The rollout of 5G combined with edge computing in remote locations will change the way maintenance is carried out in the field. Historically, service teams performed maintenance either in a reactive fashion – fixing equipment when it broke – or using a preventative calendar-based approach. Neither of these methods is satisfactory, with the former being too late and resulting in failure while the latter is necessarily too early, resulting in excessive expenditure and downtime. The availability of connected sensors has allowed service teams to shift to condition monitoring without the need for taking equipment offline for inspections. The advent of analytics takes this approach further and has given us optimised scheduling in the form of predictive maintenance.
The next step is prescriptive maintenance in which AI can recommend action based on current and predicted condition according to expected usage or environmental circumstances. This could be as simple as alerting an operator to automatically ordering parts and scheduling multiple servicing tasks depending on forecasted production needs in the short term.
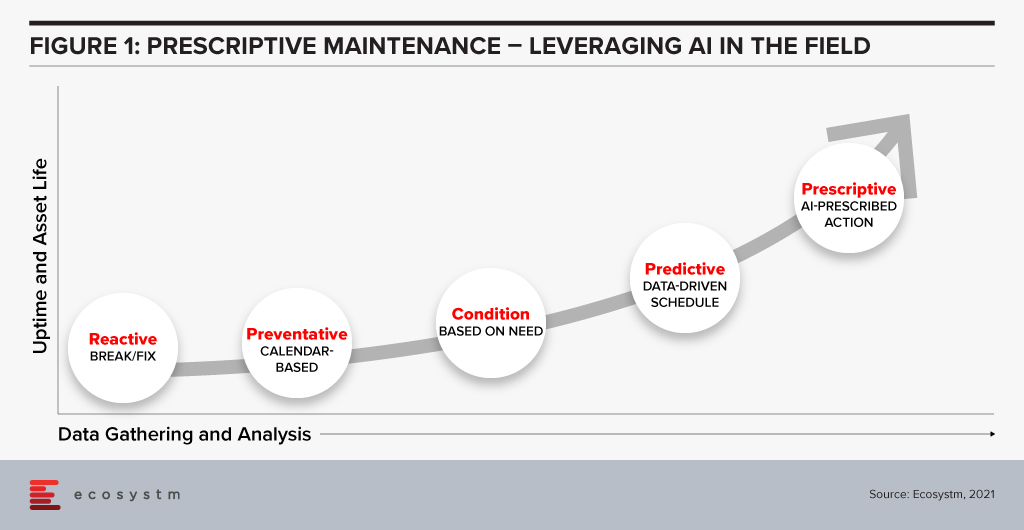
Prescriptive maintenance has only become possible with the advancement of AI and digital twin technology, but imminent improvements in connectivity and computing will take servicing to a new level. The rollout of 5G will give a boost to bandwidth, reduce latency, and increase the number of connections possible. Equipment in remote locations – such as transmission lines or machinery in resource industries – will benefit from the higher throughput of 5G connectivity, either as part of an operator’s network rollout or a private on-site deployment. Mobile machinery, particularly vehicles, which can include hundreds of sensors will no longer be required to wait until arrival before the condition can be assessed. Furthermore, vehicles equipped with external sensors can inspect stationary infrastructure as it passes by.
Edge computing – either carried out by miniature onboard devices or at smaller scale data centres embedded in 5G networks – ensure that intensive processing can be carried out closer to equipment than with a typical cloud environment. Bandwidth hungry applications, such as video and time series analysis, can be conducted with only meta data transmitted immediately and full archives uploaded with less urgency.
Prescriptive Maintenance with 5G and the Edge – Use Cases
- Transportation. Bridges built over railway lines equipped with high-speed cameras can monitor passing trains to inspect for damage. Data-intensive video analysis can be conducted on local devices for a rapid response while selected raw data can be uploaded to the cloud over 5G to improve inference models.
- Mining. Private 5G networks built-in remote sites can provide connectivity between fixed equipment, vehicles, drones, robotic dogs, workers, and remote operations centres. Autonomous haulage trucks can be monitored remotely and in the event of a breakdown, other vehicles can be automatically redirected to prevent dumping queues.
- Utilities. Emergency maintenance needs can be prioritised before extreme weather events based on meteorological forecasts and their impact on ageing parts. Machine learning can be used to understand location-specific effects of, for example, salt content in off-shore wind turbine cables. Early detection of turbine rotor cracks can recommend shutdown during high-load periods.

Data as an Asset
Effective prescriptive maintenance only becomes possible after the accumulation and integration of multiple data sources over an extended period. Inference models should understand both normal and abnormal equipment performance in various conditions, such as extreme weather, during incorrect operation, or when adjacent parts are degraded. For many smaller organisations or those deploying new equipment, the necessary volume of data will not be available without the assistance of equipment manufacturers. Moreover, even manufacturers will not have sufficient data on interaction with complementary equipment. This provides an opportunity for large operators to sell their own inference models as a new revenue stream. For example, an electrical grid operator in North America can partner with a similar, but smaller organisation in Europe to provide operational data and maintenance recommendations. Similarly, telecom providers, regional transportation providers, logistics companies, and smart cities will find industry players in other geographies that they do not naturally compete with.
