Data analysts play a vital role in today’s data-driven world, providing crucial insights that benefit decision-making processes. For those with a knack for numbers and a passion for uncovering patterns, a career as a data analyst can be both fulfilling and lucrative – it can also be a stepping stone towards other careers in data. While a data analyst focuses on data preparation and visualisation, an AI engineer specialises in creating AI solutions, a machine learning (ML) engineer concentrates on implementing ML models, and a data scientist combines elements of data analysis and ML to derive insights and predictions from data.
Tools, Skills, and Techniques of a Data Analyst
Excel Mastery. Unlocks a powerful toolbox for data manipulation and analysis. Essential skills include using a vast array of functions for calculations and data transformation. Pivot tables become your secret weapon for summarising and analysing large datasets, while charts and graphs bring your findings to life with visual clarity. Data validation ensures accuracy, and the Analysis ToolPak and Solver provide advanced functionalities for statistical analysis and complex problem-solving. Mastering Excel empowers you to transform raw data into actionable insights.
Advanced SQL. While basic skills handle simple queries, advanced users can go deeper with sorting, aggregation, and the art of JOINs to combine data from multiple tables. Common Table Expressions (CTEs) and subqueries become your allies for crafting complex queries, while aggregate functions summarise vast amounts of data. Window functions add another layer of power, allowing calculations within query results. Mastering Advanced SQL empowers you to extract hidden insights and manage data with unparalleled precision.
Data Visualisation. Crafts impactful data stories. These tools empower you to connect to various data sources, transform raw information into a usable format, and design interactive dashboards and reports. Filters and drilldowns allow users to explore your data from different angles, while calculated fields unlock deeper analysis. Parameters add a final touch of flexibility, letting viewers customise the report to their specific needs. With tools Tableau and Power BI, complex data becomes clear and engaging.
Essential Python. This powerful language excels at data analysis and automation. Libraries like NumPy and Pandas become your foundation for data manipulation and wrangling. Scikit-learn empowers you to build ML models, while SciPy and StatsModels provide a toolkit for in-depth statistical analysis. Python’s ability to interact with APIs and web scrape data expands its reach, and its automation capabilities streamline repetitive tasks. With Essential Python, you have the power to solve complex problems.
Automating the Journey. Data analysts can be masters of efficiency, and their skills translate beautifully into AI. Scripting languages like Ansible and Terraform automate repetitive tasks. Imagine streamlining the process of training and deploying AI models – a skill that directly benefits the AI development pipeline. This proficiency in automation showcases the valuable foundation data analysts provide for building and maintaining AI systems.
Developing ML Expertise. Transitioning from data analysis to AI involves building on your existing skills to develop ML expertise. As a data analyst, you may start with basic predictive models. This knowledge is expanded in AI to include deep learning and advanced ML algorithms. Also, skills in statistical analysis and visualisation help in evaluating the performance of AI models.
Growing Your AI Skills
Becoming an AI engineer requires building on a data analysis foundation to focus on advanced skills such as:
- Deep Learning. Learning frameworks like TensorFlow and PyTorch to build and train neural networks.
- Natural Language Processing (NLP). Techniques for processing and analysing large amounts of natural language data.
- AI Ethics and Fairness. Understanding the ethical implications of AI and ensuring models are fair and unbiased.
- Big Data Technologies. Using tools like Hadoop and Spark for handling large-scale data is essential for AI applications.
The Evolution of a Data Analyst: Career Opportunities
Data analysis is a springboard to AI engineering. Businesses crave talent that bridges the data-AI gap. Your data analyst skills provide the foundation (understanding data sources and transformations) to excel in AI. As you master ML, you can progress to roles like:
- AI Engineer. Works on integrating AI solutions into products and services. They work with AI frameworks like TensorFlow and PyTorch, ensuring that AI models are incorporated into products and services in a fair and unbiased manner.
- ML Engineer. Focuses on designing and implementing ML models. They focus on preprocessing data, evaluating model performance, and collaborating with data scientists and engineers to bring models into production. They need strong programming skills and experience with big data tools and ML algorithms.
- Data Scientist. Bridges the gap between data analysis and AI, often involved in both data preparation and model development. They perform exploratory data analysis, develop predictive models, and collaborate with cross-functional teams to solve complex business problems. Their role requires a comprehensive understanding of both data analysis and ML, as well as strong programming and data visualisation skills.
Conclusion
Hone your data expertise and unlock a future in AI! Mastering in-demand skills like Excel, SQL, Python, and data visualisation tools will equip you to excel as a data analyst. Your data wrangling skills will be invaluable as you explore ML and advanced algorithms. Also, your existing BI knowledge translates seamlessly into building and evaluating AI models. Remember, the data landscape is constantly evolving, so continue to learn to stay at the forefront of this dynamic field. By combining your data skills with a passion for AI, you’ll be well-positioned to tackle complex challenges and shape the future of AI.
