India is undergoing a remarkable transformation across various industries, driven by rapid technological advancements, evolving consumer preferences, and a dynamic economic landscape. From the integration of new-age technologies like GenAI to the adoption of sustainable practices, industries in India are redefining their operations and strategies to stay competitive and relevant.
Here are some organisations that are leading the way.
Download ‘From Tradition to Innovation: Industry Transformation in India’ as a PDF
Redefining Customer Experience in the Financial Sector
Financial inclusion. India’s largest bank, the State Bank of India, is leading financial inclusion with its YONO app, to enhance accessibility. Initial offerings include five core banking services: cash withdrawals, cash deposits, fund transfers, balance inquiries, and mini statements, with plans to include account opening and social security scheme enrollments.
Customer Experience. ICICI Bank leverages RPA to streamline repetitive tasks, enhancing customer service with its virtual assistant, iPal, for handling queries and transactions. HDFC Bank customer preference insights to offer tailored financial solutions, while Axis Bank embraces a cloud-first strategy to digitise its platform and improve customer interfaces.
Indian banks are also collaborating with fintechs to harness new technologies for better customer experiences. YES Bank has partnered with Paisabazaar to simplify loan applications, and Canara HSBC Life Insurance has teamed up with Artivatic.AI to enhance its insurance processes via an AI-driven platform.
Improving Healthcare Access
Indian healthcare organisations are harnessing technology to enhance efficiency, improve patient experiences, and enable remote care.
Apollo Hospitals has launched an automated patient monitoring system that alerts experts to health deteriorations, enabling timely interventions through remote monitoring. Manipal Hospitals’ video consultation app reduces emergency department pressure by providing medical advice, lab report access, bill payments, appointment bookings, and home healthcare requests, as well as home medication delivery and Fitbit monitoring. Omni Hospitals has also implemented AI-based telemedicine for enhanced patient engagement and remote monitoring.
The government is also driving the improvement of healthcare access. eSanjeevani is the world’s largest government-owned telemedicine system, with the capacity to handle up to a million patients a day.
Driving Retail Agility & Consumer Engagement
India’s Retail sector, the fourth largest globally, contributes over 10% of the nation’s GDP. To stay competitive and meet evolving consumer demands, Indian retailers are rapidly adopting digital technologies, from eCommerce platforms to AI.
Omnichannel Strategies. Reliance Retail integrates physical stores with digital platforms like JioMart to boost sales and customer engagement. Tata CLiQ’s “phygital” approach merges online and offline shopping for greater convenience while Shoppers Stop uses RFID and data analytics for improved in-store experiences, online shopping, and targeted marketing.
Retail AI. Flipkart’s AI-powered shopping assistant, Flippi uses ML for conversational product discovery and intuitive guidance. BigBasket employs IoT-led AI to optimise supply chain and improve product quality.
Reshaping the Automotive Landscape
Tech innovation, from AI/ML to connected vehicle technologies, is revolutionising the Automotive sector. This shift towards software-defined vehicles and predictive supply chain management underscores the industry’s commitment to efficiency, transparency, safety, and environmental sustainability.
Maruti Suzuki’s multi-pronged approach includes collaborating with over 60 startups through its MAIL program and engaging Accenture to drive tech change. Maruti has digitised 24 out of 26 customer touchpoints, tracking every interaction to enhance customer service. In the Auto OEM space, they are shifting to software-defined vehicles and operating models.
Tata Motors is leveraging cloud, AI/ML, and IoT to enhancing efficiency, improving safety, and driving sustainability across its operations. Key initiatives include connected vehicles, automated driving, dealer management, cybersecurity, electric powertrains, sustainability, and supply chain optimisation.
Streamlining India’s Logistics Sector
India’s logistics industry is on the cusp of a digital revolution as it embraces cutting-edge technologies to streamline processes and reduce environmental impact.
Automation and Predictive Analytics. Automation is transforming warehousing operations in India, with DHL India automating sortation centres to handle 6,000 shipments per hour. Predictive analytics is reshaping logistics decision-making, with Delhivery optimising delivery routes to ensure timely service.
