A lot has been written and spoken about DeepSeek since the release of their R1 model in January. Soon after, Alibaba, Mistral AI, and Ai2 released their own updated models, and we have seen Manus AI being touted as the next big thing to follow.
DeepSeek’s lower-cost approach to creating its model – using reinforcement learning, the mixture-of-experts architecture, multi-token prediction, group relative policy optimisation, and other innovations – has driven down the cost of LLM development. These methods are likely to be adopted by other models and are already being used today.
While the cost of AI is a challenge, it’s not the biggest for most organisations. In fact, few GenAI initiatives fail solely due to cost.
The reality is that many hurdles still stand in the way of organisations’ GenAI initiatives, which need to be addressed before even considering the business case – and the cost – of the GenAI model.
Real Barriers to GenAI
• Data. The lifeblood of any AI model is the data it’s fed. Clean, well-managed data yields great results, while dirty, incomplete data leads to poor outcomes. Even with RAG, the quality of input data dictates the quality of results. Many organisations I work with are still discovering what data they have – let alone cleaning and classifying it. Only a handful in Australia can confidently say their data is fully managed, governed, and AI-ready. This doesn’t mean GenAI initiatives must wait for perfect data, but it does explain why Agentic AI is set to boom – focusing on single applications and defined datasets.
• Infrastructure. Not every business can or will move data to the public cloud – many still require on-premises infrastructure optimised for AI. Some companies are building their own environments, but this often adds significant complexity. To address this, system manufacturers are offering easy-to-manage, pre-built private cloud AI solutions that reduce the effort of in-house AI infrastructure development. However, adoption will take time, and some solutions will need to be scaled down in cost and capacity to be viable for smaller enterprises in Asia Pacific.
• Process Change. AI algorithms are designed to improve business outcomes – whether by increasing profitability, reducing customer churn, streamlining processes, cutting costs, or enhancing insights. However, once an algorithm is implemented, changes will be required. These can range from minor contact centre adjustments to major warehouse overhauls. Change is challenging – especially when pre-coded ERP or CRM processes need modification, which can take years. Companies like ServiceNow and SS&C Blue Prism are simplifying AI-driven process changes, but these updates still require documentation and training.
• AI Skills. While IT teams are actively upskilling in data, analytics, development, security, and governance, AI opportunities are often identified by business units outside of IT. Organisations must improve their “AI Quotient” – a core understanding of AI’s benefits, opportunities, and best applications. Broad upskilling across leadership and the wider business will accelerate AI adoption and increase the success rate of AI pilots, ensuring the right people guide investments from the start.
• AI Governance. Trust is the key to long-term AI adoption and success. Being able to use AI to do the “right things” for customers, employees, and the organisation will ultimately drive the success of GenAI initiatives. Many AI pilots fail due to user distrust – whether in the quality of the initial data or in AI-driven outcomes they perceive as unethical for certain stakeholders. For example, an AI model that pushes customers toward higher-priced products or services, regardless of their actual needs, may yield short-term financial gains but will ultimately lose to ethical competitors who prioritise customer trust and satisfaction. Some AI providers, like IBM and Microsoft, are prioritising AI ethics by offering tools and platforms that embed ethical principles into AI operations, ensuring long-term success for customers who adopt responsible AI practices.
GenAI and Agentic AI initiatives are far from becoming standard business practice. Given the current economic and political uncertainty, many organisations will limit unbudgeted spending until markets stabilise. However, technology and business leaders should proactively address the key barriers slowing AI adoption within their organisations. As more AI platforms adopt the innovations that helped DeepSeek reduce model development costs, the economic hurdles to GenAI will become easier to overcome.
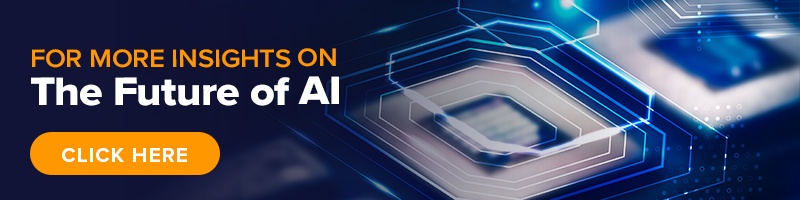
2024 was a pivotal year for cryptocurrency, driven by substantial institutional adoption. The approval and launch of spot Bitcoin and Ethereum ETFs marked a turning point, solidifying digital assets as institutional-grade. Bitcoin has evolved into a macro asset, and the ecosystem’s outlook remains robust, with signs of regulatory clarity in the US and increasing broad adoption. High-quality research from firms like VanEck, Messari, Pantera, Galaxy, and a16Z, has further strengthened my conviction.
As a “normie in web3,” my perspective comes from connecting the dots through research, not from early airdrops or token swaps. While the speculative frenzy, rug pulls, and scams at the “casino” end are off-putting, the real potential on the “computer” side of blockchains is thrilling. Events like TOKEN2049 in Dubai and Singapore highlight the ecosystem’s energy, with hundreds of side events now central to the experience.
As the web3 ecosystem evolves, new blockchains, roll-ups, and protocols vie for attention. With 60 million unique wallets in the on-chain economy, adoption is set to expand beyond this base. DeFi transaction volumes have surpassed USD 200B/month, yet the ecosystem remains in its early stages, with only 10 million users.
Despite current fragmentation, the future looks promising. Themes like tokenising real-world assets, decentralised public infrastructure, stablecoins for instant payments, and the convergence of AI and blockchain could reshape finance, identity, infrastructure, and computing. Web3 holds transformative potential, even if not in marketing terms like “unstable” coins or “unreal world assets.”
The Decentralisation Paradox of Web3
Decentralisation may have been a core tenet of web3 at the onset but is also seen as a constraint to scaling or improving user experience in certain instances. I always saw decentralisation as a progressive spectrum and not a binary. It is, however, a difficult north star to maintain, as scaling becomes an actual human coordination challenge.
In Blockchains. We have seen this phenomenon manifest with the Ethereum ecosystem in particular. Of the fifty-plus roll-ups listed on L2 Beat, only Arbitrum and OP Mainnet have progressed beyond Stage 0, with many still not posting fraud proofs to L1. Some high-performance L1s and L2s have deprioritised decentralisation in favour of scaling and UX. Whether this trade-off leads to greater vulnerability or stronger product-market fit remains to be seen – most users care more about performance than underlying technology. In 2025, we’ll likely witness the quiet demise of as many blockchains as new ones emerge.
In Finance. On the institutional side, some aspects of high-value transactions in traditional finance or TradFi, such as custody, need trusted intermediaries to minimise counterparty risk. For web3 to scale beyond the 60-million-odd wallets that participate in the on-chain economy today, we need protocols that marry blockchains’ efficiency, composability, and programmability with the trusted identity and verifiability of the regulated financial systems. While “CeDeFi” or Centralised Decentralised Finance might sound ironical to most in the crypto native world, I expect much more convergence with institutions launching tokenisation projects on public blockchains, including Ethereum and Solana. I like underway pilots, such as one by Chainlink with SWIFT, facilitating off-chain cash settlements for tokenised funds. Some of these projects will find strong traction and scale coupled with regulatory blessings in certain progressive jurisdictions in 2025.
