The challenge of AI is that it is hard to build a business case when the outcomes are inherently uncertain. Unlike a traditional process improvement procedure, there are few guarantees that AI will solve the problem it is meant to solve. Organisations that have been experimenting with AI for some time are aware of this, and have begun to formalise their Proof of Concept (PoC) process to make it easily repeatable by anyone in the organisation who has a use case for AI. PoCs can validate assumptions, demonstrate the feasibility of an idea, and rally stakeholders behind the project.
PoCs are particularly useful at a time when AI is experiencing both heightened visibility and increased scrutiny. Boards, senior management, risk, legal and cybersecurity professionals are all scrutinising AI initiatives more closely to ensure they do not put the organisation at risk of breaking laws and regulations or damaging customer or supplier relationships.
13 Steps to Building an AI PoC
Despite seeming to be lightweight and easy to implement, a good PoC is actually methodologically sound and consistent in its approach. To implement a PoC for AI initiatives, organisations need to:
- Clearly define the problem. Businesses need to understand and clearly articulate the problem they want AI to solve. Is it about improving customer service, automating manual processes, enhancing product recommendations, or predicting machinery failure?
- Set clear objectives. What will success look like for the PoC? Is it about demonstrating technical feasibility, showing business value, or both? Set tangible metrics to evaluate the success of the PoC.
- Limit the scope. PoCs should be time-bound and narrow in scope. Instead of trying to tackle a broad problem, focus on a specific use case or a subset of data.
- Choose the right data. AI is heavily dependent on data. For a PoC, select a representative dataset that’s large enough to provide meaningful results but manageable within the constraints of the PoC.
- Build a multidisciplinary team. Involve team members from IT, data science, business units, and other relevant stakeholders. Their combined perspectives will ensure both technical and business feasibility.
- Prioritise speed over perfection. Use available tools and platforms to expedite the development process. It’s more important to quickly test assumptions than to build a highly polished solution.
- Document assumptions and limitations. Clearly state any assumptions made during the PoC, as well as known limitations. This helps set expectations and can guide future work.
- Present results clearly. Once the PoC is complete, create a clear and concise report or presentation that showcases the results, methodologies, and potential implications for the business.
- Get feedback. Allow stakeholders to provide feedback on the PoC. This includes end-users, technical teams, and business leaders. Their insights will help refine the approach and guide future iterations.
- Plan for the next steps. What actions need to follow a successful PoC demonstration? This might involve a pilot project with a larger scope, integrating the AI solution into existing systems, or scaling the solution across the organisation.
- Assess costs and ROI. Evaluate the costs associated with scaling the solution and compare it with the anticipated ROI. This will be crucial for securing budget and support for further expansion.
- Continually learn and iterate. AI is an evolving field. Use the PoC as a learning experience and be prepared to continually iterate on your solutions as technologies and business needs evolve.
- Consider ethical and social implications. Ensure that the AI initiative respects privacy, reduces bias, and upholds the ethical standards of the organisation. This is critical for building trust and ensuring long-term success.
Customising AI for Your Business
The primary purpose of a PoC is to validate an idea quickly and with minimal risk. It should provide a clear path for decision-makers to either proceed with a more comprehensive implementation or to pivot and explore alternative solutions. It is important for the legal, risk and cybersecurity teams to be aware of the outcomes and support further implementation.
AI initiatives will inevitably drive significant productivity and customer experience improvements – but not every solution will be right for the business. At Ecosystm, we have come across organisations that have employed conversational AI in their contact centres to achieve entirely distinct results – so the AI experience of peers and competitors may not be relevant. A consistent PoC process that trains business and technology teams across the organisation and encourages experimentation at every possible opportunity, would be far more useful.
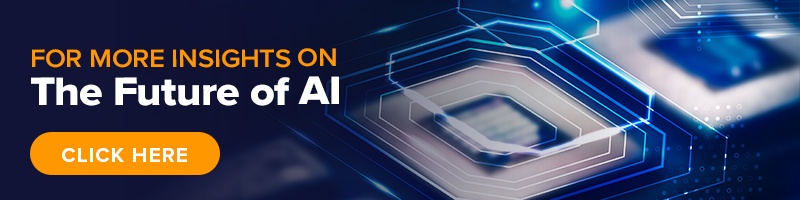