In the Ecosystm Predicts: Building an Agile & Resilient Organisation: Top 5 Trends in 2024, Principal Advisor Darian Bird said, “The emergence of Generative AI combined with the maturing of deepfake technology will make it possible for malicious agents to create personalised voice and video attacks.” Darian highlighted that this democratisation of phishing, facilitated by professional-sounding prose in various languages and tones, poses a significant threat to potential victims who rely on misspellings or oddly worded appeals to detect fraud. As we see more of these attacks and social engineering attempts, it is important to improve defence mechanisms and increase awareness.
Understanding Deepfake Technology
The term Deepfake is a combination of the words ‘deep learning’ and ‘fake’. Deepfakes are AI-generated media, typically in the form of images, videos, or audio recordings. These synthetic content pieces are designed to appear genuine, often leading to the manipulation of faces and voices in a highly realistic manner. Deepfake technology has gained spotlight due to its potential for creating convincing yet fraudulent content that blurs the line of reality.
Deepfake algorithms are powered by Generative Adversarial Networks (GANs) and continuously enhance synthetic content to closely resemble real data. Through iterative training on extensive datasets, these algorithms refine features such as facial expressions and voice inflections, ensuring a seamless emulation of authentic characteristics.
Deepfakes Becoming Increasingly Convincing
Hyper-realistic deepfakes, undetectable to the human eye and ear, have become a huge threat to the financial and technology sectors. Deepfake technology has become highly convincing, blurring the line between real and fake content. One of the early examples of a successful deepfake fraud was when a UK-based energy company lost USD 243k through a deepfake audio scam in 2019, where scammers mimicked the voice of their CEO to authorise an illegal fund transfer.
Deepfakes have evolved from audio simulations to highly convincing video manipulations where faces and expressions are altered in real-time, making it hard to distinguish between real and fake content. In 2022, for instance, a deepfake video of Elon Musk was used in a crypto scam that resulted in a loss of about USD 2 million for US consumers. This year, a multinational company in Hong Kong lost over USD 25 million when an employee was tricked into sending money to fraudulent accounts after a deepfake video call by what appeared to be his colleagues.
Regulatory Responses to Deepfakes
Countries worldwide are responding to the challenges posed by deepfake technology through regulations and awareness campaigns.
- Singapore’s Online Criminal Harms Act, that will come into effect in 2024, will empower authorities to order individuals and Internet service providers to remove or block criminal content, including deepfakes used for malicious purposes.
- The UAE National Programme for Artificial Intelligence released a deepfake guide to educate the public about both harmful and beneficial applications of this technology. The guide categorises fake content into shallow and deep fakes, providing methods to detect deepfakes using AI-based tools, with a focus on promoting positive uses of advanced technologies.
- The proposed EU AI Act aims to regulate them by imposing transparency requirements on creators, mandating them to disclose when content has been artificially generated or manipulated.
- South Korea passed a law in 2020 banning the distribution of harmful deepfakes. Offenders could be sentenced to up to five years in prison or fined up to USD 43k.
- In the US, states like California and Virginia have passed laws against deepfake pornography, while federal bills like the DEEP FAKES Accountability Act aim to mandate disclosure and counter malicious use, highlighting the diverse global efforts to address the multifaceted challenges of deepfake regulation.
Detecting and Protecting Against Deepfakes
Detecting deepfake becomes increasingly challenging as technology advances. Several methods are needed – sometimes in conjunction – to be able to detect a convincing deepfake. These include visual inspection that focuses on anomalies, metadata analysis to examine clues about authenticity, forensic analysis for pattern and audio examination, and machine learning that uses algorithms trained on real and fake video datasets to classify new videos.
However, identifying deepfakes requires sophisticated technology that many organisations may not have access to. This heightens the need for robust cybersecurity measures. Deepfakes have seen an increase in convincing and successful phishing – and spear phishing – attacks and cyber leaders need to double down on cyber practices.
