Advanced technology and the growing interconnectedness of devices are no longer futuristic concepts in health and life sciences – they’re driving a powerful transformation. Technology, combined with societal demands, is reshaping drug discovery, clinical trials, patient care, and even our understanding of the human body.
The potential to create more efficient, personalised, and effective healthcare solutions has never been greater.
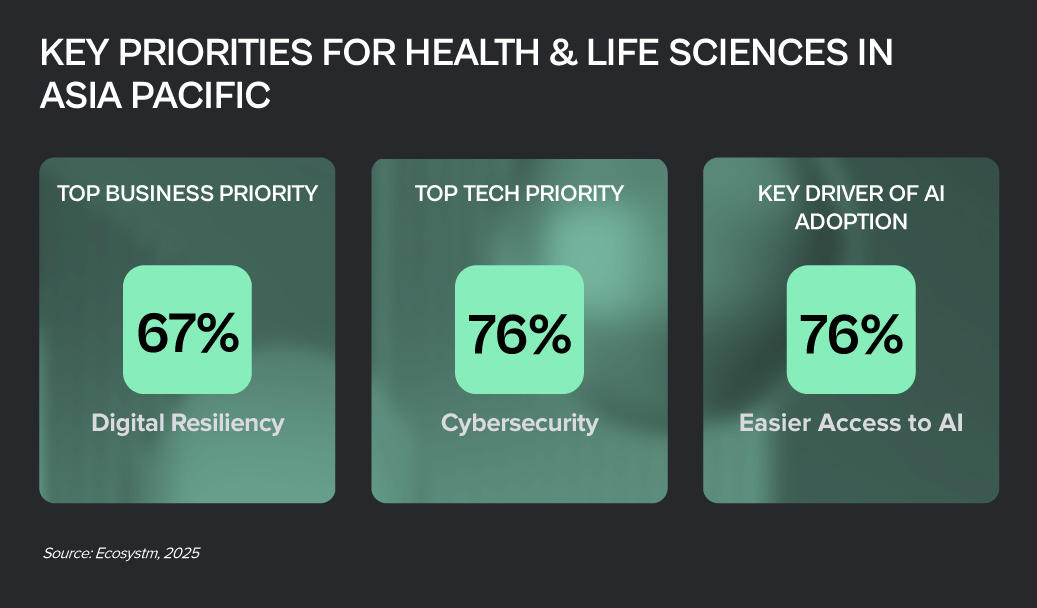
Click here to download “Future Forward: Reimagining Health & Life Sciences” as a PDF.
Modernising HR for Enhanced Efficiency & Employee Experience
The National Healthcare Group (NHG), a leading public healthcare provider in Singapore, recognised the need to modernise their HR system to better support 20,000+ healthcare professionals and improve patient services.
The iConnect@NHG initiative was launched to centralise HR functions, providing mobile access and self-service capabilities, and streamlining workflows across NHG’s integrated network of hospitals, polyclinics, and specialty centres.
The solution streamlined HR processes, giving employees easy access to essential data, career tools, and claims. The cloud-based platform improved data accuracy, reduced admin work, and integrated analytics for better decision-making and engagement. With 95% adoption, productivity and job satisfaction surged, enabling staff to focus on care delivery.
Automating Workflows for Better Patient Outcomes
Gold Coast Health handles a high volume of patient interactions across a wide range of medical services. The challenge was to streamline operations and reduce administrative burdens to improve patient care.
The solution involved automating the patient intake process by replacing paper forms with electronic versions, freeing up significant staff time.
A new clinical imaging solution also automates the uploading of wound images and descriptions into patient records, further saving time. Additionally, Gold Coast Health implemented a Discharge to Reassess system to automate follow-ups for long-term outpatient care. They are also exploring AI to simplify tasks and improve access to information, allowing clinical teams to focus more on patient care.
Streamlining Operations, Improving Care
IHH Healthcare, a global provider with over 80 hospitals across 10 countries, faced a fragmented IT landscape that hindered data management and patient care.
To resolve this, IHH migrated their core application workloads, including EMRs, to a next-gen cloud platform, unifying data across their network and enhancing analytics.
