2024 was a year marked by intense AI-driven innovation. While the hype surrounding AI may have reached a fever pitch, the technology’s transformative potential is undeniable.
The growing interest in AI can be attributed to several factors: the democratisation of AI, with tools and platforms now accessible to businesses of all sizes; AI’s appeal to business leaders, offering actionable insights and process automation; and aggressive marketing by major tech companies, which has amplified the excitement and hype surrounding AI.
2025 will be a year defined by AI, with its transformative impact rippling across industries. However, other geopolitical and social factors will also significantly shape the tech landscape.
Ecosystm analysts Achim Granzen, Alan Hesketh, Audrey William, Clay Miller, Darian Bird, Manish Goenka, Richard Wilkins, Sash Mukherjee, Simona Dimovski, and Tim Sheedy present the key trends and disruptors shaping the tech market in 2025.
Click here to download ‘Key Tech Trends & Disruptors in 2025’ as a PDF
1. Quantum Computing Will Drive Major Transformation in the Tech Industry
Advancements in qubit technology, quantum error correction, and hybrid quantum-classical systems will accelerate breakthroughs in complex problem-solving and machine learning. Quantum communications will revolutionise data security with quantum key distribution, providing nearly unbreakable communication channels. As quantum encryption becomes more widespread, it will replace current cryptographic methods, protecting sensitive data from future quantum-enabled attacks.
With quantum computing threatening encryption standards like RSA and ECC, post-quantum encryption will be critical for data security.
While the full impact of quantum computers is expected within the next few years, 2025 will be pivotal in the transition toward quantum-resistant security measures and infrastructure.
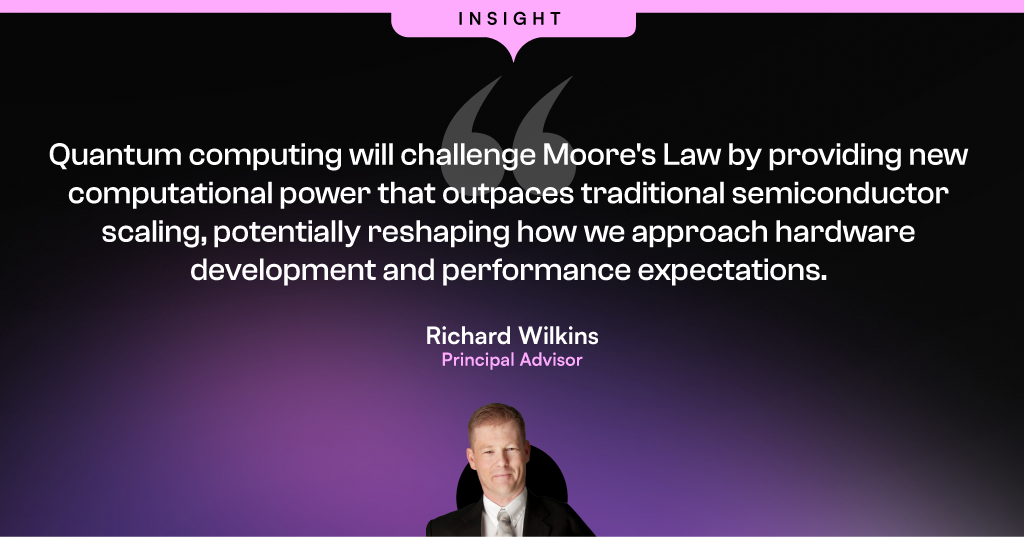
2. Many Will Try, But Few Will Succeed as Platform Companies
Hypergrowth occurs when companies shift from selling products to becoming platform providers. Unlike traditional businesses, platforms don’t own inventory; their value lies in proprietary data and software that connect buyers, sellers, and consumers. Platforms disrupt industries and often outperform legacy businesses, with examples like Uber, Amazon, and Meta, and disruptors like Lemonade in insurance and Wise in international funds transfer.
In 2025, many companies will aim to become platform businesses, with AI seen as a key driver.
They will begin creating platforms and building ecosystems around them – some within existing brands, others launching new ones or even new subsidiaries to seize this opportunity.
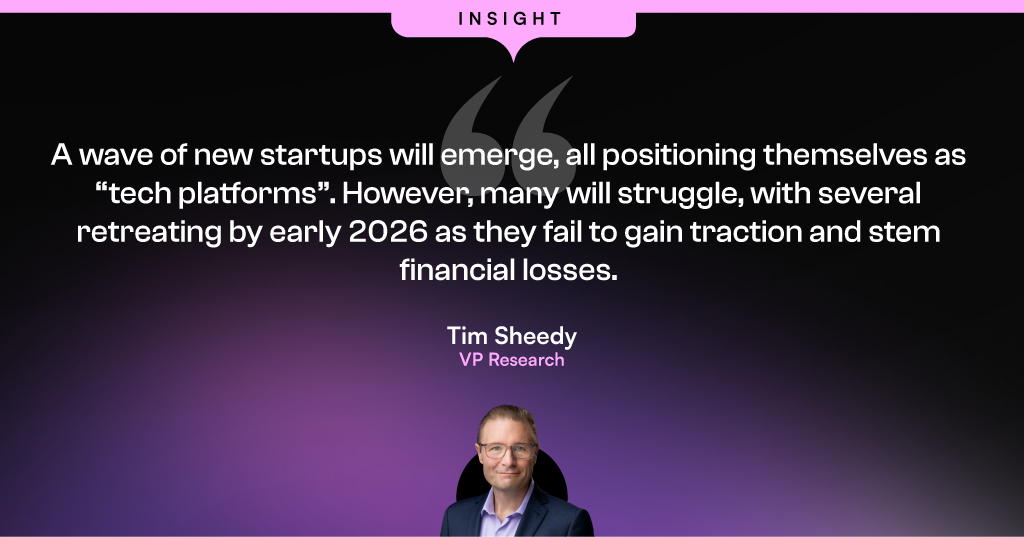
3. A Trans-Atlantic Divide Will Emerge in AI Regulation
The EU is poised to continue its rigorous approach to AI regulation, emphasisng ethical considerations and robust governance. This is evident in the recent AI Act, which imposes stringent guidelines and penalties for violations. The EU’s commitment to responsible AI development is likely to lead to a more cautious and controlled innovation landscape.
In contrast, the US, under a new administration, may adopt a more lenient regulatory stance towards AI. This shift could accelerate innovation and foster a more permissive environment for AI development. However, it may also raise concerns about potential risks and unintended consequences.
This divergence in regulatory frameworks could create significant challenges for multinational companies operating in both regions.
