AI is reshaping the tech infrastructure landscape, demanding a fundamental rethinking of organisational infrastructure strategies. Traditional infrastructure, once sufficient, now struggles to keep pace with the immense scale and complexity of AI workloads. To meet these demands, organisations are turning to high-performance computing (HPC) solutions, leveraging powerful GPUs and specialised accelerators to handle the computationally intensive nature of AI algorithms.
Real-time AI applications, from fraud detection to autonomous vehicles, require lightning-fast processing speeds and low latency. This is driving the adoption of high-speed networks and edge computing, enabling data processing closer to the source and reducing response times. AI-driven automation is also streamlining infrastructure management, automating tasks like network provisioning, security monitoring, and capacity planning. This not only reduces operational overhead but also improves efficiency and frees up valuable resources.
Ecosystm analysts Darian Bird, Peter Carr, Simona Dimovski, and Tim Sheedy present the key trends shaping the tech infrastructure market in 2025.
Click here to download ‘Building the AI Future: Top 5 Infra Trends for 2025’ as a PDF
1. The AI Buildout Will Accelerate; China Will Emerge as a Winner
In 2025, the race for AI dominance will intensify, with Nvidia emerging as the big winner despite an impending AI crash. Many over-invested companies will fold, flooding the market with high-quality gear at bargain prices. Meanwhile, surging demand for AI infrastructure – spanning storage, servers, GPUs, networking, and software like observability, hybrid cloud tools, and cybersecurity – will make it a strong year for the tech infrastructure sector.
Ironically, China’s exclusion from US tech deals has spurred its rise as a global tech giant. Forced to develop its own solutions, China is now exporting its technologies to friendly nations worldwide.
By 2025, Chinese chipmakers are expected to rival international peers, with some reaching parity.
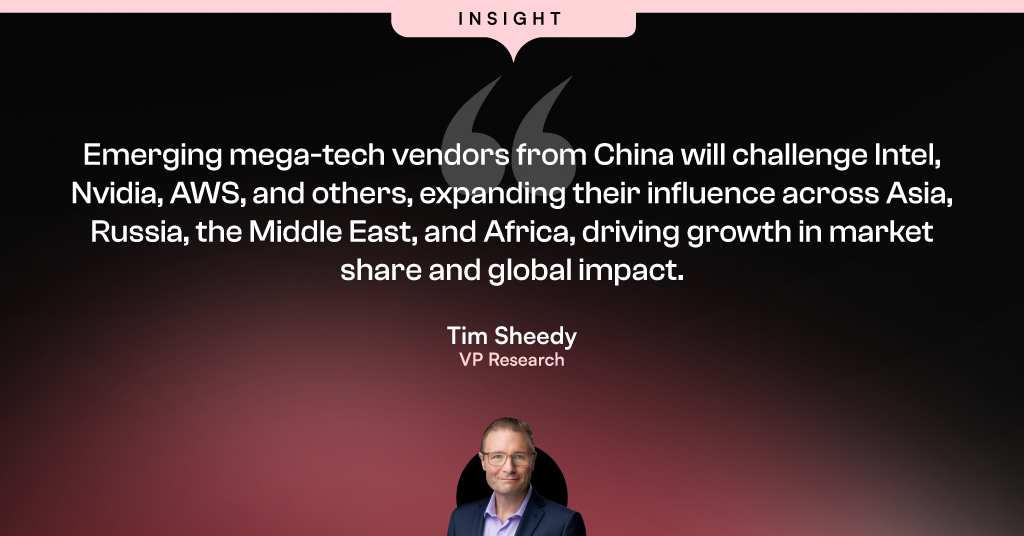
2. AI-Optimised Cloud Platforms Will Dominate Infrastructure Investments
AI-optimised cloud platforms will become the go-to infrastructure for organisations, enabling seamless integration of machine learning capabilities, scalable compute power, and efficient deployment tools.
As regulatory demands grow and AI workloads become more complex, these platforms will provide localised, compliant solutions that meet data privacy laws while delivering superior performance.
This shift will allow businesses to overcome the limitations of traditional infrastructure, democratising access to high-performance AI resources and lowering entry barriers for smaller organisations. AI-optimised cloud platforms will drive operational efficiencies, foster innovation, and help businesses maintain compliance, particularly in highly regulated industries.
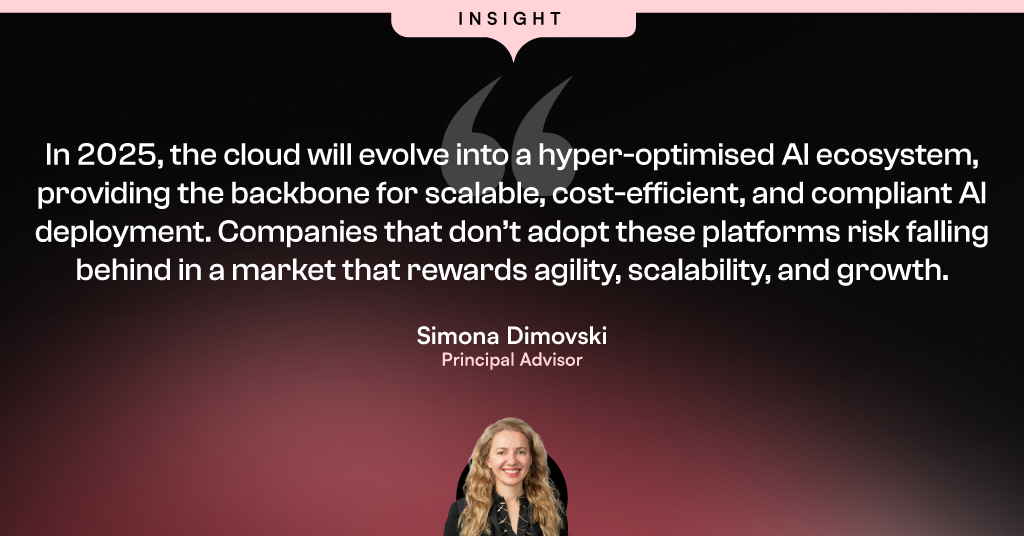
3. PaaS Architecture, Not Data Cleanup, Will Define AI Success
By 2025, as AI adoption reaches new heights, organisations will face an urgent need for AI-ready data, spurring significant investments in data infrastructure. However, the approach taken will be pivotal.
A stark divide will arise between businesses fixated on isolated data-cleaning initiatives and those embracing a Platform-as-a-Service (PaaS) architecture.
The former will struggle, often unintentionally creating more fragmented systems that increase complexity and cybersecurity risks. While data cleansing is important, focusing exclusively on it without a broader architectural vision leads to diminishing returns. On the other hand, organisations adopting PaaS architectures from the start will gain a distinct advantage through seamless integration, centralised data management, and large-scale automation, all critical for AI.
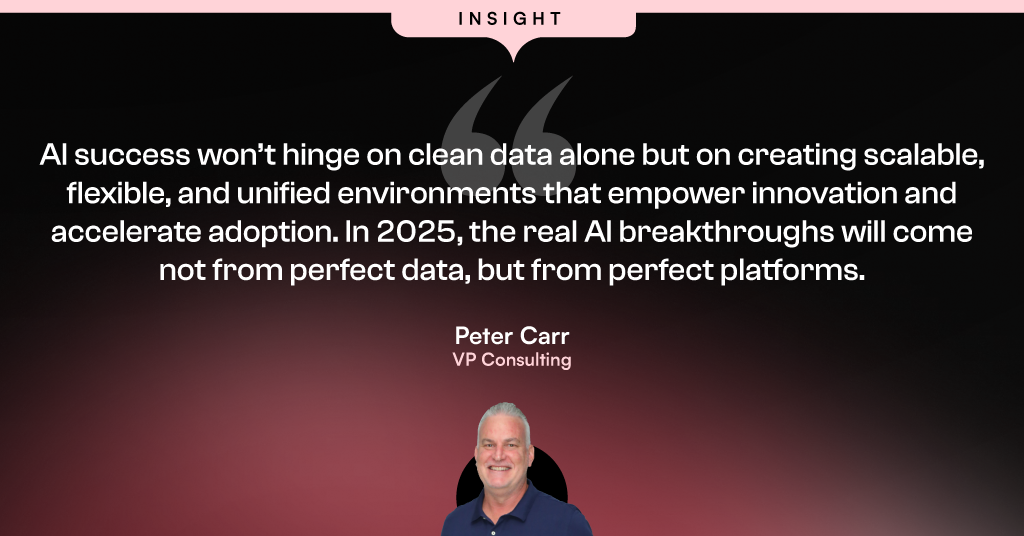
4. Small Language Models Will Push AI to the Edge
While LLMs have captured most of the headlines, small language models (SLMs) will soon help to drive AI use at the edge. These compact but powerful models are designed to operate efficiently on limited hardware, like AI PCs, wearables, vehicles, and robots. Their small size translates into energy efficiency, making them particularly useful in mobile applications. They also help to mitigate the alarming electricity consumption forecasts that could make widespread AI adoption unsustainable.
Self-contained SMLs can function independently of the cloud, allowing them to perform tasks that require low latency or without Internet access.
Connected machines in factories, warehouses, and other industrial environments will have the benefit of AI without the burden of a continuous link to the cloud.
