AI has become a business necessity today, catalysing innovation, efficiency, and growth by transforming extensive data into actionable insights, automating tasks, improving decision-making, boosting productivity, and enabling the creation of new products and services.
Generative AI stole the limelight in 2023 given its remarkable advancements and potential to automate various cognitive processes. However, now the real opportunity lies in leveraging this increased focus and attention to shine the AI lens on all business processes and capabilities. As organisations grasp the potential for productivity enhancements, accelerated operations, improved customer outcomes, and enhanced business performance, investment in AI capabilities is expected to surge.
In this eBook, Ecosystm VP Research Tim Sheedy and Vinod Bijlani and Aman Deep from HPE APAC share their insights on why it is crucial to establish tailored AI capabilities within the organisation.
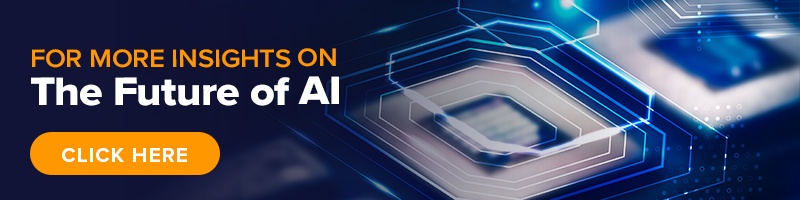
Over the past year, many organisations have explored Generative AI and LLMs, with some successfully identifying, piloting, and integrating suitable use cases. As business leaders push tech teams to implement additional use cases, the repercussions on their roles will become more pronounced. Embracing GenAI will require a mindset reorientation, and tech leaders will see substantial impact across various ‘traditional’ domains.
AIOps and GenAI Synergy: Shaping the Future of IT Operations
When discussing AIOps adoption, there are commonly two responses: “Show me what you’ve got” or “We already have a team of Data Scientists building models”. The former usually demonstrates executive sponsorship without a specific business case, resulting in a lukewarm response to many pre-built AIOps solutions due to their lack of a defined business problem. On the other hand, organisations with dedicated Data Scientist teams face a different challenge. While these teams can create impressive models, they often face pushback from the business as the solutions may not often address operational or business needs. The challenge arises from Data Scientists’ limited understanding of the data, hindering the development of use cases that effectively align with business needs.
The most effective approach lies in adopting an AIOps Framework. Incorporating GenAI into AIOps frameworks can enhance their effectiveness, enabling improved automation, intelligent decision-making, and streamlined operational processes within IT operations.
This allows active business involvement in defining and validating use-cases, while enabling Data Scientists to focus on model building. It bridges the gap between technical expertise and business requirements, ensuring AIOps initiatives are influenced by the capabilities of GenAI, address specific operational challenges and resonate with the organisation’s goals.
The Next Frontier of IT Infrastructure
Many companies adopting GenAI are openly evaluating public cloud-based solutions like ChatGPT or Microsoft Copilot against on-premises alternatives, grappling with the trade-offs between scalability and convenience versus control and data security.
Cloud-based GenAI offers easy access to computing resources without substantial upfront investments. However, companies face challenges in relinquishing control over training data, potentially leading to inaccurate results or “AI hallucinations,” and concerns about exposing confidential data. On-premises GenAI solutions provide greater control, customisation, and enhanced data security, ensuring data privacy, but require significant hardware investments due to unexpectedly high GPU demands during both the training and inferencing stages of AI models.
Hardware companies are focusing on innovating and enhancing their offerings to meet the increasing demands of GenAI. The evolution and availability of powerful and scalable GPU-centric hardware solutions are essential for organisations to effectively adopt on-premises deployments, enabling them to access the necessary computational resources to fully unleash the potential of GenAI. Collaboration between hardware development and AI innovation is crucial for maximising the benefits of GenAI and ensuring that the hardware infrastructure can adequately support the computational demands required for widespread adoption across diverse industries. Innovations in hardware architecture, such as neuromorphic computing and quantum computing, hold promise in addressing the complex computing requirements of advanced AI models.
The synchronisation between hardware innovation and GenAI demands will require technology leaders to re-skill themselves on what they have done for years – infrastructure management.
The Rise of Event-Driven Designs in IT Architecture
IT leaders traditionally relied on three-tier architectures – presentation for user interface, application for logic and processing, and data for storage. Despite their structured approach, these architectures often lacked scalability and real-time responsiveness. The advent of microservices, containerisation, and serverless computing facilitated event-driven designs, enabling dynamic responses to real-time events, and enhancing agility and scalability. Event-driven designs, are a paradigm shift away from traditional approaches, decoupling components and using events as a central communication mechanism. User actions, system notifications, or data updates trigger actions across distributed services, adding flexibility to the system.
However, adopting event-driven designs presents challenges, particularly in higher transaction-driven workloads where the speed of serverless function calls can significantly impact architectural design. While serverless computing offers scalability and flexibility, the latency introduced by initiating and executing serverless functions may pose challenges for systems that demand rapid, real-time responses. Increasing reliance on event-driven architectures underscores the need for advancements in hardware and compute power. Transitioning from legacy architectures can also be complex and may require a phased approach, with cultural shifts demanding adjustments and comprehensive training initiatives.
