDF.
Intelligent Automation & Efficiency
Komatsu Australia, a global industrial equipment manufacturer, tackled growing inefficiencies in its small parts department, where teams manually processed hundreds of PDF invoices daily from more than 250 suppliers.
To streamline this, the company deployed intelligent automation – AI now extracts and validates data from invoices against purchase orders and inputs it directly into the legacy mainframe.
The impact has been sharp: over 300 hours saved annually for one supplier, 1,100 invoices processed in three weeks, and a dramatic drop in manual errors. Employees have shifted to higher-value tasks, and a citizen developer program is enabling staff to build custom automation tools. With a scalable framework in place, Komatsu has not only transformed invoice processing but also set the stage for broader automation across the enterprise.
Data-Driven Insights & Agility
Berger Paints India Ltd., a leader in paints and coatings, needed to scale fast amid rising database loads and complex on-prem systems.
In response, Berger Paints migrated its mission-critical databases and core business applications – covering finance, manufacturing, sales, and asset management – to a high-performance cloud platform.
This shift boosted operational efficiency by 25%, doubled reporting and system response times, and enhanced scalability and disaster recovery with geographically distributed cloud regions. The move simplified access to data, driving faster, insight-driven decision-making. With streamlined infrastructure management and optimised costs, Berger Paints is now poised to leverage advanced technologies like AI/ML, setting the stage for continued innovation and growth.
Connected Operations & Customer Centricity
JSW Steel, one of India’s leading steel producers, set out to shift from a plant-centric model to a customer-first approach. The challenge: integrating complex systems like ERP, CRM, and manufacturing to streamline operations and improve order fulfillment.
With a robust integration platform, JSW Steel connected over 32 systems using 120+ APIs – automating processes and enabling real-time data flow across orders, inventory, pricing, and production.
The results speak for themselves: faster order fulfillment, reduced cost-to-serve, and real-time visibility that optimises scheduling. Scalable, composable APIs now support growth, while a 99.7% success rate across 7.2 million API calls ensures reliability. JSW Steel has transformed how it operates – running faster, serving smarter, and delivering better customer experiences across the entire order-to-cash journey.
Modernising Core Systems & Foundational Transformation
Fujitsu General, a global leader in air conditioning systems, was constrained by a 30-year-old COBOL-based mainframe and fragmented processes. The legacy system posed a Y2K-like risk and limited operational agility.
The company implemented a modern, unified ERP platform to eliminate risk, streamline operations, and boost agility.
By integrating functions across sales, production, procurement, accounting, and HR and addressing unique business needs with low-code development, the company created a clean, adaptable core system. Robust integration connected disparate data sources, while a central repository eliminated silos. The transformation delivered seamless end-to-end operations, standardised workflows, improved agility, and real-time insights – setting Fujitsu General up for continued innovation and long-term resilience.
Powering Growth with a Modern Network
As a critical supplier to India’s infrastructure boom, Hindalco needed to modernise its network across 55 sites – improving app performance, enabling real-time insights, and building a future-ready, sustainable foundation.
Hindalco replaced its ageing hub-and-spoke model with a modern mesh architecture using SD-WAN.
The new architecture prioritised key app traffic, simplified cloud access, and enabled segmentation. Centralised orchestration and SSE integration brought automation and robust security. The impact: 30% lower costs, 50% faster apps, real-time visibility, rapid deployment, and smarter bandwidth. Hindalco now runs on a lean, secure digital backbone – built for agility, performance, and scale.
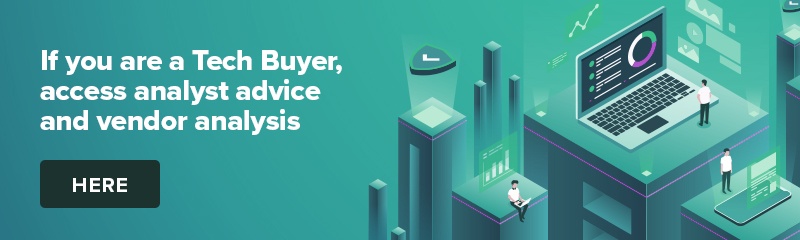
Over the past year, Ecosystm has conducted extensive research, including surveys and in-depth conversations with industry leaders, to uncover the most pressing topics and trends. And unsurprisingly, AI emerged as the dominant theme. Here are some insights from our research on the Manufacturing industry.