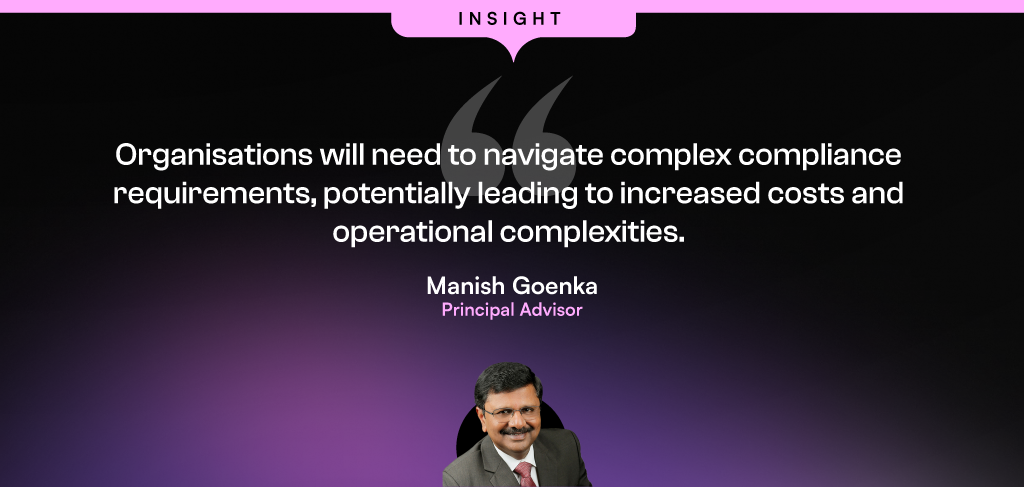
4. The Rise of AI-Driven Ecosystem Platforms Will Shape Tech Investments
By 2025, AI-driven ecosystem platforms will dominate tech investments, fueled by technological convergence, market efficiency demands, and evolving regulations. These platforms will integrate AI, IoT, cloud, and data analytics to create seamless, predictive ecosystems that transcend traditional industry boundaries.
Key drivers include advancements in AI, global supply chain disruptions, and rising ESG expectations. Regulatory shifts, such as the EU’s AI Act, will further push for compliant, ethical platforms emphasising transparency and accountability.
For businesses, this shift redefines technology as interconnected ecosystems driving efficiency, innovation, and customer value.
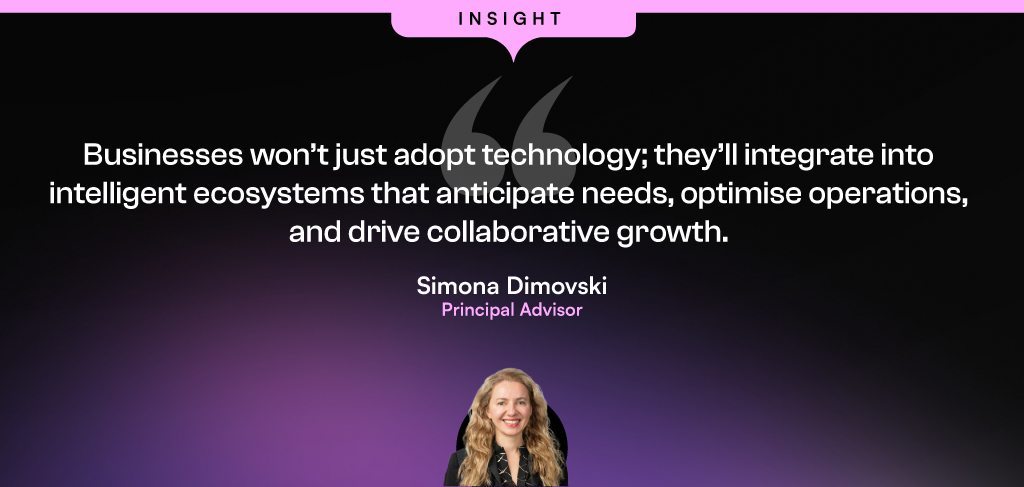
5. AI-Powered Data Fabrics Will be the Foundation for Data-Driven Success
In 2025, AI-powered data fabrics will become a core technology for large organisations.
They will transition from basic data management tools to intelligent systems that deliver value across the entire data lifecycle. Organisations will finally be able to get control of their data governance.
AI’s enhanced role will automate essential data functions, including intelligent data integration and autonomous connection to diverse data sources. AI will also enable proactive data quality management, predicting and preventing errors for improved reliability. AI-driven data fabrics will also offer automated data discovery and mapping, dynamic data quality and governance, intelligent data integration, and enhanced data access and delivery.
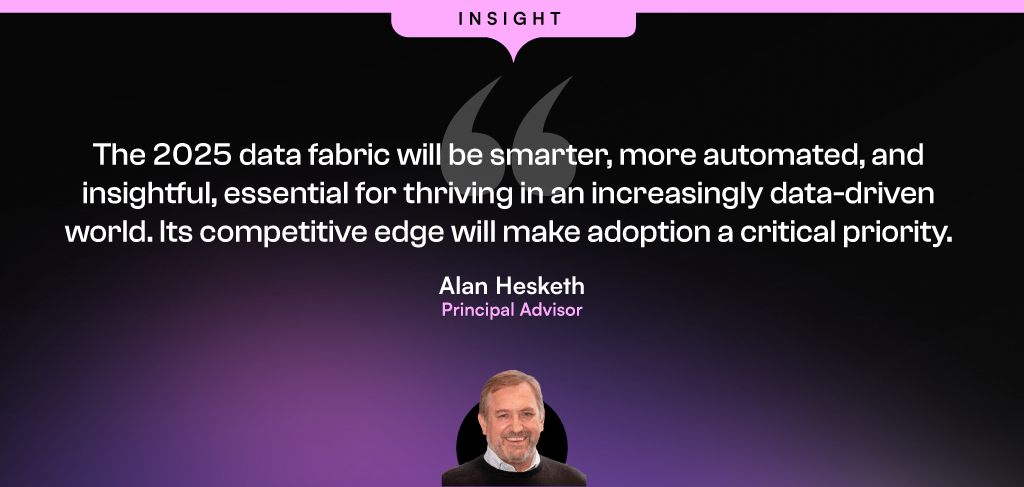
6. Focus Will Shift From AI Models to Intelligence Gaps & Performance
While many organisations are investing in AI, only those that started their transformation in 2024 are truly AI-led. Most have become AI-driven through embedded AI in enterprise systems as tech providers continue to evolve their offerings. However, these multi-vendor environments often lack synergy, creating gaps and blind spots.
In 2025, organisations will pause their investments to assess AI capabilities and identify these gaps.
Once they pinpoint the blind spots, investments will refocus not on new AI models, but on areas like model orchestration to manage workflows and ensure peak performance; vendor management to establish unified governance frameworks for flexibility and compliance; and eventually automated AI lifecycle management, with centralised inventories and monitoring to track performance and detect issues like model drift.
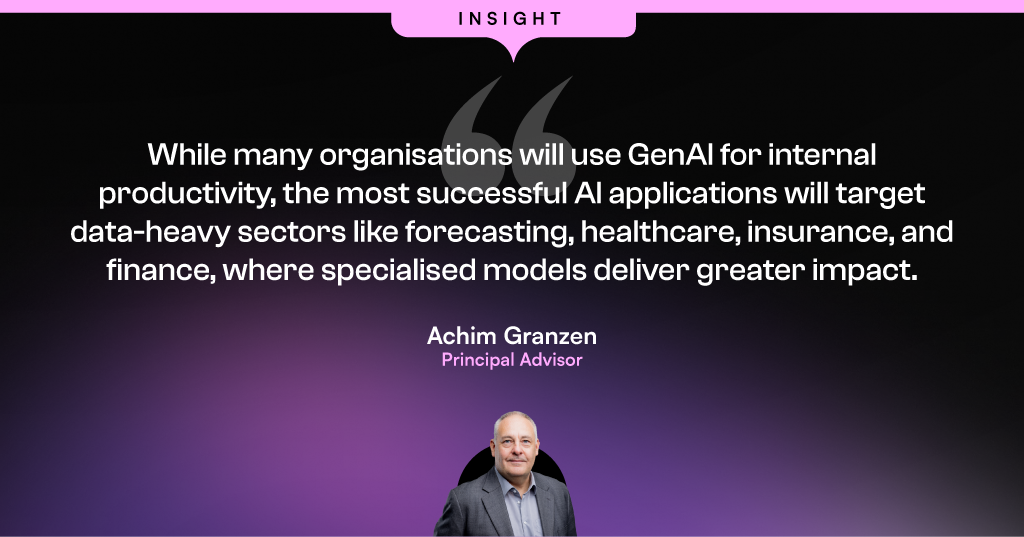
7. Specialised Small Language Models Will Gain Traction
GenAI, driven by LLMs, has dominated the spotlight, fueling both excitement and concerns about AI. However, LLM-based GenAI is entering a phase of diminishing returns, both in terms of individual model capabilities and the number of available models. Only a few providers will have the resources to develop LLMs, focusing on a limited number of models.