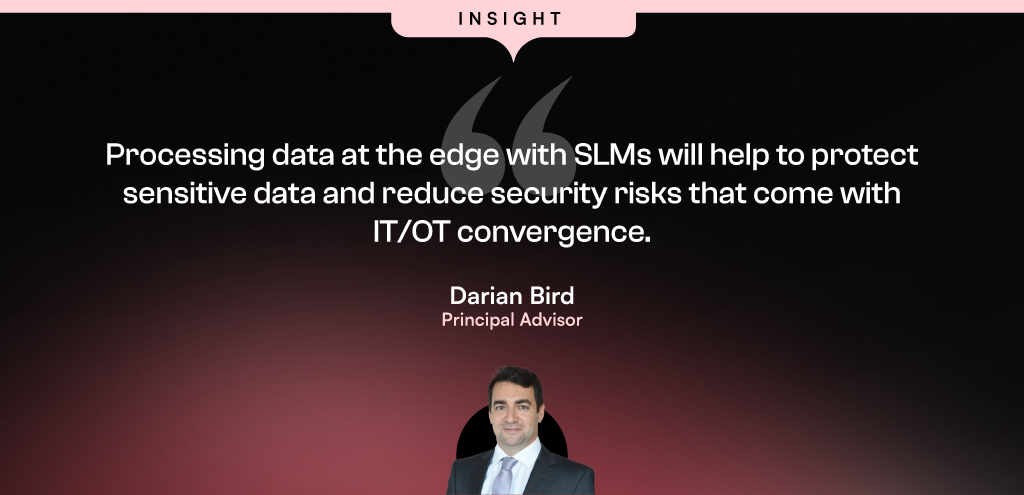
5. The Impact of AI PCs Will Remain Limited
AI PCs have been a key trend in 2024, with most brands launching AI-enabled laptops. However, enterprise feedback has been tepid as user experiences remain unchanged. Most AI use cases still rely on the public cloud, and applications have yet to be re-architected to fully leverage NPUs. Where optimisation exists, it mainly improves graphics efficiency, not smarter capabilities. Currently, the main benefit is extended battery life, explaining the absence of AI in desktop PCs, which don’t rely on batteries.
The market for AI PCs will grow as organisations and consumers adopt them, creating incentives for developers to re-architect software to leverage NPUs.
This evolution will enable better data access, storage, security, and new user-centric capabilities. However, meaningful AI benefits from these devices are still several years away.
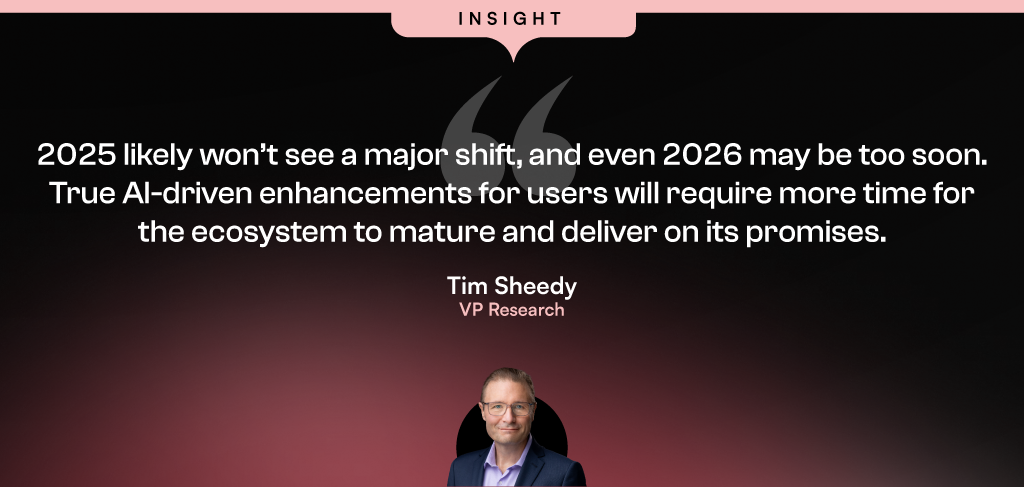
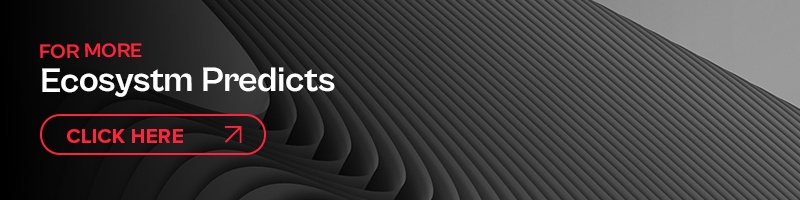
Large organisations in the banking and financial services industry have come a long way over the past two decades in cutting costs, restructuring IT systems and redefining customer relationship management. And, as if that was not enough, they now face the challenge of having to adapt to ongoing global technological shifts or the challenge of having to “do something with AI” without being AI-ready in terms of strategy, skills and culture.
Most organisations in the industry have started approaching AI implementation in a conventional way, based on how they have historically managed IT initiatives. Their first attempts at experimenting with AI have led to rapid conclusions forming seven common myths. However, as experience with AI grows, these myths are gradually being debunked. Let us put these myths to a reality check.
1. We can rely solely on external tech companies
Even in a highly regulated industry like banking and financial services, internal processes and data management practices can vary significantly from one institution to another. Experience shows that while external providers – many of whom lack direct industry experience – can offer solutions tailored to the more obvious use cases and provide customisation, they fall short when it comes to identifying less apparent opportunities and driving fundamental changes in workflows. No one understands an institution’s data better than its own employees. Therefore, a key success factor in AI implementation is active internal ownership, involving employees directly rather than delegating the task entirely to external parties. While technology providers are essential partners, organisations must also cultivate their own internal understanding of AI to ensure successful implementation.
2. AI is here to be applied to single use cases
In the early stages of experimenting with AI, many financial institutions treated it as a side project, focusing on developing minimum viable products and solving isolated problems to explore what worked and what didn’t. Given their inherently risk-averse nature, organisations often approached AI cautiously, addressing one use case at a time to avoid disrupting their broader IT landscape or core business. However, with AI’s potential for deep transformation, the financial services industry has an opportunity not only to address inefficiencies caused by manual, time-consuming tasks but also to question how data is created, captured, and used from the outset. This requires an ecosystem of visionary minds in the industry who join forces and see beyond deal generation.
3. We can staff AI projects with our highly motivated junior employees and let our senior staff focus on what they do best – managing the business
Financial institutions that still view AI as a side hustle, secondary to their day-to-day operations, often assign junior employees to handle AI implementation. However, this can be a mistake. AI projects involve numerous small yet critical decisions, and team members need the authority and experience to make informed judgments that align with the organisation’s goals. Also, resistance to change often comes from those who were not involved in shaping or developing the initiative. Experience shows that project teams with a balanced mix of seniority and diversity in perspectives tend to deliver the best results, ensuring both strategic insight and operational engagement.
4. AI projects do not pay off
Compared to conventional IT projects, the business cases for AI implementation – especially when limited to solving a few specific use cases – often do not pay off over a period of two to three years. Traditional IT projects can usually be executed with minimal involvement of subject matter experts, and their costs are easier to estimate based on reference projects. In contrast, AI projects are highly experimental, requiring multiple iterations, significant involvement from experts, and often lacking comparable reference projects. When AI solutions address only small parts of a process, the benefits may not be immediately apparent. However, if AI is viewed as part of a long-term transformational journey, gradually integrating into all areas of the organisation and unlocking new business opportunities over the next five to ten years, the true value of AI becomes clear. A conventional business case model cannot fully capture this long-term payoff.
5. We are on track with AI if we have several initiatives ongoing
Many financial institutions have begun their AI journey by launching multiple, often unrelated, use case-based projects. The large number of initiatives can give top management a false sense of progress, as if they are fully engaged in AI. However, investors and project teams often ask key questions: Where are these initiatives leading? How do they contribute? What is the AI vision and strategy, and how does it align with the business strategy? If these answers remain unclear, it’s difficult to claim that the organisation is truly on track with AI. To ensure that AI initiatives are truly impactful and aligned with business objectives, organisations must have a clear AI vision and strategy – and not rely on number of initiatives to measure progress.
6. AI implementation projects always exceed their deadlines
AI solutions in the banking and financial services industry are rarely off-the-shelf products. In cases of customisation or in-house development, particularly when multiple model-building iterations and user tests are required, project delays of three to nine months can occur. This is largely because organisations want to avoid rolling out solutions that do not perform reliably. The goal is to ensure that users have a positive experience with AI and embrace the change. Over time, as an organisation becomes more familiar with AI implementation, the process will become faster.
7. We upskill our people by giving them access to AI training
Learning by doing has always been and will remain the most effective way to learn, especially with technology. Research has shown that 90% of knowledge acquired in training is forgotten after a week if it is not applied. For organisations, the best way to digitally upskill employees is to involve them in AI implementation projects, even if it’s just a few hours per week. To evaluate their AI readiness or engagement, organisations could develop new KPIs, such as the average number of hours an employee actively engages in AI implementation or the percentage of employees serving as subject matter experts in AI projects.
Which of these myths have you believed, and where do you already see changes?