Click here to download “AI in Manufacturing: Success Stories & Insights” as a PDF
AI is revolutionising production lines, supply chains, and product development in the manufacturing sector. Yet, many manufacturers find themselves stuck between ambition and execution. Those who bridge this gap will gain a competitive edge, driving innovation and leading the industry forward.
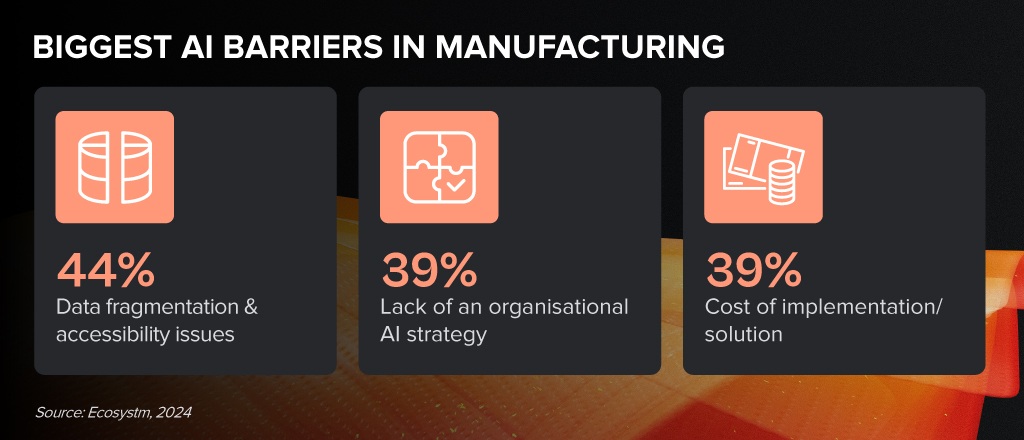
Despite the challenges, Manufacturing organisations are witnessing early AI success in these 3 areas:
- 1. Quality Control & Assurance
- 2. Supply Chain Management & Optimisation
- 3. Process Automation & Efficiency
Quality Control & Assurance
- Defect Detection. Identifying defects in products and improving quality
- Product Inspection. Implementing AI-powered vision systems to inspect products and ensure they meet quality standards
- Data Analysis. Analysing operational data and customer feedback to identify operations and product issues
“AI is the future of design. It streamlines the design process, leading to faster time-to-market and superior products.” – OPERATIONS LEADER
Supply Chain Management & Optimisation
- Inventory Management. Optimising inventory levels and reducing costs
- Supply Chain Visibility. Gaining real-time visibility into supply chain operations
- Demand Forecasting. Predicting demand for products to improve production planning and inventory management
“By leveraging AI, we’re not just optimising our supply chain; we’re pioneering sustainable practices to reduce our carbon footprint.” – CIO
Process Automation and Efficiency
- Process Optimisation. Identifying areas for improvement and potential operational bottlenecks
- Predictive Maintenance. Predicting equipment failures and preventing downtime
- Customer Feedback Analysis. Analysing customer feedback to improve design processes, products, and services
“Our goal is to build intelligent manufacturing plants. By proactively monitoring equipment health, we minimise downtime and maximise productivity – we have set a new internal standard for operational efficiency in the last two years.” – HEAD OF PRODUCTION
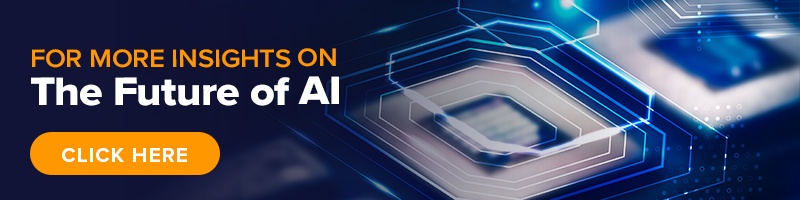
AI has become a business necessity today, catalysing innovation, efficiency, and growth by transforming extensive data into actionable insights, automating tasks, improving decision-making, boosting productivity, and enabling the creation of new products and services.
Generative AI stole the limelight in 2023 given its remarkable advancements and potential to automate various cognitive processes. However, now the real opportunity lies in leveraging this increased focus and attention to shine the AI lens on all business processes and capabilities. As organisations grasp the potential for productivity enhancements, accelerated operations, improved customer outcomes, and enhanced business performance, investment in AI capabilities is expected to surge.
