The global economy remains fragile due to multiple factors; and banking organisations will need to weather the storm. While large and well-capitalised banks are expected to fare better, there is a need for the industry to pursue new sources of value beyond traditional boundaries.
Banking industry leaders should be bold, proactive, and envision possibilities beyond current uncertainties. Technology has a key role to play in turning their innovation and resiliency goals into reality.
Read on to find out how the National Australia Bank, the Scottish National Investment Bank, the ANZ Bank, the Swiss National Bank, Mastercard, and the French banking group Crédit Agricole are leading the charge in driving innovation within the banking industry by investing in new technologies and exploring business models to better serve their customers.
Download ‘The Future of Banking’ as a PDF
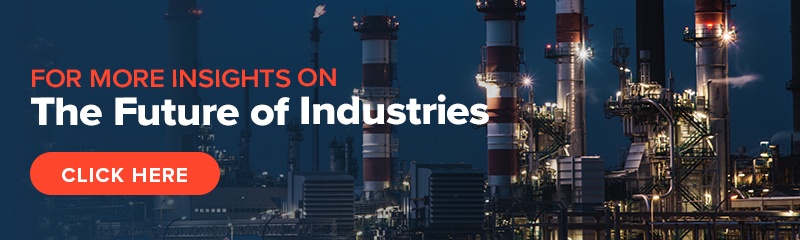
Artificial Intelligence (AI) is becoming embedded in financial services across consumer interactions and core business processes, including the use of chatbots and natural language processing (NLP) for KYC/AML risk assessment.
But what does AI mean for financial regulators? They are also consuming increasing amounts of data and are now using AI to gain new insights and inform policy decisions.
The efficiencies that AI offers can be harnessed in support of compliance within both financial regulation (RegTech) and financial supervision (SupTech). Authorities and regulated institutions have both turned to AI to help them manage the increased regulatory requirements that were put in place after the 2008 financial crisis. Ecosystm research finds that compliance is key to financial institutions (Figure 1).
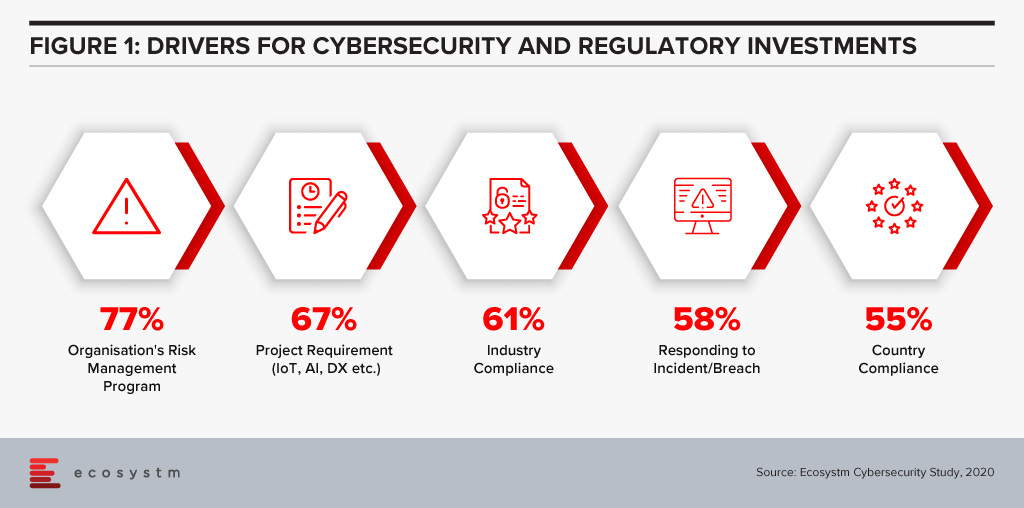
SupTech is maturing with more robust safeguards and frameworks, enabling the necessary advancements in technology implementation for AI and Machine Learning (ML) to be used for regulatory supervision. The Bank of England and the UK Financial Conduct Authority surveyed the industry in March 2019 to understand how and where AI and ML are being used, and their results indicated 80% of survey respondents were using ML. The most common application of SupTech is ML techniques, and more specifically NLP to create more efficient and effective supervisory processes.