Sustainable Practices. The logistics sector contributes one-third of global carbon emissions. To combat this, Amazon India will convert its delivery fleet to 100% EVs by 2030 to reduce emissions and fuel costs. Blue Energy Motors is also producing 10,000 heavy-duty LNG trucks annually for zero-emission logistics.
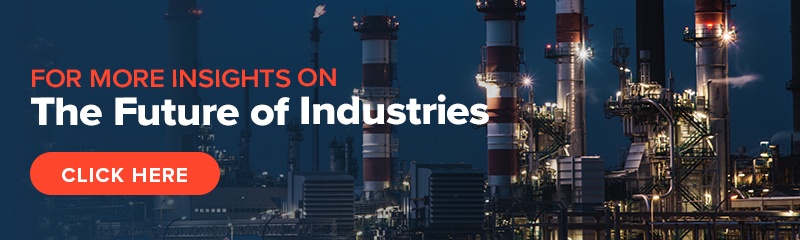
If you have seen me present recently – or even spoken to me for more than a few minutes, you’ve probably heard me go on about how the AI discussions need to change! At the moment, most senior executives, board rooms, governments, think tanks and tech evangelists are running around screaming with their hands on their ears when it comes to the impact of AI on jobs and society.
We are constantly being bombarded with the message that AI will help make knowledge workers more productive. AI won’t take people’s jobs – in fact it will help to create new jobs – you get the drift; you’ve been part of these conversations!
I was at an event recently where a leading cloud provider had a huge slide with the words: “Humans + AI Together” in large font across the screen. They then went on to demonstrate an opportunity for AI. In a live demo, they had the customer of a retailer call a store to check for stock of a dress. The call was handled by an AI solution, which engaged in a natural conversation with the customer. It verified their identity, checked dress stock at the store, processed the order, and even confirmed the customer’s intent to use their stored credit card.
So, in effect, on one slide, the tech provider emphasised that AI was not going to take our jobs, and two minutes later they showed how current AI capabilities could replace humans – today!
At an analyst event last week, representatives from three different tech providers told analysts how Microsoft Copilot is freeing up 10-15 hours a week. For a 40-hour work week, that’s a 25-38 time saving. In France (where the work week is 35 hours), that’s up to 43% of their time saved. So, by using a single AI platform, we can save 25-43% of our time – giving us the ability to work on other things.
What are the Real Benefits of AI?
The critical question is: What will we do with this saved time? Will it improve revenue or profit for businesses? AI might make us more agile, faster, more innovative but unless that translates to benefits on the bottom line, it is pointless. For example, adopting AI might mean we can create three times as many products. However, if we don’t make any more revenue and/or profit by having three times as many products, then any productivity benefit is worthless. UNLESS it is delivered through decreased costs.
We won’t need as many humans in our contact centres if AI is taking calls. Ideally, AI will lead to more personalised customer experiences – which will drive less calls to the contact centre in the first place! Even sales-related calls may disappear as personal AI bots will find deals and automatically sign us up. Of course, AI also costs money, particularly in terms of computing power. Some of the productivity uplift will be offset by the extra cost of the AI tools and platforms.
Many benefits that AI delivers will become table stakes. For example, if your competitor is updating their product four times a year and you are updating it annually, you might lose market share – so the benefits of AI might be just “keeping up with the competition”. But there are many areas where additional activity won’t deliver benefits. Organisations are unlikely to benefit from three times more promotional SMSs or EDMs and design work or brand redesigns.
I also believe that AI will create new roles. But you know what? AI will eventually do those jobs too. When automation came to agriculture, workers moved to factories. When automation came to factories, workers moved to offices. The (literally) trillion-dollar question is where workers go when automation comes to the office.
The Wider Impact of AI
The issue is that very few senior people in businesses or governments are planning for a future where maybe 30% of jobs done by knowledge workers go to AI. This could lead to the failure of economies. Government income will fall off a cliff. It will be unemployment on levels not seen since the great depression – or worse. And if we have not acknowledged these possible outcomes, how can we plan for it?