In this eBook, Ecosystm VP Research Tim Sheedy and Vinod Bijlani and Aman Deep from HPE APAC share their insights on why it is crucial to establish tailored AI capabilities within the organisation.
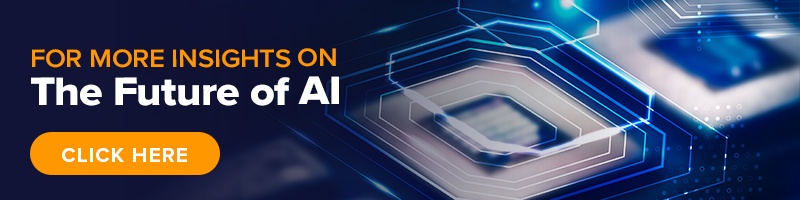
At the end of last year, I had the privilege of attending a session organised by Red Hat where they shared their Asia Pacific roadmap with the tech analyst community. The company’s approach of providing a hybrid cloud application platform centred around OpenShift has worked well with clients who favour a hybrid cloud approach. Going forward, Red Hat is looking to build and expand their business around three product innovation focus areas. At the core is their platform engineering, flanked by focus areas on AI/ML and the Edge.
The Opportunities
Besides the product innovation focus, Red Hat is also looking into several emerging areas, where they’ve seen initial client success in 2023. While use cases such as operational resilience or edge lifecycle management are long-existing trends, carbon-aware workload scheduling may just have appeared over the horizon. But two others stood out for me with a potentially huge demand in 2024.
GPU-as-a-Service. GPUaaS addresses a massive demand driven by the meteoric rise of Generative AI over the past 12 months. Any innovation that would allow customers a more flexible use of scarce and expensive resources such as GPUs can create an immediate opportunity and Red Hat might have a first mover and established base advantage. Particularly GPUaaS is an opportunity in fast growing markets, where cost and availability are strong inhibitors.
Digital Sovereignty. Digital sovereignty has been a strong driver in some markets – for example in Indonesia, which has led to most cloud hyperscalers opening their data centres onshore over the past years. Yet not the least due to the geography of Indonesia, hybrid cloud remains an important consideration, where digital sovereignty needs to be managed across a diverse infrastructure. Other fast-growing markets have similar challenges and a strong drive for digital sovereignty. Crucially, Red Hat may well have an advantage where onshore hyperscalers are not yet available (for example in Malaysia).
Strategic Focus Areas for Red Hat
Red Hat’s product innovation strategy is robust at its core, particularly in platform engineering, but needs more clarity at the periphery. They have already been addressing Edge use cases as an extension of their core platform, especially in the Automotive sector, establishing a solid foundation in this area. Their focus on AI/ML may be a bit more aspirational, as they are looking to not only AI-enable their core platform but also expand it into a platform to run AI workloads. AI may drive interest in hybrid cloud, but it will be in very specific use cases.
For Red Hat to be successful in the AI space, it must steer away from competing straight out with the cloud-native AI platforms. They must identify the use cases where AI on hybrid cloud has a true advantage. Such use cases will mainly exist in industries with a strong Edge component, potentially also with a still heavy reliance on on-site data centres. Manufacturing is the prime example.
Red Hat’s success in AI/ML use cases is tightly connected to their (continuing) success in Edge use cases, all build on the solid platform engineering foundation.
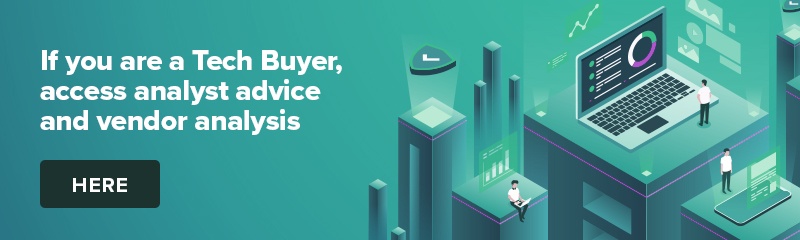
Generative AI has stolen the limelight in 2023 from nearly every other technology – and for good reason. The advances made by Generative AI providers have been incredible, with many human “thinking” processes now in line to be automated.