The shift to event-driven designs challenges IT Architects, whose traditional roles involved designing, planning, and overseeing complex systems. With Gen AI and automation enhancing design tasks, Architects will need to transition to more strategic and visionary roles. Gen AI showcases capabilities in pattern recognition, predictive analytics, and automated decision-making, promoting a symbiotic relationship with human expertise. This evolution doesn’t replace Architects but signifies a shift toward collaboration with AI-driven insights.
IT Architects need to evolve their skill set, blending technical expertise with strategic thinking and collaboration. This changing role will drive innovation, creating resilient, scalable, and responsive systems to meet the dynamic demands of the digital age.
Whether your organisation is evaluating or implementing GenAI, the need to upskill your tech team remains imperative. The evolution of AI technologies has disrupted the tech industry, impacting people in tech. Now is the opportune moment to acquire new skills and adapt tech roles to leverage the potential of GenAI rather than being disrupted by it.
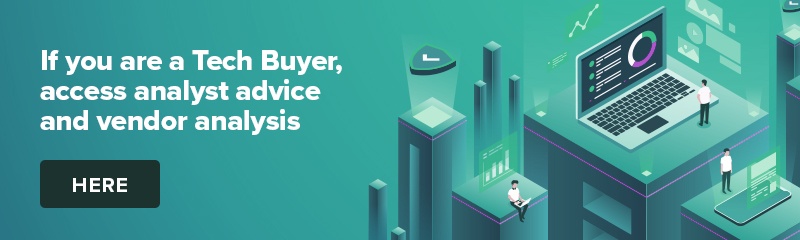
In the Ecosystm Predicts: Building an Agile & Resilient Organisation: Top 5 Trends in 2024, Principal Advisor Darian Bird said, “The emergence of Generative AI combined with the maturing of deepfake technology will make it possible for malicious agents to create personalised voice and video attacks.” Darian highlighted that this democratisation of phishing, facilitated by professional-sounding prose in various languages and tones, poses a significant threat to potential victims who rely on misspellings or oddly worded appeals to detect fraud. As we see more of these attacks and social engineering attempts, it is important to improve defence mechanisms and increase awareness.
Understanding Deepfake Technology
The term Deepfake is a combination of the words ‘deep learning’ and ‘fake’. Deepfakes are AI-generated media, typically in the form of images, videos, or audio recordings. These synthetic content pieces are designed to appear genuine, often leading to the manipulation of faces and voices in a highly realistic manner. Deepfake technology has gained spotlight due to its potential for creating convincing yet fraudulent content that blurs the line of reality.
Deepfake algorithms are powered by Generative Adversarial Networks (GANs) and continuously enhance synthetic content to closely resemble real data. Through iterative training on extensive datasets, these algorithms refine features such as facial expressions and voice inflections, ensuring a seamless emulation of authentic characteristics.
Deepfakes Becoming Increasingly Convincing
Hyper-realistic deepfakes, undetectable to the human eye and ear, have become a huge threat to the financial and technology sectors. Deepfake technology has become highly convincing, blurring the line between real and fake content. One of the early examples of a successful deepfake fraud was when a UK-based energy company lost USD 243k through a deepfake audio scam in 2019, where scammers mimicked the voice of their CEO to authorise an illegal fund transfer.
Deepfakes have evolved from audio simulations to highly convincing video manipulations where faces and expressions are altered in real-time, making it hard to distinguish between real and fake content. In 2022, for instance, a deepfake video of Elon Musk was used in a crypto scam that resulted in a loss of about USD 2 million for US consumers. This year, a multinational company in Hong Kong lost over USD 25 million when an employee was tricked into sending money to fraudulent accounts after a deepfake video call by what appeared to be his colleagues.
Regulatory Responses to Deepfakes
Countries worldwide are responding to the challenges posed by deepfake technology through regulations and awareness campaigns.
- Singapore’s Online Criminal Harms Act, that will come into effect in 2024, will empower authorities to order individuals and Internet service providers to remove or block criminal content, including deepfakes used for malicious purposes.
- The UAE National Programme for Artificial Intelligence released a deepfake guide to educate the public about both harmful and beneficial applications of this technology. The guide categorises fake content into shallow and deep fakes, providing methods to detect deepfakes using AI-based tools, with a focus on promoting positive uses of advanced technologies.
- The proposed EU AI Act aims to regulate them by imposing transparency requirements on creators, mandating them to disclose when content has been artificially generated or manipulated.
- South Korea passed a law in 2020 banning the distribution of harmful deepfakes. Offenders could be sentenced to up to five years in prison or fined up to USD 43k.
- In the US, states like California and Virginia have passed laws against deepfake pornography, while federal bills like the DEEP FAKES Accountability Act aim to mandate disclosure and counter malicious use, highlighting the diverse global efforts to address the multifaceted challenges of deepfake regulation.
Detecting and Protecting Against Deepfakes
Detecting deepfake becomes increasingly challenging as technology advances. Several methods are needed – sometimes in conjunction – to be able to detect a convincing deepfake. These include visual inspection that focuses on anomalies, metadata analysis to examine clues about authenticity, forensic analysis for pattern and audio examination, and machine learning that uses algorithms trained on real and fake video datasets to classify new videos.