This is what I call the “grown up conversation about AI”. This is acknowledging the opportunity for AI and its impacts on companies, industries, governments and societies. Once we acknowledge these likely outcomes we can plan for it.
And that’s what I’ll discuss shortly – look out for my next Ecosystm Insight: The Three Possible Solutions for AI-driven Mass Unemployment.
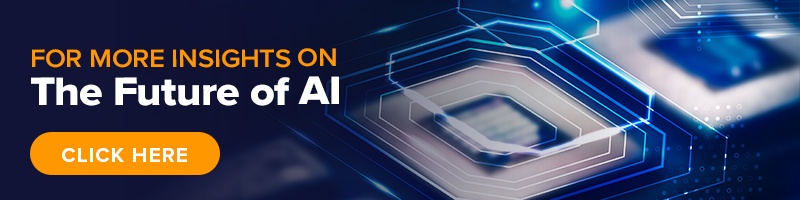
In my last Ecosystm Insights, I spoke about why organisations need to think about the Voice of the Customer (VoC) quite literally. Organisations need to listen to what their customers are telling them – not just to the survey questions they responded to, answering pre-defined questions that the organisations want to hear about.
The concept of customer feedback is evolving, and how organisations design and manage VoC programs must also change. Technology is now capable of enabling customer teams to tap into all those unsolicited, and often unstructured, raw feedback sources. Think contact centre conversations (calls, chats, chatbots, emails, complaints, call notes), CRM notes, online reviews, social media, etc. Those are all sources of raw customer feedback, waiting to be converted into customer insights.
Organisations can now find the capability of extracting customer insight from raw data across a wide range of solutions, from VoC platforms, data management platforms, contact centre solutions, text analytics players, etc. The expanding tech ecosystem presents opportunities for organisations to enhance their programs. However, navigating this breadth of options can also be confusing as they strive to identify the most suitable tools for their requirements.
As CX programs mature and shift from survey feedback to truly listening to customers, the demand for tech solutions tailored to various needs increases.
Where are tech vendors headed?
As part of my job as CX Consultant & Tech Advisor, I spend a lot of time working with my clients. But I also spend a lot of time speaking with technology vendors, who provide the solutions my clients need. Over the last few weeks and months there’s been a flurry of activity across the CX technology market with lots of product announcements around one specific topic. You guessed it, GenAI.
So, I invested some time in finding out how tech vendors are evolving their offerings. From Medallia, InMoment, Thematic, LiquidVoice, Concentrix, Snowflake, Nice, to Tethr – a broad variety of different vendors, but all with one thing in common; they help analyse customer feedback data.
And I like what I hear. The conversation has not been about GenAI because of GenAI, but about use cases and real-life applications for CX practitioners, including Insights & Research team, Contact Centre, CX, VoC, Digital teams, and so on. The list is long when we include everyone who has a role to play in creating, maintaining, and improving customer experiences.
It’s no wonder that many different vendors have started to embed those capabilities into their solutions and launch new products or features. The tech landscape is becoming increasingly fragmented at this stage.
What are an organisation’s tech options?
- The traditional VoC platform providers typically offer some text analytics capabilities (although not always included in the base price) and have started to tap into the contact centre solutions as well. Some also offer some social media or online review analysis, leaving organisations with a relatively good understanding of customer sentiment and a better understanding of their CX.
- Contact centre solutions are traditionally focused on analysing calls for Quality Assurance (QA) purposes and use surveys for agent coaching. Many contact centre players have evolved their portfolios to include text analytics or conversational intelligence to extract broader customer insights. Although at this stage they’re not always shared with the rest of the organisation (one step at a time…).
- Conversational analytics/intelligence providers have emerged over the last few years and are a powerhouse for contact centre and chatbot conversations. The contact centre really is the treasure trove of customer insights, although vastly underutilised for it so far!
- CRMs are the backbone of the customer experience management toolkit as they hold a vast amount of metadata. They’ve also been able to send surveys for a while now. Analysing unstructured data however (whether survey verbatim or otherwise) isn’t one of their strengths. This leaves organisations with a lot of data but not necessarily insights.
- Social media listening tools are often standalone tools used by the social media teams. There are not many instances of them being used for the analysis of other unstructured feedback.