But before we had Generative AI, there was the run-of-the-mill “traditional AI”. However, despite the traditional tag, these capabilities have a long way to run within your organisation. In fact, they are often easier to implement, have less risk (and more predictability) and are easier to generate business cases for. Traditional AI systems are often already embedded in many applications, systems, and processes, and can easily be purchased as-a-service from many providers.
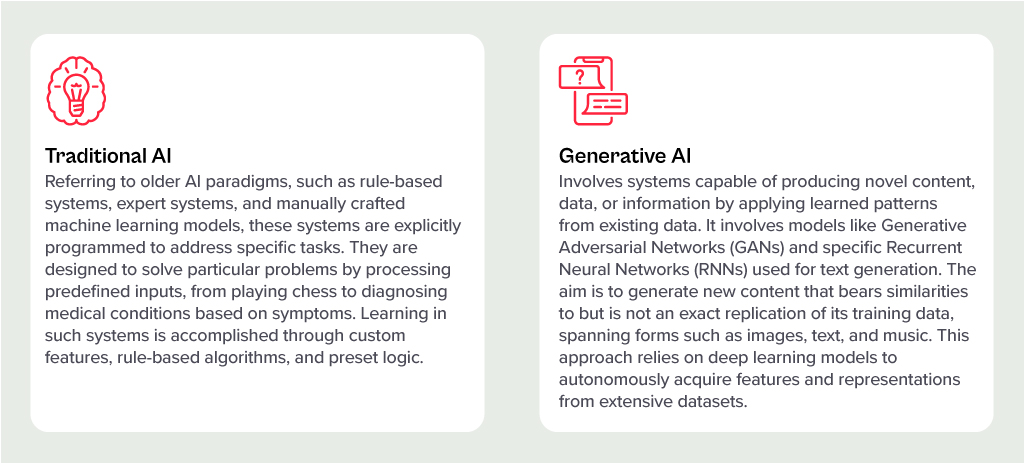
Unlocking the Potential of AI Across Industries
Many organisations around the world are exploring AI solutions today, and the opportunities for improvement are significant:
- Manufacturers are designing, developing and testing in digital environments, relying on AI to predict product responses to stress and environments. In the future, Generative AI will be called upon to suggest improvements.
- Retailers are using AI to monitor customer behaviours and predict next steps. Algorithms are being used to drive the best outcome for the customer and the retailer, based on previous behaviours and trained outcomes.
- Transport and logistics businesses are using AI to minimise fuel usage and driver expenses while maximising delivery loads. Smart route planning and scheduling is ensuring timely deliveries while reducing costs and saving on vehicle maintenance.
- Warehouses are enhancing the safety of their environments and efficiently moving goods with AI. Through a combination of video analytics, connected IoT devices, and logistical software, they are maximising the potential of their limited space.
- Public infrastructure providers (such as shopping centres, public transport providers etc) are using AI to monitor public safety. Video analytics and sensors is helping safety and security teams take public safety beyond traditional human monitoring.
AI Impacts Multiple Roles
Even within the organisation, different lines of business expect different outcomes for AI implementations.
- IT teams are monitoring infrastructure, applications, and transactions – to better understand root-cause analysis and predict upcoming failures – using AI. In fact, AIOps, one of the fastest-growing areas of AI, yields substantial productivity gains for tech teams and boosts reliability for both customers and employees.
- Finance teams are leveraging AI to understand customer payment patterns and automate the issuance of invoices and reminders, a capability increasingly being integrated into modern finance systems.
- Sales teams are using AI to discover the best prospects to target and what offers they are most likely to respond to.
- Contact centres are monitoring calls, automating suggestions, summarising records, and scheduling follow-up actions through conversational AI. This is allowing to get agents up to speed in a shorter period, ensuring greater customer satisfaction and increased brand loyalty.
Transitioning from Low-Risk to AI-Infused Growth
These are just a tiny selection of the opportunities for AI. And few of these need testing or business cases – many of these capabilities are available out-of-the-box or out of the cloud. They don’t need deep analysis by risk, legal, or cybersecurity teams. They just need a champion to make the call and switch them on.
One potential downside of Generative AI is that it is drawing unwarranted attention to well-established, low-risk AI applications. Many of these do not require much time from data scientists – and if they do, the challenge is often finding the data and creating the algorithm. Humans can typically understand the logic and rules that the models create – unlike Generative AI, where the outcome cannot be reverse-engineered.