In Infrastructure. While decentralised compute clusters for post-training and inference from the likes of io.net can lower the cost of computing for start-ups, scaling decentralised AI LLMs to make them competitive against LLMs from centralised entities like OpenAI is a nearly impossible order. New metas such as decentralised science or DeSci are exciting because they open the possibility of fast-tracking fundamental research and drug discovery.
Looking Back at 2024: What I Found Exciting
ETFs. BlackRock’s IBIT ETF became the fastest to reach USD 3 billion in AUM within 30 days and scaled to USD 40 billion in 200 days. The institutional landscape now goes beyond traditional ETFs, with major financial institutions expanding digital asset capabilities across custody, market access, and retail integration. These include institutional-grade custody from Standard Chartered and Nomura, market access from Goldman Sachs, and retail integration from fintechs such as Revolut.
Stablecoins. Stablecoin usage beyond trading has continued to grow at a healthy clip, emerging as a real killer use case in payments. Transaction volumes rose from USD 10T to USD 20T in a year, and yes, that is a trillion with a “t”! The current market capitalisation of stablecoins is approximately USD 201.5 billion, slated to triple in 2025, with Tether’s USDT at over 67% market share. We might see new fiat-backed stablecoins being launched this year, such as Ethena’s yield-bearing stablecoin, but I don’t expect USDT’s dominance to change.
RWAs. Even though stablecoins represent 97% of real-world assets on-chain and the dollar value of all other types of assets is still insignificant, the potential market for asset tokenisation is still a staggering USD 1.4T, and with regulatory clarity, even if RWAs on-chain were to quadruple, the resulting USD 50B will be a sliver of the overall opportunity. We can expect more projects in asset classes such as private credit – rwa.xyz is a great dashboard to watch this space.
DePIN. Decentralised public infrastructure across wireless, energy, compute, sensors, identity, and logistics reached a USD 50B market cap and USD 500M in ARR. Key developments include the emergence of AI as a major driver of DePIN adoption, the maturation of supply-side growth playbooks, and the shift in focus toward demand-side monetisation. More than 13 million devices globally contribute to DePINs daily, demonstrating successful supply-side scaling. Notable projects include:
- Helium Mobile: Adding 100k+ subscribers and diversifying revenue streams.
- AI Integration: Bittensor leading decentralised AI with successful subnets.
- Energy DePINs: Glow and Daylight addressing challenges in distributed energy systems.
- Identity Verification: World (formerly Worldcoin) achieving 20 million verified identities.
These trends indicate significant advancements in the web3 ecosystem, and the continued evolution of blockchain technologies and their applications in finance, infrastructure, and beyond holds immense promise for 2025 and beyond.
In my next Ecosystm Insights, I’ll present the trends in 2025 that I am excited about. Watch this space!
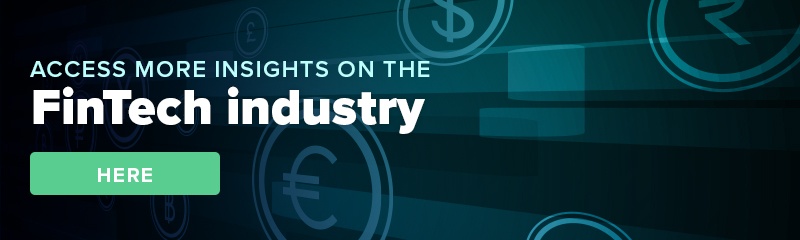
AI has already had a significant impact on the tech industry, rapidly evolving software development, data analysis, and automation. However, its potential extends into all industries – from the precision of agriculture to the intricacies of life sciences research, and the enhanced customer experiences across multiple sectors.
While we have seen the widespread adoption of AI-powered productivity tools, 2025 promises a bigger transformation. Organisations across industries will shift focus from mere innovation to quantifiable value. In sectors where AI has already shown early success, businesses will aim to scale these applications to directly impact their revenue and profitability. In others, it will accelerate research, leading to groundbreaking discoveries and innovations in the years to come. Regardless of the specific industry, one thing is certain: AI will be a driving force, reshaping business models and competitive landscapes.
Ecosystm analysts Alan Hesketh, Clay Miller, Peter Carr, Sash Mukherjee, and Steve Shipley present the top trends shaping key industries in 2025.
Click here to download ‘AI’s Impact on Industry in 2025’ as a PDF
1. GenAI Virtual Agents Will Reshape Public Sector Efficiency
Operating within highly structured, compliance-driven environments, public sector organisations are well-positioned to benefit from GenAI Agents.
These agents excel when powered LLMs tailored to sector-specific needs, informed by documented legislation, regulations, and policies. The result will be significant improvements in how governments manage rising service demands and enhance citizen interactions. From automating routine enquiries to supporting complex administrative processes, GenAI Virtual Agents will enable public sector to streamline operations without compromising compliance. Crucially, these innovations will also address jurisdictional labour and regulatory requirements, ensuring ethical and legal adherence. As GenAI technology matures, it will reshape public service delivery by combining scalability, precision, and responsiveness.
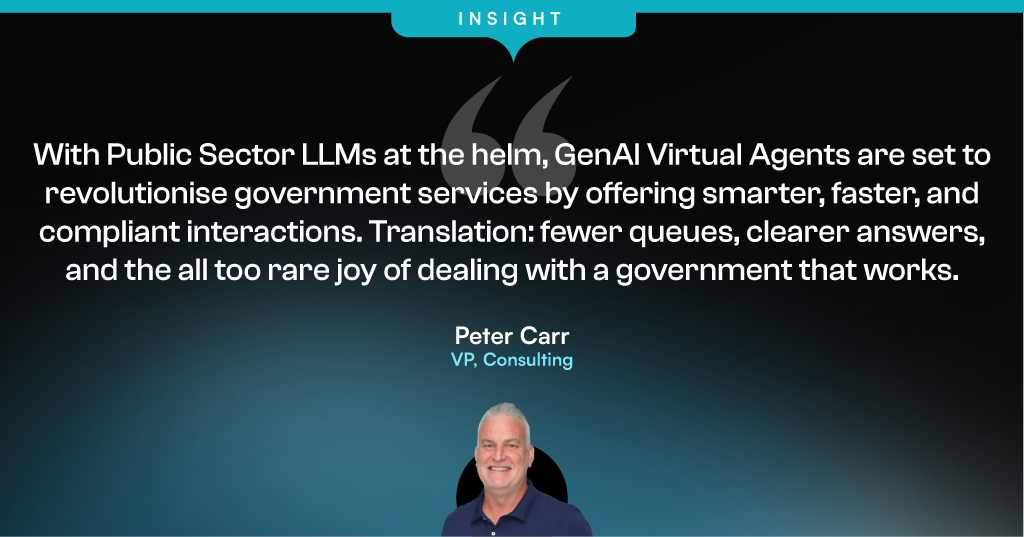
2. Healthcare Will Lead in Innovation; Lag in Adoption
In 2025, healthcare will undergo transformative innovations driven by advancements in AI, remote medicine, and biotechnology. Innovations will include personalised healthcare driven by real-time data for tailored wellness plans and preventive care, predictive AI tackling global challenges like aging populations and pandemics, virtual healthcare tools like VR therapy and chatbots enhancing accessibility, and breakthroughs in nanomedicine, digital therapeutics, and next-generation genomic sequencing.