- Digital/website feedback tools, in line with some of the above, are centred around collecting feedback, not necessarily analysing the unstructured feedback.
- Pure text analytics players are traditionally focused on analysing surveys verbatim. As this is their core offering, they tend to be proficient in it and have started to broaden their portfolios to include other unstructured feedback sources.
- Customer Data Platforms (CDP)/ Data Management Platforms (DMP) are more focused on quantitative data about customers and their experiences. Although many speak about their ability to analyse unstructured feedback as well, it doesn’t appear to be their strengths.
Conclusion
But what does that leave organisations with? Apart from very confused tech users trying to find the right solution for their organisation.
At this stage, there is immense market fragmentation, with many vendors from different core capabilities starting to incorporate capabilities to analyse unstructured data in the wake of the GenAI boom. However, a market convergence is expected.
While we watch how the market unfolds, one thing is certain. Organisations and customer teams will need to adjust – and that includes the tech stack as well as the CX program set up. With customer feedback now coming from anywhere within or outside the organisation, there is a need for a consolidated source of truth to make sense of it all and move from raw data to customer insights. While organisations will benefit immensely from a consolidated customer data repository, it’s also crucial to break down organisational silos at the same time and democratise insights as widely as possible to enable informed decision-making.
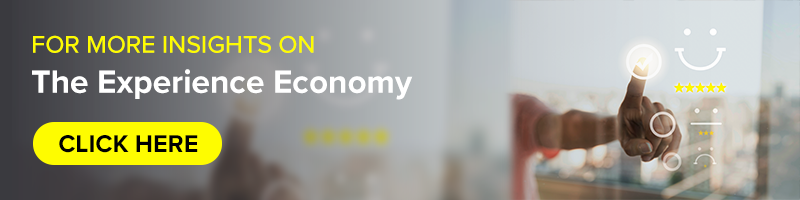
AI has become a business necessity today, catalysing innovation, efficiency, and growth by transforming extensive data into actionable insights, automating tasks, improving decision-making, boosting productivity, and enabling the creation of new products and services.
Generative AI stole the limelight in 2023 given its remarkable advancements and potential to automate various cognitive processes. However, now the real opportunity lies in leveraging this increased focus and attention to shine the AI lens on all business processes and capabilities. As organisations grasp the potential for productivity enhancements, accelerated operations, improved customer outcomes, and enhanced business performance, investment in AI capabilities is expected to surge.
In this eBook, Ecosystm VP Research Tim Sheedy and Vinod Bijlani and Aman Deep from HPE APAC share their insights on why it is crucial to establish tailored AI capabilities within the organisation.
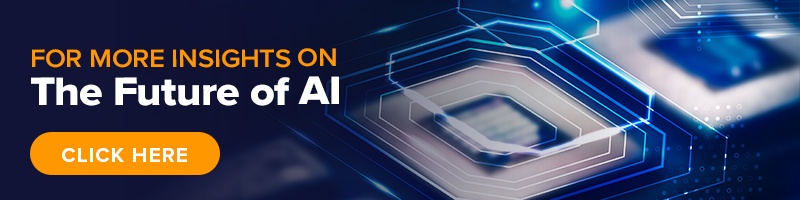
In my last Ecosystm Insights, I spoke about the implications of the shift from Predictive AI to Generative AI on ROI considerations of AI deployments. However, from my discussions with colleagues and technology leaders it became clear that there is a need to define and distinguish between Predictive AI and Generative AI better.
Predictive AI analyses historical data to predict future outcomes, crucial for informed decision-making and strategic planning. Generative AI unlocks new avenues for innovation by creating novel data and content. Organisations need both – Predictive AI for enhancing operational efficiencies and forecasting capabilities and Generative AI to drive innovation; create new products, services, and experiences; and solve complex problems in unprecedented ways.
This guide aims to demystify these categories, providing clarity on their differences, applications, and examples of the algorithms they use.
Predictive AI: Forecasting the Future
Predictive AI is extensively used in fields such as finance, marketing, healthcare and more. The core idea is to identify patterns or trends in data that can inform future decisions. Predictive AI relies on statistical, machine learning, and deep learning models to forecast outcomes.