This will see the increased popularity of small language models (SLMs), that are tailored for a specific purpose, use case, or environment. These models will be developed by startups, organisations, and enterprises with deep domain knowledge and data. They will be fully commercialised driving narrow but distinct ROI.
There will be an increased demand for GPU-as-a-service and SLM-as-a-service, and the platforms which can support these.
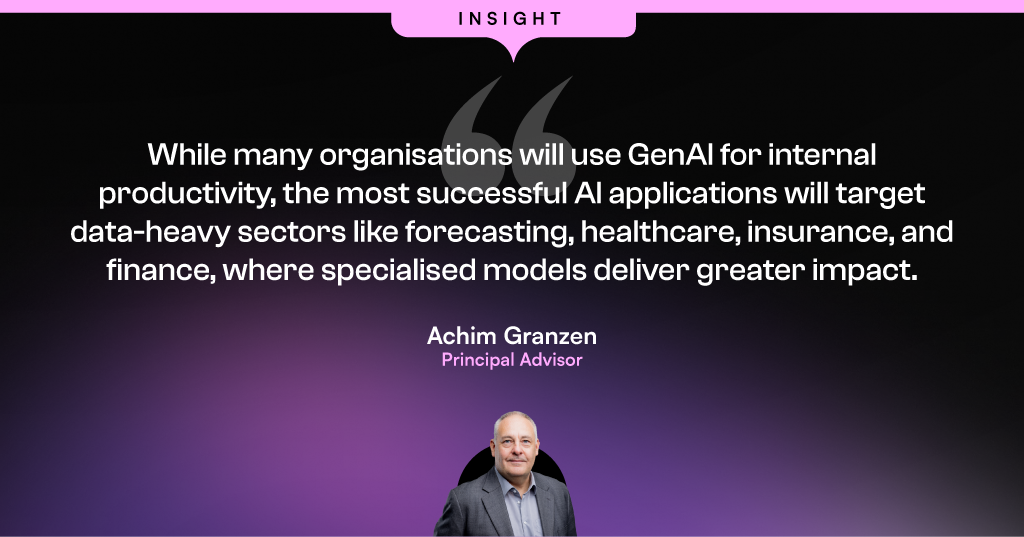
8. Multi-agent AI Systems Will Help Manage Complexity and Collaboration
Isolated AI tools that can perform narrow tasks lack the adaptability and coordination required for real-time decision-making. Multi-agent systems, in contrast, consist of decentralised agents that collaborate, share information, and make independent decisions while working toward a common goal. This approach not only improves efficiency but also enhances resilience in rapidly changing conditions.
Early use cases will be in complex environments that require cooperation between multiple stakeholders.
Multi-agent systems will optimise logistics by continuously analysing disruptions and dynamically balancing supply and demand in energy grids. These multi-agent systems will also operate in competitive modes, such as algorithmic trading, ad auctions, and ecommerce recommender systems.
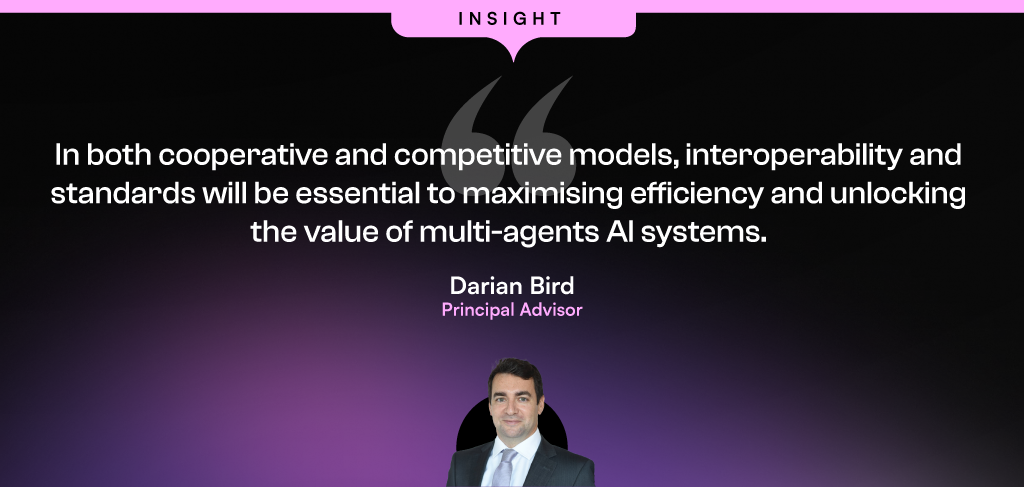
9. Super Apps Will Expand into Rural & Underserved Markets in Asia Pacific
Super apps are set to reshape rural economies, fueled by increased internet access, affordable tech, and heavy government investment in digital infrastructure. Their localised, all-in-one services unlock untapped potential in underserved regions, fostering inclusivity and innovation.
By 2025, super apps will deepen their reach across Asia, integrating communication, payments, and logistics into seamless platforms.
Leveraging affordable mobile devices, cloud-native technologies, and localised services, they will penetrate rural and underserved areas with tailored solutions like agricultural marketplaces, local logistics, and expanded government services. Enterprises investing in agile cloud infrastructure will drive this evolution, bridging the digital divide, boosting economic growth, and enhancing user experiences for millions.
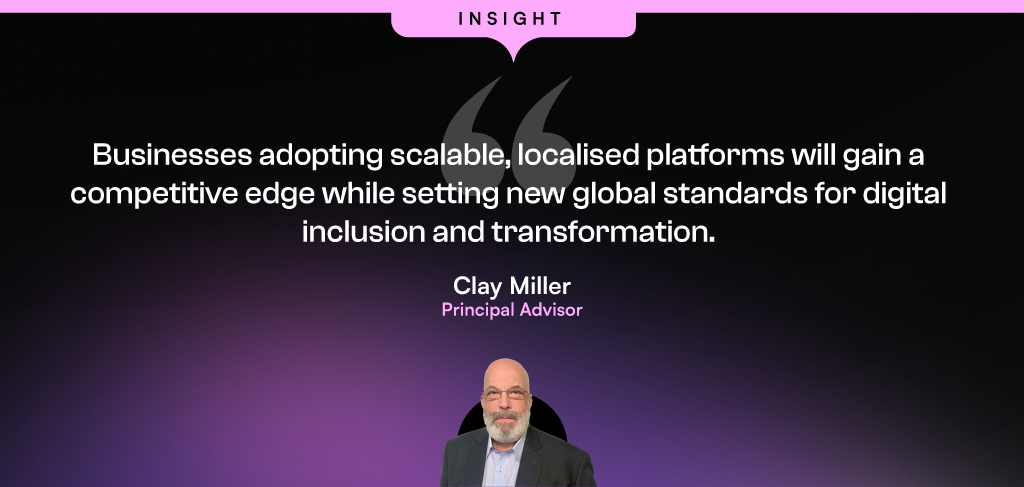
10. Intense Debates Over Remote vs. In-Office Work Will Persist in Asia Pacific
Employers in Asia Pacific will enforce stricter return-to-office policies, linking them to performance metrics and benefits to justify investments in physical spaces and enhance workforce productivity.