Additionally, they adopted an on-prem cloud solution to comply with local data residency requirements. This transformation reduced report generation time from days to hours, boosting operational efficiency and improving patient and clinician experiences. By leveraging advanced cloud technologies, IHH is strengthening their commitment to delivering world-class healthcare.
Creating Seamless & Compassionate Patient Journeys
The Narayana Health group in India is committed to providing accessible, high-quality care. However, they faced challenges with fragmented patient data, which hindered personalised care and efficient interactions.
To address this, Narayana Health centralised patient data, providing agents with a 360-degree view to offer more informed and compassionate service.
By automating tasks like call routing and form-filling, the organisation reduced average handling times and increased appointment conversions. Additionally, automated communication tools delivered timely, sensitive updates, strengthening patient relationships. The initiative has improved operational efficiency and deepened the organisation’s patient-centric focus.
Reimagining Location Services for Digital Healthcare
Halodoc, a leading digital health platform in Indonesia, connects millions of users with healthcare professionals and pharmacies.
To improve key services like home lab appointments and medicine delivery, Halodoc sought a more cost-effective and secure location service.
The transition resulted in an 88% reduction in costs for geocoding and places functionalities while enhancing data security. With better performance monitoring, Halodoc processed millions of geocoding and place requests with no major issues. This migration not only optimised costs but also resolved long-standing technical challenges, positioning Halodoc for future innovation, including machine learning and AI. The move strengthened their data security and provided a solid foundation for continued growth and high-quality healthcare delivery across Indonesia.
Driving Efficiency & Accessibility through Integrated Systems
Lupin, a global pharma leader, aimed to boost patient care, streamline operations, and enhance accessibility. By integrating systems and centralising data, Lupin wanted seamless interactions between patients, doctors, and the salesforce.
The company implemented a scalable infrastructure optimised for critical business applications, backed by high-performance server and storage technologies.
This integration improved data-driven decision-making, leading to optimised operations, reduced costs, and improved medicine quality and affordability. The robust infrastructure also ensured near-zero downtime, enhancing reliability and efficiency. Through this transformation, Lupin reinforced its commitment to providing patient-centred, affordable healthcare with faster, more efficient outcomes.
Leveraging AI for Cloud Security
Mitsubishi Tanabe Pharma’s “VISION 30” seeks to deliver personalised healthcare by 2030, focusing on precision medicine and digital solutions. The company is investing in advanced digital technologies and secure data infrastructure to achieve these goals.
To secure their expanding cloud platform, the company adopted a zero-trust model and enhanced identity management.
A security assessment identified gaps in cloud configuration, prompting tailored security improvements. GenAI was introduced to translate and summarise security alerts, reducing processing time from 10 minutes to just one minute, improving efficiency and security awareness across the team. The company is actively exploring further AI-driven solutions to strengthen security and drive their digital transformation, advancing the vision for personalised healthcare.
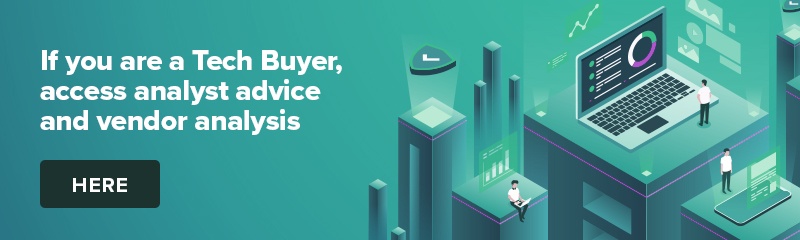
The Healthcare industry has achieved much in the last two years, despite all the struggles and pivots. However, the impact of COVID-19 on the industry is far from over.
2020 was focused on finding a vaccine against the virus, setting treatment protocols, and workforce management to handle the emergency. 2021 was focused on vaccine distribution and administration. 2022 will be the year when we start seeing the second-order impacts of the pandemic – and see healthcare providers address these impacts.