Startups and innovators will often lead the way, driven by a desire to make an impact.
However, governments will lack the will to embrace these technologies. After significant spending on crisis management, healthcare ministries will likely hesitate to commit to fresh large-scale investments.
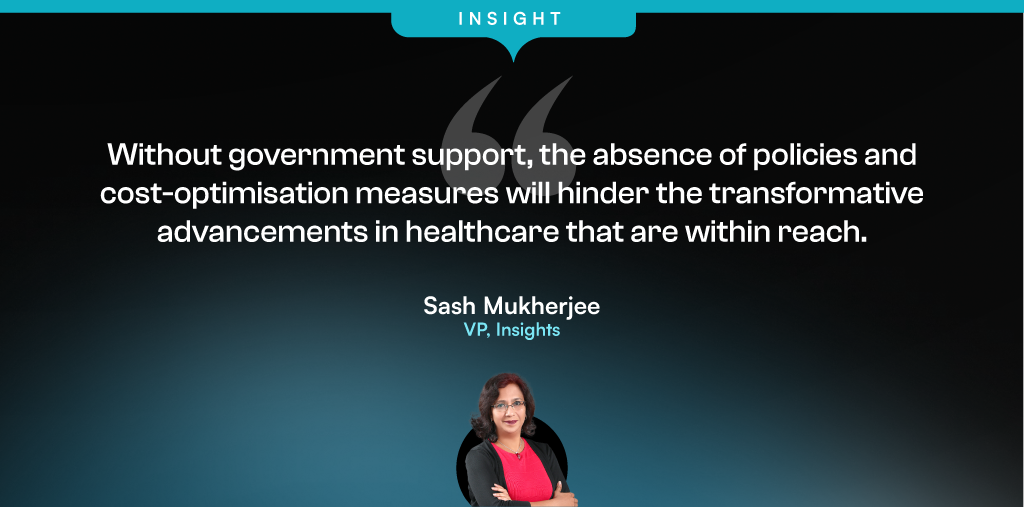
3. Agentic AI Will Move from Bank Credit Recommendation to Approval
Through 2024, we have seen a significant upturn in Agentic AI making credit approval recommendations, providing human credit managers with the ability to approve more loans more quickly. Yet, it was still the mantra that ‘AI recommends—humans approve.’ That will change in 2025.
AI will ‘approve’ much more and much larger credit requests.
The impact will be multi-faceted: banks will greatly enhance client access to credit, offering 24/7 availability and reducing the credit approval and origination cycle to mere seconds. This will drive increased consumer lending for high-value purchases, such as major appliances, electronics, and household goods.
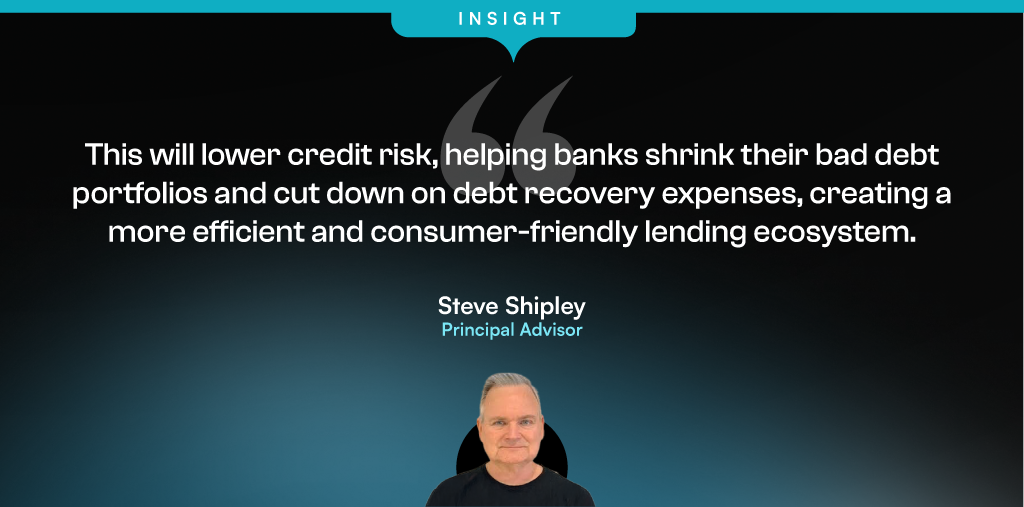
4. AI-Powered Demand Forecasting Will Transform Retail
There will be a significant shift away from math-based tools to predictive AI using an organisation’s own data. This technology will empower businesses to analyse massive datasets, including sales history, market trends, and social media, to generate highly accurate demand predictions. Adding external influencing factors such as weather and events will be simplified.
The forecasts will enable companies to optimise inventory levels, minimise stockouts and overstock situations, reduce waste, and increase profitability. Early adopters are already leveraging AI to anticipate fashion trends and adjust production accordingly.
No more worrying about capturing “Demand Influencing Factors” – it will all be derived from the organisation’s data.
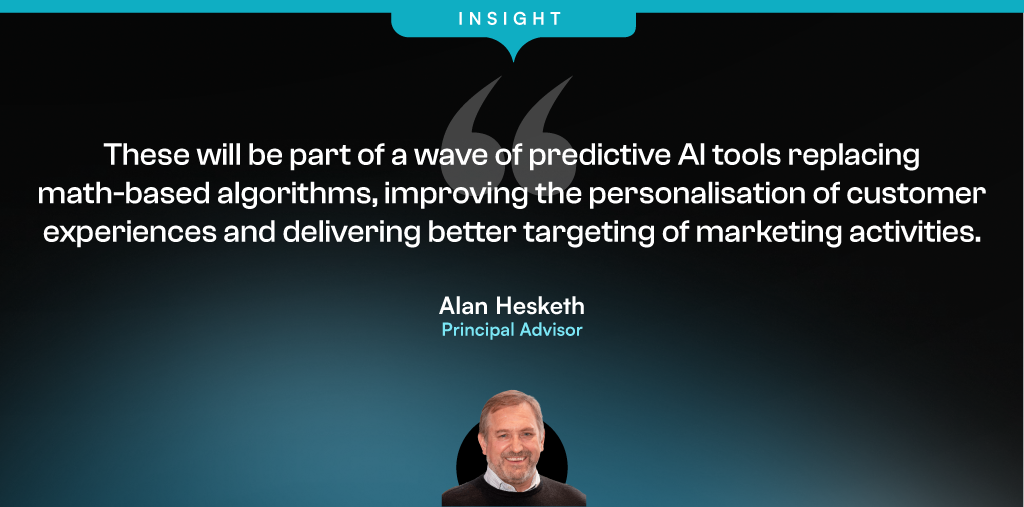
5. AI-Powered Custom-Tailored Insurance Will Be the New Norm
Insurers will harness real-time customer data, including behavioural patterns, lifestyle choices, and life stage indicators, to create dynamic policies that adapt to individual needs. Machine learning will process vast datasets to refine risk predictions and deliver highly personalised coverage. This will produce insurance products with unparalleled relevance and flexibility, closely aligning with each policyholder’s changing circumstances. Consumers will enjoy transparent pricing and tailored options that reflect their unique risk profiles, often resulting in cost savings. At the same time, insurers will benefit from enhanced risk assessment, reduced fraud, and increased customer satisfaction and loyalty.