The opportunity today is to take advantage of the attention that LLMs and other Generative AI engines are getting to incorporate AI into every conceivable aspect of a business. When organisations understand the opportunities for productivity improvements, speed enhancement, better customer outcomes and improved business performance, the spend on AI capabilities will skyrocket. Ecosystm estimates that for most organisations, AI spend will be less than 5% of their total tech spend in 2024 – but it is likely to grow to over 20% within the next 4-5 years.
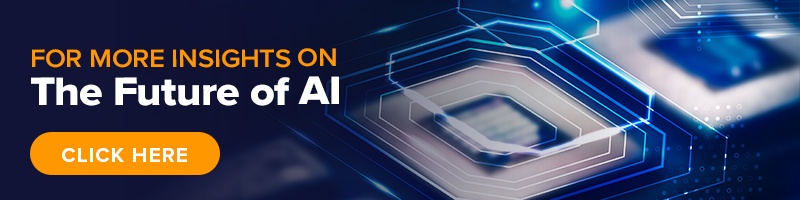
Generative AI is seeing enterprise interest and early adoption enhancing efficiency, fostering innovation, and pushing the boundaries of possibility. It has the potential of reshaping industries – and fast!
However, alongside its immense potential, Generative AI also raises concerns. Ethical considerations surrounding data privacy and security come to the forefront, as powerful AI systems handle vast amounts of sensitive information.
Addressing these concerns through responsible AI development and thoughtful regulation will be crucial to harnessing the full transformative power of Generative AI.
Read on to find out the key challenges faced in implementing Generative AI and explore emerging use cases in industries such as Financial Services, Retail, Manufacturing, and Healthcare.
Download ‘Generative AI: Industry Adoption’ as a PDF
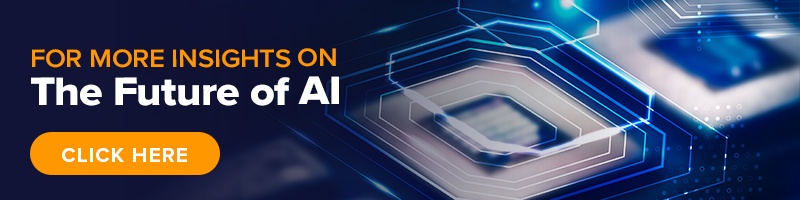
The Manufacturing industry is at crossroads today. It faces challenges such as geopolitical risks, supply chain disruptions, changing regulatory environments, workforce shortages, and changing consumer demands. Overcoming these requires innovation, collaboration, and proactive adaptation.
Fortunately, many of these challenges can be mitigated by technology. The future of Manufacturing will be shaped by advanced technology, automation, and AI. We are seeing early evidence of how smart factories, robotics, and 3D printing are transforming production processes for increased efficiency and customisation.
Manufacturing is all set to become more agile, efficient, and sustainable.
Read on to find out the changing priorities and key trends in Manufacturing; about the World Economic Forum’s Global Lighthouse Network initiative; and where Ecosystm advisor Kaushik Ghatak sees as the Future of Manufacturing.
Click here to download ‘The Future of Manufacturing’ as a PDF
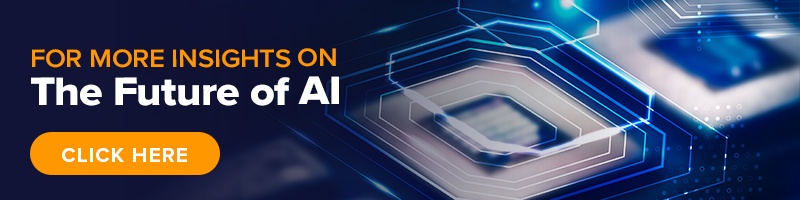
When non-organic (man-made) fabric was introduced into fashion, there were a number of harsh warnings about using polyester and man-made synthetic fibres, including their flammability.
In creating non-organic data sets, should we also be creating warnings on their use and flammability? Let’s look at why synthetic data is used in industries such as Financial Services, Automotive as well as for new product development in Manufacturing.
Synthetic Data Defined
Synthetic data can be defined as data that is artificially developed rather than being generated by actual interactions. It is often created with the help of algorithms and is used for a wide range of activities, including as test data for new products and tools, for model validation, and in AI model training. Synthetic data is a type of data augmentation which involves creating new and representative data.
Why is it used?
