In my last Ecosystm Insights, I spoke about the implications of the shift from Predictive AI to Generative AI on ROI considerations of AI deployments. However, from my discussions with colleagues and technology leaders it became clear that there is a need to define and distinguish between Predictive AI and Generative AI better.
Predictive AI analyses historical data to predict future outcomes, crucial for informed decision-making and strategic planning. Generative AI unlocks new avenues for innovation by creating novel data and content. Organisations need both – Predictive AI for enhancing operational efficiencies and forecasting capabilities and Generative AI to drive innovation; create new products, services, and experiences; and solve complex problems in unprecedented ways.
This guide aims to demystify these categories, providing clarity on their differences, applications, and examples of the algorithms they use.
Predictive AI: Forecasting the Future
Predictive AI is extensively used in fields such as finance, marketing, healthcare and more. The core idea is to identify patterns or trends in data that can inform future decisions. Predictive AI relies on statistical, machine learning, and deep learning models to forecast outcomes.
Key Algorithms in Predictive AI
- Regression Analysis. Linear and logistic regression are foundational tools for predicting a continuous or categorical outcome based on one or more predictor variables.
- Decision Trees. These models use a tree-like graph of decisions and their possible consequences, including chance event outcomes, resource costs and utility.
- Random Forest (RF). An ensemble learning method that operates by constructing a multitude of decision trees at training time to improve predictive accuracy and control over-fitting.
- Gradient Boosting Machines (GBM). Another ensemble technique that builds models sequentially, each new model correcting errors made by the previous ones, used for both regression and classification tasks.
- Support Vector Machines (SVM). A supervised machine learning model that uses classification algorithms for two-group classification problems.
Generative AI: Creating New Data
Generative AI, on the other hand, focuses on generating new data that is similar but not identical to the data it has been trained on. This can include anything from images, text, and videos to synthetic data for training other AI models. GenAI is particularly known for its ability to innovate, create, and simulate in ways that predictive AI cannot.
Key Algorithms in Generative AI
- Generative Adversarial Networks (GANs). Comprising two networks – a generator and a discriminator – GANs are trained to generate new data with the same statistics as the training set.
- Variational Autoencoders (VAEs). These are generative algorithms that use neural networks for encoding inputs into a latent space representation, then reconstructing the input data based on this representation.
- Transformer Models. Originally designed for natural language processing (NLP) tasks, transformers can be adapted for generative purposes, as seen in models like GPT (Generative Pre-trained Transformer), which can generate coherent and contextually relevant text based on a given prompt.
Comparing Predictive and Generative AI
The fundamental difference between the two lies in their primary objectives: Predictive AI aims to forecast future outcomes based on past data, while Generative AI aims to create new, original data that mimics the input data in some form.
The differences become clearer when we look at these examples.
Predictive AI Examples
- Supply Chain Management. Analyses historical supply chain data to forecast demand, manage inventory levels, reduces costs and improve delivery times.
- Healthcare. Analysing patient records to predict disease outbreaks or the likelihood of a disease in individual patients.
- Predictive Maintenance. Analyse historical and real-time data and preemptively identifies system failures or network issues, enhancing infrastructure reliability and operational efficiency.
- Finance. Using historical stock prices and indicators to predict future market trends.
Generative AI Examples
- Content Creation. Generating realistic images or art from textual descriptions using GANs.
- Text Generation. Creating coherent and contextually relevant articles, stories, or conversational responses using transformer models like GPT-3.
- Chatbots and Virtual Assistants. Advanced GenAI models are enhancing chatbots and virtual assistants, making them more realistic.
- Automated Code Generation. By the use of natural language descriptions to generate programming code and scripts, to significantly speed up software development processes.
Conclusion
Organisations that exclusively focus on Generative AI may find themselves at the forefront of innovation, by leveraging its ability to create new content, simulate scenarios, and generate original data. However, solely relying on Generative AI without integrating Predictive AI’s capabilities may limit an organisation’s ability to make data-driven decisions and forecasts based on historical data. This could lead to missed opportunities to optimise operations, mitigate risks, and accurately plan for future trends and demands. Predictive AI’s strength lies in analysing past and present data to inform strategic decision-making, crucial for long-term sustainability and operational efficiency.