This evolution will redefine the customer-insurer relationship, making insurance a more dynamic and responsive service that adjusts to life’s changes in real-time.
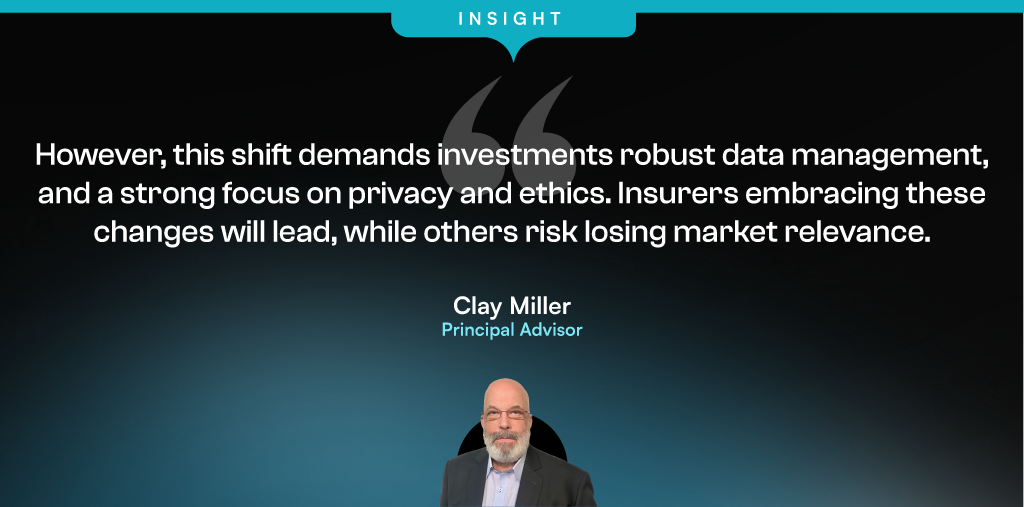
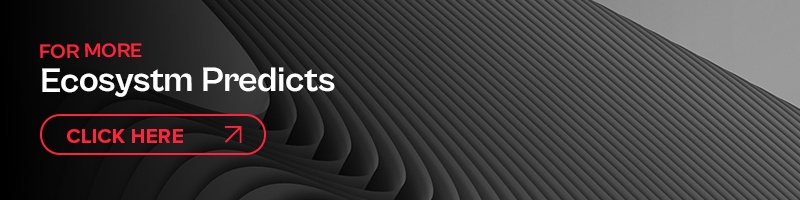
Exiting the North-South Highway 101 onto Mountain View, California, reveals how mundane innovation can appear in person. This Silicon Valley town, home to some of the most prominent tech giants, reveals little more than a few sprawling corporate campuses of glass and steel. As the industry evolves, its architecture naturally grows less inspiring. The most imposing structures, our modern-day coliseums, are massive energy-rich data centres, recursively training LLMs among other technologies. Yet, just as the unassuming exterior of the Googleplex conceals a maze of shiny new software, GenAI harbours immense untapped potential. And people are slowly realising that.
It has been over a year that GenAI burst onto the scene, hastening AI implementations and making AI benefits more identifiable. Today, we see successful use cases and collaborations all the time.
Finding Where Expectations Meet Reality
While the data centres of Mountain View thrum with the promise of a new era, it is crucial to have a quick reality check.
Just as the promise around dot-com startups reached a fever pitch before crashing, so too might the excitement surrounding AI be entering a period of adjustment. Every organisation appears to be looking to materialise the hype.
All eyes (including those of 15 million tourists) will be on Paris as they host the 2024 Olympics Games. The International Olympic Committee (IOC) recently introduced an AI-powered monitoring system to protect athletes from online abuse. This system demonstrates AI’s practical application, monitoring social media in real time, flagging abusive content, and ensuring athlete’s mental well-being. Online abuse is a critical issue in the 21st century. The IOC chose the right time, cause, and setting. All that is left is implementation. That’s where reality is met.
While the Googleplex doesn’t emanate the same futuristic aura as whatever is brewing within its walls, Google’s AI prowess is set to take centre stage as they partner with NBCUniversal as the official search AI partner of Team USA. By harnessing the power of their GenAI chatbot Gemini, NBCUniversal will create engaging and informative content that seamlessly integrates with their broadcasts. This will enhance viewership, making the Games more accessible and enjoyable for fans across various platforms and demographics. The move is part of NBCUniversal’s effort to modernise its coverage and attract a wider audience, including those who don’t watch live television and younger viewers who prefer online content.
From Silicon Valley to Main Street
While tech giants invest heavily in GenAI-driven product strategies, retailers and distributors must adapt to this new sales landscape.
Perhaps the promise of GenAI lies in the simple storefronts where it meets the everyday consumer. Just a short drive down the road from the Googleplex, one of many 37,000-square-foot Best Buys is preparing for a launch that could redefine how AI is sold.
In the most digitally vogue style possible, the chain retailer is rolling out Microsoft’s flagship AI-enabled PCs by training over 30,000 employees to sell and repair them and equipping over 1,000 store employees with AI skillsets. Best Buy are positioning themselves to revitalise sales, which have been declining for the past ten quarters. The company anticipates that the augmentation of AI skills across a workforce will drive future growth.
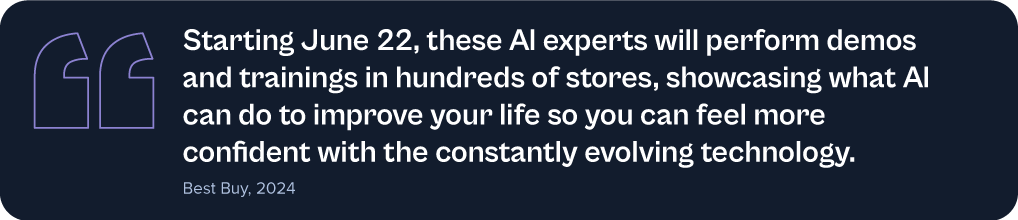
The Next Generation of User-Software Interaction
We are slowly evolving from seeking solutions to seamless integration, marking a new era of User-Centric AI.
The dynamic between humans and software has mostly been transactional: a question for an answer, or a command for execution. GenAI however, is poised to reshape this. Apple, renowned for their intuitive, user-centric ecosystem, is forging a deeper and more personalised relationship between humans and their digital tools.
Apple recently announced a collaboration with OpenAI at its WWDC, integrating ChatGPT into Siri (their digital assistant) in its new iOS 18 and macOS Sequoia rollout. According to Tim Cook, CEO, they aim to “combine generative AI with a user’s personal context to deliver truly helpful intelligence”.
Apple aims to prioritise user personalisation and control. Operating directly on the user’s device, it ensures their data remains secure while assimilating AI into their daily lives. For example, Siri now leverages “on-screen awareness” to understand both voice commands and the context of the user’s screen, enhancing its ability to assist with any task. This marks a new era of personalised GenAI, where technology understands and caters to individual needs.
We are beginning to embrace a future where LLMs assume customer-facing roles. The reality is, however, that we still live in a world where complex issues are escalated to humans.