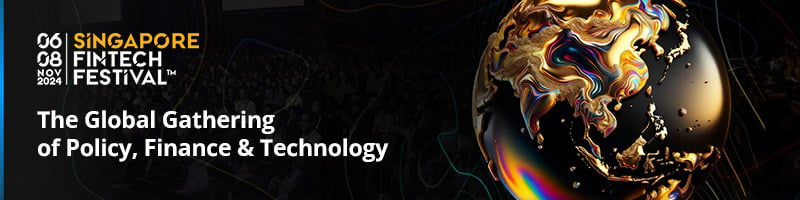
At a recently held Ecosystm roundtable, in partnership with Qlik and 121Connects, Ecosystm Principal Advisor Manoj Chugh, moderated a conversation where Indian tech and data leaders discussed building trust in data strategies. They explored ways to automate data pipelines and improve governance to drive better decisions and business outcomes. Here are the key takeaways from the session.

Data isn’t just a byproduct anymore; it’s the lifeblood of modern businesses, fuelling informed decisions and strategic growth. But with vast amounts of data, the challenge isn’t just managing it; it’s building trust. AI, once a beacon of hope, is now at risk without a reliable data foundation. Ecosystm research reveals that a staggering 66% of Indian tech leaders doubt their organisation’s data quality, and the problem of data silos is exacerbating this trust crisis.
At the Leaders Roundtable in Mumbai, I had the opportunity to moderate a discussion among data and digital leaders on the critical components of building trust in data and leveraging it to drive business value. The consensus was that building trust requires a comprehensive strategy that addresses the complexities of data management and positions the organisation for future success. Here are the key strategies that are essential for achieving these goals.
1. Adopting a Unified Data Approach
Organisations are facing a growing wave of complex workloads and business initiatives. To manage this expansion, IT teams are turning to multi-cloud, SaaS, and hybrid environments. However, this diverse landscape introduces new challenges, such as data silos, security vulnerabilities, and difficulties in ensuring interoperability between systems.

A unified data strategy is crucial to overcome these challenges. By ensuring platform consistency, robust security, and seamless data integration, organisations can simplify data management, enhance security, and align with business goals – driving informed decisions, innovation, and long-term success.
Real-time data integration is essential for timely data availability, enabling organisations to make data-driven decisions quickly and effectively. By integrating data from various sources in real-time, businesses can gain valuable insights into their operations, identify trends, and respond to changing market conditions.
Organisations that are able to integrate their IT and operational technology (OT) systems find their data accuracy increasing. By combining IT’s digital data management expertise with OT’s real-time operational insights, organisations can ensure more accurate, timely, and actionable data. This integration enables continuous monitoring and analysis of operational data, leading to faster identification of errors, more precise decision-making, and optimised processes.
2. Enhancing Data Quality with Automation and Collaboration
As the volume and complexity of data continue to grow, ensuring high data quality is essential for organisations to make accurate decisions and to drive trust in data-driven solutions. Automated data quality tools are useful for cleansing and standardising data to eliminate errors and inconsistencies.
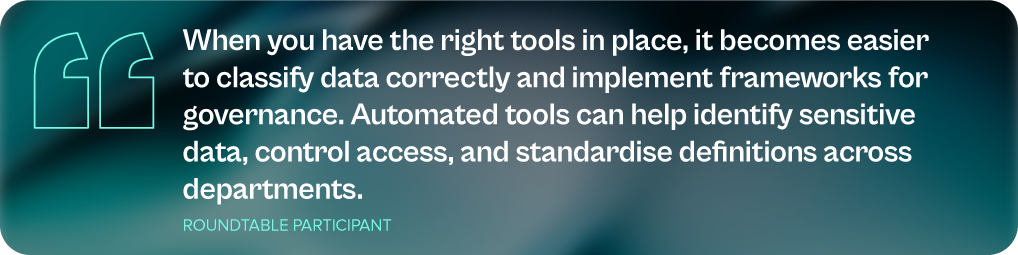
As mentioned earlier, integrating IT and OT systems can help organisations improve operational efficiency and resilience. By leveraging data-driven insights, businesses can identify bottlenecks, optimise workflows, and proactively address potential issues before they escalate. This can lead to cost savings, increased productivity, and improved customer satisfaction.
However, while automation technologies can help, organisations must also invest in training employees in data management, data visualisation, and data governance.
3. Modernising Data Infrastructure for Agility and Innovation
In today’s fast-paced business landscape, agility is paramount. Modernising data infrastructure is essential to remain competitive – the right digital infrastructure focuses on optimising costs, boosting capacity and agility, and maximising data leverage, all while safeguarding the organisation from cyber threats. This involves migrating data lakes and warehouses to cloud platforms and adopting advanced analytics tools. However, modernisation efforts must be aligned with specific business goals, such as enhancing customer experiences, optimising operations, or driving innovation. A well-modernised data environment not only improves agility but also lays the foundation for future innovations.

Technology leaders must assess whether their data architecture supports the organisation’s evolving data requirements, considering factors such as data flows, necessary management systems, processing operations, and AI applications. The ideal data architecture should be tailored to the organisation’s specific needs, considering current and future data demands, available skills, costs, and scalability.
4. Strengthening Data Governance with a Structured Approach
Data governance is crucial for establishing trust in data, and providing a framework to manage its quality, integrity, and security throughout its lifecycle. By setting clear policies and processes, organisations can build confidence in their data, support informed decision-making, and foster stakeholder trust.
A key component of data governance is data lineage – the ability to trace the history and transformation of data from its source to its final use. Understanding this journey helps organisations verify data accuracy and integrity, ensure compliance with regulatory requirements and internal policies, improve data quality by proactively addressing issues, and enhance decision-making through context and transparency.
A tiered data governance structure, with strategic oversight at the executive level and operational tasks managed by dedicated data governance councils, ensures that data governance aligns with broader organisational goals and is implemented effectively.
Are You Ready for the Future of AI?
The ultimate goal of your data management and discovery mechanisms is to ensure that you are advancing at pace with the industry. The analytics landscape is undergoing a profound transformation, promising to revolutionise how organisations interact with data. A key innovation, the data fabric, is enabling organisations to analyse unstructured data, where the true value often lies, resulting in cleaner and more reliable data models.
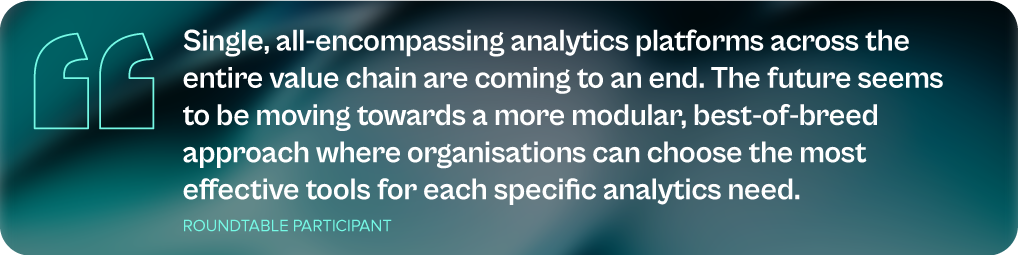
GenAI has emerged as another game-changer, empowering employees across the organisation to become citizen data scientists. This democratisation of data analytics allows for a broader range of insights and fosters a more data-driven culture. Organisations can leverage GenAI to automate tasks, generate new ideas, and uncover hidden patterns in their data.
The shift from traditional dashboards to real-time conversational tools is also reshaping how data insights are delivered and acted upon. These tools enable users to ask questions in natural language, receiving immediate and relevant answers based on the underlying data. This conversational approach makes data more accessible and actionable, empowering employees to make data-driven decisions at all levels of the organisation.
To fully capitalise on these advancements, organisations need to reassess their AI/ML strategies. By ensuring that their tech initiatives align with their broader business objectives and deliver tangible returns on investment, organisations can unlock the full potential of data-driven insights and gain a competitive edge. It is equally important to build trust in AI initiatives, through a strong data foundation. This involves ensuring data quality, accuracy, and consistency, as well as implementing robust data governance practices. A solid data foundation provides the necessary groundwork for AI and GenAI models to deliver reliable and valuable insights.
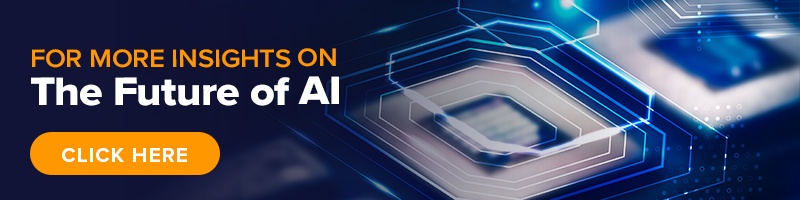
The global data protection landscape is growing increasingly complex. With the proliferation of privacy laws across jurisdictions, organisations face a daunting challenge in ensuring compliance.
From the foundational GDPR, the evolving US state-level regulations, to new regulations in emerging markets, businesses with cross-border presence must navigate a maze of requirements to protect consumer data. This complexity, coupled with the rapid pace of regulatory change, requires proactive and strategic approaches to data management and protection.
GDPR: The Catalyst for Global Data Privacy
At the forefront of this global push for data privacy stands the General Data Protection Regulation (GDPR) – a landmark legislation that has reshaped data governance both within the EU and beyond. It has become a de facto standard for data management, influencing the creation of similar laws in countries like India, China, and regions such as Southeast Asia and the US.