The main reasons why synthetic data is used instead of real data are cost, privacy, and testing. Let’s look at more specifics on this:
- Data privacy. When privacy requirements limit data availability or how it can be used. For example, in Financial Services where restrictions around data usage and customer privacy are particularly limiting, companies are starting to use synthetic data to help them identify and eliminate bias in how they treat customers – without contravening data privacy regulations.
- Data availability. When the data needed for testing a product does not exist or is not available to the testers. This is often the case for new releases.
- Data for testing. When training data is needed for machine learning algorithms. However, in many instances, such as in the case of autonomous vehicles, the data is expensive to generate in real life.
- Training across third parties using cloud. When moving private data to cloud infrastructures involves security and compliance risks. Moving synthetic versions of sensitive data to the cloud can enable organisations to share data sets with third parties for training across cloud infrastructures.
- Data cost. Producing synthetic data through a generative model is significantly more cost-effective and efficient than collecting real-world data. With synthetic data, it becomes cheaper and faster to produce new data once the generative model is set up.
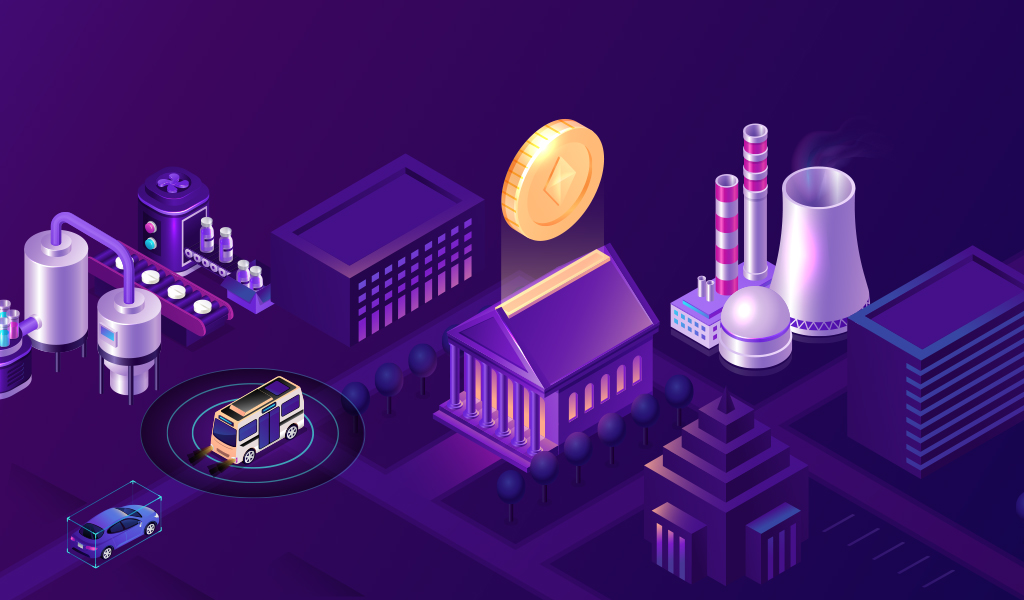
Why should it cause concern?
If real dataset contains biases, data augmented from it will contain biases, too. So, identification of optimal data augmentation strategy is important.
If the synthetic set doesn’t truly represent the original customer data set, it might contain the wrong buying signals regarding what customers are interested in or are inclined to buy.
Synthetic data also requires some form of output/quality control and internal regulation, specifically in highly regulated industries such as the Financial Services.
Creating incorrect synthetic data also can get a company in hot water with external regulators. For example, if a company created a product that harmed someone or didn’t work as advertised, it could lead to substantial financial penalties and, possibly, closer scrutiny in the future.
Conclusion
Synthetic data allows us to continue developing new and innovative products and solutions when the data necessary to do so wouldn’t otherwise be present or available due to volume, data sensitivity or user privacy challenges. Generating synthetic data comes with the flexibility to adjust its nature and environment as and when required in order to improve the performance of the model to create opportunities to check for outliers and extreme conditions.
As we return to the office, there is a growing reliance on devices to tell us how safe and secure the environment is for our return. And in specific application areas, such as Healthcare and Manufacturing, IoT data is critical for decision-making. In some sectors such as Health and Wellness, IoT devices collect personally identifiable information (PII). IoT technology is so critical to our current infrastructures that the physical wellbeing of both individuals and organisations can be at risk.
