The rollout of 5G combined with edge computing in remote locations will change the way maintenance is carried out in the field. Historically, service teams performed maintenance either in a reactive fashion – fixing equipment when it broke – or using a preventative calendar-based approach. Neither of these methods is satisfactory, with the former being too late and resulting in failure while the latter is necessarily too early, resulting in excessive expenditure and downtime. The availability of connected sensors has allowed service teams to shift to condition monitoring without the need for taking equipment offline for inspections. The advent of analytics takes this approach further and has given us optimised scheduling in the form of predictive maintenance.
The next step is prescriptive maintenance in which AI can recommend action based on current and predicted condition according to expected usage or environmental circumstances. This could be as simple as alerting an operator to automatically ordering parts and scheduling multiple servicing tasks depending on forecasted production needs in the short term.
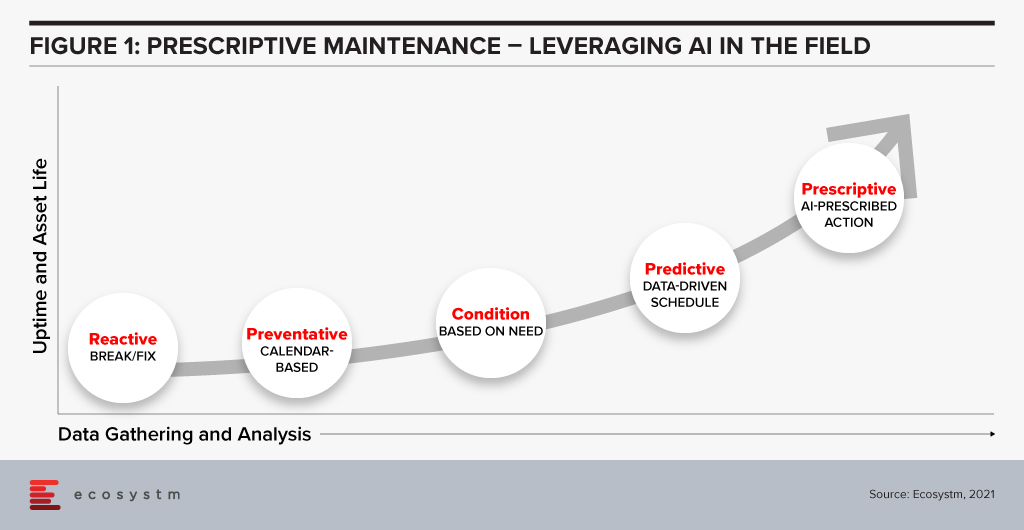
Prescriptive maintenance has only become possible with the advancement of AI and digital twin technology, but imminent improvements in connectivity and computing will take servicing to a new level. The rollout of 5G will give a boost to bandwidth, reduce latency, and increase the number of connections possible. Equipment in remote locations – such as transmission lines or machinery in resource industries – will benefit from the higher throughput of 5G connectivity, either as part of an operator’s network rollout or a private on-site deployment. Mobile machinery, particularly vehicles, which can include hundreds of sensors will no longer be required to wait until arrival before the condition can be assessed. Furthermore, vehicles equipped with external sensors can inspect stationary infrastructure as it passes by.
Edge computing – either carried out by miniature onboard devices or at smaller scale data centres embedded in 5G networks – ensure that intensive processing can be carried out closer to equipment than with a typical cloud environment. Bandwidth hungry applications, such as video and time series analysis, can be conducted with only meta data transmitted immediately and full archives uploaded with less urgency.
Prescriptive Maintenance with 5G and the Edge – Use Cases
- Transportation. Bridges built over railway lines equipped with high-speed cameras can monitor passing trains to inspect for damage. Data-intensive video analysis can be conducted on local devices for a rapid response while selected raw data can be uploaded to the cloud over 5G to improve inference models.
- Mining. Private 5G networks built-in remote sites can provide connectivity between fixed equipment, vehicles, drones, robotic dogs, workers, and remote operations centres. Autonomous haulage trucks can be monitored remotely and in the event of a breakdown, other vehicles can be automatically redirected to prevent dumping queues.
- Utilities. Emergency maintenance needs can be prioritised before extreme weather events based on meteorological forecasts and their impact on ageing parts. Machine learning can be used to understand location-specific effects of, for example, salt content in off-shore wind turbine cables. Early detection of turbine rotor cracks can recommend shutdown during high-load periods.

Data as an Asset
Effective prescriptive maintenance only becomes possible after the accumulation and integration of multiple data sources over an extended period. Inference models should understand both normal and abnormal equipment performance in various conditions, such as extreme weather, during incorrect operation, or when adjacent parts are degraded. For many smaller organisations or those deploying new equipment, the necessary volume of data will not be available without the assistance of equipment manufacturers. Moreover, even manufacturers will not have sufficient data on interaction with complementary equipment. This provides an opportunity for large operators to sell their own inference models as a new revenue stream. For example, an electrical grid operator in North America can partner with a similar, but smaller organisation in Europe to provide operational data and maintenance recommendations. Similarly, telecom providers, regional transportation providers, logistics companies, and smart cities will find industry players in other geographies that they do not naturally compete with.
Recommendations
- Employing multiple sensors. Baseline conditions and failure signatures are improved using machine learning based on feeds from multiple sensors, such as those that monitor vibration, sound, temperature, pressure, and humidity. The use of multiple sensors makes it possible to not only identify potential failure but also the reason for it and can therefore more accurately prescribe a solution to prevent an outage.
- Data assessment and integration. Prescriptive maintenance is most effective when multiple data sources are unified as inputs. Identify the location of these sources, such as ERP systems, time series on site, environmental data provided externally, or even in emails or on paper. A data fabric should be considered to ensure insights can be extracted from data no matter the environment it resides in.
- Automated action. Reduce the potential for human error or delay by automatically generating alerts and work orders for resource managers and service staff in the event of anomaly detection. Criticality measures should be adopted to help prioritise maintenance tasks and reduce alert noise.
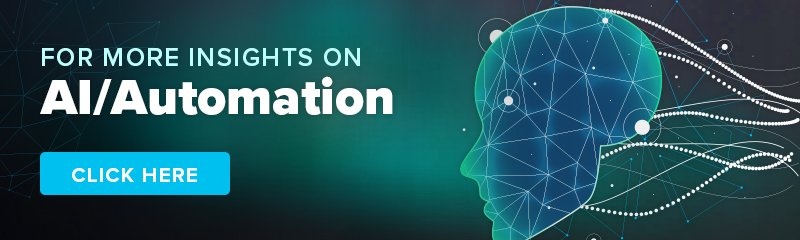
Many years ago – back in 2003 – I spent some quality time with BMC at their global analyst event in Phoenix, Arizona and they introduced the concept of “Business Service Management” (BSM). I was immediately a convert – that businesses can focus their IT Service Management initiatives on the business and customer services that the technology supports. Businesses that use BSM can have an understanding of the impact and importance of technology systems and assets because there is a direct link between these assets and the systems they support. A router that supports a customer payment platform suddenly becomes a much higher priority than one that supports an employee expense platform.