However, the GDPR is evolving to tackle new challenges and incorporate lessons from past data breaches. Amendments aim to enhance enforcement, especially in cross-border cases, expedite complaint handling, and strengthen breach penalties. Amendments to the GDPR in 2024 focus on improving enforcement efficiency. The One-Stop-Shop mechanism will be strengthened for better handling of cross-border data processing, with clearer guidelines for lead supervisory authority and faster information sharing. Deadlines for cross-border decisions will be shortened, and Data Protection Authorities (DPAs) must cooperate more closely. Rules for data transfers to third countries will be clarified, and DPAs will have stronger enforcement powers, including higher fines for non-compliance.
For organisations, these changes mean increased scrutiny and potential penalties due to faster investigations. Improved DPA cooperation can lead to more consistent enforcement across the EU, making it crucial to stay updated and adjust data protection practices. While aiming for more efficient GDPR enforcement, these changes may also increase compliance costs.
GDPR’s Global Impact: Shaping Data Privacy Laws Worldwide
Despite being drafted by the EU, the GDPR has global implications, influencing data privacy laws worldwide, including in Canada and the US.
Canada’s Personal Information Protection and Electronic Documents Act (PIPEDA) governs how the private sector handles personal data, emphasising data minimisation and imposing fines of up to USD 75,000 for non-compliance.
The US data protection landscape is a patchwork of state laws influenced by the GDPR and PIPEDA. The California Privacy Rights Act (CPRA) and other state laws like Virginia’s CDPA and Colorado’s CPA reflect GDPR principles, requiring transparency and limiting data use. Proposed federal legislation, such as the American Data Privacy and Protection Act (ADPPA), aims to establish a national standard similar to PIPEDA.
The GDPR’s impact extends beyond EU borders, significantly influencing data protection laws in non-EU European countries. Countries like Switzerland, Norway, and Iceland have closely aligned their regulations with GDPR to maintain data flows with the EU. Switzerland, for instance, revised its Federal Data Protection Act to ensure compatibility with GDPR standards. The UK, post-Brexit, retained a modified version of GDPR in its domestic law through the UK GDPR and Data Protection Act 2018. Even countries like Serbia and North Macedonia, aspiring for EU membership, have modeled their data protection laws on GDPR principles.
Data Privacy: A Local Flavour in Emerging Markets
Emerging markets are recognising the critical need for robust data protection frameworks. These countries are not just following in the footsteps of established regulations but are creating laws that address their unique economic and cultural contexts while aligning with global standards.
Brazil has over 140 million internet users – the 4th largest in the world. Any data collection or processing within the country is protected by the Lei Geral de Proteção de Dados (or LGPD), even from data processors located outside of Brazil. The LGPD also mandates organisations to appoint a Data Protection Officer (DPO) and establishes the National Data Protection Authority (ANPD) to oversee compliance and enforcement.
Saudi Arabia’s Personal Data Protection Law (PDPL) requires explicit consent for data collection and use, aligning with global norms. However, it is tailored to support Saudi Arabia’s digital transformation goals. The PDPL is overseen by the Saudi Data and Artificial Intelligence Authority (SDAIA), linking data protection with the country’s broader AI and digital innovation initiatives.
Closer Home: Changes in Asia Pacific Regulations
The Asia Pacific region is experiencing a surge in data privacy regulations as countries strive to protect consumer rights and align with global standards.
Japan. Japan’s Act on the Protection of Personal Information (APPI) is set for a major overhaul in 2025. Certified organisations will have more time to report data breaches, while personal data might be used for AI training without consent. Enhanced data rights are also being considered, giving individuals greater control over biometric and children’s data. The government is still contemplating the introduction of administrative fines and collective action rights, though businesses have expressed concerns about potential negative impacts.
South Korea. South Korea has strengthened its data protection laws with significant amendments to the Personal Information Protection Act (PIPA), aiming to provide stronger safeguards for individual personal data. Key changes include stricter consent requirements, mandatory breach notifications within 72 hours, expanded data subject rights, refined data processing guidelines, and robust safeguards for emerging technologies like AI and IoT. There are also increased penalties for non-compliance.
China. China’s Personal Information Protection Law (PIPL) imposes stringent data privacy controls, emphasising user consent, data minimisation, and restricted cross-border data transfers. Severe penalties underscore the nation’s determination to safeguard personal information.
Southeast Asia. Southeast Asian countries are actively enhancing their data privacy landscapes. Singapore’s PDPA mandates breach notifications and increased fines. Malaysia is overhauling its data protection law, while Thailand’s PDPA has also recently come into effect.
Spotlight: India’s DPDP Act
The Digital Personal Data Protection Act, 2023 (DPDP Act), officially notified about a year ago, is anticipated to come into effect soon. This principles-based legislation shares similarities with the GDPR and applies to personal data that identifies individuals, whether collected digitally or digitised later. It excludes data used for personal or domestic purposes, aggregated research data, and publicly available information. The Act adopts GDPR-like territorial rules but does not extend to entities outside India that monitor behaviour within the country.
Consent under the DPDP Act must be free, informed, and specific, with companies required to provide a clear and itemised notice. Unlike the GDPR, the Act permits processing without consent for certain legitimate uses, such as legal obligations or emergencies. It also categorises data fiduciaries based on the volume and sensitivity of the data they handle, imposing additional obligations on significant data fiduciaries while offering exemptions for smaller entities. The Act simplifies cross-border data transfers compared to the GDPR, allowing transfers to all countries unless restricted by the Indian Government. It also provides broad exemptions to the State for data processing under specific conditions. Penalties for breaches are turnover agnostic, with considerations for breach severity and mitigating actions. The full impact of the DPDP Act will be clearer once the rules are finalised and the Board becomes operational, but 97% of Indian organisations acknowledge that it will affect them.
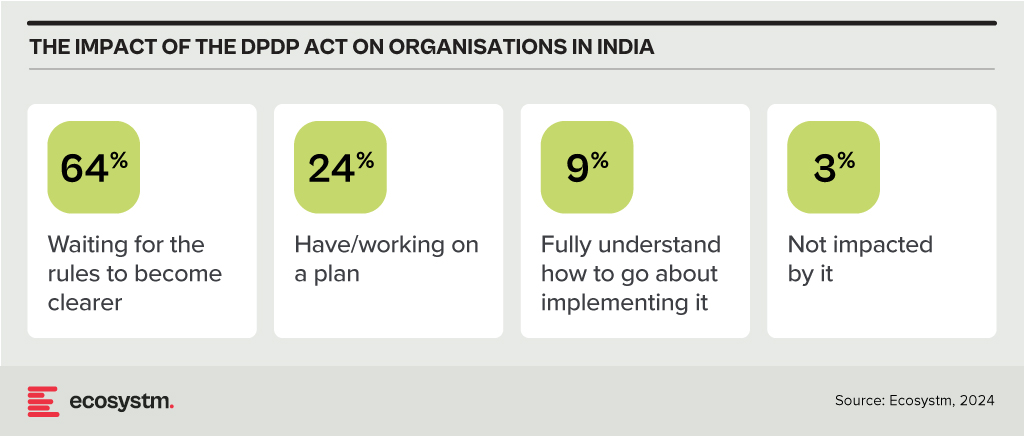
Conclusion
Data breaches pose significant risks to organisations, requiring a strong data protection strategy that combines technology and best practices. Key technological safeguards include encryption, identity access management (IAM), firewalls, data loss prevention (DLP) tools, tokenisation, and endpoint protection platforms (EPP). Along with technology, organisations should adopt best practices such as inventorying and classifying data, minimising data collection, maintaining transparency with customers, providing choices, and developing comprehensive privacy policies. Training employees and designing privacy-focused processes are also essential. By integrating robust technology with informed human practices, organisations can enhance their overall data protection strategy.
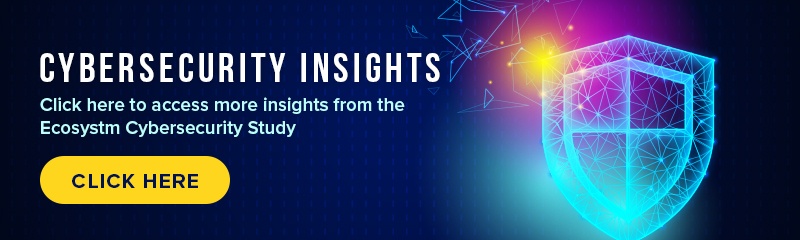
In my previous blogs, I outlined strategies for public sector organisations to incorporate technology into citizen services and internal processes. Building on those perspectives, let’s talk about the critical role of data in powering digital transformation across the public sector.
Effectively leveraging data is integral to delivering enhanced digital services and streamlining operations. Organisations must adopt a forward-looking roadmap that accounts for different data maturity levels – from core data foundations and emerging catalysts to future-state capabilities.
1. Data Essentials: Establishing the Bedrock
Data model. At the core of developing government e-services portals, strategic data modelling establishes the initial groundwork for scalable data infrastructures that can support future analytics, AI, and reporting needs. Effective data models define how information will be structured and analysed as data volumes grow. Beginning with an Entity-Relationship model, these blueprints guide the implementation of database schemas within database management systems (DBMS). This foundational approach ensures that the data infrastructure can accommodate the vast amounts of data generated by public services, crucial for maintaining public trust in government systems.
