As tech providers such as Microsoft enhance their capabilities and products, they will impact business processes and technology skills, and influence other tech providers to reshape their product and service offerings. Microsoft recently organised briefing sessions in Sydney and Singapore, to present their future roadmap, with a focus on their AI capabilities.
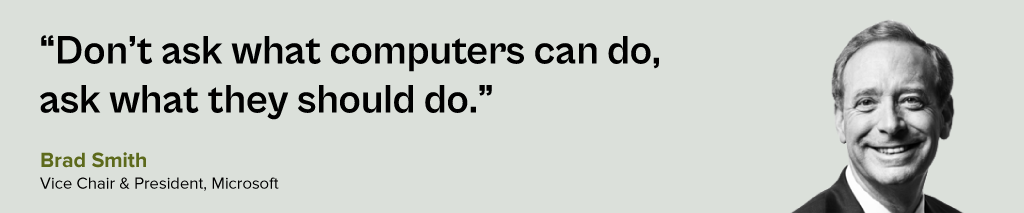
Ecosystm Advisors Achim Granzen, Peter Carr, and Tim Sheedy provide insights on Microsoft’s recent announcements and messaging.

Click here to download Ecosystm VendorSphere: Microsoft’s AI Vision – Initiatives & Impact
Ecosystm Question: What are your thoughts on Microsoft Copilot?
Tim Sheedy. The future of GenAI will not be about single LLMs getting bigger and better – it will be about the use of multiple large and small language models working together to solve specific challenges. It is wasteful to use a large and complex LLM to solve a problem that is simpler. Getting these models to work together will be key to solving industry and use case specific business and customer challenges in the future. Microsoft is already doing this with Microsoft 365 Copilot.
Achim Granzen. Microsoft’s Copilot – a shrink-wrapped GenAI tool based on OpenAI – has become a mainstream product. Microsoft has made it available to their enterprise clients in multiple ways: for personal productivity in Microsoft 365, for enterprise applications in Dynamics 365, for developers in Github and Copilot Studio, and to partners to integrate Copilot into their applications suites (E.g. Amdocs’ Customer Engagement Platform).
Ecosystm Question: How, in your opinion, is the Microsoft Copilot a game changer?
Microsoft’s Customer Copyright Commitment, initially launched as Copilot Copyright Commitment, is the true game changer.
Achim Granzen. It safeguards Copilot users from potential copyright infringement lawsuits related to data used for algorithm training or output results. In November 2023, Microsoft expanded its scope to cover commercial usage of their OpenAI interface as well.
This move not only protects commercial clients using Microsoft’s GenAI products but also extends to any GenAI solutions built by their clients. This initiative significantly reduces a key risk associated with GenAI adoption, outlined in the product terms and conditions.
However, compliance with a set of Required Mitigations and Codes of Conduct is necessary for clients to benefit from this commitment, aligning with responsible AI guidelines and best practices.
Ecosystm Question: Where will organisations need most help on their AI journeys?
Peter Carr. Unfortunately, there is no playbook for AI.
- The path to integrating AI into business strategies and operations lacks a one-size-fits-all guide. Organisations will have to navigate uncharted territories for the time being. This means experimenting with AI applications and learning from successes and failures. This exploratory approach is crucial for leveraging AI’s potential while adapting to unique organisational challenges and opportunities. So, companies that are better at agile innovation will do better in the short term.
- The effectiveness of AI is deeply tied to the availability and quality of connected data. AI systems require extensive datasets to learn and make informed decisions. Ensuring data is accessible, clean, and integrated is fundamental for AI to accurately analyse trends, predict outcomes, and drive intelligent automation across various applications.
Ecosystm Question: What advice would you give organisations adopting AI?
Tim Sheedy. It is all about opportunities and responsibility.
- There is a strong need for responsible AI – at a global level, at a country level, at an industry level and at an organisational level. Microsoft (and other AI leaders) are helping to create responsible AI systems that are fair, reliable, safe, private, secure, and inclusive. There is still a long way to go, but these capabilities do not completely indemnify users of AI. They still have a responsibility to set guardrails in their own businesses about the use and opportunities for AI.
- AI and hybrid work are often discussed as different trends in the market, with different solution sets. But in reality, they are deeply linked. AI can help enhance and improve hybrid work in businesses – and is a great opportunity to demonstrate the value of AI and tools such as Copilot.
Ecosystm Question: What should Microsoft focus on?
Tim Sheedy. Microsoft faces a challenge in educating the market about adopting AI, especially Copilot. They need to educate business, IT, and AI users on embracing AI effectively. Additionally, they must educate existing partners and find new AI partners to drive change in their client base. Success in the race for knowledge workers requires not only being first but also helping users maximise solutions. Customers have limited visibility of Copilot’s capabilities, today. Improving customer upskilling and enhancing tools to prompt users to leverage capabilities will contribute to Microsoft’s (or their competitors’) success in dominating the AI tool market.