But for most businesses, this promise was never delivered. Creating a BSM solution became a highly manual process – mapping processes, assets, and applications. Many businesses that undertook this challenge reported that by the time they had mapped their processes, the map was out of date – as processes had changed; assets had been retired, replaced, or upgraded; software had been moved to the cloud or new modules had been implemented; and architectures had changed. Effectively their BSM mapping was often a pointless task – sometimes only delivering value in the slow to change systems – back-end applications and infrastructure that delivers limited value and has a defined retirement date.
The Growth of Digital Business Strategies
Our technology systems are becoming more important than ever as digital business strategies are realised and digital interactions with customers, employees, and partners significantly increase. Many businesses expect their digital investments to remain strong well into 2022 (Figure 1). More than ever, we need to understand the link between our tech systems and the business and customer services they support.
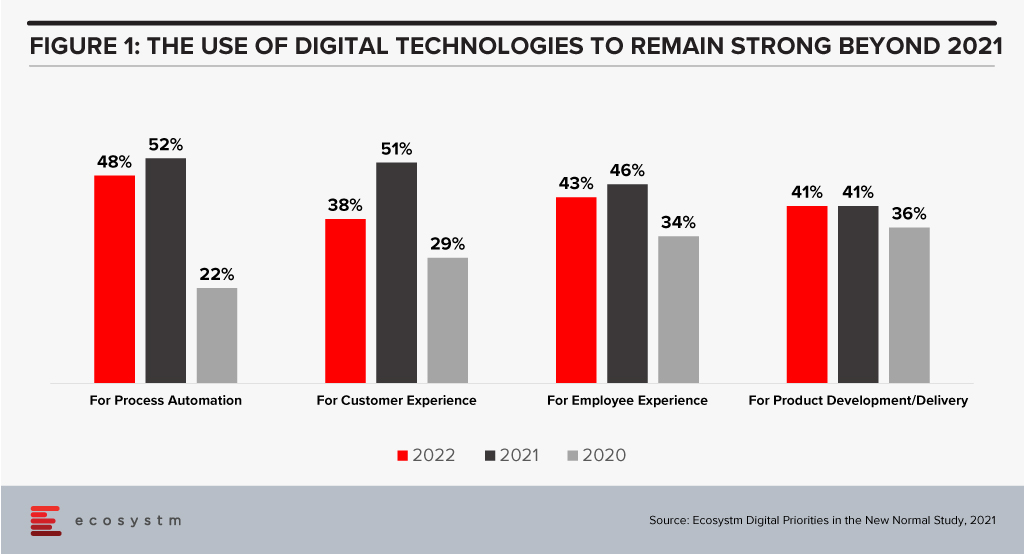
I recently had the opportunity to attend a briefing by ServiceNow regarding their new “AI-Powered Service Operations” that highlighted their service-aware CMDB – adding machine learning to their service mapping capabilities. The upgraded offering has the ability to map entire environments in hours or minutes – not months or weeks. And as a machine learning capability, it is only likely to get smarter – to learn from their customers’ use of the service and begin to recognise what applications, systems, and infrastructure are likely to be supporting each business service.
This heralds a new era in service management – one where the actual business and customer impact of outages is known immediately; where the decision to delay an upgrade or fix to a known problem can be made with a full understanding of the impacts. At one of my previous employers, email went down for about a week. It was finally attributed to an upgrade to network equipment that sat between the email system and the corporate network and the internet. The tech teams were scratching their heads for days as there was no documented link between this piece of hardware and the email system. The impact of the outage was certainly felt by the business – but had it happened at the end of the financial year, it could have impacted perhaps 10-20% of the business bookings as many deals came in at that time.
Being able to understand the link between infrastructure, cloud services, applications, databases, middleware and business processes and services is of huge value to every business – particularly as the percentage of business through digital channels and touchpoints continues to accelerate.
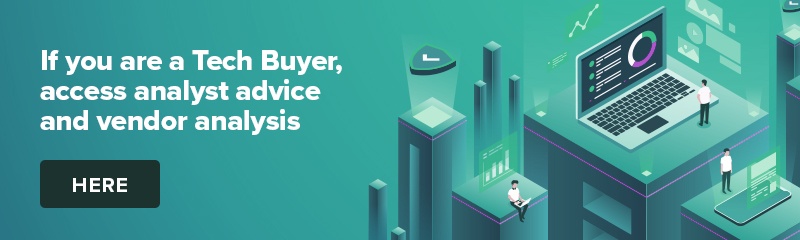
BHP – the multinational mining giant – has signed agreements with AWS and Microsoft Azure as their long-term cloud providers to support their digital transformation journey. This move is expected to accelerate BHP’s cloud journey, helping them deploy and scale their digital operations to the workforce quickly while reducing the need for on-premises infrastructure.
Ecosystm research has consistently shown that many large organisations are using the learnings from how the COVID-19 pandemic impacted their business to re-evaluate their Digital Transformation strategy – leveraging next generation cloud, machine learning and data analytics capabilities.
BHP’s Dual Cloud Strategy
BHP is set to use AWS’s analytics, machine learning, storage and compute platform to deploy digital services and improve operational performance. They will also launch an AWS Cloud Academy Program to train and upskill their employees on AWS cloud skills – joining other Australian companies supporting their digital workforce by forming cloud guilds such as National Australia Bank, Telstra and Kmart Group.
Meanwhile, BHP will use Microsoft’s Azure cloud platform to host their global applications portfolio including SAP S/4 HANA environment. This is expected to enable BHP to reduce their reliance on regional data centres and leverage Microsoft’s cloud environment, licenses and SAP applications. The deal extends their existing relationship with Microsoft where BHP is using Office 365, Dynamics 365 and HoloLens 2 platforms to support their productivity and remote operations.
Ecosystm principal Advisor, Alan Hesketh says, “This dual sourcing is likely to achieve cost benefits for BHP from a competitive negotiation stand-point, and positions BHP well to negotiate further improvements in the future. With their scale, BHP has negotiating power that most cloud service customers cannot achieve – although an effective competitive process is likely to offer tech buyers some improvements in pricing.”

Can this Strategy Work for You?
Hesketh thinks that the split between Microsoft for Operations and AWS for Analytics will provide some interesting challenges for BHP. “It is likely that high volumes of data will need to be moved between the two platforms, particularly from Operations to Analytics and AI. The trend is to run time-critical analytics directly from the operational systems using the power of in-memory databases and the scalable cloud platform.”
“As BHP states, using the cloud reduces the need to put hardware on-premises, and allows the faster deployment of digital innovations from these cloud platforms. While achieving technical and cost improvements in their Operations and Analytics domains, it may compromise the user experience (UX). The UX delivered by the two clouds is quite different – so delivering an integrated experience is likely to require an additional layer that is capable of delivering a consistent UX. BHP already has a strong network infrastructure in place, so they are likely to achieve this within their existing platforms. If there is a need to build this UX layer, it is likely to reduce the speed of deployment that BHP is targeting with the dual cloud procurement approach.”
Many businesses that have previously preferred a single cloud vendor will find that they will increasingly evaluate multiple cloud environments, in the future. The adoption of modern development environments and architectures such as containers, microservices, open-source, and DevOps will help them run their applications and processes on the most suitable cloud option.
While this strategy may well work for BHP, Hesketh adds, “Tech buyers considering a hybrid approach to cloud deployment need to have robust enterprise and technology architectures in place to make sure the users get the experience they need to support their roles.”