In 2022 the key drivers of the ongoing transformation in Healthcare will be:
- Patients. Improved self-knowledge and ownership of personal health outcomes and data
- Technology. Widespread availability and adoption of digital and cognitive technologies
- Employees. The ongoing challenges of clinical and administrative staff
- The Life Sciences Industry. The recent investments in, and success of the sector
- Policy Makers. The sharp increase in focus on population health
- Continued Uncertainty. Around challenges such as new virus strains, anti-vaccine protests, supply chain disruptions etc.
Read on to find out what Ecosystm Analysts Amit Rana, Krish Krishnan, and Sash Mukherjee think are the key achievements of the industry and the future trends in 2022 and beyond.
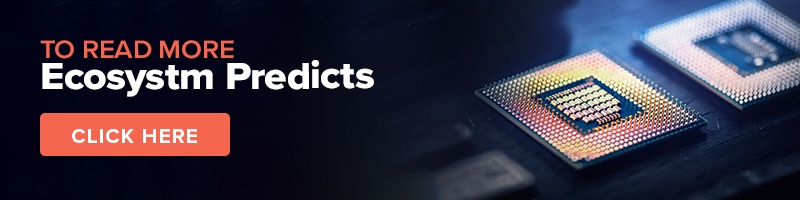
Last week I wrote about the need to remove hype from reality when it comes to AI. But what will ensure that your AI projects succeed?
It is quite obvious that success is determined by human aspects rather than technological factors. We have identified four key organisational actions that enable successful AI implementation at scale (Figure 1).
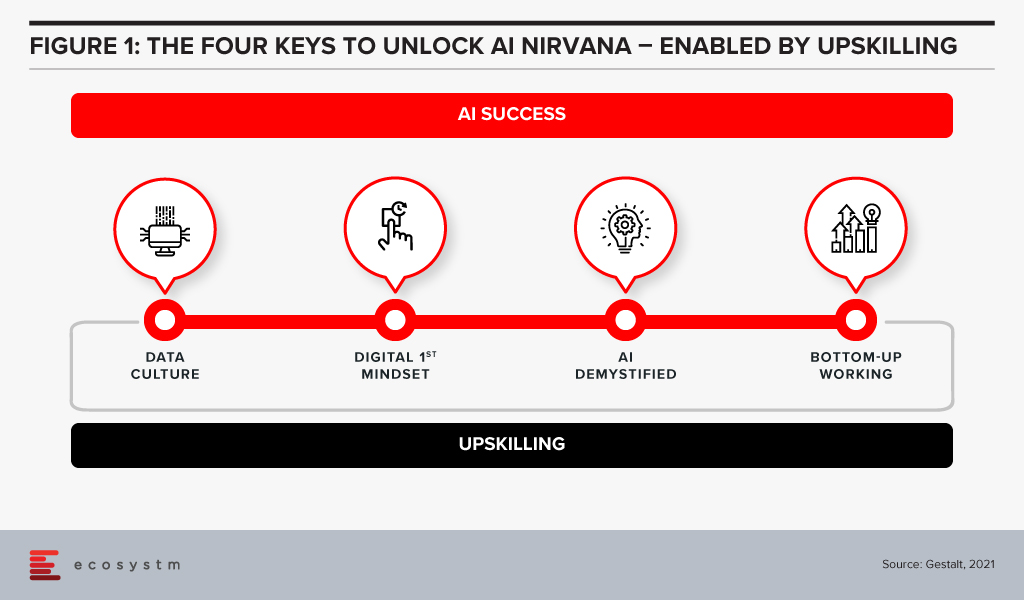
#1 Establish a Data Culture
The traditional focus for companies has been on ensuring access to good, clean data sets and the proper use of that data. Ecosystm research shows that only 28% of organisations focused on customer service, also focus on creating a data-driven organisational culture. But our experience has shown that culture is more critical than having the data. Does the organisation have a culture of using data to drive decisions? Does every level of the organisation understand and use data insights to do their day-to-day jobs? Is decision-making data-driven and decentralised, needing to be escalated only when there is ambiguity or need for strategic clarity? Do business teams push for new data sources when they are not able to get the insights they need?