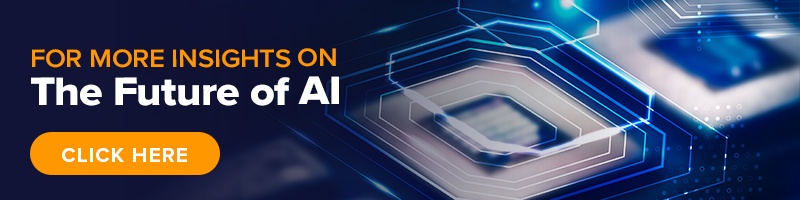
Organisations are moving beyond digitalisation to a focus on building market differentiation. It is widely acknowledged that customer-centric strategies lead to better business outcomes, including increased customer satisfaction, loyalty, competitiveness, growth, and profitability.
AI is the key enabler driving personalisation at scale. It has also become key to improving employee productivity, empowering them to focus on high-value tasks and deepening customer engagements.
Over the last month – at the Salesforce World Tour and over multiple analyst briefings – Salesforce has showcased their desire to solve customer challenges using AI innovations. They have announced a range of new AI innovations across Data Cloud, their integrated CRM platform.
Ecosystm Advisors Kaushik Ghatak, Niloy Mukherjee, Peter Carr, and Sash Mukherjee comment on Salesforce’s recent announcements and messaging.
Read on to find out more.
Download Ecosystm VendorSphere: Salesforce AI Innovations Transforming CRM as a PDF
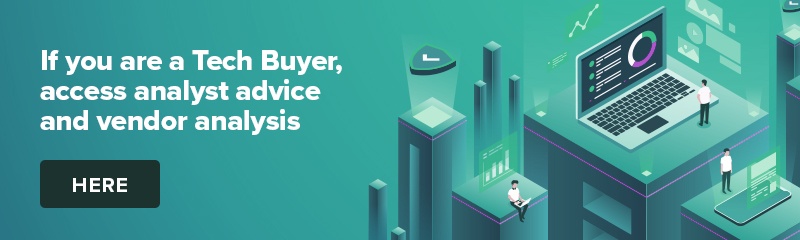
acquisition of data visualisation leader Tableau for US$15.7B. Some jaws dropped at the purchased and the price and some at the purchaser. It was inevitable that Tableau would be acquired, Oracle, IBM, or SAP could have been the suitor, but Salesforce is no surprise.
Salesforce announced on Monday, June 10th (US time) theIn all honesty, anyone who has watched Salesforce closely should neither be surprised or concerned by this acquisition. Salesforce is not merely your cloud CRM provider anymore. It has not been for years, but for some outdated perception is the reality.
Salesforce is an increasingly broad and complex enterprise software behemoth. It’s recently reported numbers highlight this. It is on track for US$20B in revenue by 2022, with year to year growth in the most recent quarterly reported numbers just shy of 25%. Sales and Service Cloud represent 60% of quarterly revenues, but the fastest growth is in the platform and increasingly new investment areas. What Salesforce does so well is to identify adjacencies to an evolving core product. The acquisition of Mulesoft in 2018 set the path to solving integration problems that challenged Salesforce deployment for customers. The purchase of Map Anything in April 2019, highlighted this adjacency approach as well as the ability of Salesforce’s ecosystem to develop partners through the AppExchange then acquire into Salesforce.
So how does Tableau fit into Salesforce?
For nearly US$16B, it had better be a precise fit. Tableau is the leader in data visualisation. It is not an analytics platform as such; one does not go to Tableau for deep statistical insight; instead, it uses it to communicate data to as broad an audience as possible. Salesforce has analytics capability as a core pillar, but this has been one of the more disappointing offerings from Salesforce and has far from reached the potential required. Salesforce will only benefit from a functionality and capability perspective with Tableau inside rather than as a partner or third-party application.
Quite simply across the product suite, and as a standalone offering, Tableau will significantly increase the visualisation, both automated and user-led capabilities of Salesforce. In terms of what it means for both companies, of course, there is good and bad. There is a very significant overlap in the customer bases of both products. It is not 100%, but there will be a balance of customer familiarity and the opportunity to cross-sell for Salesforce, and the extensive partner network that it oversees. There will be some cultural challenges, no doubt in the integration. Salesforce talks about Tableau as an independent organisation within Salesforce, and that will work until Mark Benioff believes it doesn’t. The internal but separate approach rarely works, and the Tableau logo will disappear at a point in time as a consequence.