Recommendations
- Employing multiple sensors. Baseline conditions and failure signatures are improved using machine learning based on feeds from multiple sensors, such as those that monitor vibration, sound, temperature, pressure, and humidity. The use of multiple sensors makes it possible to not only identify potential failure but also the reason for it and can therefore more accurately prescribe a solution to prevent an outage.
- Data assessment and integration. Prescriptive maintenance is most effective when multiple data sources are unified as inputs. Identify the location of these sources, such as ERP systems, time series on site, environmental data provided externally, or even in emails or on paper. A data fabric should be considered to ensure insights can be extracted from data no matter the environment it resides in.
- Automated action. Reduce the potential for human error or delay by automatically generating alerts and work orders for resource managers and service staff in the event of anomaly detection. Criticality measures should be adopted to help prioritise maintenance tasks and reduce alert noise.
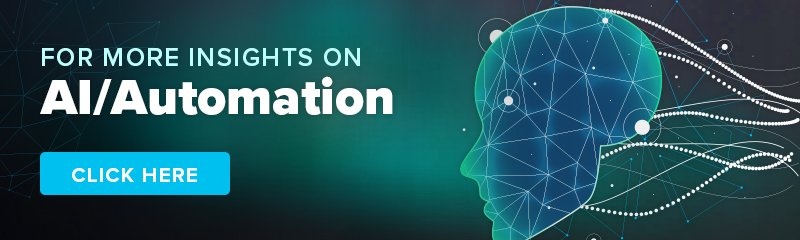
BHP – the multinational mining giant – has signed agreements with AWS and Microsoft Azure as their long-term cloud providers to support their digital transformation journey. This move is expected to accelerate BHP’s cloud journey, helping them deploy and scale their digital operations to the workforce quickly while reducing the need for on-premises infrastructure.
Ecosystm research has consistently shown that many large organisations are using the learnings from how the COVID-19 pandemic impacted their business to re-evaluate their Digital Transformation strategy – leveraging next generation cloud, machine learning and data analytics capabilities.
BHP’s Dual Cloud Strategy
BHP is set to use AWS’s analytics, machine learning, storage and compute platform to deploy digital services and improve operational performance. They will also launch an AWS Cloud Academy Program to train and upskill their employees on AWS cloud skills – joining other Australian companies supporting their digital workforce by forming cloud guilds such as National Australia Bank, Telstra and Kmart Group.
Meanwhile, BHP will use Microsoft’s Azure cloud platform to host their global applications portfolio including SAP S/4 HANA environment. This is expected to enable BHP to reduce their reliance on regional data centres and leverage Microsoft’s cloud environment, licenses and SAP applications. The deal extends their existing relationship with Microsoft where BHP is using Office 365, Dynamics 365 and HoloLens 2 platforms to support their productivity and remote operations.
Ecosystm principal Advisor, Alan Hesketh says, “This dual sourcing is likely to achieve cost benefits for BHP from a competitive negotiation stand-point, and positions BHP well to negotiate further improvements in the future. With their scale, BHP has negotiating power that most cloud service customers cannot achieve – although an effective competitive process is likely to offer tech buyers some improvements in pricing.”

Can this Strategy Work for You?
Hesketh thinks that the split between Microsoft for Operations and AWS for Analytics will provide some interesting challenges for BHP. “It is likely that high volumes of data will need to be moved between the two platforms, particularly from Operations to Analytics and AI. The trend is to run time-critical analytics directly from the operational systems using the power of in-memory databases and the scalable cloud platform.”
“As BHP states, using the cloud reduces the need to put hardware on-premises, and allows the faster deployment of digital innovations from these cloud platforms. While achieving technical and cost improvements in their Operations and Analytics domains, it may compromise the user experience (UX). The UX delivered by the two clouds is quite different – so delivering an integrated experience is likely to require an additional layer that is capable of delivering a consistent UX. BHP already has a strong network infrastructure in place, so they are likely to achieve this within their existing platforms. If there is a need to build this UX layer, it is likely to reduce the speed of deployment that BHP is targeting with the dual cloud procurement approach.”
Many businesses that have previously preferred a single cloud vendor will find that they will increasingly evaluate multiple cloud environments, in the future. The adoption of modern development environments and architectures such as containers, microservices, open-source, and DevOps will help them run their applications and processes on the most suitable cloud option.
While this strategy may well work for BHP, Hesketh adds, “Tech buyers considering a hybrid approach to cloud deployment need to have robust enterprise and technology architectures in place to make sure the users get the experience they need to support their roles.”
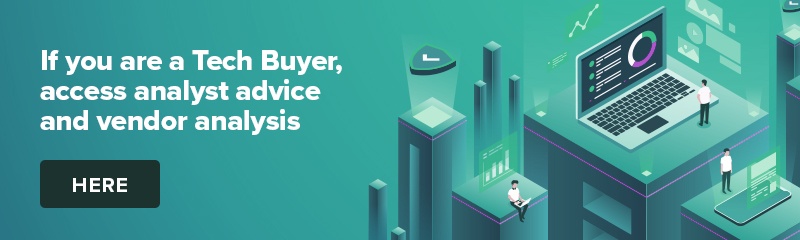