Without this kind of culture, it may be possible to implement individual pieces of automation in a specific area or process, applying brute force to see it through. In order to transform the business and truly extract the power of AI, we advise organisations to build a culture of data-driven decision-making first. That organisational mindset, will make you capable implementing AI at scale. Focusing on changing the organisational culture will deliver greater returns than trying to implement piecemeal AI projects – even in the short to mid-term.
#2 Ingrain a Digital-First Mindset
Assuming a firm has passed the data culture hurdle, it needs to consider whether it has adopted a digital-first mindset. AI is one of many technologies that impact businesses, along with AR/VR, IoT, 5G, cloud and Blockchain to name a few. Today’s environment requires firms to be capable of utilising a variety of these technologies – often together – and possessing a workforce capable of using these digital tools.
A workforce with the digital-first mindset looks for a digital solution to problems wherever appropriate. They have a good understanding of digital technologies relevant to their space and understand key digital methodologies – such as Customer 360 to deliver a truly superior customer experience or Agile methodologies to successfully manage AI at scale.
AI needs business managers at the operational levels to work with IT or AI tech teams to pinpoint processes that are right for AI. They need to make an estimation based on historical data of what specific problems require an AI solution. This is enabled by the digital-first mindset.
#3 Demystify AI
The next step is to get business leaders, functional leaders, and business operational teams – not just those who work with AI – to acquire a basic understanding of AI.
They do not need to learn the intricacies of programming or how to create neural networks or anything nearly as technical in nature. However, all levels from the leadership down should have a solid understanding of what AI can do, the basics of how it works, how the process of training data results in improved outcomes and so on. They need to understand the continuous learning nature of AI solutions, getting better over time. While AI tools may recommend an answer, human insight is often needed to make a correct decision off this recommendation.

#4 Drive Implementation Bottom-Up
AI projects need alignment, objectives, strategy – and leadership and executive buy-in. But a very important aspect of an AI-driven organisation that is able to build scalable AI, is letting projects run bottom up.
As an example, a reputed Life Sciences company embarked on a multi-year AI project to improve productivity. They wanted to use NLP, Discovery, Cognitive Assist and ML to augment clinical proficiency of doctors and expected significant benefits in drug discovery and clinical trials by leveraging the immense dataset that was built over the last 20 years.
The company ran this like any other transformation project, with a central program management team taking the lead with the help of an AI Centre of Competency. These two teams developed a compelling business case, and identified initial pilots aligned with the long-term objectives of the program. However, after 18 months, they had very few tangible outcomes. Everyone including doctors, research scientists, technicians, and administrators, who participated in the program had their own interpretation of what AI was not able to do.
Discussion revealed that the doctors and researchers felt that they were training AI to replace themselves. Seeing a tool trying to mimic the same access and understanding of numerous documents baffled them at best. They were not ready to work with AI programs step-by-step to help AI tools learn and discover new insights.
At this point, we suggested approaching the project bottom-up – wherein the participating teams would decide specific projects to take up. This developed a culture where teams collaborated as well as competed with each other, to find new ways to use AI. Employees were shown a roadmap of how their jobs would be enhanced by offloading routine decisions to AI. They were shown that AI tools augment the employees’ cognitive capabilities and made them more effective.
The team working on critical trials found these tools extremely useful and were able to collaborate with other organisations specialising in similar trials. They created the metadata and used ML algorithms to discover new insights. Working bottom-up led to a very successful AI deployment.
We have seen time and again that while leadership may set the strategy and objectives, it is best to let the teams work bottom-up to come up with the projects to implement.
#5 Invest in Upskilling
The four “keys” are important to build an AI-powered, future-proof enterprise. They are all human related – and when they come together to work as a winning formula is when organisations invest in upskilling. Upskilling is the common glue and each factor requires specific kinds of upskilling (Figure 2).