However, identifying deepfakes requires sophisticated technology that many organisations may not have access to. This heightens the need for robust cybersecurity measures. Deepfakes have seen an increase in convincing and successful phishing – and spear phishing – attacks and cyber leaders need to double down on cyber practices.
Defences can no longer depend on spotting these attacks. It requires a multi-pronged approach which combines cyber technologies, incidence response, and user education.
Preventing access to users. By employing anti-spoofing measures organisations can safeguard their email addresses from exploitation by fraudulent actors. Simultaneously, minimising access to readily available information, particularly on websites and social media, reduces the chance of spear-phishing attempts. This includes educating employees about the implications of sharing personal information and clear digital footprint policies. Implementing email filtering mechanisms, whether at the server or device level, helps intercept suspicious emails; and the filtering rules need to be constantly evaluated using techniques such as IP filtering and attachment analysis.
Employee awareness and reporting. There are many ways that organisations can increase awareness in employees starting from regular training sessions to attack simulations. The usefulness of these sessions is often questioned as sometimes they are merely aimed at ticking off a compliance box. Security leaders should aim to make it easier for employees to recognise these attacks by familiarising them with standard processes and implementing verification measures for important email requests. This should be strengthened by a culture of reporting without any individual blame.
Securing against malware. Malware is often distributed through these attacks, making it crucial to ensure devices are well-configured and equipped with effective endpoint defences to prevent malware installation, even if users inadvertently click on suspicious links. Specific defences may include disabling macros and limiting administrator privileges to prevent accidental malware installation. Strengthening authentication and authorisation processes is also important, with measures such as multi-factor authentication, password managers, and alternative authentication methods like biometrics or smart cards. Zero trust and least privilege policies help protect organisation data and assets.
Detection and Response. A robust security logging system is crucial, either through off-the shelf monitoring tools, managed services, or dedicated teams for monitoring. What is more important is that the monitoring capabilities are regularly updated. Additionally, having a well-defined incident response can swiftly mitigate post-incident harm post-incident. This requires clear procedures for various incident types and designated personnel for executing them, such as initiating password resets or removing malware. Organisations should ensure that users are informed about reporting procedures, considering potential communication challenges in the event of device compromise.
Conclusion
The rise of deepfakes has brought forward the need for a collaborative approach. Policymakers, technology companies, and the public must work together to address the challenges posed by deepfakes. This collaboration is crucial for making better detection technologies, establishing stronger laws, and raising awareness on media literacy.
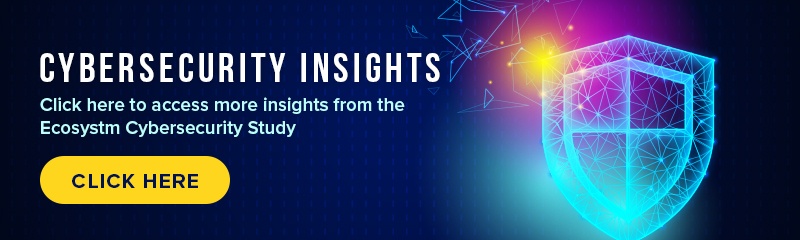
In my last Ecosystm Insights, I spoke about the implications of the shift from Predictive AI to Generative AI on ROI considerations of AI deployments. However, from my discussions with colleagues and technology leaders it became clear that there is a need to define and distinguish between Predictive AI and Generative AI better.
Predictive AI analyses historical data to predict future outcomes, crucial for informed decision-making and strategic planning. Generative AI unlocks new avenues for innovation by creating novel data and content. Organisations need both – Predictive AI for enhancing operational efficiencies and forecasting capabilities and Generative AI to drive innovation; create new products, services, and experiences; and solve complex problems in unprecedented ways.
This guide aims to demystify these categories, providing clarity on their differences, applications, and examples of the algorithms they use.
Predictive AI: Forecasting the Future
Predictive AI is extensively used in fields such as finance, marketing, healthcare and more. The core idea is to identify patterns or trends in data that can inform future decisions. Predictive AI relies on statistical, machine learning, and deep learning models to forecast outcomes.
Key Algorithms in Predictive AI
- Regression Analysis. Linear and logistic regression are foundational tools for predicting a continuous or categorical outcome based on one or more predictor variables.
- Decision Trees. These models use a tree-like graph of decisions and their possible consequences, including chance event outcomes, resource costs and utility.
- Random Forest (RF). An ensemble learning method that operates by constructing a multitude of decision trees at training time to improve predictive accuracy and control over-fitting.
- Gradient Boosting Machines (GBM). Another ensemble technique that builds models sequentially, each new model correcting errors made by the previous ones, used for both regression and classification tasks.
- Support Vector Machines (SVM). A supervised machine learning model that uses classification algorithms for two-group classification problems.
Generative AI: Creating New Data
Generative AI, on the other hand, focuses on generating new data that is similar but not identical to the data it has been trained on. This can include anything from images, text, and videos to synthetic data for training other AI models. GenAI is particularly known for its ability to innovate, create, and simulate in ways that predictive AI cannot.
Key Algorithms in Generative AI
- Generative Adversarial Networks (GANs). Comprising two networks – a generator and a discriminator – GANs are trained to generate new data with the same statistics as the training set.