Key Algorithms in Predictive AI
- Regression Analysis. Linear and logistic regression are foundational tools for predicting a continuous or categorical outcome based on one or more predictor variables.
- Decision Trees. These models use a tree-like graph of decisions and their possible consequences, including chance event outcomes, resource costs and utility.
- Random Forest (RF). An ensemble learning method that operates by constructing a multitude of decision trees at training time to improve predictive accuracy and control over-fitting.
- Gradient Boosting Machines (GBM). Another ensemble technique that builds models sequentially, each new model correcting errors made by the previous ones, used for both regression and classification tasks.
- Support Vector Machines (SVM). A supervised machine learning model that uses classification algorithms for two-group classification problems.
Generative AI: Creating New Data
Generative AI, on the other hand, focuses on generating new data that is similar but not identical to the data it has been trained on. This can include anything from images, text, and videos to synthetic data for training other AI models. GenAI is particularly known for its ability to innovate, create, and simulate in ways that predictive AI cannot.
Key Algorithms in Generative AI
- Generative Adversarial Networks (GANs). Comprising two networks – a generator and a discriminator – GANs are trained to generate new data with the same statistics as the training set.
- Variational Autoencoders (VAEs). These are generative algorithms that use neural networks for encoding inputs into a latent space representation, then reconstructing the input data based on this representation.
- Transformer Models. Originally designed for natural language processing (NLP) tasks, transformers can be adapted for generative purposes, as seen in models like GPT (Generative Pre-trained Transformer), which can generate coherent and contextually relevant text based on a given prompt.
Comparing Predictive and Generative AI
The fundamental difference between the two lies in their primary objectives: Predictive AI aims to forecast future outcomes based on past data, while Generative AI aims to create new, original data that mimics the input data in some form.
The differences become clearer when we look at these examples.
Predictive AI Examples
- Supply Chain Management. Analyses historical supply chain data to forecast demand, manage inventory levels, reduces costs and improve delivery times.
- Healthcare. Analysing patient records to predict disease outbreaks or the likelihood of a disease in individual patients.
- Predictive Maintenance. Analyse historical and real-time data and preemptively identifies system failures or network issues, enhancing infrastructure reliability and operational efficiency.
- Finance. Using historical stock prices and indicators to predict future market trends.
Generative AI Examples
- Content Creation. Generating realistic images or art from textual descriptions using GANs.
- Text Generation. Creating coherent and contextually relevant articles, stories, or conversational responses using transformer models like GPT-3.
- Chatbots and Virtual Assistants. Advanced GenAI models are enhancing chatbots and virtual assistants, making them more realistic.
- Automated Code Generation. By the use of natural language descriptions to generate programming code and scripts, to significantly speed up software development processes.
Conclusion
Organisations that exclusively focus on Generative AI may find themselves at the forefront of innovation, by leveraging its ability to create new content, simulate scenarios, and generate original data. However, solely relying on Generative AI without integrating Predictive AI’s capabilities may limit an organisation’s ability to make data-driven decisions and forecasts based on historical data. This could lead to missed opportunities to optimise operations, mitigate risks, and accurately plan for future trends and demands. Predictive AI’s strength lies in analysing past and present data to inform strategic decision-making, crucial for long-term sustainability and operational efficiency.
It is essential for companies to adopt a dual-strategy approach in their AI efforts. Together, these AI paradigms can significantly amplify an organisation’s ability to adapt, innovate, and compete in rapidly changing markets.
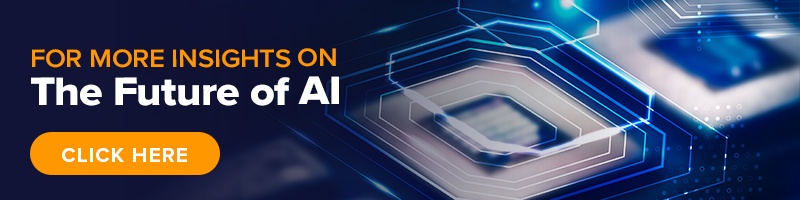
The tech industry tends to move in waves, driven by the significant, disruptive changes in technology, such as cloud and smartphones. Sometimes, it is driven by external events that bring tech buyers into sync – such as Y2K and the more recent pandemic. Some tech providers, such as SAP and Microsoft, are big enough to create their own industry waves. The two primary factors shaping the current tech landscape are AI and the consequential layoffs triggered by AI advancements.