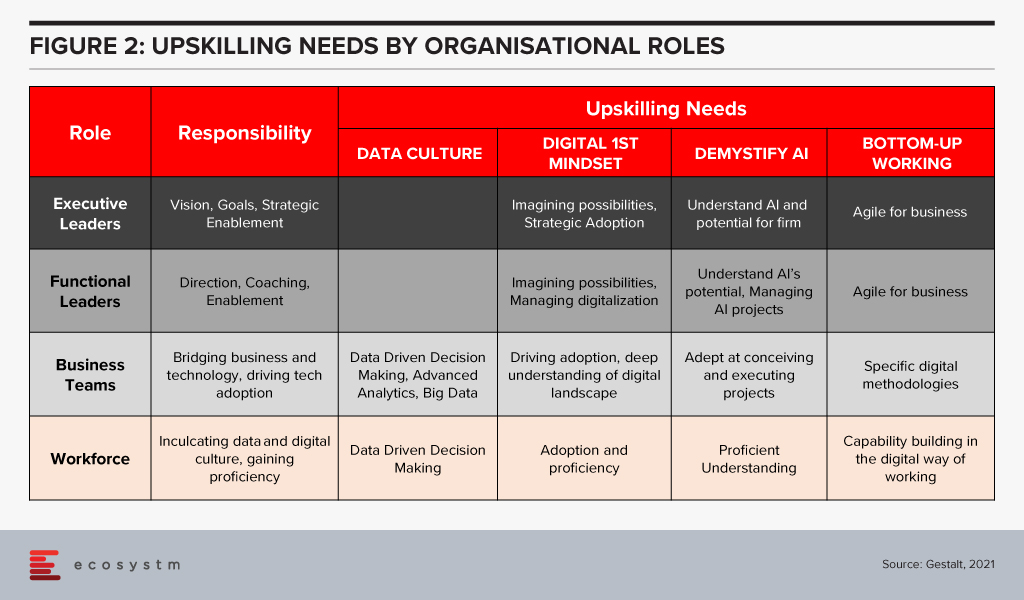
Upskilling needs vary by organisational level and the key being addressed. The bottom line is that upskilling is a universal requirement for driving AI at scale, successfully. And many organisations are realising it fast – Bosch and DBS Bank are some of the notable examples.
How much is your organisation invested in upskilling for AI implementation at scale? Share your stories in the comment box below.
Written with contributions from Ravi Pattamatta and Ratnesh Prasad
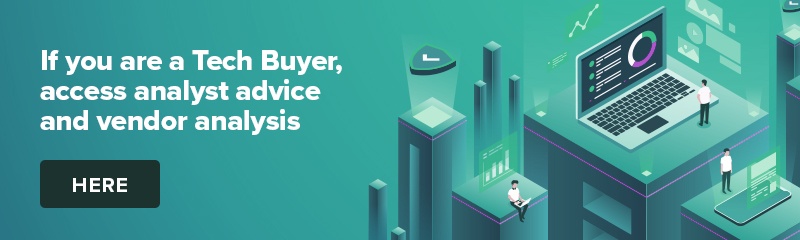
As the search for a COVID-19 vaccine intensifies, there is a global focus on the Life Sciences industry. The industry has been hit hard this year – having to deliver overtime through a disrupted supply chain, unexpected demand spikes, and reduction of revenues from their regular streams. Life sciences organisations are already challenged by the breadth of their focus – across R&D and clinical discovery; Manufacturing & Distribution; and Sales & Marketing. Increasingly, many pharmaceutical and medtech organisations choose to outsource some of these functions, which brings to fore the need for a robust compliance framework. In the Ecosystm Digital Priorities in the New Normal Study, two-thirds of life sciences organisations mention that they have either been forced to start, accelerate or refocus their Digital Transformation initiatives – the remaining one-third have put their Digital Transformation on hold. The industry is clearly at an inflection point.
Challenges of the Life Sciences Industry
Continued Focus on R&D. Life sciences companies operate in an extremely competitive global market where they have to work on new products against a backdrop of competition from generics and a global concern over rising healthcare expenditure. Apart from regulatory challenges, they also face immense competition from local manufacturers as they enter each new market.
Re-thinking their Distribution Strategy. Sales and distribution for many pharma and medtech organisations have been traditional – using agents, distributors, clinicians, and healthcare providers. But now they need to change their go-to-market strategies, target patients and consumers directly and package their product offerings into value-added services. This will require them to incorporate customer experience enhancers in their R&D, going beyond drug discovery and product innovation.