- Variational Autoencoders (VAEs). These are generative algorithms that use neural networks for encoding inputs into a latent space representation, then reconstructing the input data based on this representation.
- Transformer Models. Originally designed for natural language processing (NLP) tasks, transformers can be adapted for generative purposes, as seen in models like GPT (Generative Pre-trained Transformer), which can generate coherent and contextually relevant text based on a given prompt.
Comparing Predictive and Generative AI
The fundamental difference between the two lies in their primary objectives: Predictive AI aims to forecast future outcomes based on past data, while Generative AI aims to create new, original data that mimics the input data in some form.
The differences become clearer when we look at these examples.
Predictive AI Examples
- Supply Chain Management. Analyses historical supply chain data to forecast demand, manage inventory levels, reduces costs and improve delivery times.
- Healthcare. Analysing patient records to predict disease outbreaks or the likelihood of a disease in individual patients.
- Predictive Maintenance. Analyse historical and real-time data and preemptively identifies system failures or network issues, enhancing infrastructure reliability and operational efficiency.
- Finance. Using historical stock prices and indicators to predict future market trends.
Generative AI Examples
- Content Creation. Generating realistic images or art from textual descriptions using GANs.
- Text Generation. Creating coherent and contextually relevant articles, stories, or conversational responses using transformer models like GPT-3.
- Chatbots and Virtual Assistants. Advanced GenAI models are enhancing chatbots and virtual assistants, making them more realistic.
- Automated Code Generation. By the use of natural language descriptions to generate programming code and scripts, to significantly speed up software development processes.
Conclusion
Organisations that exclusively focus on Generative AI may find themselves at the forefront of innovation, by leveraging its ability to create new content, simulate scenarios, and generate original data. However, solely relying on Generative AI without integrating Predictive AI’s capabilities may limit an organisation’s ability to make data-driven decisions and forecasts based on historical data. This could lead to missed opportunities to optimise operations, mitigate risks, and accurately plan for future trends and demands. Predictive AI’s strength lies in analysing past and present data to inform strategic decision-making, crucial for long-term sustainability and operational efficiency.
It is essential for companies to adopt a dual-strategy approach in their AI efforts. Together, these AI paradigms can significantly amplify an organisation’s ability to adapt, innovate, and compete in rapidly changing markets.
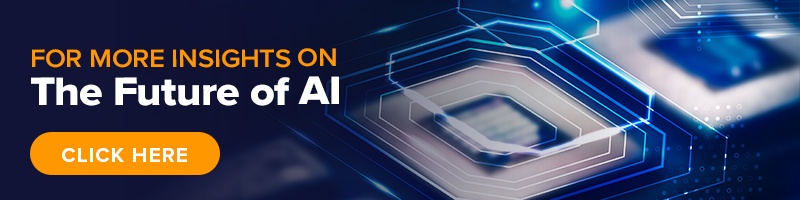
2024 and 2025 are looking good for IT services providers – particularly in Asia Pacific. All types of providers – from IT consultants to managed services VARs and systems integrators – will benefit from a few converging events.
However, amidst increasing demand, service providers are also challenged with cost control measures imposed in organisations – and this is heightened by the challenge of finding and retaining their best people as competition for skills intensifies. Providers that service mid-market clients might find it hard to compete and grow without significant process automation to compensate for the higher employee costs.
Why Organisations are Opting for IT Service
- Organisations are seeking further cost reductions. Managed services providers will see more opportunities to take cost and complexity out of organisation’s IT functions. The focus in 2024 will be less on “managing” services and more on “transforming” them using ML, AI, and automation to reduce cost and improve value.
- Big app upgrades are back on the agenda. SAP is going above and beyond to incentivise their customers and partners to migrate their on-premises and hyperscale hosted instances to true cloud ERP. Initiatives such as Rise with SAP have been further expanded and improved to accelerate the transition. Salesforce customers are also looking to streamline their deployments while also taking advantage of the new AI and data capabilities. But many of these projects will still be complex and time-consuming.
- Cloud deployments are getting more complex. For many organisations, the simple cloud migrations are done. This is the stage of replatforming, retiring, and refactoring applications to take advantage of public and hybrid cloud capabilities. These are not simple lift and shift – or switch to SaaS – engagements.
- AI will drive a greater need for process improvement and transformation. This will happen along with associated change management and training programs. While it is still early days for GenAI, before the end of 2024, many organisations will move beyond experimentation to department or enterprise wide GenAI initiatives.
- Increasing cybersecurity and data governance demands will prolong the security skill shortage. More organisations will turn to managed security services providers and cybersecurity consultants to help them develop their strategy and response to the rising threat levels.
Choosing the Right Cost Model for IT Services
Buyers of IT services must implement strict cost-control measures and consider various approaches to align costs with business and customer outcomes, including different cost models:
Fixed-Price Contracts. These contracts set a firm price for the entire project or specific deliverables. Ideal when project scope is clear, they offer budget certainty upfront but demand detailed specifications, potentially leading to higher initial quotes due to the provider assuming more risk.
Time and Materials (T&M) Contracts with Caps. Payment is based on actual time and materials used, with negotiated caps to prevent budget overruns. Combining flexibility with cost predictability, this model offers some control over total expenses.