Peter Carr. Grassroots businesses form the economic foundation of the Asia Pacific economies. Typically, these businesses do not engage with global SIs (GSIs), which drive Microsoft’s new service offerings. This leads to an adoption gap in the sector that could benefit most from operational efficiencies. To bridge this gap, Microsoft must empower non-GSI partners and managed service providers (MSPs) at the local and regional levels. They won’t achieve their goal of democratising AI, unless they do. Microsoft has the potential to advance AI technology while ensuring fair and widespread adoption.
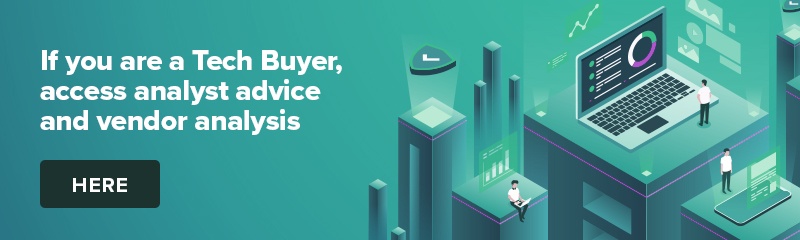
I have spent many years analysing the mobile and end-user computing markets. Going all the way back to 1995 where I was part of a Desktop PC research team, to running the European wireless and mobile comms practice, to my time at 3 Mobile in Australia and many years after, helping clients with their end-user computing strategies. From the birth of mobile data services (GPRS, WAP, and so on to 3G, 4G and 5G), from simple phones to powerful foldable devices, from desktop computers to a complex array of mobile computing devices to meet the many and varied employee needs. I am always looking for the “next big thing” – and there have been some significant milestones – Palm devices, Blackberries, the iPhone, Android, foldables, wearables, smaller, thinner, faster, more powerful laptops.
But over the past few years, innovation in this space has tailed off. Outside of the foldable space (which is already four years old), the major benefits of new devices are faster processors, brighter screens, and better cameras. I review a lot of great computers too (like many of the recent Surface devices) – and while they are continuously improving, not much has got my clients or me “excited” over the past few years (outside of some of the very cool accessibility initiatives).
The Force of AI
But this is all about to change. Devices are going to get smarter based on their data ecosystem, the cloud, and AI-specific local processing power. To be honest, this has been happening for some time – but most of the “magic” has been invisible to us. It happened when cameras took multiple shots and selected the best one; it happened when pixels were sharpened and images got brighter, better, and more attractive; it happened when digital assistants were called upon to answer questions and provide context.
Microsoft, among others, are about to make AI smarts more front and centre of the experience – Windows Copilot will add a smart assistant that can not only advise but execute on advice. It will help employees improve their focus and productivity, summarise documents and long chat threads, select music, distribute content to the right audience, and find connections. Added to Microsoft 365 Copilot it will help knowledge workers spend less time searching and reading – and more time doing and improving.
The greater integration of public and personal data with “intent insights” will also play out on our mobile devices. We are likely to see the emergence of the much-promised “integrated app”– one that can take on many of the tasks that we currently undertake across multiple applications, mobile websites, and sometimes even multiple devices. This will initially be through the use of public LLMs like Bard and ChatGPT, but as more custom, private models emerge they will serve very specific functions.
Focused AI Chips will Drive New Device Wars
In parallel to these developments, we expect the emergence of very specific AI processors that are paired to very specific AI capabilities. As local processing power becomes a necessity for some AI algorithms, the broad CPUs – and even the AI-focused ones (like Google’s Tensor Processor) – will need to be complemented by specific chips that serve specific AI functions. These chips will perform the processing more efficiently – preserving the battery and improving the user experience.
While this will be a longer-term trend, it is likely to significantly change the game for what can be achieved locally on a device – enabling capabilities that are not in the realm of imagination today. They will also spur a new wave of device competition and innovation – with a greater desire to be on the “latest and greatest” devices than we see today!
So, while the levels of device innovation have flattened, AI-driven software and chipset innovation will see current and future devices enable new levels of employee productivity and consumer capability. The focus in 2023 and beyond needs to be less on the hardware announcements and more on the platforms and tools. End-user computing strategies need to be refreshed with a new perspective around intent and intelligence. The persona-based strategies of the past have to be changed in a world where form factors and processing power are less relevant than outcomes and insights.
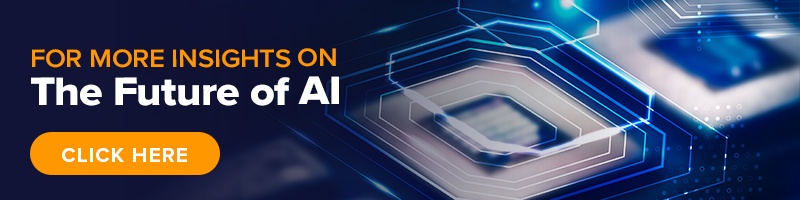
It is not hyperbole to state that AI is on the cusp of having significant implications on society, business, economies, governments, individuals, cultures, politics, the arts, manufacturing, customer experience… I think you get the idea! We cannot understate the impact that AI will have on society. In times gone by, businesses tested ideas, new products, or services with small customer segments before they went live. But with AI we are all part of this experiment on the impacts of AI on society – its benefits, use cases, weaknesses, and threats.