It is essential for companies to adopt a dual-strategy approach in their AI efforts. Together, these AI paradigms can significantly amplify an organisation’s ability to adapt, innovate, and compete in rapidly changing markets.
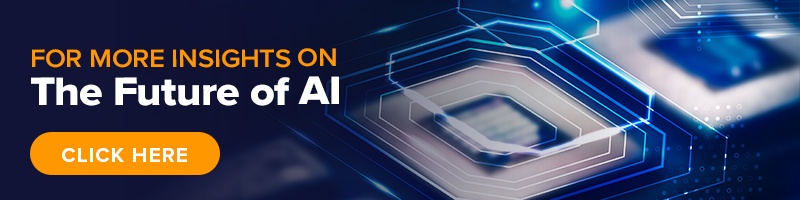
The AI landscape is undergoing a significant transformation, moving from traditional predictive AI use cases towards Generative AI (GenAI). Currently, most GenAI use cases promise an improvement in employee productivity, without focusing on how to leverage this into new or additional revenue generating streams. This raises concerns about the long-term return on investment (ROI) if this is not adequately addressed.
The Rise of Generative AI Over Predictive AI
Traditionally, predictive AI has been integral to business strategies, leveraging data to forecast future outcomes with remarkable accuracy. Industries across the board have used predictive models for a range of applications, from demand forecasting in retail to fraud detection in finance. However, the tide is changing with the emergence of GenAI technologies. GenAI, capable of creating content, designing products, and even coding, holds the promise to revolutionise how businesses operate, innovate, and compete.
The appeal of GenAI lies in its versatility and creativity, offering solutions that go beyond the capabilities of predictive models. For example, in the area of content creation, GenAI can produce written content, images, and videos at scale, potentially transforming marketing, entertainment, and education sectors. However, the current enthusiasm for GenAI’s productivity enhancements overshadows a critical aspect of technology adoption: monetisation.
The Productivity Paradox
While the emphasis on productivity improvements through GenAI applications is undoubtedly beneficial, there is a notable gap in exploring use cases that directly contribute to creating new revenue streams. This productivity paradox – prioritising operational efficiency and cost reduction – may not guarantee the sustained growth and ROI necessary from AI investments.
True innovation in AI should not only aim at making existing processes more efficient but also at uncovering opportunities for monetisation. This involves leveraging GenAI to develop new products, services, or business models to access untapped markets or enhance customer value in ways that directly impact the bottom line.
The Imperative for Strategic Reorientation
Ignoring the monetisation aspect of GenAI applications poses a significant risk to the anticipated ROI from AI investments. As businesses allocate resources to AI adoption and integration, it’s also important to consider how these technologies can generate revenue, not just save costs. Without a clear path to monetisation, the investments in AI, particularly in the cutting-edge domain of GenAI, may not prove viable in the next financial year and beyond.
To mitigate this risk, companies need to adopt a dual approach. First, they must continue to explore and exploit the productivity gains offered by GenAI, which are crucial for maintaining a competitive edge and achieving operational excellence. At the same time, businesses must strategically explore and invest in GenAI-driven opportunities for monetisation. This could mean innovating in product design, personalised customer experiences, or entirely new business models that were previously unfeasible.
Conclusion
The excitement around GenAI’s potential to transform industries is well-founded, but it must be tempered with strategic planning to ensure long-term viability and ROI. Businesses that recognise and act on the opportunity to not only improve productivity but also to monetise GenAI innovations will lead the next wave of growth in their respective sectors. The challenge lies in balancing the drive for efficiency with the pursuit of new revenue streams, ensuring that investments in AI deliver sustainable returns. As the AI landscape evolves, the ability to innovate in monetisation as much as in technology will distinguish the leaders from the followers.
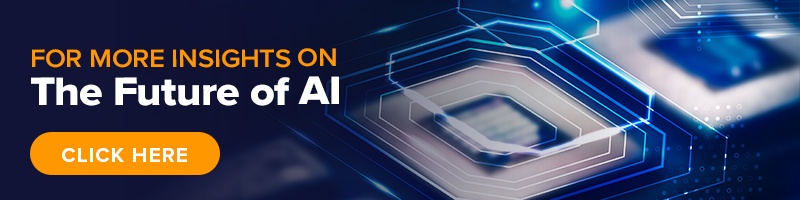