Performance-Based Pricing. Fees are tied to service provider performance, incentivising achievement of specific KPIs or milestones. This aligns provider interests with client goals, potentially resulting in cost savings and improved service quality.
Retainer Agreements with Scope Limits. Recurring fees are paid for ongoing services, with defined limits on work scope or hours within a given period. This arrangement ensures resource availability while containing expenses, particularly suitable for ongoing support services.
Other Strategies for Cost Efficiency and Effective Management
Technology leaders should also consider implementing some of the following strategies:
Phased Payments. Structuring payments in phases, tied to the completion of project milestones, helps manage cash flow and provides a financial incentive for the service provider to meet deadlines and deliverables. It also allows for regular financial reviews and adjustments if the project scope changes.
Cost Transparency and Itemisation. Detailed billing that itemises the costs of labour, materials, and other expenses provides transparency to verify charges, track spending against the budget, and identify areas for potential savings.
Volume Discounts and Negotiated Rates. Negotiating volume discounts or preferential rates for long-term or large-scale engagements, makes providers to offer reduced rates for a commitment to a certain volume of work or an extended contract duration.
Utilisation of Shared Services or Cloud Solutions. Opting for shared or cloud-based solutions where feasible, offers economies of scale and reduces the need for expensive, dedicated infrastructure and resources.
Regular Review and Adjustment. Conducting regular reviews of the services and expenses with the provider to ensure alignment with the budget and objectives, prepares organisations to adjust the scope, renegotiate terms, or implement cost-saving measures as needed.
Exit Strategy. Planning an exit strategy that include provisions for contract termination, transition services, protects an organisation in case the partnership needs to be dissolved.
Conclusion
Many businesses swing between insourcing and outsourcing technology capabilities – with the recent trend moving towards insourcing development and outsourcing infrastructure to the public cloud. But 2024 will see demand for all types of IT services across nearly every geography and industry. Tech services providers can bring significant value to your business – but improved management, monitoring, and governance will ensure that this value is delivered at a fair cost.
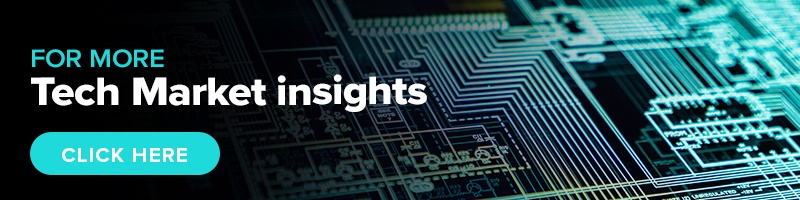
As tech providers such as Microsoft enhance their capabilities and products, they will impact business processes and technology skills, and influence other tech providers to reshape their product and service offerings. Microsoft recently organised briefing sessions in Sydney and Singapore, to present their future roadmap, with a focus on their AI capabilities.
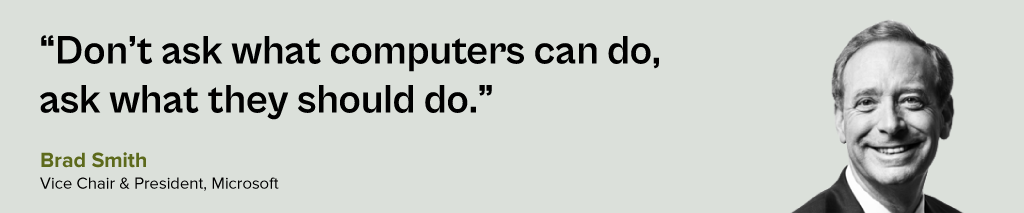
Ecosystm Advisors Achim Granzen, Peter Carr, and Tim Sheedy provide insights on Microsoft’s recent announcements and messaging.

Click here to download Ecosystm VendorSphere: Microsoft’s AI Vision – Initiatives & Impact
Ecosystm Question: What are your thoughts on Microsoft Copilot?
Tim Sheedy. The future of GenAI will not be about single LLMs getting bigger and better – it will be about the use of multiple large and small language models working together to solve specific challenges. It is wasteful to use a large and complex LLM to solve a problem that is simpler. Getting these models to work together will be key to solving industry and use case specific business and customer challenges in the future. Microsoft is already doing this with Microsoft 365 Copilot.
Achim Granzen. Microsoft’s Copilot – a shrink-wrapped GenAI tool based on OpenAI – has become a mainstream product. Microsoft has made it available to their enterprise clients in multiple ways: for personal productivity in Microsoft 365, for enterprise applications in Dynamics 365, for developers in Github and Copilot Studio, and to partners to integrate Copilot into their applications suites (E.g. Amdocs’ Customer Engagement Platform).
Ecosystm Question: How, in your opinion, is the Microsoft Copilot a game changer?
Microsoft’s Customer Copyright Commitment, initially launched as Copilot Copyright Commitment, is the true game changer.
Achim Granzen. It safeguards Copilot users from potential copyright infringement lawsuits related to data used for algorithm training or output results. In November 2023, Microsoft expanded its scope to cover commercial usage of their OpenAI interface as well.
This move not only protects commercial clients using Microsoft’s GenAI products but also extends to any GenAI solutions built by their clients. This initiative significantly reduces a key risk associated with GenAI adoption, outlined in the product terms and conditions.