However, remote collaboration will remain integral, even for in-office teams.
The push for human-centred tech will grow, focusing on employee well-being and flexibility through AI-powered tools and hybrid platforms. Companies will prioritise enhancing employee experiences with personalised, adaptable workspaces, while office designs will increasingly incorporate biophilic elements, blending nature and technology to support seamless collaboration and remote integration.
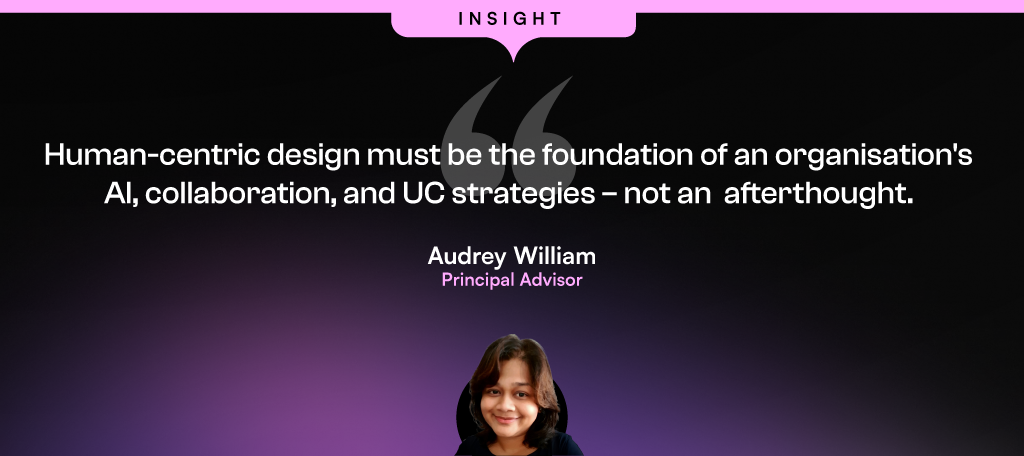
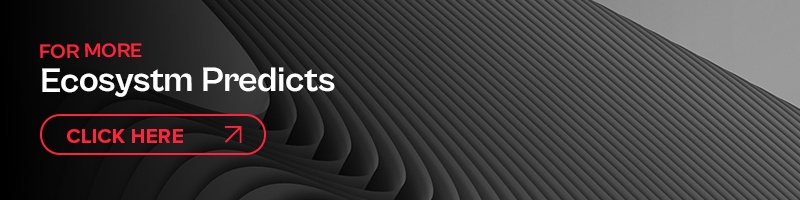
At a recently held Ecosystm roundtable, in partnership with Qlik and 121Connects, Ecosystm Principal Advisor Manoj Chugh, moderated a conversation where Indian tech and data leaders discussed building trust in data strategies. They explored ways to automate data pipelines and improve governance to drive better decisions and business outcomes. Here are the key takeaways from the session.

Data isn’t just a byproduct anymore; it’s the lifeblood of modern businesses, fuelling informed decisions and strategic growth. But with vast amounts of data, the challenge isn’t just managing it; it’s building trust. AI, once a beacon of hope, is now at risk without a reliable data foundation. Ecosystm research reveals that a staggering 66% of Indian tech leaders doubt their organisation’s data quality, and the problem of data silos is exacerbating this trust crisis.
At the Leaders Roundtable in Mumbai, I had the opportunity to moderate a discussion among data and digital leaders on the critical components of building trust in data and leveraging it to drive business value. The consensus was that building trust requires a comprehensive strategy that addresses the complexities of data management and positions the organisation for future success. Here are the key strategies that are essential for achieving these goals.
1. Adopting a Unified Data Approach
Organisations are facing a growing wave of complex workloads and business initiatives. To manage this expansion, IT teams are turning to multi-cloud, SaaS, and hybrid environments. However, this diverse landscape introduces new challenges, such as data silos, security vulnerabilities, and difficulties in ensuring interoperability between systems.

A unified data strategy is crucial to overcome these challenges. By ensuring platform consistency, robust security, and seamless data integration, organisations can simplify data management, enhance security, and align with business goals – driving informed decisions, innovation, and long-term success.
Real-time data integration is essential for timely data availability, enabling organisations to make data-driven decisions quickly and effectively. By integrating data from various sources in real-time, businesses can gain valuable insights into their operations, identify trends, and respond to changing market conditions.
Organisations that are able to integrate their IT and operational technology (OT) systems find their data accuracy increasing. By combining IT’s digital data management expertise with OT’s real-time operational insights, organisations can ensure more accurate, timely, and actionable data. This integration enables continuous monitoring and analysis of operational data, leading to faster identification of errors, more precise decision-making, and optimised processes.
2. Enhancing Data Quality with Automation and Collaboration
As the volume and complexity of data continue to grow, ensuring high data quality is essential for organisations to make accurate decisions and to drive trust in data-driven solutions. Automated data quality tools are useful for cleansing and standardising data to eliminate errors and inconsistencies.
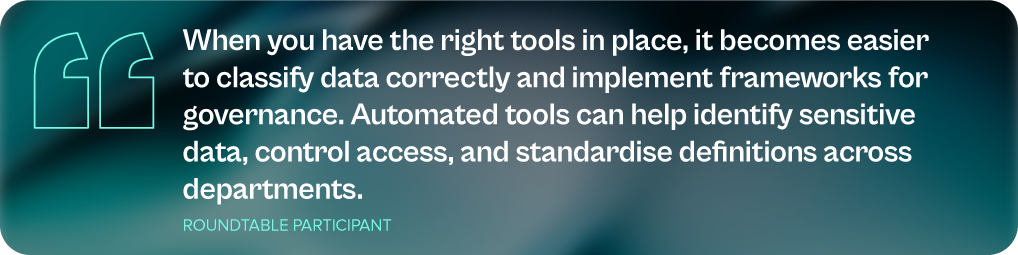
As mentioned earlier, integrating IT and OT systems can help organisations improve operational efficiency and resilience. By leveraging data-driven insights, businesses can identify bottlenecks, optimise workflows, and proactively address potential issues before they escalate. This can lead to cost savings, increased productivity, and improved customer satisfaction.
However, while automation technologies can help, organisations must also invest in training employees in data management, data visualisation, and data governance.
3. Modernising Data Infrastructure for Agility and Innovation
In today’s fast-paced business landscape, agility is paramount. Modernising data infrastructure is essential to remain competitive – the right digital infrastructure focuses on optimising costs, boosting capacity and agility, and maximising data leverage, all while safeguarding the organisation from cyber threats. This involves migrating data lakes and warehouses to cloud platforms and adopting advanced analytics tools. However, modernisation efforts must be aligned with specific business goals, such as enhancing customer experiences, optimising operations, or driving innovation. A well-modernised data environment not only improves agility but also lays the foundation for future innovations.