The digital enterprise landscape is evolving. Examples such as the Salesforce Einstein Service Agent, its first fully autonomous AI agent, aim to revolutionise chatbot experiences. Built on the Einstein 1 Platform, it uses LLMs to understand context and generate conversational responses grounded in trusted business data. It offers 24/7 service, can be deployed quickly with pre-built templates, and handles simple tasks autonomously.
The technology does show promise, but it is important to acknowledge that GenAI is not yet fully equipped to handle the nuanced and complex scenarios that full customer-facing roles need. As technology progresses in the background, companies are beginning to adopt a hybrid approach, combining AI capabilities with human expertise.
AI for All: Democratising Innovation
The transformations happening inside the Googleplex, and its neighbouring giants, is undeniable. The collaborative efforts of Google, SAP, Microsoft, Apple, and Salesforce, amongst many other companies leverage GenAI in unique ways and paint a picture of a rapidly evolving tech ecosystem. It’s a landscape where AI is no longer confined to research labs or data centres, but is permeating our everyday lives, from Olympic broadcasts to customer service interactions, and even our personal devices.
The accessibility of AI is increasing, thanks to efforts like Best Buy’s employee training and Apple’s on-device AI models. Microsoft’s Copilot and Power Apps empower individuals without technical expertise to harness AI’s capabilities. Tools like Canva and Uizard empower anybody with UI/UX skills. Platforms like Coursera offer certifications in AI. It’s never been easier to self-teach and apply such important skills. While the technology continues to mature, it’s clear that the future of AI isn’t just about what the machines can do for us—it’s about what we can do with them. The on-ramp to technological discovery is no longer North-South Highway 101 or the Googleplex that lays within, but rather a network of tools and resources that’s rapidly expanding, inviting everyone to participate in the next wave of technological transformation.
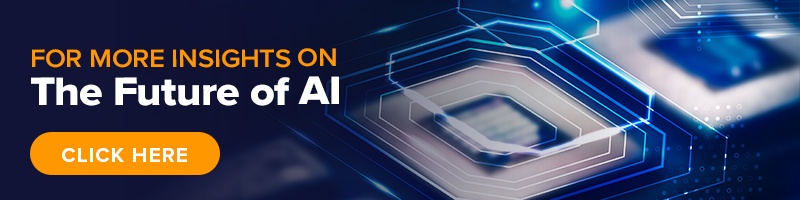
Southeast Asia’s massive workforce – 3rd largest globally – faces a critical upskilling gap, especially with the rise of AI. While AI adoption promises a USD 1 trillion GDP boost by 2030, unlocking this potential requires a future-proof workforce equipped with AI expertise.
Governments and technology providers are joining forces to build strong AI ecosystems, accelerating R&D and nurturing homegrown talent. It’s a tight race, but with focused investments, Southeast Asia can bridge the digital gap and turn its AI aspirations into reality.
Read on to find out how countries like Singapore, Thailand, Vietnam, and The Philippines are implementing comprehensive strategies to build AI literacy and expertise among their populations.
Download ‘Upskilling for the Future: Building AI Capabilities in Southeast Asia’ as a PDF
Big Tech Invests in AI Workforce
Southeast Asia’s tech scene heats up as Big Tech giants scramble for dominance in emerging tech adoption.
Microsoft is partnering with governments, nonprofits, and corporations across Indonesia, Malaysia, the Philippines, Thailand, and Vietnam to equip 2.5M people with AI skills by 2025. Additionally, the organisation will also train 100,000 Filipino women in AI and cybersecurity.
Singapore sets ambitious goal to triple its AI workforce by 2028. To achieve this, AWS will train 5,000 individuals annually in AI skills over the next three years.
NVIDIA has partnered with FPT Software to build an AI factory, while also championing AI education through Vietnamese schools and universities. In Malaysia, they have launched an AI sandbox to nurture 100 AI companies targeting USD 209M by 2030.
Singapore Aims to be a Global AI Hub
Singapore is doubling down on upskilling, global leadership, and building an AI-ready nation.
Singapore has launched its second National AI Strategy (NAIS 2.0) to solidify its global AI leadership. The aim is to triple the AI talent pool to 15,000, establish AI Centres of Excellence, and accelerate public sector AI adoption. The strategy focuses on developing AI “peaks of excellence” and empowering people and businesses to use AI confidently.
In keeping with this vision, the country’s 2024 budget is set to train workers who are over 40 on in-demand skills to prepare the workforce for AI. The country will also invest USD 27M to build AI expertise, by offering 100 AI scholarships for students and attracting experts from all over the globe to collaborate with the country.
Thailand Aims for AI Independence
Thailand’s ‘Ignite Thailand’ 2030 vision focuses on boosting innovation, R&D, and the tech workforce.
Thailand is launching the second phase of its National AI Strategy, with a USD 42M budget to develop an AI workforce and create a Thai Large Language Model (ThaiLLM). The plan aims to train 30,000 workers in sectors like tourism and finance, reducing reliance on foreign AI.
The Thai government is partnering with Microsoft to build a new data centre in Thailand, offering AI training for over 100,000 individuals and supporting the growing developer community.
Building a Digital Vietnam
Vietnam focuses on AI education, policy, and empowering women in tech.
Vietnam’s National Digital Transformation Programme aims to create a digital society by 2030, focusing on integrating AI into education and workforce training. It supports AI research through universities and looks to address challenges like addressing skill gaps, building digital infrastructure, and establishing comprehensive policies.
The Vietnamese government and UNDP launched Empower Her Tech, a digital skills initiative for female entrepreneurs, offering 10 online sessions on GenAI and no-code website creation tools.
The Philippines Gears Up for AI
The country focuses on investment, public-private partnerships, and building a tech-ready workforce.
With its strong STEM education and programming skills, the Philippines is well-positioned for an AI-driven market, allocating USD 30M for AI research and development.
The Philippine government is partnering with entities like IBPAP, Google, AWS, and Microsoft to train thousands in AI skills by 2025, offering both training and hands-on experience with cutting-edge technologies.
The strategy also funds AI research projects and partners with universities to expand AI education. Companies like KMC Teams will help establish and manage offshore AI teams, providing infrastructure and support.
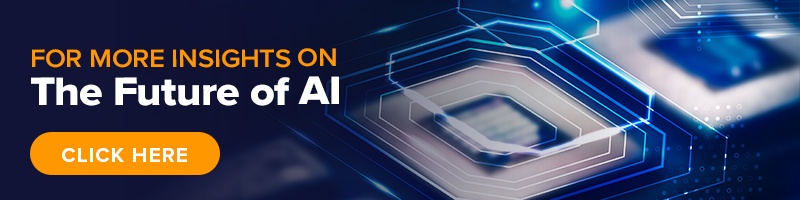
When OpenAI released ChatGPT, it became obvious – and very fast – that we were entering a new era of AI. Every tech company scrambled to release a comparable service or to infuse their products with some form of GenAI. Microsoft, piggybacking on its investment in OpenAI was the fastest to market with impressive text and image generation for the mainstream. Copilot is now embedded across its software, including Microsoft 365, Teams, GitHub, and Dynamics to supercharge the productivity of developers and knowledge workers. However, the race is on – AWS and Google are actively developing their own GenAI capabilities.
AWS Catches Up as Enterprise Gains Importance
Without a consumer-facing AI assistant, AWS was less visible during the early stages of the GenAI boom. They have since rectified this with a USD 4B investment into Anthropic, the makers of Claude. This partnership will benefit both Amazon and Anthropic, bringing the Claude 3 family of models to enterprise customers, hosted on AWS infrastructure.