However, compliance with a set of Required Mitigations and Codes of Conduct is necessary for clients to benefit from this commitment, aligning with responsible AI guidelines and best practices.
Ecosystm Question: Where will organisations need most help on their AI journeys?
Peter Carr. Unfortunately, there is no playbook for AI.
- The path to integrating AI into business strategies and operations lacks a one-size-fits-all guide. Organisations will have to navigate uncharted territories for the time being. This means experimenting with AI applications and learning from successes and failures. This exploratory approach is crucial for leveraging AI’s potential while adapting to unique organisational challenges and opportunities. So, companies that are better at agile innovation will do better in the short term.
- The effectiveness of AI is deeply tied to the availability and quality of connected data. AI systems require extensive datasets to learn and make informed decisions. Ensuring data is accessible, clean, and integrated is fundamental for AI to accurately analyse trends, predict outcomes, and drive intelligent automation across various applications.
Ecosystm Question: What advice would you give organisations adopting AI?
Tim Sheedy. It is all about opportunities and responsibility.
- There is a strong need for responsible AI – at a global level, at a country level, at an industry level and at an organisational level. Microsoft (and other AI leaders) are helping to create responsible AI systems that are fair, reliable, safe, private, secure, and inclusive. There is still a long way to go, but these capabilities do not completely indemnify users of AI. They still have a responsibility to set guardrails in their own businesses about the use and opportunities for AI.
- AI and hybrid work are often discussed as different trends in the market, with different solution sets. But in reality, they are deeply linked. AI can help enhance and improve hybrid work in businesses – and is a great opportunity to demonstrate the value of AI and tools such as Copilot.
Ecosystm Question: What should Microsoft focus on?
Tim Sheedy. Microsoft faces a challenge in educating the market about adopting AI, especially Copilot. They need to educate business, IT, and AI users on embracing AI effectively. Additionally, they must educate existing partners and find new AI partners to drive change in their client base. Success in the race for knowledge workers requires not only being first but also helping users maximise solutions. Customers have limited visibility of Copilot’s capabilities, today. Improving customer upskilling and enhancing tools to prompt users to leverage capabilities will contribute to Microsoft’s (or their competitors’) success in dominating the AI tool market.
Peter Carr. Grassroots businesses form the economic foundation of the Asia Pacific economies. Typically, these businesses do not engage with global SIs (GSIs), which drive Microsoft’s new service offerings. This leads to an adoption gap in the sector that could benefit most from operational efficiencies. To bridge this gap, Microsoft must empower non-GSI partners and managed service providers (MSPs) at the local and regional levels. They won’t achieve their goal of democratising AI, unless they do. Microsoft has the potential to advance AI technology while ensuring fair and widespread adoption.
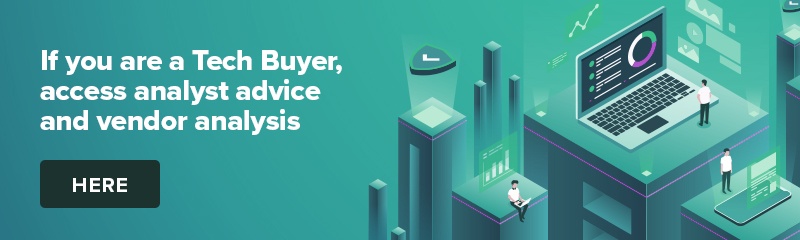
The AI landscape is undergoing a significant transformation, moving from traditional predictive AI use cases towards Generative AI (GenAI). Currently, most GenAI use cases promise an improvement in employee productivity, without focusing on how to leverage this into new or additional revenue generating streams. This raises concerns about the long-term return on investment (ROI) if this is not adequately addressed.
The Rise of Generative AI Over Predictive AI
Traditionally, predictive AI has been integral to business strategies, leveraging data to forecast future outcomes with remarkable accuracy. Industries across the board have used predictive models for a range of applications, from demand forecasting in retail to fraud detection in finance. However, the tide is changing with the emergence of GenAI technologies. GenAI, capable of creating content, designing products, and even coding, holds the promise to revolutionise how businesses operate, innovate, and compete.
The appeal of GenAI lies in its versatility and creativity, offering solutions that go beyond the capabilities of predictive models. For example, in the area of content creation, GenAI can produce written content, images, and videos at scale, potentially transforming marketing, entertainment, and education sectors. However, the current enthusiasm for GenAI’s productivity enhancements overshadows a critical aspect of technology adoption: monetisation.
The Productivity Paradox
While the emphasis on productivity improvements through GenAI applications is undoubtedly beneficial, there is a notable gap in exploring use cases that directly contribute to creating new revenue streams. This productivity paradox – prioritising operational efficiency and cost reduction – may not guarantee the sustained growth and ROI necessary from AI investments.
True innovation in AI should not only aim at making existing processes more efficient but also at uncovering opportunities for monetisation. This involves leveraging GenAI to develop new products, services, or business models to access untapped markets or enhance customer value in ways that directly impact the bottom line.