Tracking Global Regulations. Governments across the world are trying to manage their healthcare budgets. They are also more focused on chronic disease management. The focus has shifted to value-based medicine in general, but pharma and medtech products are being increasingly held accountable by health outcomes. Governments are increasingly implementing drug reforms around what clinicians can prescribe. Global Life Sciences organisations have to constantly monitor the regulations in the multiple countries where they operate and sell. They are also accountable for their entire supply chain, especially ensuring a high product quality and fraud prevention.
The global Ecosystm AI study reveals the top priorities for Life Sciences organisations, focused on adopting emerging technologies (Figure 1). They appear to be investing in emerging technology especially in their R&D and clinical discovery and Manufacturing functions.
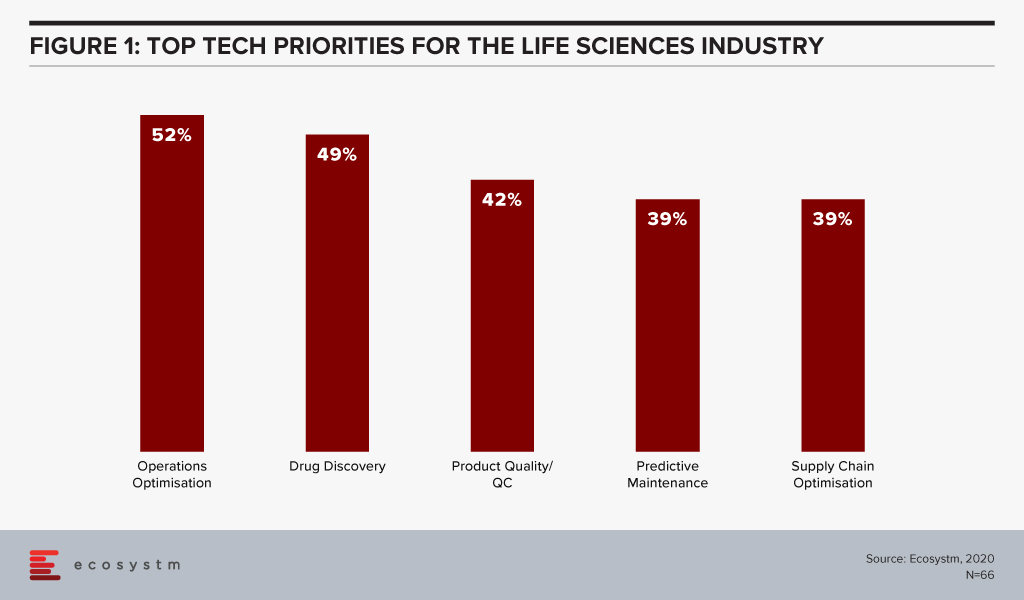
Technology as an Enabler of Life Sciences Transformation
Discovery and Development
With the evolution of technology, Life Sciences organisations are able to automate much of the mundane tasks around drug discovery and apply AI and machine learning to transform their drug discovery and development process. They are increasingly leveraging their ecosystem of smaller pharma and medtech companies, research laboratories, academic institutions, and technology providers to make the process more time and cost efficient.
Using an AI algorithm, the researchers at the Massachusetts Institute of Technology have discovered an antibiotic compound that can kill many species of antibiotic-resistant bacteria. MIT’s algorithm screens millions of chemical compounds and chooses the antibiotics which have the potential to eliminate bacteria resistant to existing drugs. Harvard’s Wyss Institute for Biologically Inspired Engineering is manufacturing 3D printed organ-on-a-chip to give insights on cell, tissue, and organ biology to help the pharma sector with drug development, disease modelling and finally in the development of personalised medicine.
Life Sciences are also engaging more with technology partners – whether emerging start-ups or established players. Pfizer and Saama are working together on AI clinical data mining. The companies are developing and deploying an AI-based analytical tool where Pfizer provides clinical data and domain knowledge to train models on the Saama Life Science Analytics Cloud (LSAC). Saama was identified as a partner at a hackathon. Sanofi and Google have established a new virtual Innovation Lab to develop scientific and commercial solutions, using multiple Google capabilities from cloud computing to AI.
