In my last Ecosystm Insight, I spoke about the importance of data architecture in defining the data flow, data management systems required, the data processing operations, and AI applications. Data Mesh and Data Fabric are both modern architectural approaches designed to address the complexities of managing and accessing data across a large organisation. While they share some commonalities, such as improving data accessibility and governance, they differ significantly in their methodologies and focal points.
Data Mesh
- Philosophy and Focus. Data Mesh is primarily focused on the organisational and architectural approach to decentralise data ownership and governance. It treats data as a product, emphasising the importance of domain-oriented decentralised data ownership and architecture. The core principles of Data Mesh include domain-oriented decentralised data ownership, data as a product, self-serve data infrastructure as a platform, and federated computational governance.
- Implementation. In a Data Mesh, data is managed and owned by domain-specific teams who are responsible for their data products from end to end. This includes ensuring data quality, accessibility, and security. The aim is to enable these teams to provide and consume data as products, improving agility and innovation.
- Use Cases. Data Mesh is particularly effective in large, complex organisations with many independent teams and departments. It’s beneficial when there’s a need for agility and rapid innovation within specific domains or when the centralisation of data management has become a bottleneck.
Data Fabric
- Philosophy and Focus. Data Fabric focuses on creating a unified, integrated layer of data and connectivity across an organisation. It leverages metadata, advanced analytics, and AI to improve data discovery, governance, and integration. Data Fabric aims to provide a comprehensive and coherent data environment that supports a wide range of data management tasks across various platforms and locations.
- Implementation. Data Fabric typically uses advanced tools to automate data discovery, governance, and integration tasks. It creates a seamless environment where data can be easily accessed and shared, regardless of where it resides or what format it is in. This approach relies heavily on metadata to enable intelligent and automated data management practices.
- Use Cases. Data Fabric is ideal for organisations that need to manage large volumes of data across multiple systems and platforms. It is particularly useful for enhancing data accessibility, reducing integration complexity, and supporting data governance at scale. Data Fabric can benefit environments where there’s a need for real-time data access and analysis across diverse data sources.
Both approaches aim to overcome the challenges of data silos and improve data accessibility, but they do so through different methodologies and with different priorities.
Data Mesh and Data Fabric Vendors
The concepts of Data Mesh and Data Fabric are supported by various vendors, each offering tools and platforms designed to facilitate the implementation of these architectures. Here’s an overview of some key players in both spaces:
Data Mesh Vendors
Data Mesh is more of a conceptual approach than a product-specific solution, focusing on organisational structure and data decentralisation. However, several vendors offer tools and platforms that support the principles of Data Mesh, such as domain-driven design, product thinking for data, and self-serve data infrastructure:
- Thoughtworks. As the originator of the Data Mesh concept, Thoughtworks provides consultancy and implementation services to help organisations adopt Data Mesh principles.
- Starburst. Starburst offers a distributed SQL query engine (Starburst Galaxy) that allows querying data across various sources, aligning with the Data Mesh principle of domain-oriented, decentralised data ownership.
- Databricks. Databricks provides a unified analytics platform that supports collaborative data science and analytics, which can be leveraged to build domain-oriented data products in a Data Mesh architecture.
- Snowflake. With its Data Cloud, Snowflake facilitates data sharing and collaboration across organisational boundaries, supporting the Data Mesh approach to data product thinking.
- Collibra. Collibra provides a data intelligence cloud that offers data governance, cataloguing, and privacy management tools essential for the Data Mesh approach. By enabling better data discovery, quality, and policy management, Collibra supports the governance aspect of Data Mesh.
Data Fabric Vendors
Data Fabric solutions often come as more integrated products or platforms, focusing on data integration, management, and governance across a diverse set of systems and environments:
- Informatica. The Informatica Intelligent Data Management Cloud includes features for data integration, quality, governance, and metadata management that are core to a Data Fabric strategy.
- Talend. Talend provides data integration and integrity solutions with strong capabilities in real-time data collection and governance, supporting the automated and comprehensive approach of Data Fabric.
- IBM. IBM’s watsonx.data is a fully integrated data and AI platform that automates the lifecycle of data across multiple clouds and systems, embodying the Data Fabric approach to making data easily accessible and governed.
- TIBCO. TIBCO offers a range of products, including TIBCO Data Virtualization and TIBCO EBX, that support the creation of a Data Fabric by enabling comprehensive data management, integration, and governance.
- NetApp. NetApp has a suite of cloud data services that provide a simple and consistent way to integrate and deliver data across cloud and on-premises environments. NetApp’s Data Fabric is designed to enhance data control, protection, and freedom.
The choice of vendor or tool for either Data Mesh or Data Fabric should be guided by the specific needs, existing technology stack, and strategic goals of the organisation. Many vendors provide a range of capabilities that can support different aspects of both architectures, and the best solution often involves a combination of tools and platforms. Additionally, the technology landscape is rapidly evolving, so it’s wise to stay updated on the latest offerings and how they align with the organisation’s data strategy.
