Traditional network architectures are inherently fragile, often relying on a single transport type to connect branches, production facilities, and data centres. The imperative for networks to maintain resilience has grown significantly, particularly due to the delivery of customer-facing services at branches and the increasing reliance on interconnected machines in operational environments. The cost of network downtime can now be quantified in terms of both lost customers and reduced production.
Distributed Enterprises Face New Challenges
As the importance of maintaining resiliency grows, so does the complexity of network management. Distributed enterprises must provide connectivity under challenging conditions, such as:
- Remote access for employees using video conferencing
- Local breakout for cloud services to avoid backhauling
- IoT devices left unattended in public places
- Customers accessing digital services at the branch or home
- Sites in remote areas requiring the same quality of service
Network managers require intelligent tools to remain in control without adding any unnecessary burden to end users. The number of endpoints and speed of change has made it impossible for human operators to manage without assistance from AI.
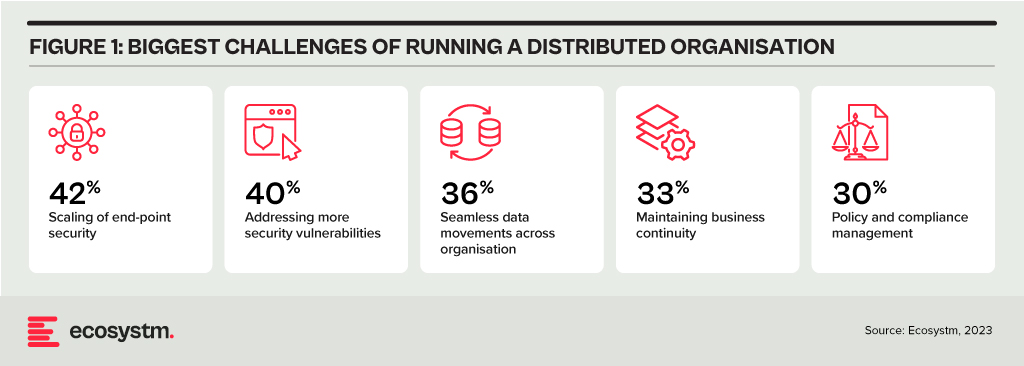
AI-Enhanced Network Management
Modern network operations centres are enhancing their visibility by aggregating data from diverse systems and consolidating them within a unified management platform. Machine learning (ML) and AI are employed to analyse data originating from enterprise networks, telecom Points of Presence (PoPs), IoT devices, cloud service providers, and user experience monitoring. These technologies enable the early identification of network issues before they reach critical levels. Intelligent networks can suggest strategies to enhance network resilience, forecast how modifications may impact performance, and are increasingly capable of autonomous responses to evolving conditions.
Here are some critical ways that AI/ML can help build resilient networks.
- Alert Noise Reduction. Network operations centres face thousands of alerts each day. As a result, operators battle with alert fatigue and are challenged to identify critical issues. Through the application of ML, contemporary monitoring tools can mitigate false positives, categorise interconnected alerts, and assist operators in prioritising the most pressing concerns. An operations team, augmented with AI capabilities could potentially de-prioritise up to 90% of alerts, allowing a concentrated focus on factors that impact network performance and resilience.
- Data Lakes. Networking vendors are building their own proprietary data lakes built upon telemetry data generated by the infrastructure they have deployed at customer sites. This vast volume of data allows them to use ML to create a tailored baseline for each customer and to recommend actions to optimise the environment.
- Root Cause Analysis. To assist network operators in diagnosing an issue, AIOps can sift through thousands of data points and correlate them to identify a root cause. Through the integration of alerts with change feeds, operators can understand the underlying causes of network problems or outages. By using ML to understand the customer’s unique environment, AIOps can progressively accelerate time to resolution.
- Proactive Response. As management layers become capable of recommending corrective action, proactive response also becomes possible, leading to self-healing networks. With early identification of sub-optimal conditions, intelligent systems can conduct load balancing, redirect traffic to higher performing SaaS regions, auto-scale cloud instances, or terminate selected connections.
- Device Profiling. In a BYOD environment, network managers require enhanced visibility to discover devices and enforce appropriate policies on them. Automated profiling against a validated database ensures guest access can be granted without adding friction to the onboarding process. With deep packet inspection, devices can be precisely classified based on behaviour patterns.
- Dynamic Bandwidth Aggregation. A key feature of an SD-WAN is that it can incorporate diverse transport types, such as fibre, 5G, and low earth orbit (LEO) satellite connectivity. Rather than using a simple primary and redundant architecture, bandwidth aggregation allows all circuits to be used simultaneously. By infusing intelligence into the SD-WAN layer, the process of path selection can dynamically prioritise traffic by directing it over higher quality or across multiple links. This approach guarantees optimal performance, even in the face of network degradation.
- Generative AI for Process Efficiency. Every tech company is trying to understand how they can leverage the power of Generative AI, and networking providers are no different. The most immediate use case will be to improve satisfaction and scalability for level 1 and level 2 support. A Generative AI-enabled service desk could provide uninterrupted support during high-volume periods, such as during network outages, or during off-peak hours.