Technology leaders must assess whether their data architecture supports the organisation’s evolving data requirements, considering factors such as data flows, necessary management systems, processing operations, and AI applications. The ideal data architecture should be tailored to the organisation’s specific needs, considering current and future data demands, available skills, costs, and scalability.
4. Strengthening Data Governance with a Structured Approach
Data governance is crucial for establishing trust in data, and providing a framework to manage its quality, integrity, and security throughout its lifecycle. By setting clear policies and processes, organisations can build confidence in their data, support informed decision-making, and foster stakeholder trust.
A key component of data governance is data lineage – the ability to trace the history and transformation of data from its source to its final use. Understanding this journey helps organisations verify data accuracy and integrity, ensure compliance with regulatory requirements and internal policies, improve data quality by proactively addressing issues, and enhance decision-making through context and transparency.
A tiered data governance structure, with strategic oversight at the executive level and operational tasks managed by dedicated data governance councils, ensures that data governance aligns with broader organisational goals and is implemented effectively.
Are You Ready for the Future of AI?
The ultimate goal of your data management and discovery mechanisms is to ensure that you are advancing at pace with the industry. The analytics landscape is undergoing a profound transformation, promising to revolutionise how organisations interact with data. A key innovation, the data fabric, is enabling organisations to analyse unstructured data, where the true value often lies, resulting in cleaner and more reliable data models.
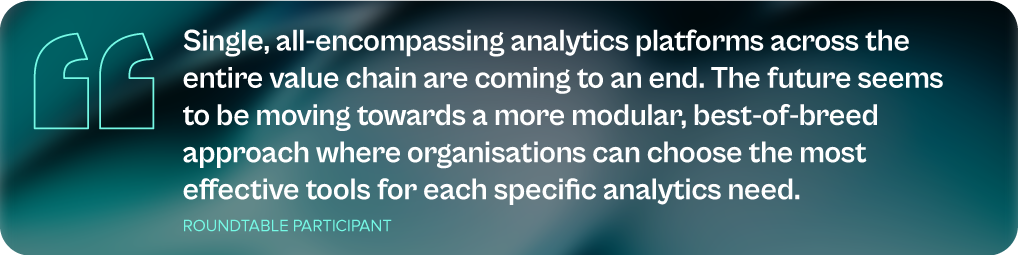
GenAI has emerged as another game-changer, empowering employees across the organisation to become citizen data scientists. This democratisation of data analytics allows for a broader range of insights and fosters a more data-driven culture. Organisations can leverage GenAI to automate tasks, generate new ideas, and uncover hidden patterns in their data.
The shift from traditional dashboards to real-time conversational tools is also reshaping how data insights are delivered and acted upon. These tools enable users to ask questions in natural language, receiving immediate and relevant answers based on the underlying data. This conversational approach makes data more accessible and actionable, empowering employees to make data-driven decisions at all levels of the organisation.
To fully capitalise on these advancements, organisations need to reassess their AI/ML strategies. By ensuring that their tech initiatives align with their broader business objectives and deliver tangible returns on investment, organisations can unlock the full potential of data-driven insights and gain a competitive edge. It is equally important to build trust in AI initiatives, through a strong data foundation. This involves ensuring data quality, accuracy, and consistency, as well as implementing robust data governance practices. A solid data foundation provides the necessary groundwork for AI and GenAI models to deliver reliable and valuable insights.
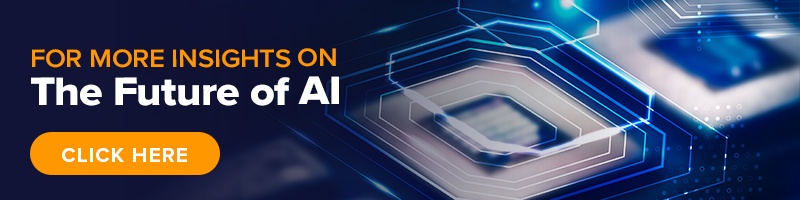
In my previous blogs, I outlined strategies for public sector organisations to incorporate technology into citizen services and internal processes. Building on those perspectives, let’s talk about the critical role of data in powering digital transformation across the public sector.
Effectively leveraging data is integral to delivering enhanced digital services and streamlining operations. Organisations must adopt a forward-looking roadmap that accounts for different data maturity levels – from core data foundations and emerging catalysts to future-state capabilities.
1. Data Essentials: Establishing the Bedrock
Data model. At the core of developing government e-services portals, strategic data modelling establishes the initial groundwork for scalable data infrastructures that can support future analytics, AI, and reporting needs. Effective data models define how information will be structured and analysed as data volumes grow. Beginning with an Entity-Relationship model, these blueprints guide the implementation of database schemas within database management systems (DBMS). This foundational approach ensures that the data infrastructure can accommodate the vast amounts of data generated by public services, crucial for maintaining public trust in government systems.
Cloud Databases. Cloud databases provide flexible, scalable, and cost-effective storage solutions, allowing public sector organisations to handle vast amounts of data generated by public services. Data warehouses, on the other hand, are centralised repositories designed to store structured data, enabling advanced querying and reporting capabilities. This combination allows for robust data analytics and AI-driven insights, ensuring that the data infrastructure can support future growth and evolving analytical needs.
Document management. Incorporating a document or records management system (DMS/RMS) early in the data portfolio of a government e-services portal is crucial for efficient operations. This system organises extensive paperwork and records like applications, permits, and legal documents systematically. It ensures easy storage, retrieval, and management, preventing issues with misplaced documents.
Emerging Catalysts: Unleashing Data’s Potential
Digital Twins. A digital twin is a sophisticated virtual model of a physical object or system. It surpasses traditional reporting methods through advanced analytics, including predictive insights and data mining. By creating detailed virtual replicas of infrastructure, utilities, and public services, digital twins allow for real-time monitoring, efficient resource management, and proactive maintenance. This holistic approach contributes to more efficient, sustainable, and livable cities, aligning with broader goals of urban development and environmental sustainability.
Data Fabric. Data Fabric, including Data Lakes and Data Lakehouses, represents a significant leap in managing complex data environments. It ensures data is accessible for various analyses and processing needs across platforms. Data Lakes store raw data in its original format, crucial for initial data collection when future data uses are uncertain. In Cloud DB or Data Fabric setups, Data Lakes play a foundational role by storing unprocessed or semi-structured data. Data Lakehouses combine Data Lakes’ storage with data warehouses’ querying capabilities, offering flexibility, and efficiency for handling different types of data in sophisticated environments.
Data Exchange and MOUs. Even with advanced data management technologies like data fabrics, Data Lakes, and Data Lakehouses, achieving higher maturity in digital government ecosystems often depends on establishing data-sharing agreements. Memorandums of Understanding (MoUs) exemplify these agreements, crucial for maximising efficiency and collaboration. MoUs outline terms, conditions, and protocols for sharing data beyond regulatory requirements, defining its scope, permitted uses, governance standards, and responsibilities of each party. This alignment ensures data integrity, privacy, and security while facilitating collaboration that enhances innovation and service delivery. Such agreements also pave the way for potential commercialisation of shared data resources, opening new market opportunities.