The Imperative for Strategic Reorientation
Ignoring the monetisation aspect of GenAI applications poses a significant risk to the anticipated ROI from AI investments. As businesses allocate resources to AI adoption and integration, it’s also important to consider how these technologies can generate revenue, not just save costs. Without a clear path to monetisation, the investments in AI, particularly in the cutting-edge domain of GenAI, may not prove viable in the next financial year and beyond.
To mitigate this risk, companies need to adopt a dual approach. First, they must continue to explore and exploit the productivity gains offered by GenAI, which are crucial for maintaining a competitive edge and achieving operational excellence. At the same time, businesses must strategically explore and invest in GenAI-driven opportunities for monetisation. This could mean innovating in product design, personalised customer experiences, or entirely new business models that were previously unfeasible.
Conclusion
The excitement around GenAI’s potential to transform industries is well-founded, but it must be tempered with strategic planning to ensure long-term viability and ROI. Businesses that recognise and act on the opportunity to not only improve productivity but also to monetise GenAI innovations will lead the next wave of growth in their respective sectors. The challenge lies in balancing the drive for efficiency with the pursuit of new revenue streams, ensuring that investments in AI deliver sustainable returns. As the AI landscape evolves, the ability to innovate in monetisation as much as in technology will distinguish the leaders from the followers.
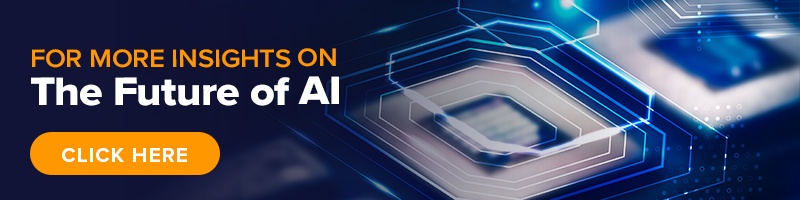
2024 has started cautiously for organisations, with many choosing to continue with tech projects that have already initiated, while waiting for clearer market conditions before starting newer transformation projects. This means that tech providers must continue to refine their market messaging and enhance their service/product offerings to strengthen their market presence in the latter part of the year. Ecosystm analysts present five key considerations for tech providers as they navigate evolving market and customer trends, this year.
Navigating Market Dynamics

Continuing Economic Uncertainties. Organisations will focus on ongoing projects and consider expanding initiatives in the latter part of the year. This means that tech providers should maintain visibility and trust with existing clients. They also need to help their customers meet multiple KPIs.
Popularity of Generative AI. For organisations, this will be the time to go beyond the novelty factor and assess practical business outcomes, allied costs, and change management. Tech providers need to include ROI discussions for short-term and mid-term perspectives as organisations move beyond pilots.
Infrastructure Market Disruption. Tech leaders will keep an eye out for advancements and disruptions in the market (likely to originate from the semiconductor sector). The disruptions might require tech vendors to re-assess the infrastructure partner ecosystem.
Need for New Tech Skills. Tech leaders will evaluate Generative AI’s impact on AIOps and IT Architecture; invest in upskilling for talent retention. Tech providers must prioritise creating user-friendly experiences to make technology accessible to business users. Training and partner enablement will also need a higher focus.
Increased Focus on Governance. Tech leaders will consult tech vendors on how to implement safeguards for data usage, sharing, and cybersecurity. This opens up opportunities in offering governance-related services.
5 Key Considerations for Tech Vendors
#1 Get Ready for the Year of the AI Startup

While many AI companies have been around for years, this will be the year that many of them make a significant play into enterprises in Asia Pacific. This comes at a time when many organisations are attempting to reduce tech debt and simplify their tech architecture.
For these AI startups to succeed, they will need to create watertight business cases, and do a lot of the hard work in pre-integrating their solutions with the larger platforms to reduce the time to value and simplify the systems integration work.
To respond to these emerging threats, existing tech providers will need to not only accelerate their own use of AI in their platforms, but also ramp up the education and promotion of these capabilities.
#2 Lead With Data, Not AI Capabilities

Organisations recognise the need for AI to enhance their workforce, improve customer experience, and automate processes. However, the initial challenge lies in improving data quality, as trust in early AI models hinges on high-quality training data for long-term success.
Tech vendors that can help with data source discovery, metadata analysis, and seamless data pipeline creation will emerge as trusted AI partners. Transformation tools that automate deduplication and quality assurance tasks empower data scientists to focus on high-value work. Automation models like Segment Anything enhance unstructured data labeling, particularly for images. Finally synthetic data will gain importance as quality sources become scarce.
Tech vendors will be tempted to capitalise on the Generative AI hype but for sake of positive early experiences, they should begin with data quality.
#3 Prepare Thoroughly for AI-driven Business Demand

Besides pureplay AI opportunities, AI will drive a renewed and increased interest in data and data management. Tech and service providers can capitalise on this by understanding the larger picture around their clients’ data maturity and governance. Initial conversations around AI can be door openers to bigger, transformational engagements.
Tech vendors should avoid the pitfall of downplaying AI risks. Instead, they should make all efforts to own and drive the conversation with their clients. They need to be forthcoming about their in-house responsible AI guidelines and understand what is happening in AI legislation world-wide (hint: a lot!)
Tech providers must establish strong client partnerships for AI initiatives to succeed. They must address risk and benefit equally to reap the benefits of larger AI-driven transformation engagements.
