You know AI is the absolute next biggest thing. You know it is going to change our world!! It is the little technology trick start-ups use to disrupt industries. It enables crazy applications we have never thought of before! A few days ago, we were dazzled to learn of an AI app that promises to give one a credit rating score based on reading your face – essentially from just a photograph it can tell a prospective financier what the likelihood of your paying back the loan is!
Artificial Intelligence is real and has started becoming mainstream – chatbots using AI to answer queries are everywhere. AI is being used in stock trades, contact centre applications, bank loans processing, crop harvests, self-driving vehicles, and streaming entertainment. It is now part of boardroom discussions and strategic initiatives of CEOs. McKinsey predicts AI will add USD 13 trillion to the global economy by 2030.
Hype vs Reality
So much to like – but why then do we often find leaders shrugging their shoulders? Despite all the good news above there is also another side to AI. For all the green indicators, there are also some red flags (Figure 1). In fact, if one googles “Hype vs reality” the majority of the results returned are to do with AI!!!!
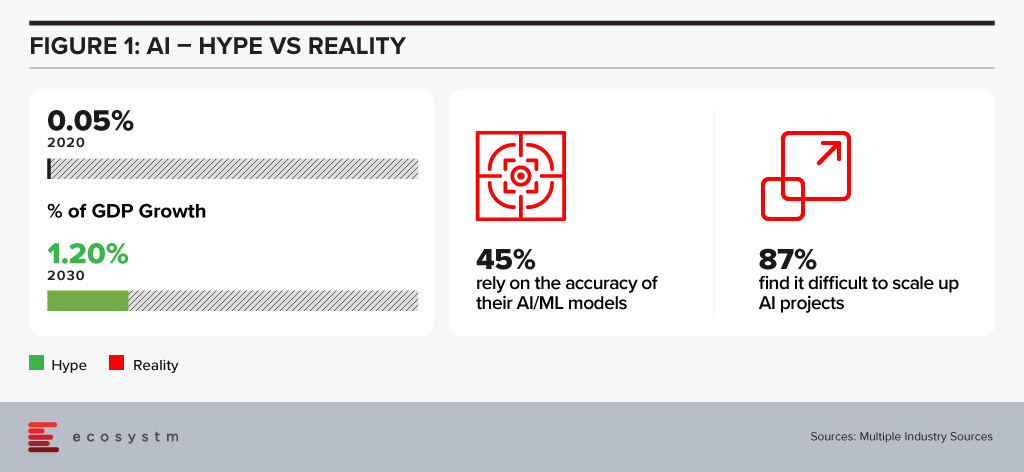
Our experience shows that broad swaths of executives are skeptical of AI. Leaders in a variety of businesses from large multinational banks, consumer packaged goods companies to appliance makers have privately expressed their disappointment at not being able to make AI work for them. They cannot bridge the gap between the AI hype and reality in their businesses.
The data available also bears this out – VentureBeat estimates that 87% of ML projects never make it into production. Ecosystm research suggests that only 7% of organisations have an AI centre of excellence (CoE) – while the remaining depend on ad-hoc implementations. There are several challenges that organisations face in procuring and implementing a successful AI solution – both technology and business (Figure 2).
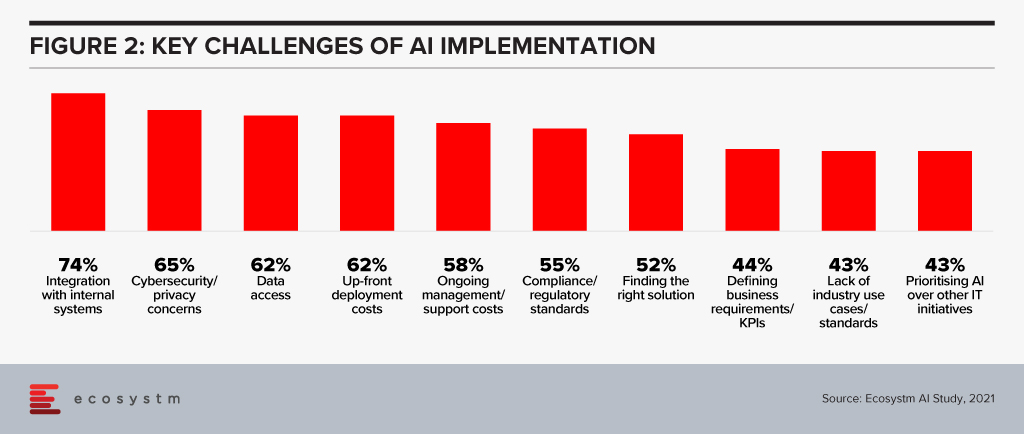
Visible Patterns Emerge from Successful AI Use Cases
This brings us to an interesting dichotomy – the reality of failed implementations versus the hype surrounding AI. Digital native companies or early adopters of AI form most of the success stories. Traditional companies find it tougher to embark on a successful AI journey. There have been studies that show a staggering gap in the ROI of AI projects between early adopters versus others, and the gulf between the high performers and the rest when using AI.