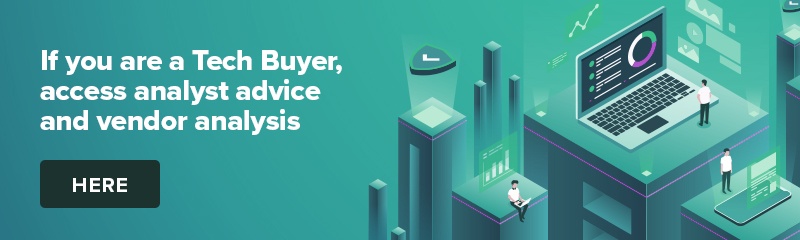
In my last Ecosystm Insights, I spoke about why organisations need to think about the Voice of the Customer (VoC) quite literally. Organisations need to listen to what their customers are telling them – not just to the survey questions they responded to, answering pre-defined questions that the organisations want to hear about.
The concept of customer feedback is evolving, and how organisations design and manage VoC programs must also change. Technology is now capable of enabling customer teams to tap into all those unsolicited, and often unstructured, raw feedback sources. Think contact centre conversations (calls, chats, chatbots, emails, complaints, call notes), CRM notes, online reviews, social media, etc. Those are all sources of raw customer feedback, waiting to be converted into customer insights.
Organisations can now find the capability of extracting customer insight from raw data across a wide range of solutions, from VoC platforms, data management platforms, contact centre solutions, text analytics players, etc. The expanding tech ecosystem presents opportunities for organisations to enhance their programs. However, navigating this breadth of options can also be confusing as they strive to identify the most suitable tools for their requirements.
As CX programs mature and shift from survey feedback to truly listening to customers, the demand for tech solutions tailored to various needs increases.
Where are tech vendors headed?
As part of my job as CX Consultant & Tech Advisor, I spend a lot of time working with my clients. But I also spend a lot of time speaking with technology vendors, who provide the solutions my clients need. Over the last few weeks and months there’s been a flurry of activity across the CX technology market with lots of product announcements around one specific topic. You guessed it, GenAI.
So, I invested some time in finding out how tech vendors are evolving their offerings. From Medallia, InMoment, Thematic, LiquidVoice, Concentrix, Snowflake, Nice, to Tethr – a broad variety of different vendors, but all with one thing in common; they help analyse customer feedback data.
And I like what I hear. The conversation has not been about GenAI because of GenAI, but about use cases and real-life applications for CX practitioners, including Insights & Research team, Contact Centre, CX, VoC, Digital teams, and so on. The list is long when we include everyone who has a role to play in creating, maintaining, and improving customer experiences.
It’s no wonder that many different vendors have started to embed those capabilities into their solutions and launch new products or features. The tech landscape is becoming increasingly fragmented at this stage.
What are an organisation’s tech options?
- The traditional VoC platform providers typically offer some text analytics capabilities (although not always included in the base price) and have started to tap into the contact centre solutions as well. Some also offer some social media or online review analysis, leaving organisations with a relatively good understanding of customer sentiment and a better understanding of their CX.
- Contact centre solutions are traditionally focused on analysing calls for Quality Assurance (QA) purposes and use surveys for agent coaching. Many contact centre players have evolved their portfolios to include text analytics or conversational intelligence to extract broader customer insights. Although at this stage they’re not always shared with the rest of the organisation (one step at a time…).
- Conversational analytics/intelligence providers have emerged over the last few years and are a powerhouse for contact centre and chatbot conversations. The contact centre really is the treasure trove of customer insights, although vastly underutilised for it so far!
- CRMs are the backbone of the customer experience management toolkit as they hold a vast amount of metadata. They’ve also been able to send surveys for a while now. Analysing unstructured data however (whether survey verbatim or otherwise) isn’t one of their strengths. This leaves organisations with a lot of data but not necessarily insights.
- Social media listening tools are often standalone tools used by the social media teams. There are not many instances of them being used for the analysis of other unstructured feedback.
- Digital/website feedback tools, in line with some of the above, are centred around collecting feedback, not necessarily analysing the unstructured feedback.
- Pure text analytics players are traditionally focused on analysing surveys verbatim. As this is their core offering, they tend to be proficient in it and have started to broaden their portfolios to include other unstructured feedback sources.
- Customer Data Platforms (CDP)/ Data Management Platforms (DMP) are more focused on quantitative data about customers and their experiences. Although many speak about their ability to analyse unstructured feedback as well, it doesn’t appear to be their strengths.
Conclusion
But what does that leave organisations with? Apart from very confused tech users trying to find the right solution for their organisation.
At this stage, there is immense market fragmentation, with many vendors from different core capabilities starting to incorporate capabilities to analyse unstructured data in the wake of the GenAI boom. However, a market convergence is expected.
While we watch how the market unfolds, one thing is certain. Organisations and customer teams will need to adjust – and that includes the tech stack as well as the CX program set up. With customer feedback now coming from anywhere within or outside the organisation, there is a need for a consolidated source of truth to make sense of it all and move from raw data to customer insights. While organisations will benefit immensely from a consolidated customer data repository, it’s also crucial to break down organisational silos at the same time and democratise insights as widely as possible to enable informed decision-making.
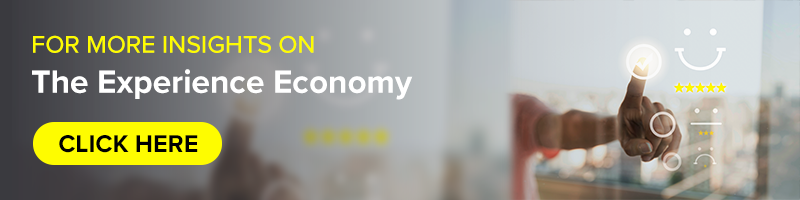