While many of the AI startups have been around for over five years, this will be the year they emerge as legitimate solutions providers to organisations. Amidst the acceleration of AI-driven layoffs, individuals from these startups will go on to start new companies, creating the next round of startups that will add value to businesses in the future.
Tech Sourcing Strategies Need to Change
The increase in startups implies a change in the way businesses manage and source their tech solutions. Many organisations are trying to reduce tech debt, by typically consolidating the number of providers and tech platforms. However, leveraging the numerous AI capabilities may mean looking beyond current providers towards some of the many AI startups that are emerging in the region and globally.
The ripple effect of these decisions is significant. If organisations opt to enhance the complexity of their technology architecture and increase the number of vendors under management, the business case must be watertight. There will be less of the trial-and-error approach towards AI from 2023, with a heightened emphasis on clear and measurable value.
AI Startups Worth Monitoring
Here is a selection of AI startups that are already starting to make waves across Asia Pacific and the globe.
- ADVANCE.AI provides digital transformation, fraud prevention, and process automation solutions for enterprise clients. The company offers services in security and compliance, digital identity verification, and biometric solutions. They partner with over 1,000 enterprise clients across Southeast Asia and India across sectors, such as Banking, Fintech, Retail, and eCommerce.
- Megvii is a technology company based in China that specialises in AI, particularly deep learning. The company offers full-stack solutions integrating algorithms, software, hardware, and AI-empowered IoT devices. Products include facial recognition software, image recognition, and deep learning technology for applications such as consumer IoT, city IoT, and supply chain IoT.
- I’mCloud is based in South Korea and specialises in AI, big data, and cloud storage solutions. The company has become a significant player in the AI and big data industry in South Korea. They offer high-quality AI-powered chatbots, including for call centres and interactive educational services.
- H2O.ai provides an AI platform, the H2O AI Cloud, to help businesses, government entities, non-profits, and academic institutions create, deploy, monitor, and share data models or AI applications for various use cases. The platform offers automated machine learning capabilities powered by H2O-3, H2O Hydrogen Torch, and Driverless AI, and is designed to help organisations work more efficiently on their AI projects.
- Frame AI provides an AI-powered customer intelligence platform. The software analyses human interactions and uses AI to understand the driving factors of business outcomes within customer service. It aims to assist executives in making real-time decisions about the customer experience by combining data about customer interactions across various platforms, such as helpdesks, contact centres, and CRM transcripts.
- Uizard offers a rapid, AI-powered UI design tool for designing wireframes, mockups, and prototypes in minutes. The company’s mission is to democratise design and empower non-designers to build digital, interactive products. Uizard’s AI features allow users to generate UI designs from text prompts, convert hand-drawn sketches into wireframes, and transform screenshots into editable designs.
- Moveworks provides an AI platform that is designed to automate employee support. The platform helps employees to automate tasks, find information, query data, receive notifications, and create content across multiple business applications.
- Tome develops a storytelling tool designed to reduce the time required for creating slides. The company’s online platform creates or emphasises points with narration or adds interactive embeds with live data or content from anywhere on the web, 3D renderings, and prototypes.
- Jasper is an AI writing tool designed to assist in generating marketing copy, such as blog posts, product descriptions, company bios, ad copy, and social media captions. It offers features such as text and image AI generation, integration with Grammarly and other Chrome extensions, revision history, auto-save, document sharing, multi-user login, and a plagiarism checker.
- Eightfold AI provides an AI-powered Talent Intelligence Platform to help organisations recruit, retain, and grow a diverse global workforce. The platform uses AI to match the right people to the right projects, based on their skills, potential, and learning ability, enabling organisations to make informed talent decisions. They also offer solutions for diversity, equity, and inclusion (DEI), skills intelligence, and governance, among others.