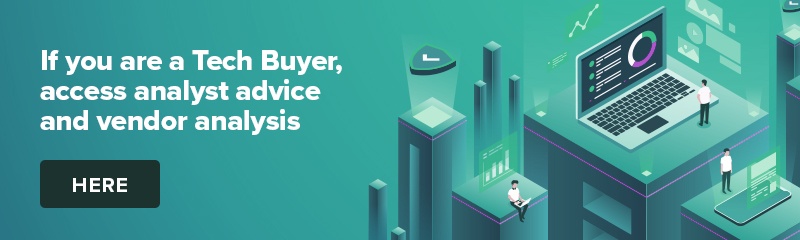
In this Insight, our guest author Anupam Verma talks about how the Global Capability Centres (GCCs) in India are poised to become Global Transformation Centres. “In the post-COVID world, industry boundaries are blurring, and business models are being transformed for the digital age. While traditional functions of GCCs will continue to be providing efficiencies, GCCs will be ‘Digital Transformation Centres’ for global businesses.”
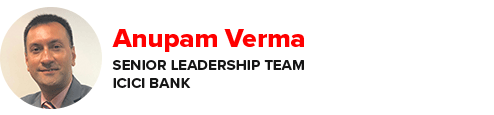
India has a lot to offer to the world of technology and transformation. Attracted by the talent pool, enabling policies, digital infrastructure, and competitive cost structure, MNCs have long embraced India as a preferred destination for Global Capability Centres (GCCs). It has been reported that India has more than 1,700 GCCs with an estimated global market share of over 50%.
GCCs employ around 1 million Indian professionals and has an immense impact on the economy, contributing an estimated USD 30 billion. US MNCs have the largest presence in the market and the dominating industries are BSFI, Engineering & Manufacturing, Tech & Consulting.
GCC capabilities have always been evolving
The journey began with MNCs setting up captives for cost optimisation & operational excellence. GCCs started handling operations (such as back-office and business support functions), IT support (such as app development and maintenance, remote IT infrastructure, and help desk) and customer service contact centres for the parent organisation.
In the second phase, MNCs started leveraging GCCs as centers of excellence (CoE). The focus then was product innovation, Engineering Design & R&D. BFSI and Professional Services firms started expanding the scope to cover research, underwriting, and consulting etc. Some global MNCs that have large GCCs in India are Apple, Microsoft, Google, Nissan, Ford, Qualcomm, Cisco, Wells Fargo, Bank of America, Barclays, Standard Chartered, and KPMG.
In the post-COVID world, industry boundaries are blurring, and business models are being transformed for the digital age. While traditional functions of GCCs will continue to be providing efficiencies, GCCs will be “Digital Transformation Centres” for global businesses.
The New Age GCC in the post-COVID world
On one hand, the pandemic broke through cultural barriers that had prevented remote operations and work. The world became remote everything! On the other hand, it accelerated digital adoption in organisations. Businesses are re-imagining customer experiences and fast-tracking digital transformation enabled by technology (Figure 1). High digital adoption and rising customer expectations will also be a big catalyst for change.
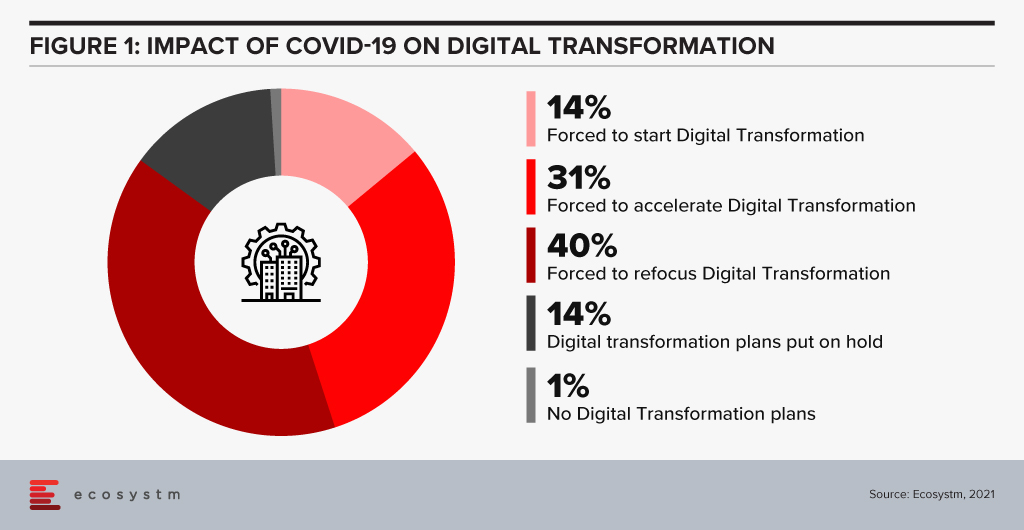
In last few years, India has seen a surge in talent pool in emerging technologies such as data analytics, experience design, AI/ML, robotic process automation, IoT, cloud, blockchain and cybersecurity. GCCs in India will leverage this talent pool and play a pivotal role in enabling digital transformation at a global scale. GCCs will have direct and significant impacts on global business performance and top line growth creating long-term stakeholder value – and not be only about cost optimisation.
GCCs in India will also play an important role in digitisation and automation of existing processes, risk management and fraud prevention using data analytics and managing new risks like cybersecurity.
More and more MNCs in traditional businesses will add GCCs in India over the next decade and the existing 1,700 plus GCCs will grow in scale and scope focussing on innovation. Shift of supply chains to India will also be supported by Engineering R & D Centres. GCCs passed the pandemic test with flying colours when an exceptionally large workforce transitioned to the Work from Home model. In a matter of weeks, the resilience, continuity, and efficiency of GCCs returned to pre-pandemic levels with a distributed and remote workforce.
A Final Take
Having said that, I believe the growth spurt in GCCs in India will come from new-age businesses. Consumer-facing platforms (eCommerce marketplaces, Healthtechs, Edtechs, and Fintechs) are creating digital native businesses. As of June 2021, there are more than 700 unicorns trying to solve different problems using technology and data. Currently, very few unicorns have GCCs in India (notable names being Uber, Grab, Gojek). However, this segment will be one of the biggest growth drivers.
Currently, only 10% of the GCCs in India are from Asia Pacific organisations. Some of the prominent names being Hitachi, Rakuten, Panasonic, Samsung, LG, and Foxconn. Asian MNCs have an opportunity to move fast and stay relevant. This segment is also expected to grow disproportionately.
New age GCCs in India have the potential to be the crown jewel for global MNCs. For India, this has a huge potential for job creation and development of Smart City ecosystems. In this decade, growth of GCCs will be one of the core pillars of India’s journey to a USD 5 trillion economy.
The views and opinions mentioned in the article are personal.
Anupam Verma is part of the Senior Leadership team at ICICI Bank and his responsibilities have included leading the Bank’s strategy in South East Asia to play a significant role in capturing Investment, NRI remittance, and trade flows between SEA and India.