#4 Converge Network & Security Capabilities

Networking and security vendors will need to develop converged offerings as these two technologies increasingly overlap in the hybrid working era. Organisations are now entering a new phase of maturity as they evolve their remote working policies and invest in tools to regain control. They will require simplified management, increased visibility, and to provide a consistent user experience, wherever employees are located.
There has already been a widespread adoption of SD-WAN and now organisations are starting to explore next generation SSE technologies. Procuring these capabilities from a single provider will help to remove complexity from networks as the number of endpoints continue to grow.
Tech providers should take a land and expand approach, getting a foothold with SASE modules that offer rapid ROI. They should focus on SWG and ZTNA deals with an eye to expanding in CASB and FWaaS, as customers gain experience.
#5 Double Down on Your Partner Ecosystem

The IT services market, particularly in Asia Pacific, is poised for significant growth. Factors, including the imperative to cut IT operational costs, the growing complexity of cloud migrations and transformations, change management for Generative AI capabilities, and rising security and data governance needs, will drive increased spending on IT services.
Tech services providers – consultants, SIs, managed services providers, and VARs – will help drive organisations’ tech spend and strategy. This is a good time to review partners, evaluating whether they can take the business forward, or whether there is a need to expand or change the partner mix.
Partner reviews should start with an evaluation of processes and incentives to ensure they foster desired behaviour from customers and partners. Tech vendors should develop a 21st century partner program to improve chances of success.
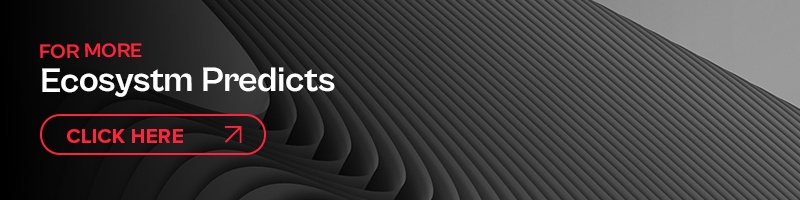
At the end of last year, I had the privilege of attending a session organised by Red Hat where they shared their Asia Pacific roadmap with the tech analyst community. The company’s approach of providing a hybrid cloud application platform centred around OpenShift has worked well with clients who favour a hybrid cloud approach. Going forward, Red Hat is looking to build and expand their business around three product innovation focus areas. At the core is their platform engineering, flanked by focus areas on AI/ML and the Edge.
The Opportunities
Besides the product innovation focus, Red Hat is also looking into several emerging areas, where they’ve seen initial client success in 2023. While use cases such as operational resilience or edge lifecycle management are long-existing trends, carbon-aware workload scheduling may just have appeared over the horizon. But two others stood out for me with a potentially huge demand in 2024.
GPU-as-a-Service. GPUaaS addresses a massive demand driven by the meteoric rise of Generative AI over the past 12 months. Any innovation that would allow customers a more flexible use of scarce and expensive resources such as GPUs can create an immediate opportunity and Red Hat might have a first mover and established base advantage. Particularly GPUaaS is an opportunity in fast growing markets, where cost and availability are strong inhibitors.
Digital Sovereignty. Digital sovereignty has been a strong driver in some markets – for example in Indonesia, which has led to most cloud hyperscalers opening their data centres onshore over the past years. Yet not the least due to the geography of Indonesia, hybrid cloud remains an important consideration, where digital sovereignty needs to be managed across a diverse infrastructure. Other fast-growing markets have similar challenges and a strong drive for digital sovereignty. Crucially, Red Hat may well have an advantage where onshore hyperscalers are not yet available (for example in Malaysia).
Strategic Focus Areas for Red Hat
Red Hat’s product innovation strategy is robust at its core, particularly in platform engineering, but needs more clarity at the periphery. They have already been addressing Edge use cases as an extension of their core platform, especially in the Automotive sector, establishing a solid foundation in this area. Their focus on AI/ML may be a bit more aspirational, as they are looking to not only AI-enable their core platform but also expand it into a platform to run AI workloads. AI may drive interest in hybrid cloud, but it will be in very specific use cases.
For Red Hat to be successful in the AI space, it must steer away from competing straight out with the cloud-native AI platforms. They must identify the use cases where AI on hybrid cloud has a true advantage. Such use cases will mainly exist in industries with a strong Edge component, potentially also with a still heavy reliance on on-site data centres. Manufacturing is the prime example.
Red Hat’s success in AI/ML use cases is tightly connected to their (continuing) success in Edge use cases, all build on the solid platform engineering foundation.
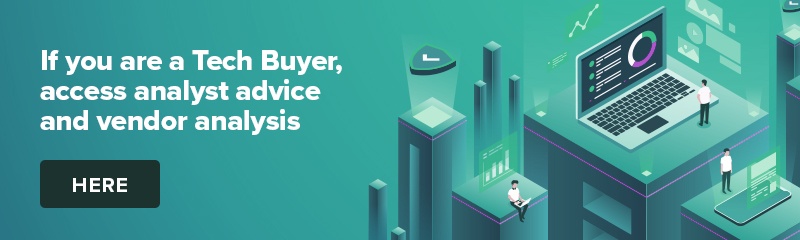