Cloud Databases. Cloud databases provide flexible, scalable, and cost-effective storage solutions, allowing public sector organisations to handle vast amounts of data generated by public services. Data warehouses, on the other hand, are centralised repositories designed to store structured data, enabling advanced querying and reporting capabilities. This combination allows for robust data analytics and AI-driven insights, ensuring that the data infrastructure can support future growth and evolving analytical needs.
Document management. Incorporating a document or records management system (DMS/RMS) early in the data portfolio of a government e-services portal is crucial for efficient operations. This system organises extensive paperwork and records like applications, permits, and legal documents systematically. It ensures easy storage, retrieval, and management, preventing issues with misplaced documents.
Emerging Catalysts: Unleashing Data’s Potential
Digital Twins. A digital twin is a sophisticated virtual model of a physical object or system. It surpasses traditional reporting methods through advanced analytics, including predictive insights and data mining. By creating detailed virtual replicas of infrastructure, utilities, and public services, digital twins allow for real-time monitoring, efficient resource management, and proactive maintenance. This holistic approach contributes to more efficient, sustainable, and livable cities, aligning with broader goals of urban development and environmental sustainability.
Data Fabric. Data Fabric, including Data Lakes and Data Lakehouses, represents a significant leap in managing complex data environments. It ensures data is accessible for various analyses and processing needs across platforms. Data Lakes store raw data in its original format, crucial for initial data collection when future data uses are uncertain. In Cloud DB or Data Fabric setups, Data Lakes play a foundational role by storing unprocessed or semi-structured data. Data Lakehouses combine Data Lakes’ storage with data warehouses’ querying capabilities, offering flexibility, and efficiency for handling different types of data in sophisticated environments.
Data Exchange and MOUs. Even with advanced data management technologies like data fabrics, Data Lakes, and Data Lakehouses, achieving higher maturity in digital government ecosystems often depends on establishing data-sharing agreements. Memorandums of Understanding (MoUs) exemplify these agreements, crucial for maximising efficiency and collaboration. MoUs outline terms, conditions, and protocols for sharing data beyond regulatory requirements, defining its scope, permitted uses, governance standards, and responsibilities of each party. This alignment ensures data integrity, privacy, and security while facilitating collaboration that enhances innovation and service delivery. Such agreements also pave the way for potential commercialisation of shared data resources, opening new market opportunities.
Future-Forward Capabilities: Pioneering New Frontiers
Data Mesh. Data Mesh is a decentralised approach to data architecture and organisational design, ideal for complex stakeholder ecosystems like digital conveyancing solutions. Unlike centralised models, Data Mesh allows each domain to manage its data independently. This fosters collaboration while ensuring secure and governed data sharing, essential for efficient conveyancing processes. Data Mesh enhances data quality and relevance by holding stakeholders directly accountable for their data, promoting integrity and adaptability to market changes. Its focus on interoperability and self-service data access enhances user satisfaction and operational efficiency, catering flexibly to diverse user needs within the conveyancing ecosystem.
Data Embassies. A Data Embassy stores and processes data in a foreign country under the legal jurisdiction of its origin country, beneficial for digital conveyancing solutions serving international markets. This approach ensures data security and sovereignty, governed by the originating nation’s laws to uphold privacy and legal integrity in conveyancing transactions. Data Embassies enhance resilience against physical and cyber threats by distributing data across international locations, ensuring continuous operation despite disruptions. They also foster international collaboration and trust, potentially attracting more investment and participation in global real estate markets. Technologically, Data Embassies rely on advanced data centres, encryption, cybersecurity, cloud, and robust disaster recovery solutions to maintain uninterrupted conveyancing services and compliance with global standards.
Conclusion
By developing a cohesive roadmap that progressively integrates cutting-edge architectures, cross-stakeholder partnerships, and avant-garde juridical models, agencies can construct a solid data ecosystem. One where information doesn’t just endure disruption, but actively facilitates organisational resilience and accelerates mission impact. Investing in an evolutionary data strategy today lays the crucial groundwork for delivering intelligent, insight-driven public services for decades to come. The time to fortify data’s transformative potential is now.
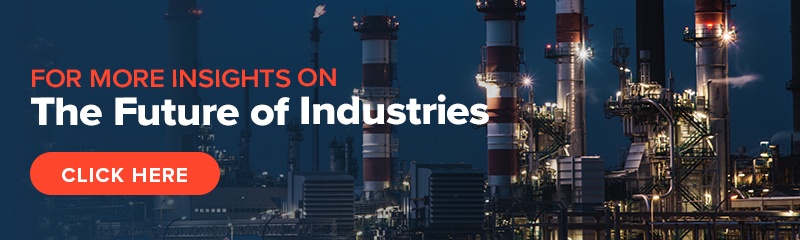
In my last Ecosystm Insight, I spoke about the importance of data architecture in defining the data flow, data management systems required, the data processing operations, and AI applications. Data Mesh and Data Fabric are both modern architectural approaches designed to address the complexities of managing and accessing data across a large organisation. While they share some commonalities, such as improving data accessibility and governance, they differ significantly in their methodologies and focal points.
Data Mesh
- Philosophy and Focus. Data Mesh is primarily focused on the organisational and architectural approach to decentralise data ownership and governance. It treats data as a product, emphasising the importance of domain-oriented decentralised data ownership and architecture. The core principles of Data Mesh include domain-oriented decentralised data ownership, data as a product, self-serve data infrastructure as a platform, and federated computational governance.
- Implementation. In a Data Mesh, data is managed and owned by domain-specific teams who are responsible for their data products from end to end. This includes ensuring data quality, accessibility, and security. The aim is to enable these teams to provide and consume data as products, improving agility and innovation.
- Use Cases. Data Mesh is particularly effective in large, complex organisations with many independent teams and departments. It’s beneficial when there’s a need for agility and rapid innovation within specific domains or when the centralisation of data management has become a bottleneck.
Data Fabric
- Philosophy and Focus. Data Fabric focuses on creating a unified, integrated layer of data and connectivity across an organisation. It leverages metadata, advanced analytics, and AI to improve data discovery, governance, and integration. Data Fabric aims to provide a comprehensive and coherent data environment that supports a wide range of data management tasks across various platforms and locations.
- Implementation. Data Fabric typically uses advanced tools to automate data discovery, governance, and integration tasks. It creates a seamless environment where data can be easily accessed and shared, regardless of where it resides or what format it is in. This approach relies heavily on metadata to enable intelligent and automated data management practices.
- Use Cases. Data Fabric is ideal for organisations that need to manage large volumes of data across multiple systems and platforms. It is particularly useful for enhancing data accessibility, reducing integration complexity, and supporting data governance at scale. Data Fabric can benefit environments where there’s a need for real-time data access and analysis across diverse data sources.
Both approaches aim to overcome the challenges of data silos and improve data accessibility, but they do so through different methodologies and with different priorities.
Data Mesh and Data Fabric Vendors
The concepts of Data Mesh and Data Fabric are supported by various vendors, each offering tools and platforms designed to facilitate the implementation of these architectures. Here’s an overview of some key players in both spaces:
Data Mesh Vendors
Data Mesh is more of a conceptual approach than a product-specific solution, focusing on organisational structure and data decentralisation. However, several vendors offer tools and platforms that support the principles of Data Mesh, such as domain-driven design, product thinking for data, and self-serve data infrastructure:
- Thoughtworks. As the originator of the Data Mesh concept, Thoughtworks provides consultancy and implementation services to help organisations adopt Data Mesh principles.
- Starburst. Starburst offers a distributed SQL query engine (Starburst Galaxy) that allows querying data across various sources, aligning with the Data Mesh principle of domain-oriented, decentralised data ownership.
- Databricks. Databricks provides a unified analytics platform that supports collaborative data science and analytics, which can be leveraged to build domain-oriented data products in a Data Mesh architecture.
- Snowflake. With its Data Cloud, Snowflake facilitates data sharing and collaboration across organisational boundaries, supporting the Data Mesh approach to data product thinking.
- Collibra. Collibra provides a data intelligence cloud that offers data governance, cataloguing, and privacy management tools essential for the Data Mesh approach. By enabling better data discovery, quality, and policy management, Collibra supports the governance aspect of Data Mesh.
Data Fabric Vendors
Data Fabric solutions often come as more integrated products or platforms, focusing on data integration, management, and governance across a diverse set of systems and environments:
- Informatica. The Informatica Intelligent Data Management Cloud includes features for data integration, quality, governance, and metadata management that are core to a Data Fabric strategy.
- Talend. Talend provides data integration and integrity solutions with strong capabilities in real-time data collection and governance, supporting the automated and comprehensive approach of Data Fabric.
- IBM. IBM’s watsonx.data is a fully integrated data and AI platform that automates the lifecycle of data across multiple clouds and systems, embodying the Data Fabric approach to making data easily accessible and governed.
- TIBCO. TIBCO offers a range of products, including TIBCO Data Virtualization and TIBCO EBX, that support the creation of a Data Fabric by enabling comprehensive data management, integration, and governance.