Future-Forward Capabilities: Pioneering New Frontiers
Data Mesh. Data Mesh is a decentralised approach to data architecture and organisational design, ideal for complex stakeholder ecosystems like digital conveyancing solutions. Unlike centralised models, Data Mesh allows each domain to manage its data independently. This fosters collaboration while ensuring secure and governed data sharing, essential for efficient conveyancing processes. Data Mesh enhances data quality and relevance by holding stakeholders directly accountable for their data, promoting integrity and adaptability to market changes. Its focus on interoperability and self-service data access enhances user satisfaction and operational efficiency, catering flexibly to diverse user needs within the conveyancing ecosystem.
Data Embassies. A Data Embassy stores and processes data in a foreign country under the legal jurisdiction of its origin country, beneficial for digital conveyancing solutions serving international markets. This approach ensures data security and sovereignty, governed by the originating nation’s laws to uphold privacy and legal integrity in conveyancing transactions. Data Embassies enhance resilience against physical and cyber threats by distributing data across international locations, ensuring continuous operation despite disruptions. They also foster international collaboration and trust, potentially attracting more investment and participation in global real estate markets. Technologically, Data Embassies rely on advanced data centres, encryption, cybersecurity, cloud, and robust disaster recovery solutions to maintain uninterrupted conveyancing services and compliance with global standards.
Conclusion
By developing a cohesive roadmap that progressively integrates cutting-edge architectures, cross-stakeholder partnerships, and avant-garde juridical models, agencies can construct a solid data ecosystem. One where information doesn’t just endure disruption, but actively facilitates organisational resilience and accelerates mission impact. Investing in an evolutionary data strategy today lays the crucial groundwork for delivering intelligent, insight-driven public services for decades to come. The time to fortify data’s transformative potential is now.
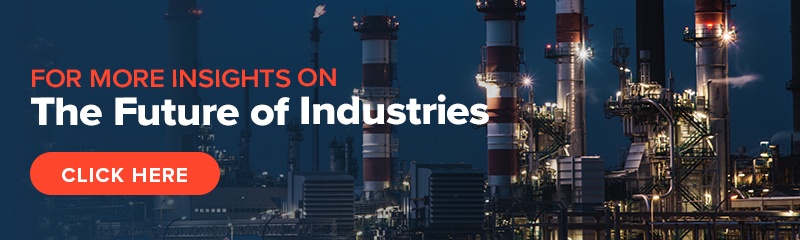
In my last Ecosystm Insight, I spoke about the importance of data architecture in defining the data flow, data management systems required, the data processing operations, and AI applications. Data Mesh and Data Fabric are both modern architectural approaches designed to address the complexities of managing and accessing data across a large organisation. While they share some commonalities, such as improving data accessibility and governance, they differ significantly in their methodologies and focal points.
Data Mesh
- Philosophy and Focus. Data Mesh is primarily focused on the organisational and architectural approach to decentralise data ownership and governance. It treats data as a product, emphasising the importance of domain-oriented decentralised data ownership and architecture. The core principles of Data Mesh include domain-oriented decentralised data ownership, data as a product, self-serve data infrastructure as a platform, and federated computational governance.
- Implementation. In a Data Mesh, data is managed and owned by domain-specific teams who are responsible for their data products from end to end. This includes ensuring data quality, accessibility, and security. The aim is to enable these teams to provide and consume data as products, improving agility and innovation.
- Use Cases. Data Mesh is particularly effective in large, complex organisations with many independent teams and departments. It’s beneficial when there’s a need for agility and rapid innovation within specific domains or when the centralisation of data management has become a bottleneck.
Data Fabric
- Philosophy and Focus. Data Fabric focuses on creating a unified, integrated layer of data and connectivity across an organisation. It leverages metadata, advanced analytics, and AI to improve data discovery, governance, and integration. Data Fabric aims to provide a comprehensive and coherent data environment that supports a wide range of data management tasks across various platforms and locations.
- Implementation. Data Fabric typically uses advanced tools to automate data discovery, governance, and integration tasks. It creates a seamless environment where data can be easily accessed and shared, regardless of where it resides or what format it is in. This approach relies heavily on metadata to enable intelligent and automated data management practices.
- Use Cases. Data Fabric is ideal for organisations that need to manage large volumes of data across multiple systems and platforms. It is particularly useful for enhancing data accessibility, reducing integration complexity, and supporting data governance at scale. Data Fabric can benefit environments where there’s a need for real-time data access and analysis across diverse data sources.
Both approaches aim to overcome the challenges of data silos and improve data accessibility, but they do so through different methodologies and with different priorities.
Data Mesh and Data Fabric Vendors
The concepts of Data Mesh and Data Fabric are supported by various vendors, each offering tools and platforms designed to facilitate the implementation of these architectures. Here’s an overview of some key players in both spaces:
Data Mesh Vendors
Data Mesh is more of a conceptual approach than a product-specific solution, focusing on organisational structure and data decentralisation. However, several vendors offer tools and platforms that support the principles of Data Mesh, such as domain-driven design, product thinking for data, and self-serve data infrastructure:
- Thoughtworks. As the originator of the Data Mesh concept, Thoughtworks provides consultancy and implementation services to help organisations adopt Data Mesh principles.
- Starburst. Starburst offers a distributed SQL query engine (Starburst Galaxy) that allows querying data across various sources, aligning with the Data Mesh principle of domain-oriented, decentralised data ownership.
- Databricks. Databricks provides a unified analytics platform that supports collaborative data science and analytics, which can be leveraged to build domain-oriented data products in a Data Mesh architecture.
- Snowflake. With its Data Cloud, Snowflake facilitates data sharing and collaboration across organisational boundaries, supporting the Data Mesh approach to data product thinking.
- Collibra. Collibra provides a data intelligence cloud that offers data governance, cataloguing, and privacy management tools essential for the Data Mesh approach. By enabling better data discovery, quality, and policy management, Collibra supports the governance aspect of Data Mesh.
Data Fabric Vendors
Data Fabric solutions often come as more integrated products or platforms, focusing on data integration, management, and governance across a diverse set of systems and environments:
- Informatica. The Informatica Intelligent Data Management Cloud includes features for data integration, quality, governance, and metadata management that are core to a Data Fabric strategy.
- Talend. Talend provides data integration and integrity solutions with strong capabilities in real-time data collection and governance, supporting the automated and comprehensive approach of Data Fabric.
- IBM. IBM’s watsonx.data is a fully integrated data and AI platform that automates the lifecycle of data across multiple clouds and systems, embodying the Data Fabric approach to making data easily accessible and governed.
- TIBCO. TIBCO offers a range of products, including TIBCO Data Virtualization and TIBCO EBX, that support the creation of a Data Fabric by enabling comprehensive data management, integration, and governance.
- NetApp. NetApp has a suite of cloud data services that provide a simple and consistent way to integrate and deliver data across cloud and on-premises environments. NetApp’s Data Fabric is designed to enhance data control, protection, and freedom.
The choice of vendor or tool for either Data Mesh or Data Fabric should be guided by the specific needs, existing technology stack, and strategic goals of the organisation. Many vendors provide a range of capabilities that can support different aspects of both architectures, and the best solution often involves a combination of tools and platforms. Additionally, the technology landscape is rapidly evolving, so it’s wise to stay updated on the latest offerings and how they align with the organisation’s data strategy.