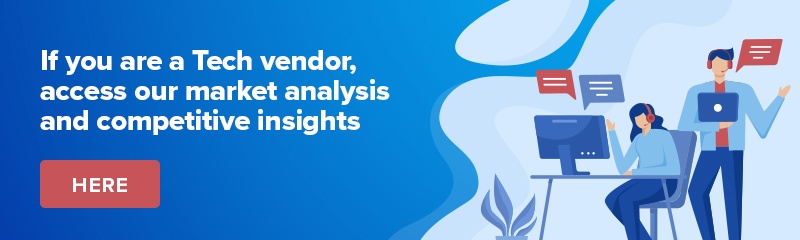
Organisations have found that it is not always desirable to send data to the cloud due to concerns about latency, connectivity, energy, privacy and security. So why not create learning processes at the Edge?
What challenges does IoT bring?
Sensors are now generating such an increasing volume of data that it is not practical that all of it be sent to the cloud for processing. From a data privacy perspective, some sensor data is sensitive and sending data and images to the cloud will be subject to privacy and security constraints.
Regardless of the speed of communications, there will always be a demand for more data from more sensors – along with more security checks and higher levels of encryption – causing the potential for communication bottlenecks.
As the network hardware itself consumes power, sending a constant stream of data to the cloud can be taxing for sensor devices. The lag caused by the roundtrip to the cloud can be prohibitive in applications that require real-time response inputs.
Machine learning (ML) at the Edge should be prioritised to leverage that constant flow of data and address the requirement for real-time responses based on that data. This should be aided by both new types of ML algorithms and by visual processing units (VPUs) being added to the network.
By leveraging ML on Edge networks in production facilities, for example, companies can look out for potential warning signs and do scheduled maintenance to avoid any nasty surprises. Remember many sensors are linked intrinsically to public safety concerns such as water processing, supply of gas or oil, and public transportation such as metros or trains.
Ecosystm research shows that deploying IoT has its set of challenges (Figure 1) – many of these challenges can be mitigated by processing data at the Edge.
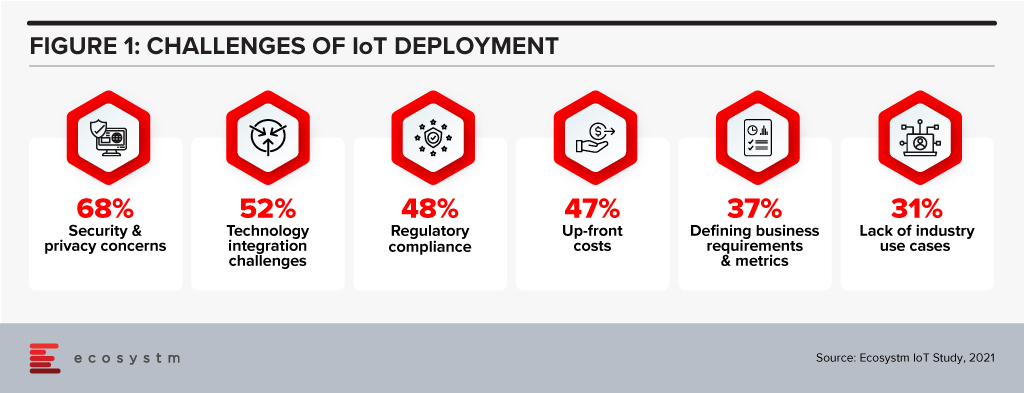
Predictive analytics is a fundamental value proposition for IoT, where responding faster to issues or taking action before issues occur, is key to a high return on investment. So, using edge computing for machine learning located within or close to the point of data gathering can in some cases be a more practical or socially beneficial approach.
In IoT the role of an edge computer is to pre-process data and act before the data is passed on to the main server. This allows a faster, low latency response and minimal traffic between the cloud server processing and the Edge. However, a better understanding of the benefits of edge computing is required if it has to be beneficial for a number of outcomes.
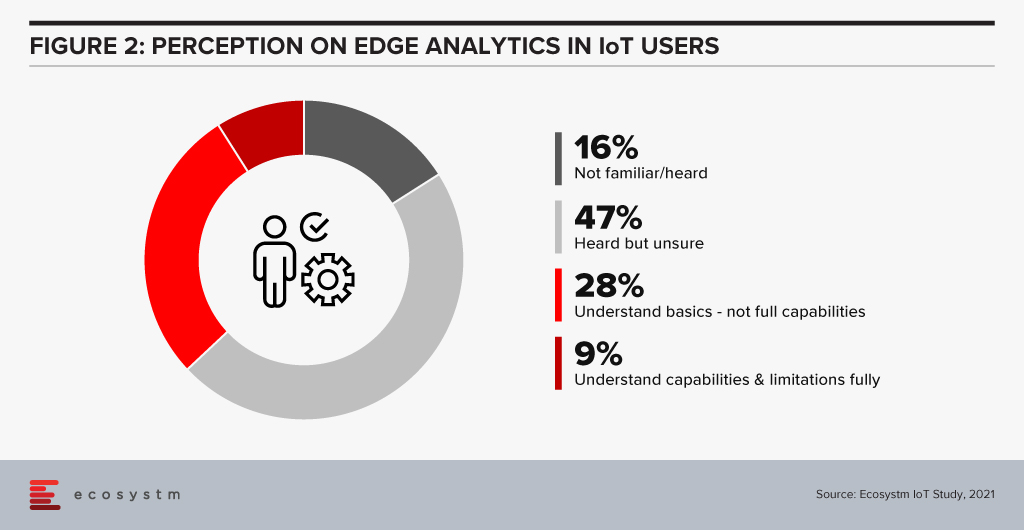

If we can get machine learning happening in the field, at the Edge, then we reduce the time lag and also create an extra trusted layer in unmanned production or automated utilities situations. This can create more trusted environments in terms of possible threats to public services.
What kind of examples of machine learning in the field can we see?
Healthcare
Health systems can improve hospital patient flow through machine learning (ML) at the Edge. ML offers predictive models to assist decision-makers with complex hospital patient flow information based on near real-time data.
For example, an academic medical centre created an ML pipeline that leveraged all its data – patient administration, EHR and clinical and claims data – to create learnings that could predict length of stay, emergency department (ED) arrival models, ED admissions, aggregate discharges, and total bed census. These predictive models proved effective as the medical centre reduced patient wait times and staff overtime and was able to demonstrate improved patient outcomes. And for a medical centre that use sensors to monitor patients and gather requests for medicine or assistance, Edge processing means keeping private healthcare data in-house rather than sending it off to cloud servers.
Retail
A retail store could use numerous cameras for self-checkout and inventory management and to monitor foot traffic. Such specific interaction details could slow down a network and can be replaced by an on-site Edge server with lower latency and a lower total cost. This is useful for standalone grocery pop-up sites such as in Sweden and Germany.
In Retail, k-nearest neighbours is often used in ML for abnormal activity analysis – this learning algorithm can also be used for visual pattern recognition used as part of retailers’ loss prevention tactics.
Summary
Working with the data locally on the Edge, creates reduced latency, reduced cloud usage and costs, independence from a network connection, more secure data, and increased data privacy.
Cloud and Edge computing that uses machine learning can together provide the best of both worlds: decentralised local storage, processing and reaction, and then uploading to the cloud, enabling additional insights, data backups (redundancy), and remote access.