As GenAI quickly emerges from shadow IT to an enterprise-grade tool, AWS is catching up by capitalising on their position as cloud leader. Many organisations view AWS as a strategic partner, already housing their data, powering critical applications, and providing an environment that developers are accustomed to. The ability to augment models with private data already residing in AWS data repositories will make it an attractive GenAI partner.
AWS has announced the general availability of Amazon Q, their suite of GenAI tools aimed at developers and businesses. Amazon Q Developer expands on what was launched as Code Whisperer last year. It helps developers accelerate the process of building, testing, and troubleshooting code, allowing them to focus on higher-value work. The tool, which can directly integrate with a developer’s chosen IDE, uses NLP to develop new functions, modernise legacy code, write security tests, and explain code.
Amazon Q Business is an AI assistant that can safely ingest an organisation’s internal data and connect with popular applications, such as Amazon S3, Salesforce, Microsoft Exchange, Slack, ServiceNow, and Jira. Access controls can be implemented to ensure data is only shared with authorised users. It leverages AWS’s visualisation tool, QuickSight, to summarise findings. It also integrates directly with applications like Slack, allowing users to query it directly.
Going a step further, Amazon Q Apps (in preview) allows employees to build their own lightweight GenAI apps using natural language. These employee-created apps can then be published to an enterprise’s app library for broader use. This no-code approach to development and deployment is part of a drive to use AI to increase productivity across lines of business.
AWS continues to expand on Bedrock, their managed service providing access to foundational models from companies like Mistral AI, Stability AI, Meta, and Anthropic. The service also allows customers to bring their own model in cases where they have already pre-trained their own LLM. Once a model is selected, organisations can extend its knowledge base using Retrieval-Augmented Generation (RAG) to privately access proprietary data. Models can also be refined over time to improve results and offer personalised experiences for users. Another feature, Agents for Amazon Bedrock, allows multi-step tasks to be performed by invoking APIs or searching knowledge bases.
To address AI safety concerns, Guardrails for Amazon Bedrock is now available to minimise harmful content generation and avoid negative outcomes for users and brands. Contentious topics can be filtered by varying thresholds, and Personally Identifiable Information (PII) can be masked. Enterprise-wide policies can be defined centrally and enforced across multiple Bedrock models.
Google Targeting Creators
Due to the potential impact on their core search business, Google took a measured approach to entering the GenAI field, compared to newer players like OpenAI and Perplexity. The useability of Google’s chatbot, Gemini, has improved significantly since its initial launch under the moniker Bard. Its image generator, however, was pulled earlier this year while it works out how to carefully tread the line between creativity and sensitivity. Based on recent demos though, it plans to target content creators with images (Imagen 3), video generation (Veo), and music (Lyria).
Like Microsoft, Google has seen that GenAI is a natural fit for collaboration and office productivity. Gemini can now assist the sidebar of Workspace apps, like Docs, Sheets, Slides, Drive, Gmail, and Meet. With Google Search already a critical productivity tool for most knowledge workers, it is determined to remain a leader in the GenAI era.
At their recent Cloud Next event, Google announced the Gemini Code Assist, a GenAI-powered development tool that is more robust than its previous offering. Using RAG, it can customise suggestions for developers by accessing an organisation’s private codebase. With a one-million-token large context window, it also has full codebase awareness making it possible to make extensive changes at once.
The Hardware Problem of AI
The demands that GenAI places on compute and memory have created a shortage of AI chips, causing the valuation of GPU giant, NVIDIA, to skyrocket into the trillions of dollars. Though the initial training is most hardware-intensive, its importance will only rise as organisations leverage proprietary data for custom model development. Inferencing is less compute-heavy for early use cases, such as text generation and coding, but will be dwarfed by the needs of image, video, and audio creation.
Realising compute and memory will be a bottleneck, the hyperscalers are looking to solve this constraint by innovating with new chip designs of their own. AWS has custom-built specialised chips – Trainium2 and Inferentia2 – to bring down costs compared to traditional compute instances. Similarly, Microsoft announced the Maia 100, which it developed in conjunction with OpenAI. Google also revealed its 6th-generation tensor processing unit (TPU), Trillium, with significant increase in power efficiency, high bandwidth memory capacity, and peak compute performance.
The Future of the GenAI Landscape
As enterprises gain experience with GenAI, they will look to partner with providers that they can trust. Challenges around data security, governance, lineage, model transparency, and hallucination management will all need to be resolved. Additionally, controlling compute costs will begin to matter as GenAI initiatives start to scale. Enterprises should explore a multi-provider approach and leverage specialised data management vendors to ensure a successful GenAI journey.
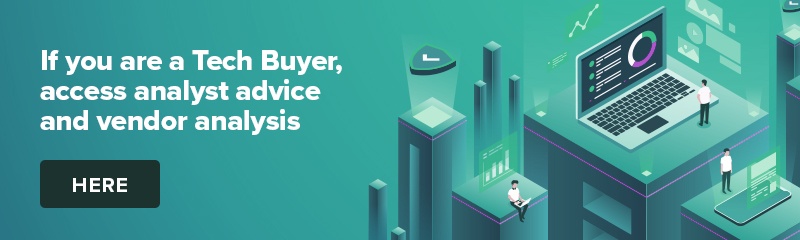
Historically, data scientists have been the linchpins in the world of AI and machine learning, responsible for everything from data collection and curation to model training and validation. However, as the field matures, we’re witnessing a significant shift towards specialisation, particularly in data engineering and the strategic role of Large Language Models (LLMs) in data curation and labelling. The integration of AI into applications is also reshaping the landscape of software development and application design.
The Growth of Embedded AI
AI is being embedded into applications to enhance user experience, optimise operations, and provide insights that were previously inaccessible. For example, natural language processing (NLP) models are being used to power conversational chatbots for customer service, while machine learning algorithms are analysing user behaviour to customise content feeds on social media platforms. These applications leverage AI to perform complex tasks, such as understanding user intent, predicting future actions, or automating decision-making processes, making AI integration a critical component of modern software development.
This shift towards AI-embedded applications is not only changing the nature of the products and services offered but is also transforming the roles of those who build them. Since the traditional developer may not possess extensive AI skills, the role of data scientists is evolving, moving away from data engineering tasks and increasingly towards direct involvement in development processes.
The Role of LLMs in Data Curation
The emergence of LLMs has introduced a novel approach to handling data curation and processing tasks traditionally performed by data scientists. LLMs, with their profound understanding of natural language and ability to generate human-like text, are increasingly being used to automate aspects of data labelling and curation. This not only speeds up the process but also allows data scientists to focus more on strategic tasks such as model architecture design and hyperparameter tuning.
The accuracy of AI models is directly tied to the quality of the data they’re trained on. Incorrectly labelled data or poorly curated datasets can lead to biased outcomes, mispredictions, and ultimately, the failure of AI projects. The role of data engineers and the use of advanced tools like LLMs in ensuring the integrity of data cannot be overstated.