If we look back to figure 2 and analyse the challenges, we will see certain common themes – many of which are now commonplace wisdom, if not trite. Leadership alignment around AI strategy is the most common one. Getting clean data, aligning strategy with execution, and building the capabilities to use AI are all touted as critical requirements for successful execution. These themes all point to the insight that it is the human element that is more critical – not the technology.
As practitioners we have come across numerous examples of AI projects which go off-track because of human issues. Let’s take the example of an organisation that had enhancing call centre capabilities and capacity using RPA tools, as a key business mandate. There was strong leadership support and enthusiasm. It was clear that a large number of basic level tickets raised by the centre could be resolved using digital agents. This would result in substantial gains in customer experience, through faster ticket resolution and higher employee productivity – it was estimated to be above 30%. However, after two months of launching the pilot only a very small percentage of cases were identified for migration to digital agents.
Very soon, it became clear that these tools were being perceived as a replacement for human skills, rather than to augment their capabilities. The most vocal proponent of the initiative – the head of the customer experience team – became its critic, as he felt that the small savings were not worth the risk of higher agent turnover rates due to perceived job insecurity.
This was turned around by a three-day workshop focused on demonstrating how the job responsibility of agents could be enhanced as portions of their job got automated. The processes were redesigned to isolate parts which could be fully automated and to club non-automated components together driving more responsibility and discretion for agents. Once enhanced responsibility of the call centre staff was identified, managers felt more comfortable and were willing to support the initiative. In the end, the goals set at the start of the project were all met.
In my next blog I will share with you what we consider the winning formula for a successful AI deployment. In the meantime, share with us your AI stories – both of your challenges and successes.
Written with contributions from Ravi Pattamatta and Ratnesh Prasad
In this blog, our guest author Shameek Kundu talks about the importance of making AI/ machine learning models reliable and safe. “Getting data and algorithms right has always been important, particularly in regulated industries such as banking, insurance, life sciences and healthcare. But the bar is much higher now: more data, from more sources, in more formats, feeding more algorithms, with higher stakes.”
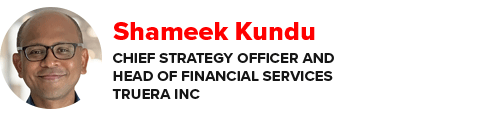
Building trust in algorithms is essential. Not (just) because regulators want it, but because it is good for customers and business. The good news is that with the right approach and tooling, it is also achievable.
Getting data and algorithms right has always been important, particularly in regulated industries such as banking, insurance, life sciences and healthcare. But the bar is much higher now: more data, from more sources, in more formats, feeding more algorithms, with higher stakes. With the increased use of Artificial Intelligence/ Machine Learning (AI/ML), today’s algorithms are also more powerful and difficult to understand.
A false dichotomy
At this point in the conversation, I get one of two reactions. One is of distrust in AI/ML and a belief that it should have little role to play in regulated industries. Another is of nonchalance; after all, most of us feel comfortable using ‘black-boxes’ (e.g., airplanes, smartphones) in our daily lives without being able to explain how they work. Why hold AI/ML to special standards?
Both make valid points. But the skeptics miss out on the very real opportunity cost of not using AI/ML – whether it is living with historical biases in human decision-making or simply not being able to do things that are too complex for a human to do, at scale. For example, the use of alternative data and AI/ML has helped bring financial services to many who have never had access before.