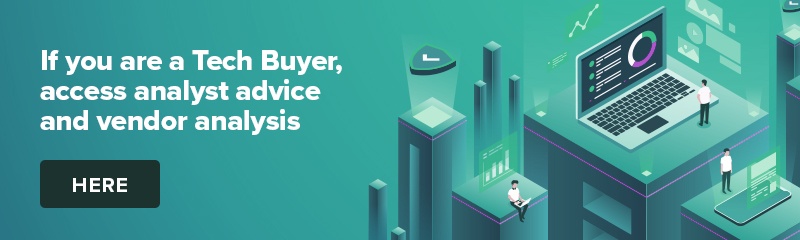
Last week I wrote about the need to remove hype from reality when it comes to AI. But what will ensure that your AI projects succeed?
It is quite obvious that success is determined by human aspects rather than technological factors. We have identified four key organisational actions that enable successful AI implementation at scale (Figure 1).
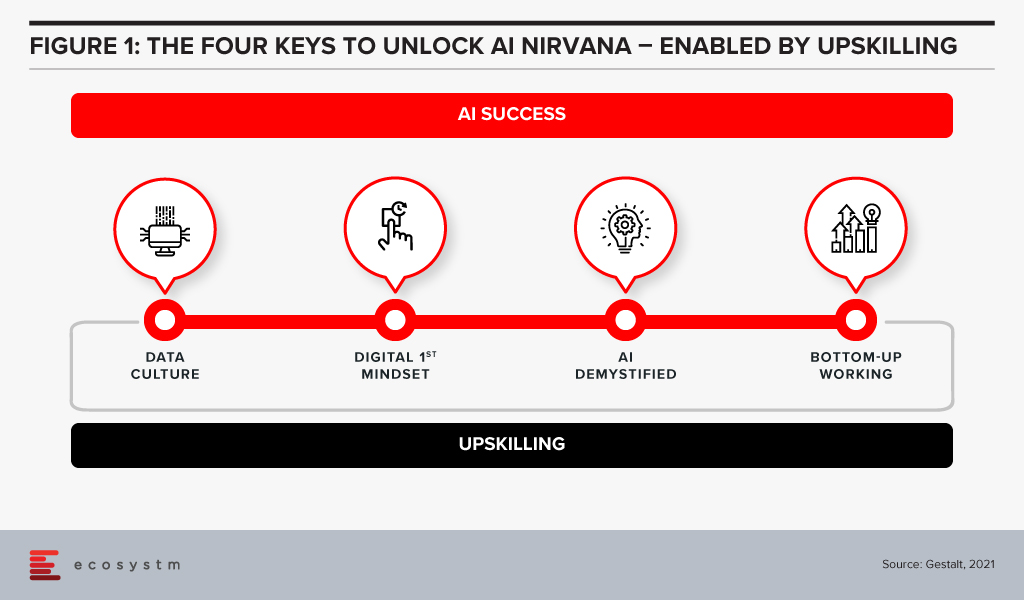
#1 Establish a Data Culture
The traditional focus for companies has been on ensuring access to good, clean data sets and the proper use of that data. Ecosystm research shows that only 28% of organisations focused on customer service, also focus on creating a data-driven organisational culture. But our experience has shown that culture is more critical than having the data. Does the organisation have a culture of using data to drive decisions? Does every level of the organisation understand and use data insights to do their day-to-day jobs? Is decision-making data-driven and decentralised, needing to be escalated only when there is ambiguity or need for strategic clarity? Do business teams push for new data sources when they are not able to get the insights they need?
Without this kind of culture, it may be possible to implement individual pieces of automation in a specific area or process, applying brute force to see it through. In order to transform the business and truly extract the power of AI, we advise organisations to build a culture of data-driven decision-making first. That organisational mindset, will make you capable implementing AI at scale. Focusing on changing the organisational culture will deliver greater returns than trying to implement piecemeal AI projects – even in the short to mid-term.
#2 Ingrain a Digital-First Mindset
Assuming a firm has passed the data culture hurdle, it needs to consider whether it has adopted a digital-first mindset. AI is one of many technologies that impact businesses, along with AR/VR, IoT, 5G, cloud and Blockchain to name a few. Today’s environment requires firms to be capable of utilising a variety of these technologies – often together – and possessing a workforce capable of using these digital tools.
A workforce with the digital-first mindset looks for a digital solution to problems wherever appropriate. They have a good understanding of digital technologies relevant to their space and understand key digital methodologies – such as Customer 360 to deliver a truly superior customer experience or Agile methodologies to successfully manage AI at scale.
AI needs business managers at the operational levels to work with IT or AI tech teams to pinpoint processes that are right for AI. They need to make an estimation based on historical data of what specific problems require an AI solution. This is enabled by the digital-first mindset.
#3 Demystify AI
The next step is to get business leaders, functional leaders, and business operational teams – not just those who work with AI – to acquire a basic understanding of AI.
They do not need to learn the intricacies of programming or how to create neural networks or anything nearly as technical in nature. However, all levels from the leadership down should have a solid understanding of what AI can do, the basics of how it works, how the process of training data results in improved outcomes and so on. They need to understand the continuous learning nature of AI solutions, getting better over time. While AI tools may recommend an answer, human insight is often needed to make a correct decision off this recommendation.

#4 Drive Implementation Bottom-Up
AI projects need alignment, objectives, strategy – and leadership and executive buy-in. But a very important aspect of an AI-driven organisation that is able to build scalable AI, is letting projects run bottom up.
As an example, a reputed Life Sciences company embarked on a multi-year AI project to improve productivity. They wanted to use NLP, Discovery, Cognitive Assist and ML to augment clinical proficiency of doctors and expected significant benefits in drug discovery and clinical trials by leveraging the immense dataset that was built over the last 20 years.
The company ran this like any other transformation project, with a central program management team taking the lead with the help of an AI Centre of Competency. These two teams developed a compelling business case, and identified initial pilots aligned with the long-term objectives of the program. However, after 18 months, they had very few tangible outcomes. Everyone including doctors, research scientists, technicians, and administrators, who participated in the program had their own interpretation of what AI was not able to do.
Discussion revealed that the doctors and researchers felt that they were training AI to replace themselves. Seeing a tool trying to mimic the same access and understanding of numerous documents baffled them at best. They were not ready to work with AI programs step-by-step to help AI tools learn and discover new insights.
At this point, we suggested approaching the project bottom-up – wherein the participating teams would decide specific projects to take up. This developed a culture where teams collaborated as well as competed with each other, to find new ways to use AI. Employees were shown a roadmap of how their jobs would be enhanced by offloading routine decisions to AI. They were shown that AI tools augment the employees’ cognitive capabilities and made them more effective.
The team working on critical trials found these tools extremely useful and were able to collaborate with other organisations specialising in similar trials. They created the metadata and used ML algorithms to discover new insights. Working bottom-up led to a very successful AI deployment.
We have seen time and again that while leadership may set the strategy and objectives, it is best to let the teams work bottom-up to come up with the projects to implement.
#5 Invest in Upskilling
The four “keys” are important to build an AI-powered, future-proof enterprise. They are all human related – and when they come together to work as a winning formula is when organisations invest in upskilling. Upskilling is the common glue and each factor requires specific kinds of upskilling (Figure 2).