Defences can no longer depend on spotting these attacks. It requires a multi-pronged approach which combines cyber technologies, incidence response, and user education.
Preventing access to users. By employing anti-spoofing measures organisations can safeguard their email addresses from exploitation by fraudulent actors. Simultaneously, minimising access to readily available information, particularly on websites and social media, reduces the chance of spear-phishing attempts. This includes educating employees about the implications of sharing personal information and clear digital footprint policies. Implementing email filtering mechanisms, whether at the server or device level, helps intercept suspicious emails; and the filtering rules need to be constantly evaluated using techniques such as IP filtering and attachment analysis.
Employee awareness and reporting. There are many ways that organisations can increase awareness in employees starting from regular training sessions to attack simulations. The usefulness of these sessions is often questioned as sometimes they are merely aimed at ticking off a compliance box. Security leaders should aim to make it easier for employees to recognise these attacks by familiarising them with standard processes and implementing verification measures for important email requests. This should be strengthened by a culture of reporting without any individual blame.
Securing against malware. Malware is often distributed through these attacks, making it crucial to ensure devices are well-configured and equipped with effective endpoint defences to prevent malware installation, even if users inadvertently click on suspicious links. Specific defences may include disabling macros and limiting administrator privileges to prevent accidental malware installation. Strengthening authentication and authorisation processes is also important, with measures such as multi-factor authentication, password managers, and alternative authentication methods like biometrics or smart cards. Zero trust and least privilege policies help protect organisation data and assets.
Detection and Response. A robust security logging system is crucial, either through off-the shelf monitoring tools, managed services, or dedicated teams for monitoring. What is more important is that the monitoring capabilities are regularly updated. Additionally, having a well-defined incident response can swiftly mitigate post-incident harm post-incident. This requires clear procedures for various incident types and designated personnel for executing them, such as initiating password resets or removing malware. Organisations should ensure that users are informed about reporting procedures, considering potential communication challenges in the event of device compromise.
Conclusion
The rise of deepfakes has brought forward the need for a collaborative approach. Policymakers, technology companies, and the public must work together to address the challenges posed by deepfakes. This collaboration is crucial for making better detection technologies, establishing stronger laws, and raising awareness on media literacy.
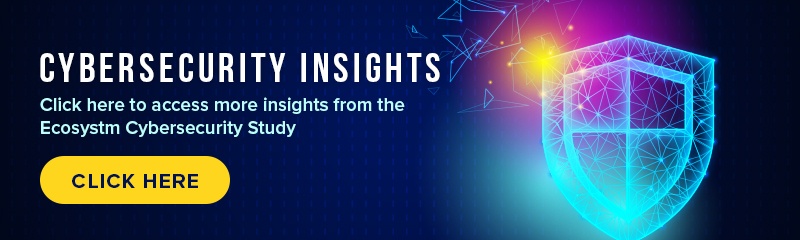
In my last Ecosystm Insights, I spoke about the implications of the shift from Predictive AI to Generative AI on ROI considerations of AI deployments. However, from my discussions with colleagues and technology leaders it became clear that there is a need to define and distinguish between Predictive AI and Generative AI better.
Predictive AI analyses historical data to predict future outcomes, crucial for informed decision-making and strategic planning. Generative AI unlocks new avenues for innovation by creating novel data and content. Organisations need both – Predictive AI for enhancing operational efficiencies and forecasting capabilities and Generative AI to drive innovation; create new products, services, and experiences; and solve complex problems in unprecedented ways.
This guide aims to demystify these categories, providing clarity on their differences, applications, and examples of the algorithms they use.
Predictive AI: Forecasting the Future
Predictive AI is extensively used in fields such as finance, marketing, healthcare and more. The core idea is to identify patterns or trends in data that can inform future decisions. Predictive AI relies on statistical, machine learning, and deep learning models to forecast outcomes.
Key Algorithms in Predictive AI
- Regression Analysis. Linear and logistic regression are foundational tools for predicting a continuous or categorical outcome based on one or more predictor variables.