Let us focus on the use of NLP, specifically on how it has been used by banking authorities for policy decision making during the COVID-19 crisis. AI has the potential to read and comprehend significant details from text. NLP, which is an important subset of AI, can be seen to have supported operations to stay updated with the compliance and regulatory policy shifts during this challenging period.
Use of NLP in Policy Making During COVID-19
The Financial Stability Board (FSB) coordinates at the international level, the work of national financial authorities and international standard-setting bodies in order to develop and promote the implementation of effective regulatory, supervisory and other financial sector policies. A recent FSB report delivered to G20 Finance Ministers and Central Bank Governors for their virtual meeting in October 2020 highlighted a number of AI use cases in national institutions.
We illustrate several use cases from their October report to show how NLP has been deployed specifically for the COVID-19 situation. These cases demonstrate AI aiding supervisory team in banks and in automating information extraction from regulatory documents using NLP.
De Nederlandsche Bank (DNB)
The DNB is developing an interactive reporting dashboard to provide insight for supervisors on COVID-19 related risks. The dashboard that is in development, enables supervisors to have different data views as needed (e.g. over time, by bank). Planned SupTech improvements include incorporating public COVID-19 information and/or analysing comment fields with text analysis.
Monetary Authority of Singapore (MAS)
MAS deployed automation tools using NLP to gather international news and stay abreast of COVID-19 related developments. MAS also used NLP to analyse consumer feedback on COVID-19 issues, and monitor vulnerabilities in the different customer and product segments. MAS also collected weekly data from regulated institutions to track the take-up of credit relief measures as the pandemic unfolded. Data aggregation and transformation were automated and visualised for monitoring.
US Federal Reserve Bank Board of Governors
One of the Federal Reserve Banks in the US is currently working on a project to develop an NLP tool used to analyse public websites of supervised regulated institutions to identify information on “work with your customer” programs, in response to the COVID-19 crisis.
Bank of England
The Bank developed a Policy Response Tracker using web scraping (targeted at the English versions of each authority/government website) and NLP for the extraction of key words, topics and actions taken in each jurisdiction. The tracker pulls information daily from the official COVID-19 response pages then runs it through specific criteria (e.g. user-defined keywords, metrics and risks) to sift and present a summary of the information to supervisors.
Market Implications
Even with its enhanced efficiencies, NLP in SupTech is still an aid to decision making and cannot replace the need for human judgement. NLP in policy decision is performing clearly defined information gathering tasks with greater efficiency and speed. But NLP cannot change the quality of the data provided, so data selection and choice are still critical to effective policy making.
For authorities, the use of SupTech could improve oversight, surveillance, and analytical capabilities. These efficiency gains and possible improvement in quality arising from automation of previously manual processes could be consideration for adoption.
Attention will be paid in 2021 to focusing on automation of processes using AI (Figure 2).
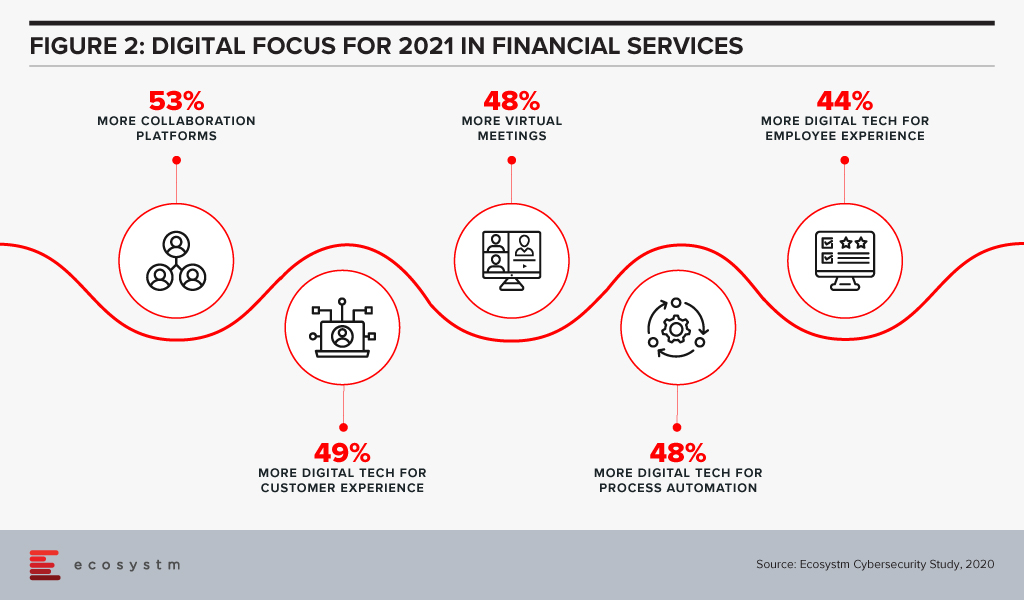
Based on a survey done by the FSB of its members (Figure 3), the majority of their respondents had a SupTech innovation or data strategy in place, with the use of such strategies growing significantly since 2016.