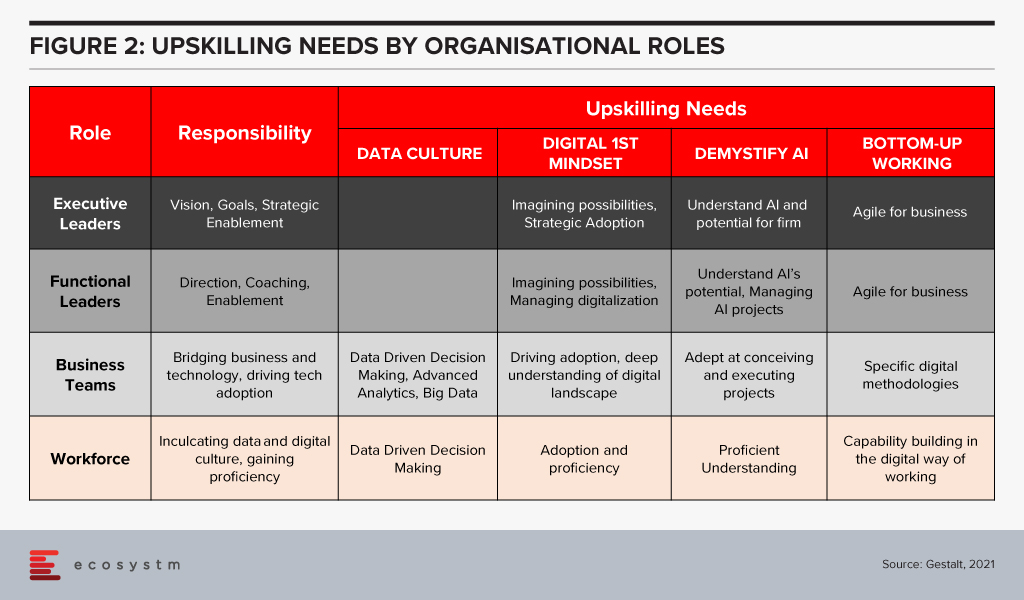
Upskilling needs vary by organisational level and the key being addressed. The bottom line is that upskilling is a universal requirement for driving AI at scale, successfully. And many organisations are realising it fast – Bosch and DBS Bank are some of the notable examples.
How much is your organisation invested in upskilling for AI implementation at scale? Share your stories in the comment box below.
Written with contributions from Ravi Pattamatta and Ratnesh Prasad
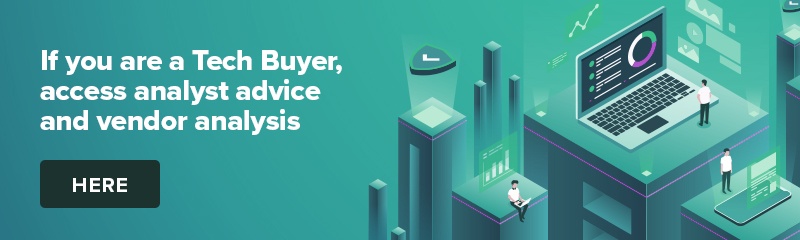
You know AI is the absolute next biggest thing. You know it is going to change our world!! It is the little technology trick start-ups use to disrupt industries. It enables crazy applications we have never thought of before! A few days ago, we were dazzled to learn of an AI app that promises to give one a credit rating score based on reading your face – essentially from just a photograph it can tell a prospective financier what the likelihood of your paying back the loan is!
Artificial Intelligence is real and has started becoming mainstream – chatbots using AI to answer queries are everywhere. AI is being used in stock trades, contact centre applications, bank loans processing, crop harvests, self-driving vehicles, and streaming entertainment. It is now part of boardroom discussions and strategic initiatives of CEOs. McKinsey predicts AI will add USD 13 trillion to the global economy by 2030.
Hype vs Reality
So much to like – but why then do we often find leaders shrugging their shoulders? Despite all the good news above there is also another side to AI. For all the green indicators, there are also some red flags (Figure 1). In fact, if one googles “Hype vs reality” the majority of the results returned are to do with AI!!!!
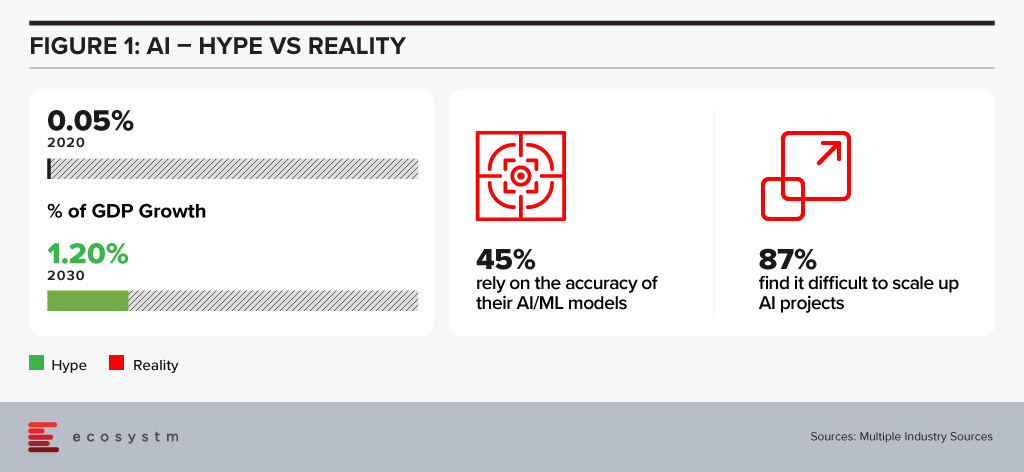
Our experience shows that broad swaths of executives are skeptical of AI. Leaders in a variety of businesses from large multinational banks, consumer packaged goods companies to appliance makers have privately expressed their disappointment at not being able to make AI work for them. They cannot bridge the gap between the AI hype and reality in their businesses.
The data available also bears this out – VentureBeat estimates that 87% of ML projects never make it into production. Ecosystm research suggests that only 7% of organisations have an AI centre of excellence (CoE) – while the remaining depend on ad-hoc implementations. There are several challenges that organisations face in procuring and implementing a successful AI solution – both technology and business (Figure 2).
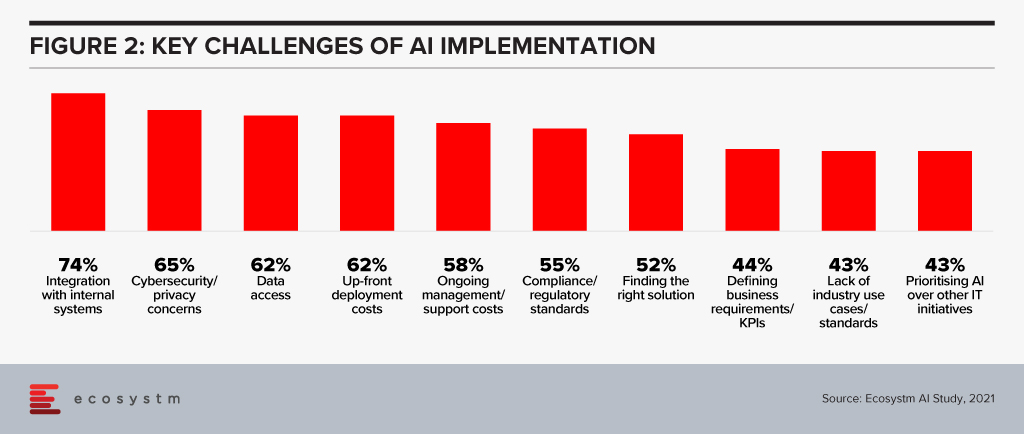
Visible Patterns Emerge from Successful AI Use Cases
This brings us to an interesting dichotomy – the reality of failed implementations versus the hype surrounding AI. Digital native companies or early adopters of AI form most of the success stories. Traditional companies find it tougher to embark on a successful AI journey. There have been studies that show a staggering gap in the ROI of AI projects between early adopters versus others, and the gulf between the high performers and the rest when using AI.