- Decision Trees. These models use a tree-like graph of decisions and their possible consequences, including chance event outcomes, resource costs and utility.
- Random Forest (RF). An ensemble learning method that operates by constructing a multitude of decision trees at training time to improve predictive accuracy and control over-fitting.
- Gradient Boosting Machines (GBM). Another ensemble technique that builds models sequentially, each new model correcting errors made by the previous ones, used for both regression and classification tasks.
- Support Vector Machines (SVM). A supervised machine learning model that uses classification algorithms for two-group classification problems.
Generative AI: Creating New Data
Generative AI, on the other hand, focuses on generating new data that is similar but not identical to the data it has been trained on. This can include anything from images, text, and videos to synthetic data for training other AI models. GenAI is particularly known for its ability to innovate, create, and simulate in ways that predictive AI cannot.
Key Algorithms in Generative AI
- Generative Adversarial Networks (GANs). Comprising two networks – a generator and a discriminator – GANs are trained to generate new data with the same statistics as the training set.
- Variational Autoencoders (VAEs). These are generative algorithms that use neural networks for encoding inputs into a latent space representation, then reconstructing the input data based on this representation.
- Transformer Models. Originally designed for natural language processing (NLP) tasks, transformers can be adapted for generative purposes, as seen in models like GPT (Generative Pre-trained Transformer), which can generate coherent and contextually relevant text based on a given prompt.
Comparing Predictive and Generative AI
The fundamental difference between the two lies in their primary objectives: Predictive AI aims to forecast future outcomes based on past data, while Generative AI aims to create new, original data that mimics the input data in some form.
The differences become clearer when we look at these examples.
Predictive AI Examples
- Supply Chain Management. Analyses historical supply chain data to forecast demand, manage inventory levels, reduces costs and improve delivery times.
- Healthcare. Analysing patient records to predict disease outbreaks or the likelihood of a disease in individual patients.
- Predictive Maintenance. Analyse historical and real-time data and preemptively identifies system failures or network issues, enhancing infrastructure reliability and operational efficiency.
- Finance. Using historical stock prices and indicators to predict future market trends.
Generative AI Examples
- Content Creation. Generating realistic images or art from textual descriptions using GANs.
- Text Generation. Creating coherent and contextually relevant articles, stories, or conversational responses using transformer models like GPT-3.
- Chatbots and Virtual Assistants. Advanced GenAI models are enhancing chatbots and virtual assistants, making them more realistic.
- Automated Code Generation. By the use of natural language descriptions to generate programming code and scripts, to significantly speed up software development processes.
Conclusion
Organisations that exclusively focus on Generative AI may find themselves at the forefront of innovation, by leveraging its ability to create new content, simulate scenarios, and generate original data. However, solely relying on Generative AI without integrating Predictive AI’s capabilities may limit an organisation’s ability to make data-driven decisions and forecasts based on historical data. This could lead to missed opportunities to optimise operations, mitigate risks, and accurately plan for future trends and demands. Predictive AI’s strength lies in analysing past and present data to inform strategic decision-making, crucial for long-term sustainability and operational efficiency.
It is essential for companies to adopt a dual-strategy approach in their AI efforts. Together, these AI paradigms can significantly amplify an organisation’s ability to adapt, innovate, and compete in rapidly changing markets.
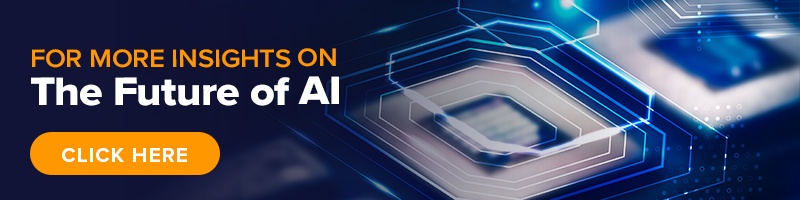