The Impact on Traditional Developers
Traditional software developers have primarily focused on writing code, debugging, and software maintenance, with a clear emphasis on programming languages, algorithms, and software architecture. However, as applications become more AI-driven, there is a growing need for developers to understand and integrate AI models and algorithms into their applications. This requirement presents a challenge for developers who may not have specialised training in AI or data science. This is seeing an increasing demand for upskilling and cross-disciplinary collaboration to bridge the gap between traditional software development and AI integration.
Clear Role Differentiation: Data Engineering and Data Science
In response to this shift, the role of data scientists is expanding beyond the confines of traditional data engineering and data science, to include more direct involvement in the development of applications and the embedding of AI features and functions.
Data engineering has always been a foundational element of the data scientist’s role, and its importance has increased with the surge in data volume, variety, and velocity. Integrating LLMs into the data collection process represents a cutting-edge approach to automating the curation and labelling of data, streamlining the data management process, and significantly enhancing the efficiency of data utilisation for AI and ML projects.
Accurate data labelling and meticulous curation are paramount to developing models that are both reliable and unbiased. Errors in data labelling or poorly curated datasets can lead to models that make inaccurate predictions or, worse, perpetuate biases. The integration of LLMs into data engineering tasks is facilitating a transformation, freeing them from the burdens of manual data labelling and curation. This has led to a more specialised data scientist role that allocates more time and resources to areas that can create greater impact.
The Evolving Role of Data Scientists
Data scientists, with their deep understanding of AI models and algorithms, are increasingly working alongside developers to embed AI capabilities into applications. This collaboration is essential for ensuring that AI models are effectively integrated, optimised for performance, and aligned with the application’s objectives.
- Model Development and Innovation. With the groundwork of data preparation laid by LLMs, data scientists can focus on developing more sophisticated and accurate AI models, exploring new algorithms, and innovating in AI and ML technologies.
- Strategic Insights and Decision Making. Data scientists can spend more time analysing data and extracting valuable insights that can inform business strategies and decision-making processes.
- Cross-disciplinary Collaboration. This shift also enables data scientists to engage more deeply in interdisciplinary collaboration, working closely with other departments to ensure that AI and ML technologies are effectively integrated into broader business processes and objectives.
- AI Feature Design. Data scientists are playing a crucial role in designing AI-driven features of applications, ensuring that the use of AI adds tangible value to the user experience.
- Model Integration and Optimisation. Data scientists are also involved in integrating AI models into the application architecture, optimising them for efficiency and scalability, and ensuring that they perform effectively in production environments.
- Monitoring and Iteration. Once AI models are deployed, data scientists work on monitoring their performance, interpreting outcomes, and making necessary adjustments. This iterative process ensures that AI functionalities continue to meet user needs and adapt to changing data landscapes.
- Research and Continued Learning. Finally, the transformation allows data scientists to dedicate more time to research and continued learning, staying ahead of the rapidly evolving field of AI and ensuring that their skills and knowledge remain cutting-edge.
Conclusion
The integration of AI into applications is leading to a transformation in the roles within the software development ecosystem. As applications become increasingly AI-driven, the distinction between software development and AI model development is blurring. This convergence needs a more collaborative approach, where traditional developers gain AI literacy and data scientists take on more active roles in application development. The evolution of these roles highlights the interdisciplinary nature of building modern AI-embedded applications and underscores the importance of continuous learning and adaptation in the rapidly advancing field of AI.
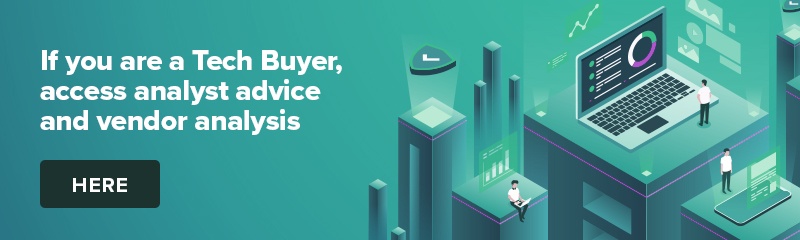
Customer teams in Singapore face a complex challenge. Organisations recognise the significance of a distinctive customer experience (CX) and adaptability to market shifts in a competitive landscape. They also prioritise enhancing employee experience (EX) and reducing costs. Balancing these priorities requires recalibrating across people, processes, and technologies.
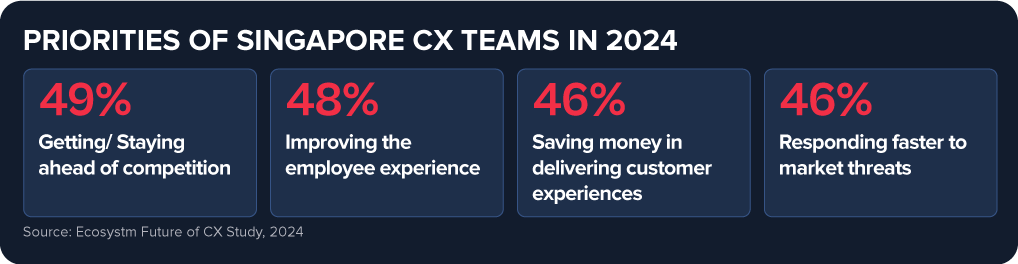
This underscores the pivotal role of data in CX transformation. When CX teams and contact centres prioritise data in all their initiatives, they gain deep insights into customer journeys, facilitating proactive service delivery, enhancing self-service mechanisms, and fostering genuine innovation in customer engagement.
Here are 5 ways organisations in Singapore can achieve these business objectives.
Download ‘5 Ways to Succeed in Singapore’s Competitive Battle to Win Customer Hearts’ as a PDF.
#1 Build a Strategy around Voice & Omnichannel Orchestration
Customers seek flexibility to choose channels that suit their preferences, often switching between them. When channels are well-coordinated, customers enjoy consistent experiences, and CX teams and contact centre agents gain real-time insights into interactions, regardless of the chosen channel. This boosts key metrics like First Call Resolution (FCR) and reduces Average Handle Time (AHT).
This doesn’t diminish the significance of voice. Voice remains crucial, especially for understanding complex inquiries and providing an alternative when customers face persistent challenges on other channels. Regardless of the channel chosen, prioritising omnichannel orchestration is essential.
Ensure seamless orchestration from voice to back and front offices, including social channels, as customers switch between channels.

#2 Unify Customer Data through an Intelligent Data Hub
Accessing real-time, accurate data is essential for effective customer and agent engagement. However, organisations often face challenges with data silos and lack of interconnected data, hindering omnichannel experiences.
A Customer Data Platform (CDP) can eliminate data silos and provide actionable insights.
- Identify behavioural trends by understanding patterns to personalise interactions.
- Spot real-time customer issues across channels.
- Uncover compliance gaps and missed sales opportunities from unstructured data.
- Look at customer journeys to proactively address their needs and exceed expectations.

#3 Transform CX & EX with AI
GenAI and Large Language Models (LLMs) is revolutionising how brands address customer and employee challenges, boosting efficiency, and enhancing service quality.
Despite 62% of Singapore organisations investing in virtual assistants/conversational AI, many have yet to integrate emerging technologies to elevate their CX & EX capabilities.
Agent Assist solutions provide real-time insights before customer interactions, optimising service delivery and saving time. With GenAI, they can automate mundane tasks like call summaries, freeing agents to focus on high-value tasks such as sales collaboration, proactive feedback management, personalised outbound calls, and upskilling.