If we look back to figure 2 and analyse the challenges, we will see certain common themes – many of which are now commonplace wisdom, if not trite. Leadership alignment around AI strategy is the most common one. Getting clean data, aligning strategy with execution, and building the capabilities to use AI are all touted as critical requirements for successful execution. These themes all point to the insight that it is the human element that is more critical – not the technology.
As practitioners we have come across numerous examples of AI projects which go off-track because of human issues. Let’s take the example of an organisation that had enhancing call centre capabilities and capacity using RPA tools, as a key business mandate. There was strong leadership support and enthusiasm. It was clear that a large number of basic level tickets raised by the centre could be resolved using digital agents. This would result in substantial gains in customer experience, through faster ticket resolution and higher employee productivity – it was estimated to be above 30%. However, after two months of launching the pilot only a very small percentage of cases were identified for migration to digital agents.
Very soon, it became clear that these tools were being perceived as a replacement for human skills, rather than to augment their capabilities. The most vocal proponent of the initiative – the head of the customer experience team – became its critic, as he felt that the small savings were not worth the risk of higher agent turnover rates due to perceived job insecurity.
This was turned around by a three-day workshop focused on demonstrating how the job responsibility of agents could be enhanced as portions of their job got automated. The processes were redesigned to isolate parts which could be fully automated and to club non-automated components together driving more responsibility and discretion for agents. Once enhanced responsibility of the call centre staff was identified, managers felt more comfortable and were willing to support the initiative. In the end, the goals set at the start of the project were all met.
In my next blog I will share with you what we consider the winning formula for a successful AI deployment. In the meantime, share with us your AI stories – both of your challenges and successes.
Written with contributions from Ravi Pattamatta and Ratnesh Prasad
Since the start of this millennium, no region has transformed as much as Asia. There has been significant paradigm shifts in the region and the perception that innovation starts in the US or in Europe and percolates through to Asia after a time lag, has been shattered. Asia is constantly demonstrating how dynamic, and technology-focused it is. This is getting fueled by the impact of the growing middle class on consumerism and the spirit of innovation across the region. The region has also seen a surge in new and upcoming business leaders who are embracing change and looking beyond success to creating impact.
What is Driving Innovation in Asia?
The “If you ain’t got it, build it” attitude. One of the key drivers of this shift is the age of the average population in Asia. According to the UN the Asia Pacific region has nearly 60% of the world’s youth population (between the age of 17-24). With youth comes dynamism, a desire to change the world, and innovation. As this age group enters the workforce, they will transform their lives and the companies they work in. They are already showing a spirit of agility when it comes to solving challenges – they will build what they do not have.
The Need to enable Foundational Shifts. The younger generation is more aware of environmental, social and governance issues that the world continues to face. Many of the countries in the region are emerging economies, where these issues become more apparent. COVID-19 has also inculcated an empathy in people and they are thinking of future success in terms of impact. The desire to enable foundational shifts is giving direction to the transformation journey in the region. The wonderful new paradigm that is the Digital Economy allows us to cut across all segments; and technology and its advancements has immense potential to create a more sustainable and inclusive future for the world.
Realising the Power of Momentum. The pandemic has caused major disruptions in the region. But every crisis also presents an opportunity to perhaps re-imagine a brighter world through a digital lens. The other thing that the pandemic has done is made people and organisations realise that to succeed they need to be open to change – and that momentum is important. As organisations had to pivot fast, they realised what I have been saying for years – we shouldn’t “let perfect get in the way of better”. This adaptability and the readiness to fail fast and learn from the mistakes early for eventual success, is leading to faster and more agile transformation journeys.
Where are we seeing the most impact?
Industries are Transforming. There are industries such as Healthcare and Education that had to transform out of a necessity and urgency brought about by the COVID-19 pandemic. This has led to a greater impetus for change and optimism in these industries. These industries will continue to transform as governments focus significantly on creating “Social Safety Nets” and technology plays a key role in enabling critical services across Health, Education and Food Security. Then there are industries, such as the Financial Services and Retail, that had a strong customer focus and were well on their digital journeys before the pandemic. The pandemic boosted these efforts.
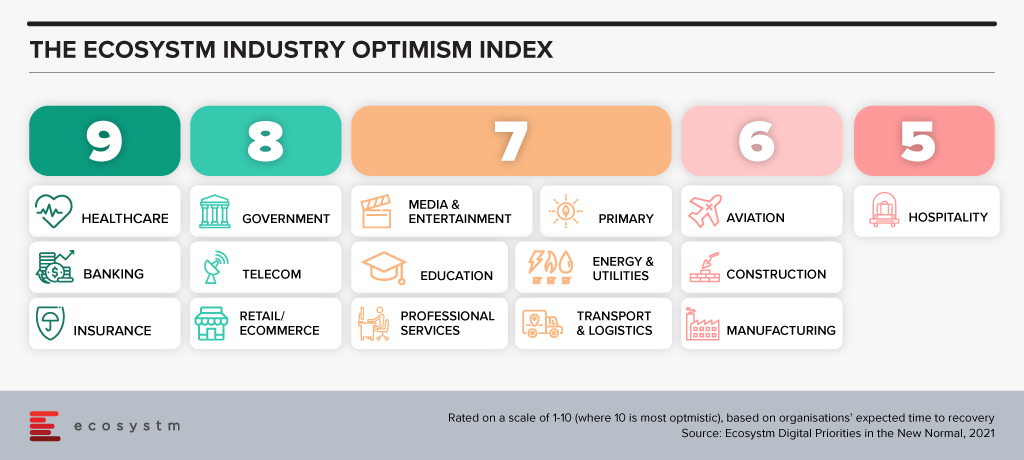
But these are not the only industries that are transforming. There are industries that have been impacted more than others. There are several instances of how organisations in these industries are demonstrating not only resilience but innovation. The Travel & Hospitality industry has had several such instances. As business models evolve the industry will see significant changes in digital channels to market, booking engines, corporate service offerings and others, as the overall Digital Strategy is overhauled.
Technologies are Evolving. Organisations depended on their tech partners to help them make the fast pivot required to survive and succeed in the last year – and tech companies have not disappointed. They have evolved their capabilities and continue to offer innovative solutions that can solve many of the ongoing business challenges that organisations face in their innovation journey. More and more technologies such as AI, machine learning, robotics, and digital twins are getting enmeshed together to offer better options for business growth, process efficiency and customer engagement. And the 5G rollouts will only accelerate that. The initial benefits being realized from early adoption of 5G has been for consumers. But there is a much bigger impact that is waiting to be realised as 5G empowers governments and businesses to make critical decisions at the edge.