- NetApp. NetApp has a suite of cloud data services that provide a simple and consistent way to integrate and deliver data across cloud and on-premises environments. NetApp’s Data Fabric is designed to enhance data control, protection, and freedom.
The choice of vendor or tool for either Data Mesh or Data Fabric should be guided by the specific needs, existing technology stack, and strategic goals of the organisation. Many vendors provide a range of capabilities that can support different aspects of both architectures, and the best solution often involves a combination of tools and platforms. Additionally, the technology landscape is rapidly evolving, so it’s wise to stay updated on the latest offerings and how they align with the organisation’s data strategy.
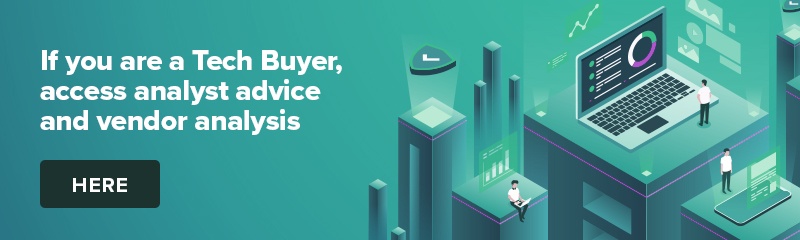
The data architecture outlines how data is managed in an organisation and is crucial for defining the data flow, data management systems required, the data processing operations, and AI applications. Data architects and engineers define data models and structures based on these requirements, supporting initiatives like data science. Before we delve into the right data architecture for your AI journey, let’s talk about the data management options. Technology leaders have the challenge of deciding on a data management system that takes into consideration factors such as current and future data needs, available skills, costs, and scalability. As data strategies become vital to business success, selecting the right data management system is crucial for enabling data-driven decisions and innovation.
Data Warehouse
A Data Warehouse is a centralised repository that stores vast amounts of data from diverse sources within an organisation. Its main function is to support reporting and data analysis, aiding businesses in making informed decisions. This concept encompasses both data storage and the consolidation and management of data from various sources to offer valuable business insights. Data Warehousing evolves alongside technological advancements, with trends like cloud-based solutions, real-time capabilities, and the integration of AI and machine learning for predictive analytics shaping its future.
Core Characteristics
- Integrated. It integrates data from multiple sources, ensuring consistent definitions and formats. This often includes data cleansing and transformation for analysis suitability.
- Subject-Oriented. Unlike operational databases, which prioritise transaction processing, it is structured around key business subjects like customers, products, and sales. This organisation facilitates complex queries and analysis.
- Non-Volatile. Data in a Data Warehouse is stable; once entered, it is not deleted. Historical data is retained for analysis, allowing for trend identification over time.
- Time-Variant. It retains historical data for trend analysis across various time periods. Each entry is time-stamped, enabling change tracking and trend analysis.
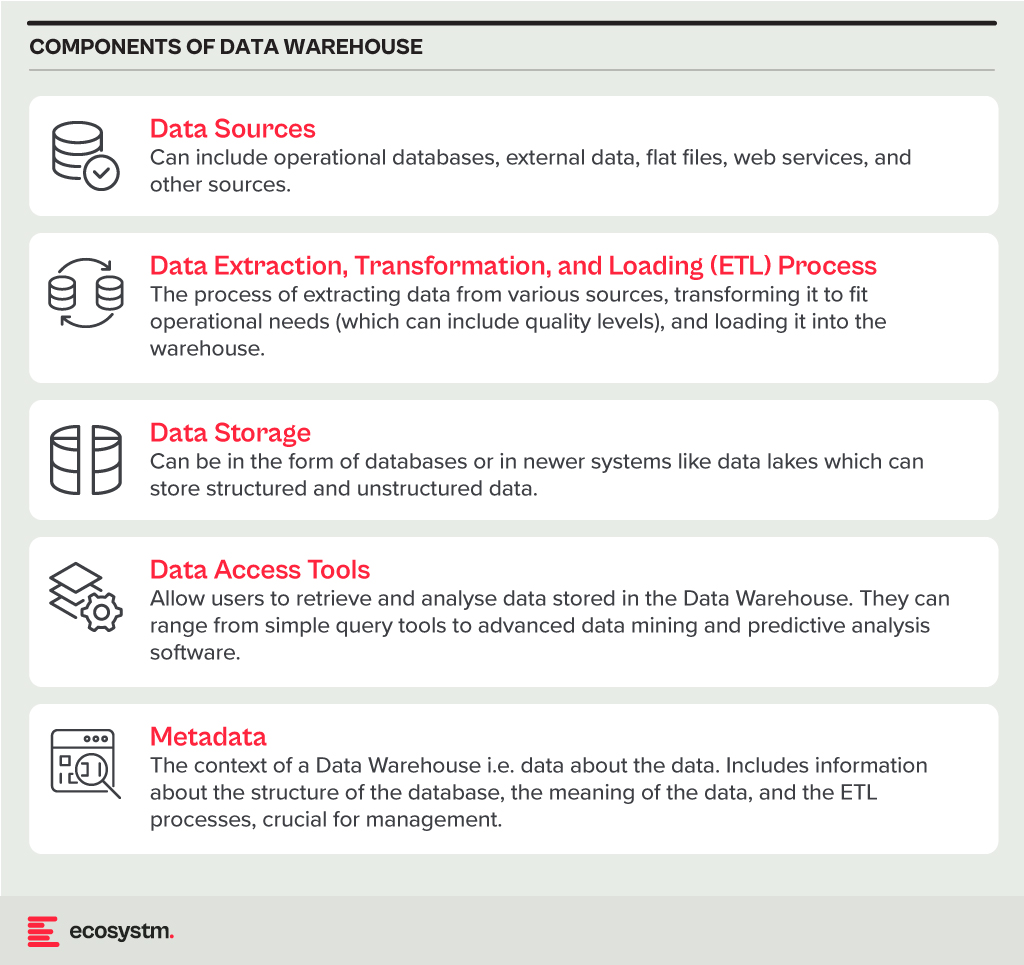
Benefits
- Better Decision Making. Data Warehouses consolidate data from multiple sources, offering a comprehensive business view for improved decision-making.
- Enhanced Data Quality. The ETL process ensures clean and consistent data entry, crucial for accurate analysis.
- Historical Analysis. Storing historical data enables trend analysis over time, informing future strategies.
- Improved Efficiency. Data Warehouses enable swift access and analysis of relevant data, enhancing efficiency and productivity.
Challenges
- Complexity. Designing and implementing a Data Warehouse can be complex and time-consuming.
- Cost. The cost of hardware, software, and specialised personnel can be significant.
- Data Security. Storing large amounts of sensitive data in one place poses security risks, requiring robust security measures.
Data Lake
A Data Lake is a centralised repository for storing, processing, and securing large volumes of structured and unstructured data. Unlike traditional Data Warehouses, which are structured and optimised for analytics with predefined schemas, Data Lakes retain raw data in its native format. This flexibility in data usage and analysis makes them crucial in modern data architecture, particularly in the age of big data and cloud.
Core Characteristics
- Schema-on-Read Approach. This means the data structure is not defined until the data is read for analysis. This offers more flexible data storage compared to the schema-on-write approach of Data Warehouses.
- Support for Multiple Data Types. Data Lakes accommodate diverse data types, including structured (like databases), semi-structured (like JSON, XML files), unstructured (like text and multimedia files), and binary data.
- Scalability. Designed to handle vast amounts of data, Data Lakes can easily scale up or down based on storage needs and computational demands, making them ideal for big data applications.
- Versatility. Data Lakes support various data operations, including batch processing, real-time analytics, machine learning, and data visualisation, providing a versatile platform for data science and analytics.
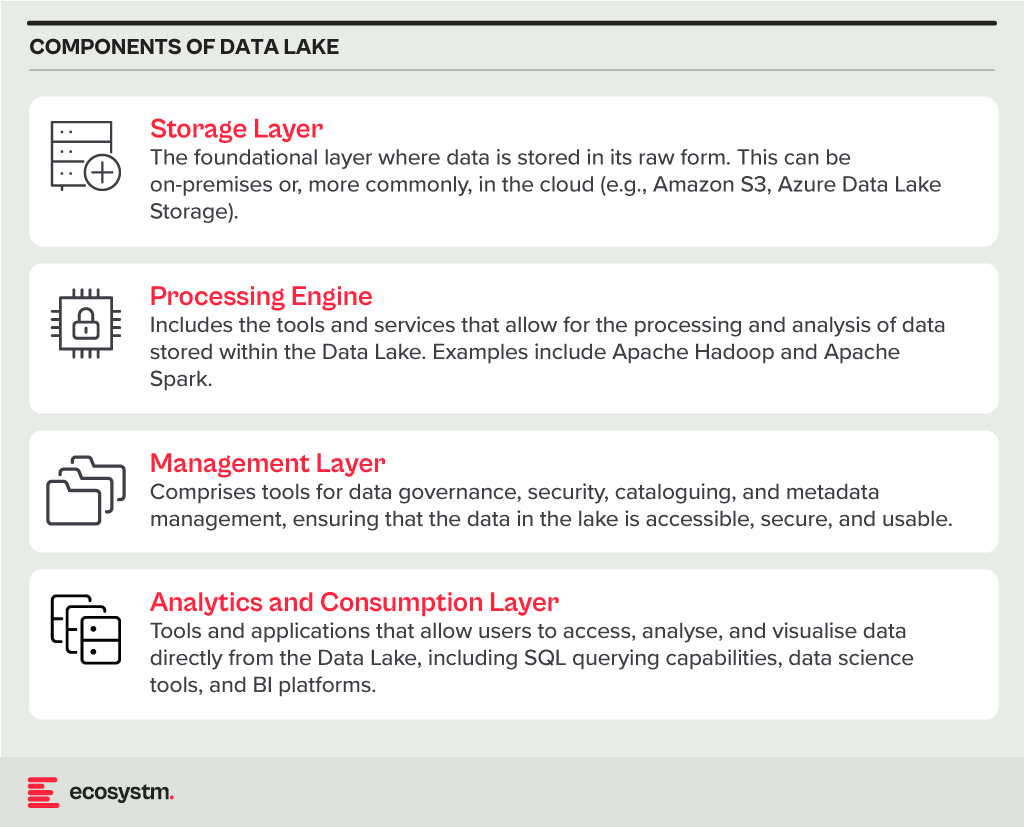
Benefits
- Flexibility. Data Lakes offer diverse storage formats and a schema-on-read approach for flexible analysis.