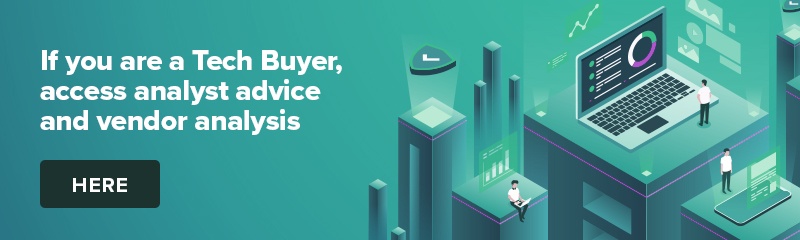
2023 has started amidst concerns. Economists are talking about slowdowns, recessions, and downsizing. In the past, every time the economy has been uncertain, we have seen a downtrend in tech spend by companies.
2023 will be different!
Today, all organisations know of the power of digital transformation and will continue to invest in technology to counter the market uncertainties. They will respond to the emerging forces of innovation, deploy automation to counter skills gaps, and focus on being nimble and agile businesses – all with the help of technology.
Here are the 5 trends that will impact tech spending in 2023.
- Organisations will aim for “Big Ticket Innovations”. This will see innovation becoming integral to strategic discussions on culture, people, process, and technology; and the resurgence of emerging technologies.
- AI will replace Cloud as organisations’ transformation goal. Organisations will evolve their AI roadmaps and strategies – focusing on both short-term wins and long-term success factors. They will also make an effort to identify digital debt and weed out applications and services that are sub-optimal for AI
- Building the right data platform architecture will gain importance. Data platforms will become free from the constraints of operational technologies. This will see a reduction of dependence on centralised data repositories; and an uptick in adoption of data fabric architecture to manage distributed data
- Organisations will invest in proactive cyber protection amidst escalating threats. Organisations will focus on immutable backups, data masking techniques and on building a single pane of glass view of all cyber tools and applications
- Sustainability will drive tech investments. This will see an evaluation of all infrastructure (whether cloud or on-premises) with a focus on cost and sustainability and a growing need to integrate all organisational data – across digital, IT, and OT systems
To find out more, read below.
Download 5 Trends Impacting Tech Investments in 2023 as a PDF.
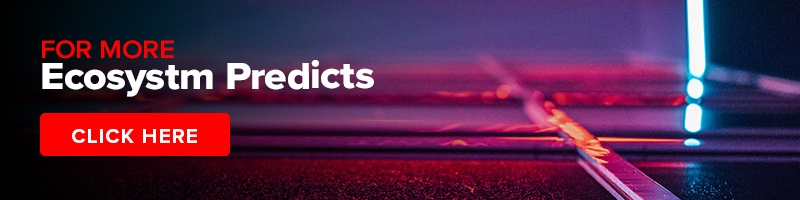
Data & AI initiatives are firmly at the core of any organisation’s tech-led transformation efforts. Businesses today realise the value of real-time data insights to deliver the agility that is required to succeed in today’s competitive, and often volatile, market.
But organisations continue to struggle with their data & AI initiatives for a variety of reasons. Organisations in ASEAN report some common challenges in implementing successful data & AI initiatives.
Here are 5 insights to build scalable AI.
- Data Access a Key Stumbling Block. Many organisations find that they no longer need to rely on centralised data repositories.
- Organisations Need Data Creativity. A true data-first organisation derives value from their data & AI investments across the entire organisation, cross-leveraging data.
- Governance Not Built into Organisational Psyche. A data-first organisation needs all employees to have a data-driven mindset. This can only be driven by clear guidelines that are laid out early on and adhered to by data generators, managers, and consumers.
- Lack of End-to-End Data Lifecycle Management. It is critical to have observability, intelligence, and automation built into the entire data lifecycle.
- Democratisation of Data & AI Should Be the Goal. The true value of data & AI solutions will be fully realised when the people who benefit from the solutions are the ones managing the solutions and running the queries that will help them deliver better value to the business.
Read below to find out more.
Download 5 Insights to Help Organisations Build Scalable AI – An ASEAN View as a PDF
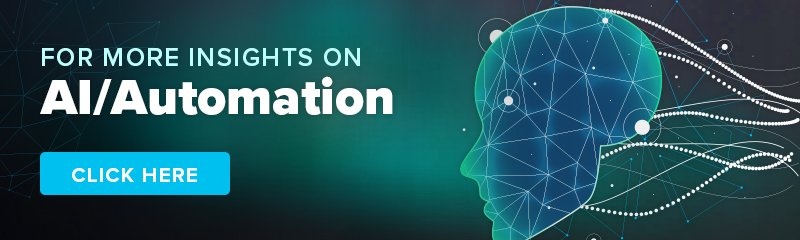
In the rush towards digital transformation, individual lines of business in organisations, have built up collections of unconnected systems, each generating a diversity of data. While these systems are suitable for rapidly launching services and are aimed at solving individual challenges, digital enterprises will need to take a platform approach to unlock the full value of the data they generate.
Data-driven enterprises can increase revenue and shift to higher margin offerings through personalisation tools, such as recommendation engines and dynamic pricing. Cost cutting can be achieved with predictive maintenance that relies on streaming sensor data integrated with external data sources. Increasingly, advanced organisations will monetise their integrated data by providing insights as a service.
Digital enterprises face new challenges – growing complexity, data explosion, and skills gap.
Here are 5 ways in which IT teams can mitigate these challenges.
- Data & AI projects must focus on data access. When the organisation can unify data and transmit it securely wherever it needs to, it will be ready to begin developing applications that utilise machine learning, deep learning, and AI.
- Transformation requires a hybrid cloud platform. Hybrid cloud provides the ability to place each workload in an environment that makes the most sense for the business, while still reaping the benefits of a unified platform.
- Application modernisation unlocks future value. The importance of delivering better experiences to internal and external stakeholders has not gone down; new experiences need modern applications.
- Data management needs to be unified and automated. Digital transformation initiatives result in ever-expanding technology estates and growing volumes of data that cannot be managed with manual processes.
- Cyber strategy should be Zero Trust – backed by the right technologies. Organisations have to build Digital Trust with privacy, protection, and compliance at the core. The Zero Trust strategy should be backed by automated identity governance, robust access and management policies, and least privilege.
Read below to find out more.
Download The Future of Business: 5 Ways IT Teams Can Help Unlock the Value of Data as a PDF
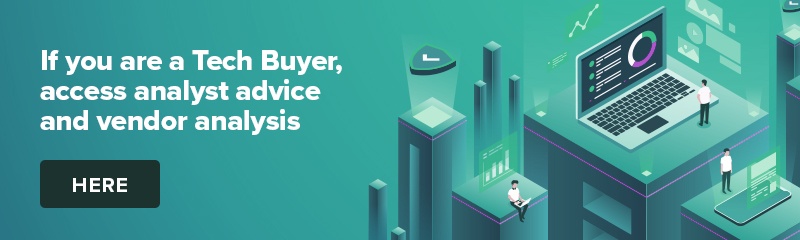
Earlier this month, I had the privilege of attending Oracle’s Executive Leadership Forum, to mark the launch of the Oracle Cloud Singapore Region. Oracle now has 34 cloud regions worldwide across 17 countries and intends to expand their footprint further to 44 regions by the end of 2022. They are clearly aiming for rapid expansion across the globe, leveraging their customers’ need to migrate to the cloud. The new Singapore region aims to support the growing demand for enterprise cloud services in Southeast Asia, as organisations continue to focus on business and digital transformation for recovery and future success.