Going beyond chatbots and Agent Assist solutions, predictive AI algorithms leverage customer data to forecast trends and optimise resource allocation. AI-driven identity validation swiftly confirms customer identities, mitigating fraud risks.

#4 Augment Existing Systems for Success
Despite the rise in digital interactions, many organisations struggle to fully modernise their legacy systems.
For those managing multiple disparate systems yet aiming to lead in CX transformation, a platform that integrates desired capabilities for holistic CX and EX experiences is vital.
A unified platform streamlines application management, ensuring cohesion, unified KPIs, enhanced security, simplified maintenance, and single sign-on for agents. This approach offers consistent experiences across channels and early issue detection, eliminating the need to navigate multiple applications or projects.
Capabilities that a platform should have:
- Programmable APIs to deliver messages across preferred social and messaging channels.
- Modernisation of outdated IVRs with self-service automation.
- Transformation of static mobile apps into engaging experience tools.
- Fraud prevention across channels through immediate phone number verification APIs.

#5 Focus on Proactive CX
In the new CX economy, organisations must meet customers on their terms, proactively engaging them before they initiate interactions. This will require organisations to re-evaluate all aspects of their CX delivery.
- Redefine the Contact Centre. Transform it into an “Intelligent” Data Hub providing unified and connected experiences. Leverage intelligent APIs to proactively manage customer interactions seamlessly across journeys.
- Reimagine the Agent’s Role. Empower agents to be AI-powered brand ambassadors, with access to prior and real-time interactions, instant decision-making abilities, and data-led knowledge bases.
- Redesign the Channel and Brand Experience. Ensure consistent omnichannel experiences through data unification and coherency. Use programmable APIs to personalise conversations and identify customer preferences for real-time or asynchronous messaging. Incorporate innovative technologies such as video to enhance the channel experience.
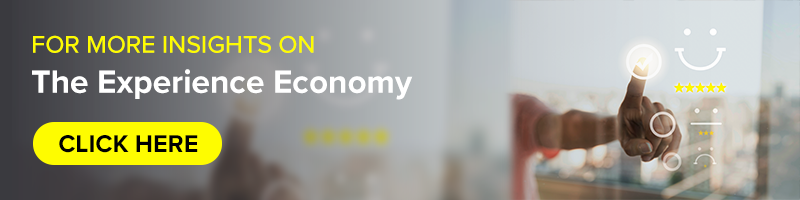
Customer feedback is at the heart of Customer Experience (CX). But it’s changing. What we consider customer feedback, how we collect and analyse it, and how we act on it is changing. Today, an estimated 80-90% of customer data is unstructured. Are you able and ready to leverage insights from that vast amount of customer feedback data?
Let’s begin with the basics: What is VoC and why is there so much buzz around it now?
Voice of the Customer (VoC) traditionally refers to customer feedback programs. In its most basic form that means organisations are sending surveys to customers to ask for feedback. And for a long time that really was the only way for organisations to understand what their customers thought about their brand, products, and services.
But that was way back then. Over the last few years, we’ve seen the market (organisations and vendors) dipping their toes into the world of unsolicited feedback.
What’s unsolicited feedback, you ask?
Unsolicited feedback simply means organisations didn’t actually ask for it and they’re often not in control over it, but the customer provides feedback in some way, shape, or form. That’s quite a change to the traditional survey approach, where they got answers to questions they specifically asked (solicited feedback).
Unsolicited feedback is important for many reasons:
- Organisations can tap into a much wider range of feedback sources, from surveys to contact centre phone calls, chats, emails, complaints, social media conversations, online reviews, CRM notes – the list is long.
- Surveys have many advantages, but also many disadvantages. From only hearing from a very specific customer type (those who respond and are typically at the extreme ends of the feedback sentiment), getting feedback on the questions they ask, and hearing from a very small portion of the customer base (think email open rates and survey fatigue).
- With unsolicited feedback organisations hear from 100% of the customers who interact with the brand. They hear what customers have to say, and not just how they answer predefined questions.
It is a huge step up, especially from the traditional post-call survey. Imagine a customer just spent 30 min on the line with an agent explaining their problem and frustration, just to receive a survey post call, to tell the organisation what they just told the agent, and how they felt about the experience. Organisations should already know that. In fact, they probably do – they just haven’t started tapping into that data yet. At least not for CX and customer insights purposes.
When does GenAI feature?
We can now tap into those raw feedback sources and analyse the unstructured data in a way never seen before. Long gone are the days of manual excel survey verbatim read-throughs or coding (although I’m well aware that that’s still happening!). Tech, in particular GenAI and Large Language Models (LLMs), are now assisting organisations in decluttering all the messy conversations and unstructured data. Not only is the quality of the analysis greatly enhanced, but the insights are also presented in user-friendly formats. Customer teams ask for the insights they need, and the tools spit it out in text form, graphs, tables, and so on.
The time from raw data to insights has reduced drastically, from hours and days down to seconds. Not only has the speed, quality, and ease of analysis improved, but many vendors are now integrating recommendations into their offerings. The tools can provide “basic” recommendations to help customer teams to act on the feedback, based on the insights uncovered.
Think of all the productivity gains and spare time organisations now have to act on the insights and drive positive CX improvements.
What does that mean for CX Teams and Organisations?
Including unsolicited feedback into the analysis to gain customer insights also changes how organisations set up and run CX and insights programs.
It’s important to understand that feedback doesn’t belong to a single person or team. CX is a team sport and particularly when it comes to acting on insights. It’s essential to share these insights with the right people, at the right time.
Some common misperceptions:
- Surveys have “owners” and only the owners can see that feedback.
- Feedback that comes through a specific channel, is specific to that channel or product.
- Contact centre feedback is only collected to coach staff.
If that’s how organisations have built their programs, they’ll have to rethink what they’re doing.
If organisations think about some of the more commonly used unstructured feedback, such as that from the contact centre or social media, it’s important to note that this feedback isn’t solely about the contact centre or social media teams. It’s about something else. In fact, it’s usually about something that created friction in the customer experience, that was generated by another team in the organisation. For example: An incorrect bill can lead to a grumpy social media post or a faulty product can lead to a disgruntled call to the contact centre. If the feedback is only shared with the social media or contact centre team, how will the underlying issues be resolved? The frontline teams service customers, but organisations also need to fix the underlying root causes that created the friction in the first place.
And that’s why organisations need to start consolidating the feedback data and democratise it.
It’s time to break down data and organisational silos and truly start thinking about the customer. No more silos. Instead, organisations must focus on a centralised customer data repository and data democratisation to share insights with the right people at the right time.
In my next Ecosystm Insights, I will discuss some of the tech options that CX teams have. Stay tuned!
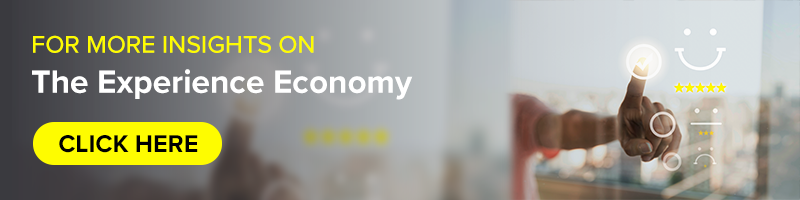