- Cost-Effectiveness. Cloud-hosted Data Lakes are cost-effective with scalable storage solutions.
- Advanced Analytics Capabilities. The raw, granular data in Data Lakes is ideal for advanced analytics, machine learning, and AI applications, providing deeper insights than traditional data warehouses.
Challenges
- Complexity and Management. Without proper management, a Data Lake can quickly become a “Data Swamp” where data is disorganised and unusable.
- Data Quality and Governance. Ensuring the quality and governance of data within a Data Lake can be challenging, requiring robust processes and tools.
- Security. Protecting sensitive data within a Data Lake is crucial, requiring comprehensive security measures.
Data Lakehouse
A Data Lakehouse is an innovative data management system that merges the strengths of Data Lakes and Data Warehouses. This hybrid approach strives to offer the adaptability and expansiveness of a Data Lake for housing extensive volumes of raw, unstructured data, while also providing the structured, refined data functionalities typical of a Data Warehouse. By bridging the gap between these two traditional data storage paradigms, Lakehouses enable more efficient data analytics, machine learning, and business intelligence operations across diverse data types and use cases.
Core Characteristics
- Unified Data Management. A Lakehouse streamlines data governance and security by managing both structured and unstructured data on one platform, reducing organizational data silos.
- Schema Flexibility. It supports schema-on-read and schema-on-write, allowing data to be stored and analysed flexibly. Data can be ingested in raw form and structured later or structured at ingestion.
- Scalability and Performance. Lakehouses scale storage and compute resources independently, handling large data volumes and complex analytics without performance compromise.
- Advanced Analytics and Machine Learning Integration. By providing direct access to both raw and processed data on a unified platform, Lakehouses facilitate advanced analytics, real-time analytics, and machine learning.
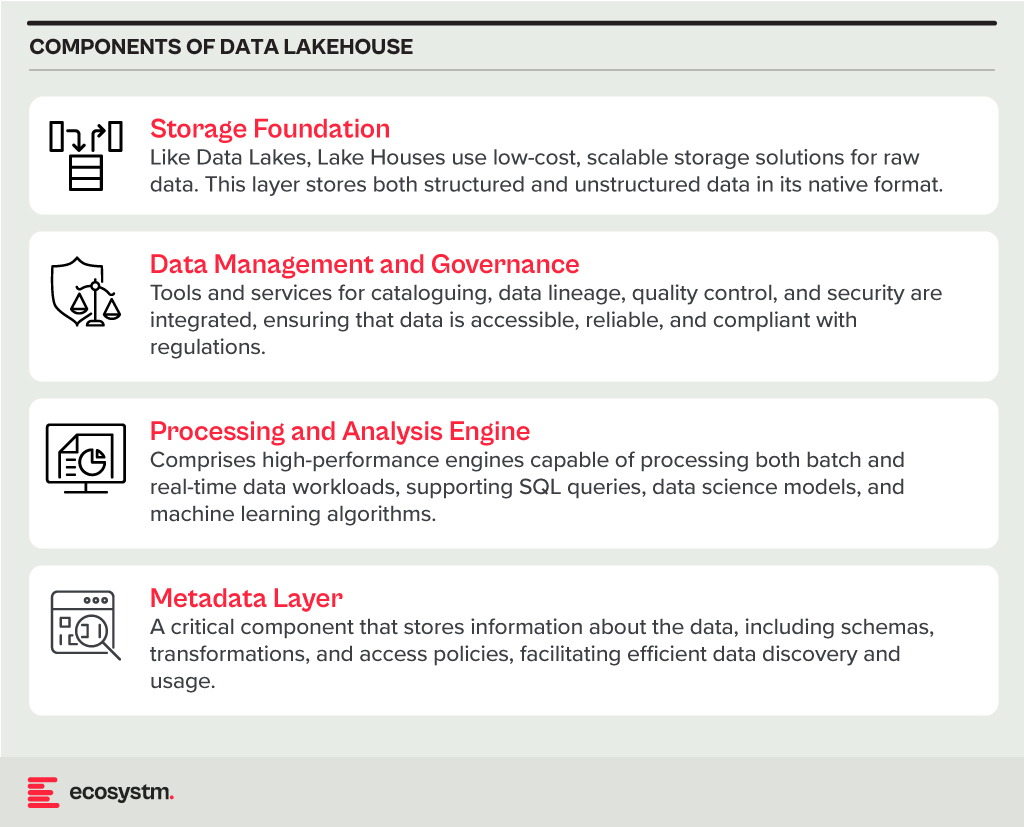
Benefits
- Versatility in Data Analysis. Lakehouses support diverse data analytics, spanning from traditional BI to advanced machine learning, all within one platform.
- Cost-Effective Scalability. The ability to scale storage and compute independently, often in a cloud environment, makes Lakehouses cost-effective for growing data needs.
- Improved Data Governance. Centralising data management enhances governance, security, and quality across all types of data.
Challenges
- Complexity in Implementation. Designing and implementing a Lakehouse architecture can be complex, requiring expertise in both Data Lakes and Data Warehouses.
- Data Consistency and Quality. Though crucial for reliable analytics, ensuring data consistency and quality across diverse data types and sources can be challenging.
- Governance and Security. Comprehensive data governance and security strategies are required to protect sensitive information and comply with regulations.
The choice between Data Warehouse, Data Lake, or Lakehouse systems is pivotal for businesses in harnessing the power of their data. Each option offers distinct advantages and challenges, requiring careful consideration of organisational needs and goals. By embracing the right data management system, organisations can pave the way for informed decision-making, operational efficiency, and innovation in the digital age.
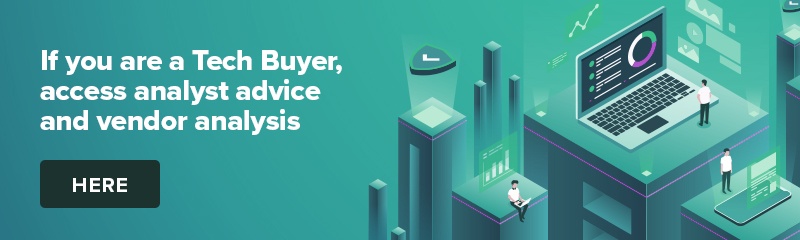
AI systems are creating huge amounts of data at a rapid rate. While this flood of information is extremely valuable, it is also difficult to analyse and understand. Organisations need to make sense of these large data sets to derive useful insights and make better decisions. Data visualisation plays a pivotal role in the interpretation of complex data, making it accessible, understandable, and actionable. Well-designed visualisation can translate complex, high-dimensional data into intuitive, visually appealing representations, helping stakeholders to understand patterns, trends, and anomalies that would otherwise be challenging to recognise.
There are some data visualisation methods that you are using already; and some that you definitely should master as data complexity increases and there is more demand from business teams for better data visualisation.
Download Common Data Visualisation Methods as a PDF
Add These to Your Data Visualisation Repertoire
There are additional visualisation tools that you should be using to tell a better data story. Each of these visualisation techniques serves specific purposes in data analysis, offering unique advantages for representing data insights.