Here are my key takeaways from the session:
#1 Enabling the Digital Futures
The theme for the session revolved around Digital Futures. Ecosystm research shows that 77% of enterprises in Southeast Asia are looking at technology to pivot, shift, change and adapt for the Digital Futures. Organisations are re-evaluating and accelerating the use of digital technology for back-end and customer workloads, as well as product development and innovation. Real-time data access lies at the backbone of these technologies. This means that Digital & IT Teams must build the right and scalable infrastructure to empower a digital, data-driven organisation. However, being truly data-driven requires seamless data access, irrespective of where they are generated or stored, to unlock the full value of the data and deliver the insights needed. Oracle Cloud is focused on empowering this data-led economy through data sovereignty, lower latency, and resiliency.
The Oracle Cloud Singapore Region brings to Southeast Asia an integrated suite of applications and the Oracle Cloud Infrastructure (OCI) platform that aims to help run native applications, migrate, and modernise them onto cloud. There has been a growing interest in hybrid cloud in the region, especially in large enterprises. Oracle’s offering will give companies the flexibility to run their workloads on their cloud and/or on premises. With the disruption that the pandemic has caused, it is likely that Oracle customers will increasingly use the local region for backup and recovery of their on-premises workloads.
#2 Partnering for Success
Oracle has a strong partner ecosystem of collaboration platforms, consulting and advisory firms and co-location providers, that will help them consolidate their global position. To begin with they rely on third-party co-location providers such as Equinix and Digital Realty for many of their data centres. While Oracle will clearly benefit from these partnerships, the benefit that they can bring to their partners is their ability to build a data fabric – the architecture and services. Organisations are looking to build a digital core and layer data and AI solutions on top of the core; Oracle’s ability to handle complex data structures will be important to their tech partners and their route to market.
#3 Customers Benefiting from Oracle’s Core Strengths
The session included some customer engagement stories, that highlight Oracle’s unique strengths in the enterprise market. One of Oracle’s key clients in the region, Beyonics – a precision manufacturing company for the Healthcare, Automotive and Technology sectors – spoke about how Oracle supported them in their migration and expansion of ERP platform from 7 to 22 modules onto the cloud. Hakan Yaren, CIO, APL Logistics says, “We have been hosting our data lake initiative on OCI and the data lake has helped us consolidate all these complex data points into one source of truth where we can further analyse it”.
In both cases what was highlighted was that Oracle provided the platform with the right capacity and capabilities for their business growth. This demonstrates the strength of Oracle’s enterprise capabilities. They are perhaps the only tech vendor that can support enterprises equally for their database, workloads, and hardware requirements. As organisations look to transform and innovate, they will benefit from the strength of these enterprise-wide capabilities that can address multiple pain points of their digital journeys.
#4 Getting Front and Centre of the Start-up Ecosystem
One of the most exciting announcements for me was Oracle’s focus on the start-up ecosystem. They make a start with a commitment to offer 100 start-ups in Singapore USD 30,000 each, in Oracle Cloud credits over the next two years. This is good news for the country’s strong start-up community. It will be good to see Oracle build further on this support so that start-ups can also benefit from Oracles’ enterprise offerings. This will be a win-win for Oracle. The companies they support could be “soonicorns” – the unicorns of tomorrow; and Oracle will get the opportunity to grow their accounts as these companies grow. Given the momentum of the data economy, these start-ups can benefit tremendously from the core differentiators that OCI can bring to their data fabric design. While this is a good start, Oracle should continue to engage with the start-up community – not just in Singapore but across Southeast Asia.
#5 Commitment to Sustainability at the Core of the Digital Futures
Another area where Oracle is aligning themselves to the future is in their commitment to sustainability. Earlier this year they pledged to power their global operations with 100% renewable energy by 2025, with goals set for clean cloud, hardware recycling, waste reduction and responsible sourcing. As Jacqueline Poh, Managing Director, EDB Singapore pointed out, sustainability can no longer be an afterthought and must form part of the core growth strategy. Oracle has aligned themselves to the SG Green Plan that aims to achieve sustainability targets under the UN’s 2030 Sustainable Development Agenda.
Cloud infrastructure is going to be pivotal in shaping the future of the Digital Economy; but the ability to keep sustainability at its core will become a key differentiator. To quote Sir David Attenborough from his speech at COP26, “In my lifetime, I’ve witnessed a terrible decline. In yours, you could and should witness a wonderful recovery”
Conclusion
Oracle operates in a hyper competitive world – AWS, Microsoft and Google have emerged as the major hyperscalers over the last few years. With their global expansion plans and targeted offerings to help enterprises achieve their transformation goals, Oracle is positioned well to claim a larger share of the cloud market. Their strength lies in the enterprise market, and their cloud offerings should see them firmly entrenched in that segment. I hope however, that they will keep an equal focus on their commitment to the start-up ecosystem. Most of today’s hyperscalers have been successful in building scale by deeply entrenching themselves in the core innovation ecosystem – building on the ‘possibilities’ of the future rather than just on the ‘financial returns’ today.
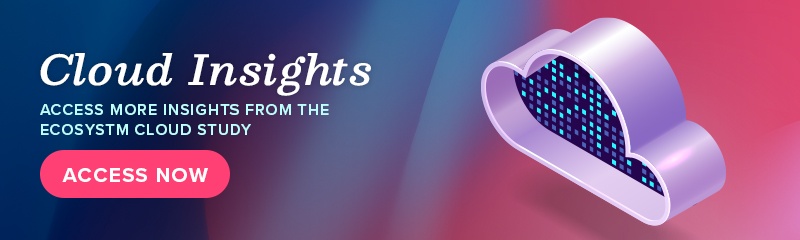
AI has become intrinsic to our personal lives – we are often completely unaware of technology’s influence on our daily lives. For enterprises too, tech solutions often come embedded with AI capabilities. Today, an organisation’s ability to automate processes and decisions is often dependent more on their desire and appetite for tech adoption, than the technology itself.
In 2022 the key focus for enterprises will be on being able to trust their Data & AI solutions. This will include trust in their IT infrastructure, architecture and AI services; and stretch to being able to participate in trusted data sharing models. Technology vendors will lead this discussion and showcase their solutions in the light of trust.
Read what Ecosystm analysts, Darian Bird, Niloy Mukherjee, Peter Carr and Tim Sheedy think will be the leading Data & AI trends in 2022.
Click here to download Ecosystm Predicts: The Top 5 Trends for Data & AI in 2022 as PDF
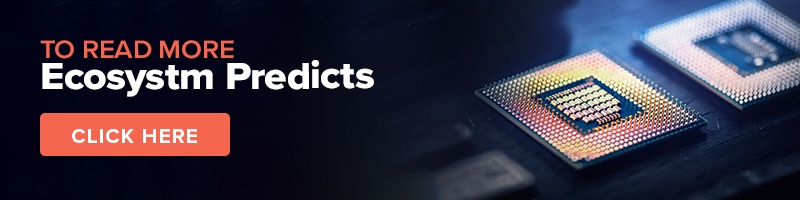