Tech Start-ups are Flourishing. There are immense opportunities for technology start-ups to grow their market presence through innovative products and services. To succeed these companies need to have a strong investment roadmap; maintain a strong focus on customer engagement; and offer technology solutions that can fulfil the global needs of their customers. Technologies that promote efficiency and eliminate mundane tasks for humans are the need of the hour. However, as the reliance on technology-led transformation increases, tech vendors are becoming acutely aware that they cannot be best-in-class across the different technologies that an organisation will require to transform. Here is where having a robust partner ecosystem helps. Partnerships are bringing innovation to scale in Asia.
We can expect Asia to emerge as a powerhouse as businesses continue to innovate, embed technology in their product and service offerings – and as tech start-ups continue to support their innovation journeys.
Ecosystm CEO Amit Gupta gets face to face with Garrett Ilg, President Asia Pacific & Japan, Oracle to discuss the rise of the Asia Digital economies, the impact of the growing middle class on consumerism and the spirit of innovation across the region.
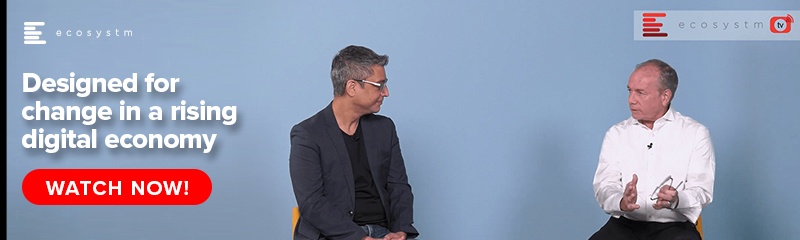
In this blog, our guest author Shameek Kundu talks about the importance of making AI/ machine learning models reliable and safe. “Getting data and algorithms right has always been important, particularly in regulated industries such as banking, insurance, life sciences and healthcare. But the bar is much higher now: more data, from more sources, in more formats, feeding more algorithms, with higher stakes.”
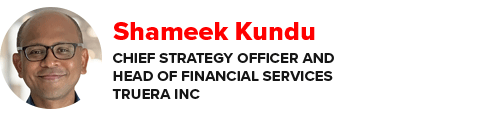
Building trust in algorithms is essential. Not (just) because regulators want it, but because it is good for customers and business. The good news is that with the right approach and tooling, it is also achievable.
Getting data and algorithms right has always been important, particularly in regulated industries such as banking, insurance, life sciences and healthcare. But the bar is much higher now: more data, from more sources, in more formats, feeding more algorithms, with higher stakes. With the increased use of Artificial Intelligence/ Machine Learning (AI/ML), today’s algorithms are also more powerful and difficult to understand.
A false dichotomy
At this point in the conversation, I get one of two reactions. One is of distrust in AI/ML and a belief that it should have little role to play in regulated industries. Another is of nonchalance; after all, most of us feel comfortable using ‘black-boxes’ (e.g., airplanes, smartphones) in our daily lives without being able to explain how they work. Why hold AI/ML to special standards?
Both make valid points. But the skeptics miss out on the very real opportunity cost of not using AI/ML – whether it is living with historical biases in human decision-making or simply not being able to do things that are too complex for a human to do, at scale. For example, the use of alternative data and AI/ML has helped bring financial services to many who have never had access before.
On the other hand, cheerleaders for unfettered use of AI/ML might be overlooking the fact that a human being (often with a limited understanding of AI/ML) is always accountable for and/ or impacted by the algorithm. And fairly or otherwise, AI/ML models do elicit concerns around their opacity – among regulators, senior managers, customers and the broader society. In many situations, ensuring that the human can understand the basis of algorithmic decisions is a necessity, not a luxury.
A way forward
Reconciling these seemingly conflicting requirements is possible. But it requires serious commitment from business and data/ analytics leaders – not (just) because regulators demand it, but because it is good for their customers and their business, and the only way to start capturing the full value from AI/ML.
1. ‘Heart’, not just ‘Head’
It is relatively easy to get people excited about experimenting with AI/ML. But when it comes to actually trusting the model to make decisions for us, we humans are likely to put up our defences. Convincing a loan approver, insurance under-writer, medical doctor or front-line sales-person to trust an AI/ML model – over their own knowledge or intuition – is as much about the ‘heart’ as the ‘head’. Helping them understand, on their own terms, how the alternative is at least as good as their current way of doing things, is crucial.
2. A Broad Church
Even in industries/ organisations that recognise the importance of governing AI/ML, there is a tendency to define it narrowly. For example, in Financial Services, one might argue that “an ML model is just another model” and expect existing Model Risk teams to deal with any incremental risks from AI/ML.
There are two issues with this approach:
First, AI/ML models tend to require a greater focus on model quality (e.g., with respect to stability, overfitting and unjust bias) than their traditional alternatives. The pace at which such models are expected to be introduced and re-calibrated is also much higher, stretching traditional model risk management approaches.
Second, poorly designed AI/ML models create second order risks. While not unique to AI/ML, these risks become accentuated due to model complexity, greater dependence on (high-volume, often non-traditional) data and ubiquitous adoption. One example is poor customer experience (e.g., badly communicated decisions) and unfair treatment (e.g., unfair denial of service, discrimination, misselling, inappropriate investment recommendations). Another is around the stability, integrity and competitiveness of financial markets (e.g., unintended collusion with other market players). Obligations under data privacy, sovereignty and security requirements could also become more challenging.
The only way to respond holistically is to bring together a broad coalition – of data managers and scientists, technologists, specialists from risk, compliance, operations and cyber-security, and business leaders.
3. Automate, Automate, Automate
A key driver for the adoption and effectiveness of AI/ ML is scalability. The techniques used to manage traditional models are often inadequate in the face of more data-hungry, widely used and rapidly refreshed AI/ML models. Whether it is during the development and testing phase, formal assessment/ validation or ongoing post-production monitoring, it is impossible to govern AI/ML at scale using manual processes alone.
o, somewhat counter-intuitively, we need more automation if we are to build and sustain trust in AI/ML. As humans are accountable for the outcomes of AI/ ML models, we can only be ‘in charge’ if we have the tools to provide us reliable intelligence on them – before and after they go into production. As the recent experience with model performance during COVID-19 suggests, maintaining trust in AI/ML models is an ongoing task.
***
I have heard people say “AI is too important to be left to the experts”. Perhaps. But I am yet to come across an AI/ML practitioner who is not keenly aware of the importance of making their models reliable and safe. What I have noticed is that they often lack suitable tools – to support them in analysing and monitoring models, and to enable conversations to build trust with stakeholders. If AI is to be adopted at scale, that must change.
Shameek Kundu is Chief Strategy Officer and Head of Financial Services at TruEra Inc. TruEra helps enterprises analyse, improve and monitor quality of machine
Have you evaluated the tech areas on your AI requirements? Get access to AI insights and key industry trends from our AI research.
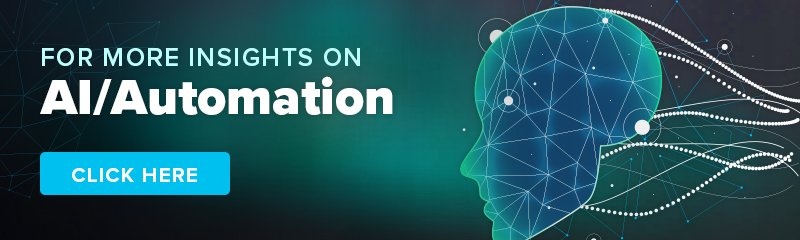