Initiating an AI-Driven Network Management Journey
Network managers who take advantage of AI can build highly resilient networks that maximise uptime, deliver consistently high performance, and remain secure. Some important considerations when getting started include:
- Data Catalogue. Take stock of the data sources that are available to you, whether they come from network equipment telemetry, applications, or the data lake of a managed services provider. Understand how they can be integrated into an AIOps solution.
- Start Small. Begin with a pilot in an area where good data sources are available. This will help you assess the impact that AI could have on reducing alerts, improving mean time to repair (MTTR), increasing uptime, or addressing the skills gap.
- Develop an SD-WAN/SASE Roadmap. Many advanced AI benefits are built into an SD-WAN or SASE. Most organisations already have or will soon adopt SD-WAN but begin assessing the SASE framework to decide if it is suitable for your organisation.
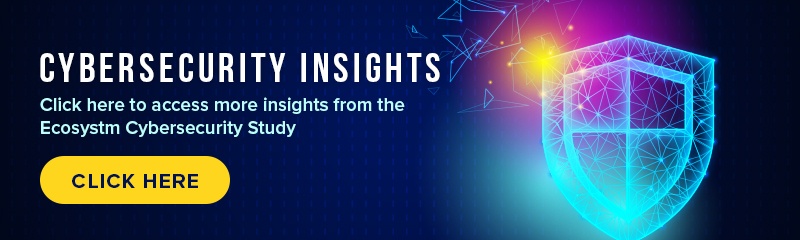
The opportunities that can be created by 5G continue to excite businesses and consumers alike. As 5G rollouts gather pace, new consumer experiences and business models emerge. For consumers, enhanced mobile broadband offers superior experience, driving the consumption of much more data-rich content and the more widespread application of emerging technologies such as augmented reality (AR). For businesses, the low latency, higher bandwidth, and the ability to handle massive machine type communications promised by 5G create opportunities for a dizzying array of uses cases, usually linked to IoT technology.
As enterprise use cases like autonomous driving, remote surgery and software-defined factories are enabled by 5G, the impact of cybersecurity breaches becomes much greater. Breaches can potentially have a catastrophic impact – they could lead to serious damage to or the destruction of sensitive critical infrastructures, such as power stations and transportation systems.
Security vulnerabilities associated with 5G are underpinned by a change in network architecture. The latency benefits of 5G require a more distributed architecture to enable use cases which require real-time data processing. This leads to the virtualisation of higher-level network functions formerly performed by physical appliances. So 5G networks will necessarily create a greatly expanded attack surface. If an attacker gains control of the software managing the networks, they can also control the network and potentially cause chaos.
One of the major benefits of 5G is massively increased bandwidth. This is also a huge benefit for attackers. An increase in available bandwidth makes it much easier to generate attack traffic from compromised connected devices and vulnerable networks. As volumetric DDoS attacks grow in terms of frequency, magnitude, and sophistication, traditional defences such as out-of-band scrubbing centres and manual interventions become inadequate and expensive.
In a 5G World, Security Postures must be Agile and not Act as a Bottleneck to Performance
5G use cases require a radical shift in cybersecurity posture and a new set of security considerations. Networks managed by enterprises and service providers need to scale up to handle larger capacity requirements and scale out to accommodate the increased demands of edge computing and the growing volumes of IoT endpoints. Security infrastructure must change accordingly with upgrades to both physical and virtual components. Importantly, security postures must also be sufficiently agile to change with new requirements while ensuring that security does not act as a bottleneck to network performance.
A common response to the increasing complexity of distributed cloud and IoT environments – where existing tools cannot always detect new and emerging threats – is to deploy brand new security tools. This seems like a great solution but can lead to significant problems and compromise security. Over time, the deployment of multiple security tools creates an estate of siloed security products, sometimes reporting to their own dashboards. Although this management challenge is typically being addressed by service providers and large enterprises, most commonly with SIEM, they must continually ensure that there is provision for the centralisation of security alerts, so that cybersecurity staff do not face the challenge of monitoring multiple consoles and cross-referencing between disparate screens and information formats. Applying security policy changes is a laborious and time-consuming task in a multi-dashboard environment – representing a security threat in its own right.
In the case of large volumetric attacks, redirecting suspicious traffic to scrubbing centres adds latency and imposes a significant financial burden, since mitigation costs are directly tied to the volume of the data traffic. Large enterprises and service providers should consider adopting new DDoS protection approaches that incorporate AI, real-time analysis, and telemetry to automate a more intelligent and cost-effective detection and mitigation process.
Different Policies Required to Reflect Specific Needs of Each Use Case
5G allows mobile service providers to partition their network resources, to address a diverse set of use cases with differing performance and functional requirements. These varying service performance profiles have a direct impact on security protocol choices and policy implementation. For instance, the service in one use case, such as a Smart City application, may require extremely long device battery life, which constrains the security protocol in some other way (e.g., how often re-authentication is performed). In another example, the use case may be very privacy-sensitive, requiring unusually intensive security procedures (e.g., very frequent reallocation of temporary identities).
The complexity associated with securing highly distributed and virtualised networks powered by 5G, will grow enormously and be hampered by an ever-increasing skills shortage. The only way to address these challenges is to create an intelligent security infrastructure that is sufficiently agile to scale with the network and use AI to detect, contain and eliminate threats. Security managers will need a unified view of all assets – physical and virtual – so that multiple security policies can be enforced and managed.