- Arthur provides a centralised platform for model monitoring. The company’s platform is model and platform agnostic, and monitors machine learning models to ensure they deliver accurate, transparent, and fair results. They also offer services for explainability and bias mitigation.
- DNSFilter is a cloud-based, AI-driven content filtering and threat protection service, that can be deployed and configured within minutes, requiring no software installation.
- Spot AI specialises in building a modern AI Camera System to create safer workplaces and smarter operations for every organisation. The company’s AI Camera System combines cloud and edge computing to make video footage actionable, allowing customers to instantly surface and resolve problems. They offer intelligent video recorders, IP cameras, cloud dashboards, and advanced AI alerts to proactively deliver insights without the need to manually review video footage.
- People.ai is an AI-powered revenue intelligence platform that helps customers win more revenue by providing sales, RevOps, marketing, enablement, and customer success teams with valuable insights. The company’s platform is designed to speed up complex enterprise sales cycles by engaging the right people in the right accounts, ultimately helping teams to sell more and faster with the same headcount.
These examples highlight a few startups worth considering, but the landscape is rich with innovative options for organisations to explore. Similar to other emerging tech sectors, the AI startup market will undergo consolidation over time, and incumbent providers will continue to improve and innovate their own AI capabilities. Till then, these startups will continue to influence enterprise technology adoption and challenge established providers in the market.
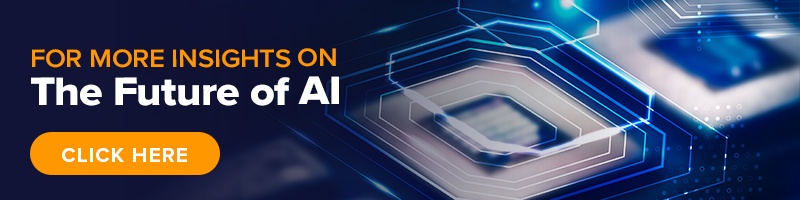
The impact of AI on Customer Experience (CX) has been profound and continues to expand. AI allows a a range of advantages, including improved operational efficiency, cost savings, and enhanced experiences for both customers and employees.
AI-powered solutions have the capability to analyse vast volumes of customer data in real-time, providing organisations with invaluable insights into individual preferences and behaviour. When executed effectively, the ability to capture, analyse, and leverage customer data at scale gives organisations significant competitive edge. Most importantly, AI unlocks opportunities for innovation.
Read on to discover the transformative impact of AI on customer experiences.
Click here to download ‘Customer Experience Redefined: The Role of AI’ as a PDF
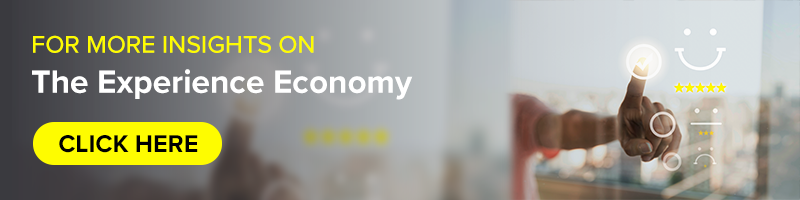
Generative AI is seeing enterprise interest and early adoption enhancing efficiency, fostering innovation, and pushing the boundaries of possibility. It has the potential of reshaping industries – and fast!
However, alongside its immense potential, Generative AI also raises concerns. Ethical considerations surrounding data privacy and security come to the forefront, as powerful AI systems handle vast amounts of sensitive information.
Addressing these concerns through responsible AI development and thoughtful regulation will be crucial to harnessing the full transformative power of Generative AI.
Read on to find out the key challenges faced in implementing Generative AI and explore emerging use cases in industries such as Financial Services, Retail, Manufacturing, and Healthcare.