What seemed preposterous just six months ago is not only possible but EASY! Do you want a virtual version of yourself, a friend, your CEO, or your deceased family member? Sure – just feed the data. Will succession planning be more about recording all conversations and interactions with an executive so their avatar can make the decisions when they leave? Why not? How about you turn the thousands of hours of recorded customer conversations with your contact centre team into a virtual contact centre team? Your head of product can present in multiple countries in multiple languages, tailored to the customer segments, industries, geographies, or business needs at the same moment.
AI has the potential to create digital clones of your employees, it can spread fake news as easily as real news, it can be used for deception as easily as for benefit. Is your organisation prepared for the social, personal, cultural, and emotional impacts of AI? Do you know how AI will evolve in your organisation?
When we focus on the future of AI, we often interview AI leaders, business leaders, futurists, and analysts. I haven’t seen enough focus on psychologists, sociologists, historians, academics, counselors, or even regulators! The Internet and social media changed the world more than we ever imagined – at this stage, it looks like these two were just a rehearsal for the real show – Artificial Intelligence.
Lack of Government or Industry Regulation Means You Need to Self-Regulate
These rapid developments – and the notable silence from governments, lawmakers, and regulators – make the requirement for an AI Ethics Policy for your organisation urgent! Even if you have one, it probably needs updating, as the scenarios that AI can operate within are growing and changing literally every day.
- For example, your customer service team might want to create a virtual customer service agent from a real person. What is the policy on this? How will it impact the person?
- Your marketing team might be using ChatGPT or Bard for content creation. Do you have a policy specifically for the creation and use of content using assets your business does not own?
- What data is acceptable to be ingested by a public Large Language Model (LLM). Are are you governing data at creation and publishing to ensure these policies are met?
- With the impending public launch of Microsoft’s Co-Pilot AI service, what data can be ingested by Co-Pilot? How are you governing the distribution of the insights that come out of that capability?
If policies are not put in place, data tagged, staff trained, before using a tool such as Co-Pilot, your business will be likely to break some privacy or employment laws – on the very first day!
What do the LLMs Say About AI Ethics Policies?
So where do you go when looking for an AI Ethics policy? ChatGPT and Bard of course! I asked the two for a modern AI Ethics policy.
You can read what they generated in the graphic below.
I personally prefer the ChatGPT4 version as it is more prescriptive. At the same time, I would argue that MOST of the AI tools that your business has access to today don’t meet all of these principles. And while they are tools and the ethics should dictate the way the tools are used, with AI you cannot always separate the process and outcome from the tool.
For example, a tool that is inherently designed to learn an employee’s character, style, or mannerisms cannot be unbiased if it is based on a biased opinion (and humans have biases!).
LLMs take data, content, and insights created by others, and give it to their customers to reuse. Are you happy with your website being used as a tool to train a startup on the opportunities in the markets and customers you serve?
By making content public, you acknowledge the risk of others using it. But at least they visited your website or app to consume it. Not anymore…
A Policy is Useless if it Sits on a Shelf
Your AI ethics policy needs to be more than a published document. It should be the beginning of a conversation across the entire organisation about the use of AI. Your employees need to be trained in the policy. It needs to be part of the culture of the business – particularly as low and no-code capabilities push these AI tools, practices, and capabilities into the hands of many of your employees.
Nearly every business leader I interview mentions that their organisation is an “intelligent, data-led, business.” What is the role of AI in driving this intelligent business? If being data-driven and analytical is in the DNA of your organisation, soon AI will also be at the heart of your business. You might think you can delay your investments to get it right – but your competitors may be ahead of you.
So, as you jump head-first into the AI pool, start to create, improve and/or socialise your AI Ethics Policy. It should guide your investments, protect your brand, empower your employees, and keep your business resilient and compliant with legacy and new legislation and regulations.
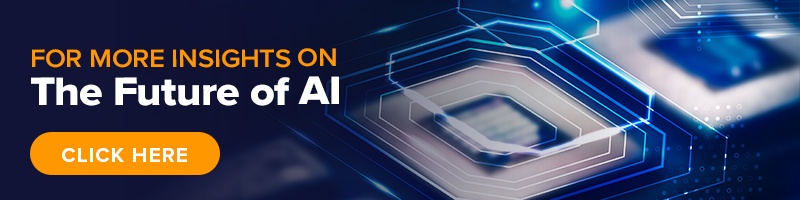