There are a few differences between the integration of Tableau and the most comparable business Mulesoft. Mulesoft was literally up the street from Salesforce in San Francisco and culturally was based on many of the premises of Salesforce. For Seattle based Tableau, there will be a few differences culturally, although nothing that cannot be overcome with communication, honesty and much hard work on the cultural integration.
The on-premise and cloud capability of Tableau may disappear quicker than the road map that Tableau had, again, Salesforce places great import on the SaaS, no Software approach. Advanced analytics and AI capabilities of Tableau are not its fundamental value proposition so that Einstein will remain the lead there, with some added capability. The non-customer centric user of Tableau provides new client opportunities for Salesforce.
The final point of the acquisition is that it proves in 2019 and the future, you cannot be a one trick software firm. To remain relevant, you need multiple capabilities. Tableau struggled with this, VMware famously struggled until the “invention” of hybrid cloud to be more than virtualisation, and SAS Institute and ESRI remain the poster firms for relying on one old product suite.
Capture Point
Salesforce paid a premium for Tableau, even in a capital-rich 2019. In the world of Salesforce, that is rarely the point. One of the challenging aspects in the Salesforce 360 portfolio is fundamentally sharpened; it gains new users, new capabilities and opportunities for the core product to expand. As with all acquisition, the trick will be the integration, cultural alignment, and keeping developers and partners on board.
Salesforce has agreed to acquire Tableau Software Inc for US$15.3 billion in a deal that is expected to close in the third quarter of 2019. It seems all independent BI and analytics companies (except SAS!) eventually get snapped up – Business Objects by SAP, Hyperion by Oracle, Cognos by IBM. The move comes less than a week after Google acquired BI and analytics provider Looker.
In a move that feels “back to the future”,Today, many businesses use Tableau (over 86,000), including a lot of Salesforce customers. They have chosen Tableau because it is easy to deploy and use, and like Salesforce own applications, it targets the ultimate decision maker – the business user – and sometimes even the consumer. Recent research into the BI systems integrators in Asia Pacific shows that Tableau is one of the leading analytics platforms for the partner community in the region – the big SIs have many people focused on Tableau. But that dominance is being challenged by a re-energised Microsoft, whose Power BI is also witnessing strong growth – and who is typically the price leader in the market.
For Salesforce customers, there is some overlap between products – their own Einstein Analytics tools do much of what Tableau can do – although Tableau helps customers see insights from data stored both on the cloud and inside their own data centres. It also moves Salesforce closer to the Customer 360 vision – the ability to get a view of customers across the Commerce, Marketing and Service Clouds. Salesforce customers not using Tableau today will get a better user experience by using Tableau as the visualisation platform.
History has shown that it is hard to make such acquisitions successful. Tableau was a huge success because it was independent. The same was for Business Objects and Cognos before their acquisitions. History has shown that when the large BI and analytics vendors are acquired, others move into that space. While Salesforce has announced they will run Tableau as a separate business, it will no longer be independent. Partners will need to be maintained and provided a growth path – and partners are the cornerstone of Tableau’s success. Some of these partners might have strong ties to other software or cloud platforms too such as SAP, Oracle, AWS or Google. Customers of Tableau might feel sales pressure to move to a Salesforce environment – and will likely see Salesforce integration happen at a deeper level than on other platforms.
Tableau’s independence will disappear. However keeping Tableau as a separate business may not be the long term goal for Salesforce – it might be to offer the best application and analytics solution in the market – to make the entire suite more attractive to more potential buyers and users. It may be to take Salesforce beyond the current users in their customers to many other users who may not need the full application but need the analytics and visualisations that the data can provide. If this is the case, then the company is onto a winner with the Tableau acquisition.
BUT…
The long term goal is not analytics reports delivered to employees. It is not visualisation. It is automation. It is applications doing smart, AI-driven analysis, and deciding for employees. It is about taking the human out of the process. In a factory you don’t need a report to tell you a machine is down – you need to book a repair person automatically – or a service technician to visit before the machine has even broken down. And you don’t need a visualised report to show that a machine is beyond its life expectancy. You need the machine replaced before it fails catastrophically.
Too often, we are putting humans in processes where they are not required. We are making visualisations more attractive and easier to consume when, in reality, we just needed the task automated. While we employ humans, there will be a need to make decisions more effectively, and we will still require tools like Tableau. But don’t let the pretty pictures distract you from the main prize – intelligent automation.
If you would like to speak to Tim Sheedy or another analyst at Ecosystm about what the acquisition Tableau by Salesforce might mean to your business or industry, please feel free to schedule an inquiry call on the profile page.