Download ‘Generative AI: Industry Adoption’ as a PDF
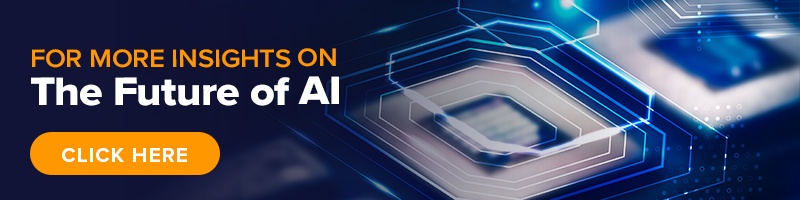
Verint has announced the intention to acquire Conversocial, a US-based social media management system provider for USD 50 million to integrate social messaging capabilities across Verint’s cloud platform. The deal is expected to be closed in Verint’s third quarter subject to customary closing conditions and regulatory clearances.
Verint has been expanding their digital engagement capabilities through acquisitions. In June, Verint expanded their Workforce Management (WFM) offerings to include AI-driven insights for better hiring decisions through the acquisition of HireIQ. To extend Verint’s omnichannel cloud Voice of the Customer (VoC) portfolio, Verint acquired Foresee. Verint is also building IVA capabilities and recently launched a low code version of their IVA solution to make it easier for brands to build the solution without the need for technical knowledge.
The Need to Enhance Digital Engagement
Using self-service and messaging as the first point of connection to engage with a brand is growing rapidly. It accelerated during the pandemic, and it is common now for individuals to engage with their financial institution, airlines, retail and others through social media. Now that customers are demanding it, brands are lifting their game and engaging with customers on the platform of their choices. This acquisition will allow Verint to deepen their digital engagement with customers across Marketing, Contact Centres and digital functions – it follows the pulse of today’s customers.
Digital discussions are accelerating and having a platform that can orchestrate as well as understand all the data from each digital and social messaging channel is important. Verint is taking the data discussion seriously and earlier this year they launched Engagement Data Management Solution (EDM). The ‘’data’’ piece is huge and cuts across functions – from back-office communications to gathering data across all channels and touchpoints. However, where this is going wrong for some enterprises is that all the data they collect sits in multiple repositories; in some instances the data has not been analysed for years! Managing the multiple social experiences, including data management and insights from these multiple sources, will be key to delivering proactive customer experience.
The data discussion is particularly significant for a vendor such as Verint – they are well known for their speech analytics and compliance management capabilities. These are all critical to managing multiple channels of conversation. They help agents to be accurate, efficient, and compliant; allow organisations to use asynchronous channels and social messaging and digital channels; immediately rectified errors through monitoring the data on the channels and so on. More importantly, they allow organisations to pick up points from conversations that can be passed on to Marketing to gauge the effectiveness of the messaging and campaigns. Organisations can ‘’identify and fix” problems by truly listening to customers.
Why Conversocial
If we look at the Conversocial customer stories, we realise how relevant their offerings are to industry requirements. They offer brands the ability to engage through an automated channel and chatbots. Whirlpool appears to have benefited by integrating channels to deliver better customer care as well as communicating with field engineers through WhatsApp. Another customer, Freshly – a meals delivery company – saw a spike in incoming queries at the start of the pandemic. They were able to use automation to ease of the load and say that 50% of the conversations were handled in-channel through automation without the need for human agent intervention. They were also able to use Facebook Messenger as a preferred contact channel and decreased their cost-per-contact.
This acquisition demonstrates how Verint is taking the digital and the data discussion seriously. CX Vendors that do not move fast in building end-to-end digital capabilities will find it hard to compete in a highly competitive CX market.
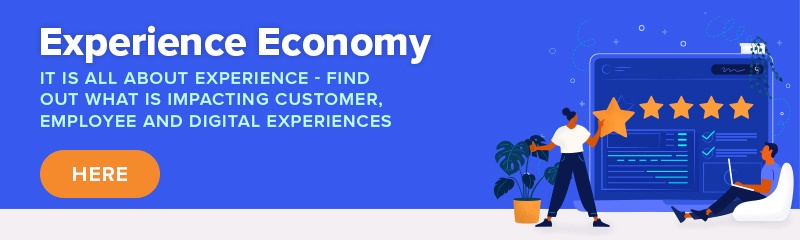