On the other hand, cheerleaders for unfettered use of AI/ML might be overlooking the fact that a human being (often with a limited understanding of AI/ML) is always accountable for and/ or impacted by the algorithm. And fairly or otherwise, AI/ML models do elicit concerns around their opacity – among regulators, senior managers, customers and the broader society. In many situations, ensuring that the human can understand the basis of algorithmic decisions is a necessity, not a luxury.
A way forward
Reconciling these seemingly conflicting requirements is possible. But it requires serious commitment from business and data/ analytics leaders – not (just) because regulators demand it, but because it is good for their customers and their business, and the only way to start capturing the full value from AI/ML.
1. ‘Heart’, not just ‘Head’
It is relatively easy to get people excited about experimenting with AI/ML. But when it comes to actually trusting the model to make decisions for us, we humans are likely to put up our defences. Convincing a loan approver, insurance under-writer, medical doctor or front-line sales-person to trust an AI/ML model – over their own knowledge or intuition – is as much about the ‘heart’ as the ‘head’. Helping them understand, on their own terms, how the alternative is at least as good as their current way of doing things, is crucial.
2. A Broad Church
Even in industries/ organisations that recognise the importance of governing AI/ML, there is a tendency to define it narrowly. For example, in Financial Services, one might argue that “an ML model is just another model” and expect existing Model Risk teams to deal with any incremental risks from AI/ML.
There are two issues with this approach:
First, AI/ML models tend to require a greater focus on model quality (e.g., with respect to stability, overfitting and unjust bias) than their traditional alternatives. The pace at which such models are expected to be introduced and re-calibrated is also much higher, stretching traditional model risk management approaches.
Second, poorly designed AI/ML models create second order risks. While not unique to AI/ML, these risks become accentuated due to model complexity, greater dependence on (high-volume, often non-traditional) data and ubiquitous adoption. One example is poor customer experience (e.g., badly communicated decisions) and unfair treatment (e.g., unfair denial of service, discrimination, misselling, inappropriate investment recommendations). Another is around the stability, integrity and competitiveness of financial markets (e.g., unintended collusion with other market players). Obligations under data privacy, sovereignty and security requirements could also become more challenging.
The only way to respond holistically is to bring together a broad coalition – of data managers and scientists, technologists, specialists from risk, compliance, operations and cyber-security, and business leaders.
3. Automate, Automate, Automate
A key driver for the adoption and effectiveness of AI/ ML is scalability. The techniques used to manage traditional models are often inadequate in the face of more data-hungry, widely used and rapidly refreshed AI/ML models. Whether it is during the development and testing phase, formal assessment/ validation or ongoing post-production monitoring, it is impossible to govern AI/ML at scale using manual processes alone.
o, somewhat counter-intuitively, we need more automation if we are to build and sustain trust in AI/ML. As humans are accountable for the outcomes of AI/ ML models, we can only be ‘in charge’ if we have the tools to provide us reliable intelligence on them – before and after they go into production. As the recent experience with model performance during COVID-19 suggests, maintaining trust in AI/ML models is an ongoing task.
***
I have heard people say “AI is too important to be left to the experts”. Perhaps. But I am yet to come across an AI/ML practitioner who is not keenly aware of the importance of making their models reliable and safe. What I have noticed is that they often lack suitable tools – to support them in analysing and monitoring models, and to enable conversations to build trust with stakeholders. If AI is to be adopted at scale, that must change.
Shameek Kundu is Chief Strategy Officer and Head of Financial Services at TruEra Inc. TruEra helps enterprises analyse, improve and monitor quality of machine
Have you evaluated the tech areas on your AI requirements? Get access to AI insights and key industry trends from our AI research.
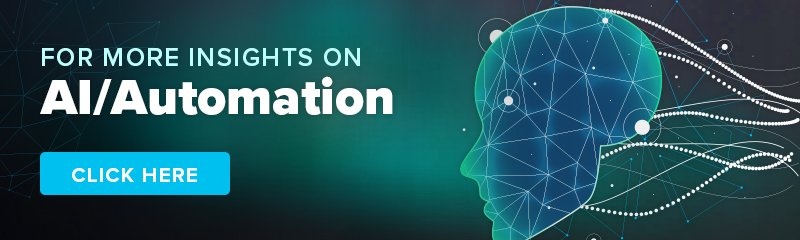