Trust & Data
IoT are also vulnerable to breaches if not properly secured. And with a significant increase in cybersecurity events over the last year, the reliance on data from IoT is driving the need for better data integrity. Security features such as data integrity and device authentication can be accomplished through the use of digital certificates and these features need to be designed as part of the device prior to manufacturing. Because if you cannot trust either the IoT devices and their data, there is no point in collecting, running analytics, and executing decisions based on the information collected.
We discuss the role of embedding digital certificates into the IoT device at manufacture to enable better security and ongoing management of the device.
Securing IoT Data from the Edge
So much of what is happening on networks in terms of real-time data collection happens at the Edge. But because of the vast array of IoT devices connecting at the Edge, there has not been a way of baking trust into the manufacture of the devices. With a push to get the devices to market, many manufacturers historically have bypassed efforts on security. Devices have been added on the network at different times from different sources.
There is a need to verify the IoT devices and secure them, making sure to have an audit trail on what you are connecting to and communicating with.
So from a product design perspective, this leads us to several questions:
- How do we ensure the integrity of data from devices if we cannot authenticate them?
- How do we ensure that the operational systems being automated are controlled as intended?
- How do we authenticate the device on the network making the data request?
Using a Public Key Infrastructure (PKI) approach maintains assurance, integrity and confidentiality of data streams. PKI has become an important way to secure IoT device applications, and this needs to be built into the design of the device. Device authentication is also an important component, in addition to securing data streams. With good design and a PKI management that is up to the task you should be able to proceed with confidence in the data created at the Edge.
Johnson Controls/DigiCert have designed a new way of managing PKI certification for IoT devices through their partnership and integration of the DigiCert ONE™ PKI management platform and the Johnson Controls OpenBlue IoT device platform. Based on an advanced, container-based design, DigiCert ONE allows organisations to implement robust PKI deployment and management in any environment, roll out new services and manage users and devices across your organisation at any scale no matter the stage of their lifecycle. This creates an operational synergy within the Operational Technology (OT) and IoT spaces to ensure that hardware, software and communication remains trusted throughout the lifecycle.

Rationale on the Role of Certification in IoT Management
Digital certificates ensure the integrity of data and device communications through encryption and authentication, ensuring that transmitted data are genuine and have not been altered or tampered with. With government regulations worldwide mandating secure transit (and storage) of PII data, PKI can help ensure compliance with the regulations by securing the communication channel between the device and the gateway.
Connected IoT devices interact with each other through machine to machine (M2M) communication. Each of these billions of interactions will require authentication of device credentials for the endpoints to prove the device’s digital identity. In such scenarios, an identity management approach based on passwords or passcodes is not practical, and PKI digital certificates are by far the best option for IoT credential management today.
Creating lifecycle management for connected devices, including revocation of expired certificates, is another example where PKI can help to secure IoT devices. Having a robust management platform that enables device management, revocation and renewal of certificates is a critical component of a successful PKI. IoT devices will also need regular patches and upgrades to their firmware, with code signing being critical to ensure the integrity of the downloaded firmware – another example of the close linkage between the IoT world and the PKI world.
Summary
PKI certification benefits both people and processes. PKI enables identity assurance while digital certificates validate the identity of the connected device. Use of PKI for IoT is a necessary trend for sense of trust in the network and for quality control of device management.
Identifying the IoT device is critical in managing its lifespan and recognizing its legitimacy in the network. Building in the ability for PKI at the device’s manufacture is critical to enable the device for its lifetime. By recognizing a device, information on it can be maintained in an inventory and its lifecycle and replacement can be better managed. Once a certificate has been distributed and certified, having the control of PKI systems creates life-cycle management.
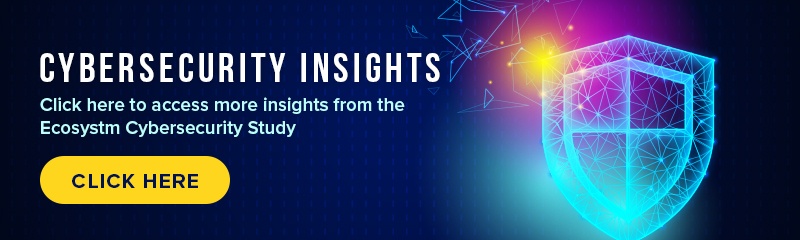