Tech providers also keep evolving their capabilities in the Life Sciences industry for more efficient drug discovery and better treatment protocols. Microsoft’s Project Hanover uses machine learning to develop a personalised drug protocol to manage acute myeloid leukaemia. Similarly, Apple’s ResearchKit – an open-source framework is meant to help researchers and developers create iOS-based applications in the field of medical research.
Manufacturing and Logistics
The industry also faces the challenges faced by any Manufacturing organisation and has the need to deploy manufacturing analytics, and advanced supply chain technology for better process and optimisation and agility. There is also the need for complete visibility over their supply chain and inventory for traceability, safety, and fraud prevention. Emerging technologies such as Blockchain will become increasingly relevant for real-time track and trace capability.
The MediLedger Network was established as an open network to the entire pharma supply chain. The project brings a consortium of some of the world’s largest pharmaceutical companies, and logistics providers to improve drug supply chain management.
Since the data on the distributed ledger is encrypted, it creates a secure system without any vulnerabilities. This eliminates counterfeit products and ultimately ensures the quality of the pharma products and promotes increased patient safety. To foster security and improve the supply chain, the United States Food and Drug Administration (USFDA) successfully completed a pilot with a group including IBM, KPMG, Merck and Walmart to support U.S. Drug Supply Chain Security Act (DSCSA) to trace vaccines and prescription medicines throughout the country.
Diagnostics and Personalised Healthcare
As more devices (consumer and enterprise) and applications enter the market, people will take ownership and interest in their own health outcomes. This is seeing a continued growth in online communities and comparison sites (on physicians, hospitals, and pharmaceutical products). Increasingly, insurance providers will use data from wearable devices for a more personalised approach; promoting and rewarding good health practices.
Beyond the use of wearables and health and wellness apps, we will also see an exponential increase of home-based healthcare products and services – whether for primary care and chronic disease management, or long-term and palliative care. As patients become more engaged with their care, the life sciences industry is beginning to serve them through personalised approach, medicines, right diagnosis and through advanced medical devices and products.
An online tool developed by the University of Virginia Health Systems helps identify patients that have a high risk of getting a stroke and helps them reduce that risk. This tool calculates the patient’s probability of suffering a stroke by measuring the severity of their metabolic syndrome – taking into account a number of conditions that include high blood pressure, abnormal cholesterol levels and excess body fat. Life Sciences organisations are increasingly having to invest in customer-focused solutions such as these.
Wearables with special smart software to monitor health parameters, gauge drug compatibility and monitor complications are being implemented by Life Sciences organisations. The US FDA approved a pill called Abilify MyCite fitted with a tiny ingestible sensor that communicates with a patch worn by the patient to transmit data on a smartphone. Medtech companies continue to develop FDA approved health devices that can monitor chronic conditions. Smart continuous glucose monitoring (CGM) and insulin pens send blood glucose level data to smartphone applications allowing the wearer to easily check their information and detect trends.
Technologies such as AR/VR are also enabling Life Sciences companies with their diagnostics. Regeneron Pharmaceuticals has created an AR/VR app called “In My Eyes” to better diagnose vision impairment in patients.
What is interesting about these personalised products is that not only do they improve clinical outcomes, they also give Life Sciences companies access to rich data that can be used for further product development and improvement.
The Life Sciences industry will continue to operate in an unpredictable and competitive market. This is evident by the several mergers and acquisitions that we witness in the industry. As they continue to use cutting-edge technology for their R&D practices, they will leverage technology to transform other functions as well.
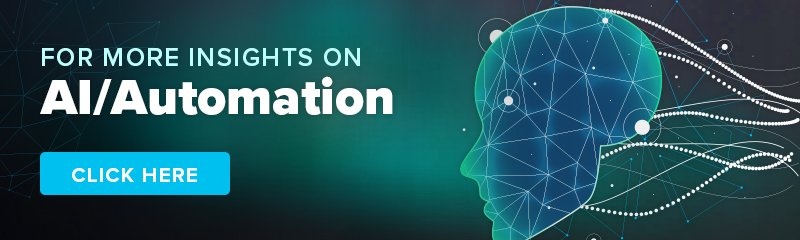