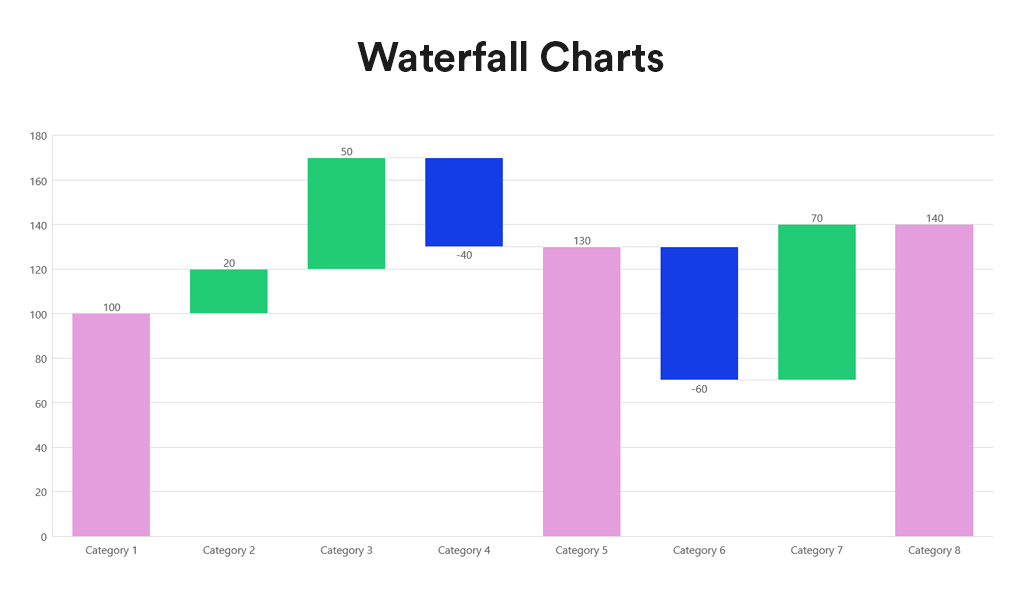
Waterfall charts depict the impact of intermediate positive and negative values on an initial value, often resulting in a final value. They are commonly employed in financial analysis to illustrate the contribution of various factors to a total, making them ideal for visualising step-by-step financial contributions or tracking the cumulative effect of sequentially introduced factors.
Advantages:
- Sequential Analysis. Ideal for understanding the cumulative effect of sequentially introduced positive or negative values.
- Financial Reporting. Commonly used for financial statements to break down the contributions of various elements to a net result, such as revenues, costs, and profits over time.
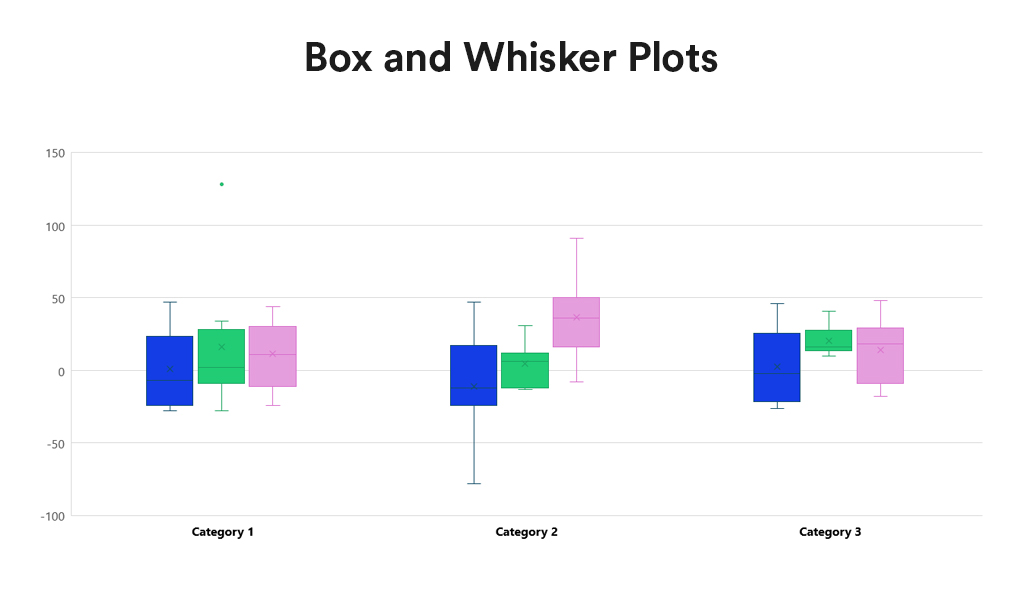
Box and Whisker Plots summarise data distribution using a five-number summary: minimum, first quartile (Q1), median, third quartile (Q3), and maximum. They are valuable for showcasing data sample variations without relying on specific statistical assumptions. Box and Whisker Plots excel in comparing distributions across multiple groups or datasets, providing a concise overview of various statistics.
Advantages:
- Distribution Clarity. Provide a clear view of the data distribution, including its central tendency, variability, and skewness.
- Outlier Identification. Easily identify outliers, offering insights into the spread and symmetry of the data.
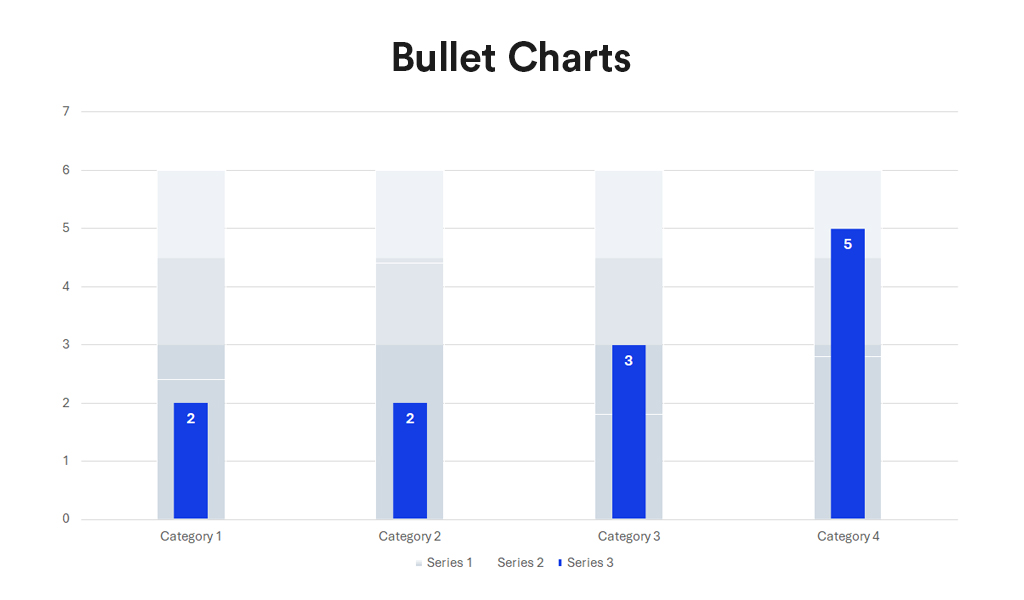
Bullet charts, a bar graph variant, serve as a replacement for dashboard gauges and meters. They showcase a primary measure alongside one or more other measures for context, such as a target or previous period’s performance, often incorporating qualitative ranges like poor, satisfactory, and good. Ideal for performance dashboards with limited space, bullet charts efficiently demonstrate progress towards goals.
Advantages:
- Compactness. Offer a compact and straightforward way to monitor performance against a target.
- Efficiency. More efficient than gauges and meters in dashboard design, as they take up less space and can display more information, making them ideal for comparing multiple measures.
Conclusion
Each data visualisation type has its unique strengths, making it better suited for certain types of data and analysis than others. The key to effective data visualisation lies in matching the visualisation type to your data’s specific needs, considering the story you want, to tell or the insights you aim to glean. Choosing the right data representation helps you to make informed decisions that enhance your data analysis and communication efforts.
Incorporating Waterfall Charts, Box and Whisker Plots, and Bullet Charts into the data visualisation toolkit allows for a broader range of insights to be derived from your data. From analysing financial data, comparing distributions, to tracking performance metrics, these additional types of visualisation can communicate complex data stories clearly and effectively. As with all data visualisation, the key is to choose the type that best matches the organisation’s data story, making it accessible and understandable to the audience.
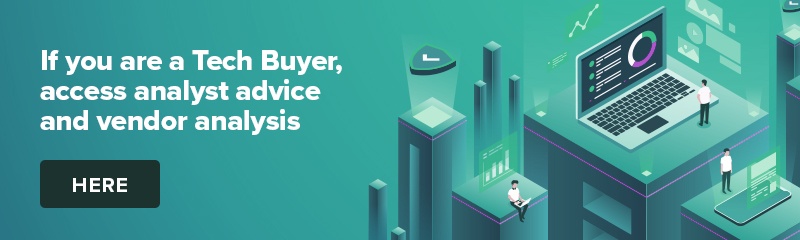
AI has become a business necessity today, catalysing innovation, efficiency, and growth by transforming extensive data into actionable insights, automating tasks, improving decision-making, boosting productivity, and enabling the creation of new products and services.
Generative AI stole the limelight in 2023 given its remarkable advancements and potential to automate various cognitive processes. However, now the real opportunity lies in leveraging this increased focus and attention to shine the AI lens on all business processes and capabilities. As organisations grasp the potential for productivity enhancements, accelerated operations, improved customer outcomes, and enhanced business performance, investment in AI capabilities is expected to surge.
In this eBook, Ecosystm VP Research Tim Sheedy and Vinod Bijlani and Aman Deep from HPE APAC share their insights on why it is crucial to establish tailored AI capabilities within the organisation.
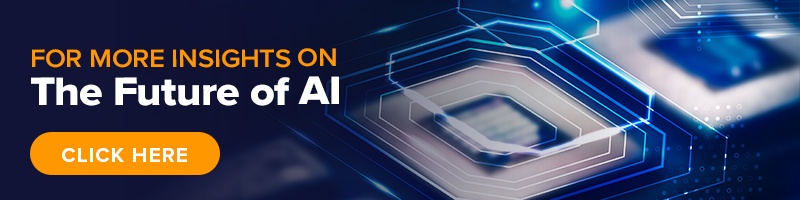