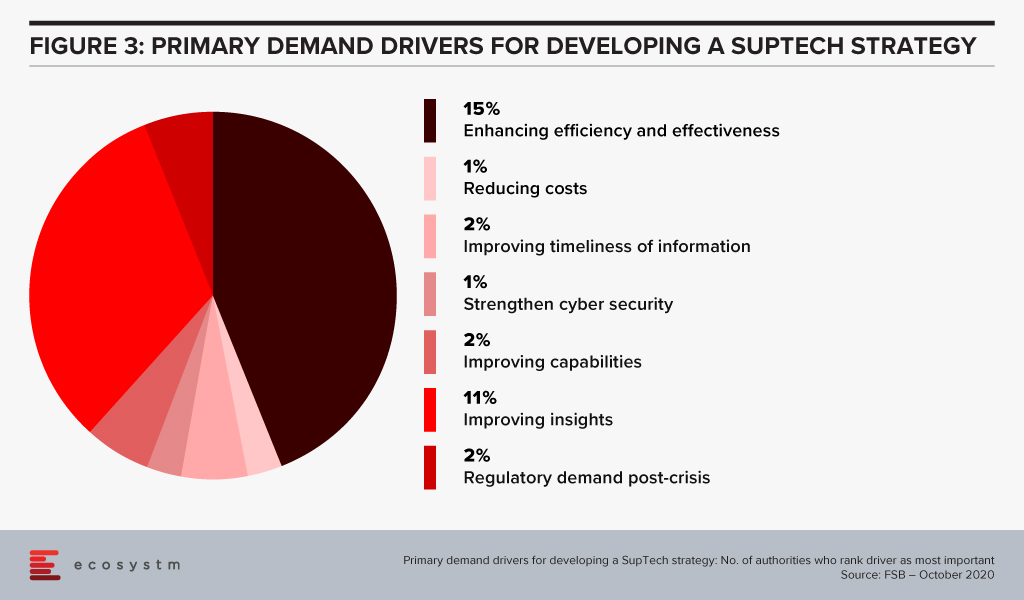
Summary
For more mainstream adoption, data standards and use of effective governance frameworks will be important. As seen from the FSB survey, SupTech applications are now used in reporting, data management and virtual assistance. But institutions still send the transaction data history in different reporting formats which results in a slower process of data analysing and data gathering. AI, using NLP, can help with this by streamlining data collection and data analytics. While time and cost savings are obvious benefits, the ability to identify key information (the proverbial needle in the haystack) can be a significant efficiency advantage.
Singapore FinTech Festival 2020: Infrastructure Summit
For more insights, attend the Singapore FinTech Festival 2020: Infrastructure Summit which will cover topics tied to creating infrastructure for a digital economy; and RegTech and SupTech policies to drive innovation and efficiencies in a co-Covid-19 world.
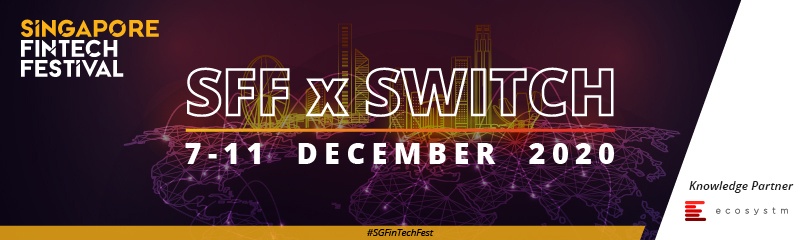