ASEAN, poised to become the world’s 4th largest economy by 2030, is experiencing a digital boom. With an estimated 125,000 new internet users joining daily, it is the fastest-growing digital market globally. These users are not just browsing, but are actively engaged in data-intensive activities like gaming, eCommerce, and mobile business. As a result, monthly data usage is projected to soar from 9.2 GB per user in 2020 to 28.9 GB per user by 2025, according to the World Economic Forum. Businesses and governments are further fuelling this transformation by embracing Cloud, AI, and digitisation.
Investments in data centre capacity across Southeast Asia are estimated to grow at a staggering pace to meet this growing demand for data. While large hyperscale facilities are currently handling much of the data needs, edge computing – a distributed model placing data centres closer to users – is fast becoming crucial in supporting tomorrow’s low-latency applications and services.
The Big & the Small: The Evolving Data Centre Landscape
As technology pushes boundaries with applications like augmented reality, telesurgery, and autonomous vehicles, the demand for ultra-low latency response times is skyrocketing. Consider driverless cars, which generate a staggering 5 TB of data per hour and rely heavily on real-time processing for split-second decisions. This is where edge data centres come in. Unlike hyperscale data centres, edge data centres are strategically positioned closer to users and devices, minimising data travel distances and enabling near-instantaneous responses; and are typically smaller with a capacity ranging from 500 KW to 2 MW. In comparison, large data centres have a capacity of more than 80MW.
While edge data centres are gaining traction, cloud-based hyperscalers such as AWS, Microsoft Azure, and Google Cloud remain a dominant force in the Southeast Asian data centre landscape. These facilities require substantial capital investment – for instance, it took almost USD 1 billion to build Meta’s 150 MW hyperscale facility in Singapore – but offer immense processing power and scalability. While hyperscalers have the resources to build their own data centres in edge locations or emerging markets, they often opt for colocation facilities to familiarise themselves with local markets, build out operations, and take a “wait and see” approach before committing significant investments in the new market.
The growth of data centres in Southeast Asia – whether edge, cloud, hyperscale, or colocation – can be attributed to a range of factors. The region’s rapidly expanding digital economy and increasing internet penetration are the prime reasons behind the demand for data storage and processing capabilities. Additionally, stringent data sovereignty regulations in many Southeast Asian countries require the presence of local data centres to ensure compliance with data protection laws. Indonesia’s Personal Data Protection Law, for instance, allows personal data to be transferred outside of the country only where certain stringent security measures are fulfilled. Finally, the rising adoption of cloud services is also fuelling the need for onshore data centres to support cloud infrastructure and services.
Notable Regional Data Centre Hubs
Singapore. Singapore imposed a moratorium on new data centre developments between 2019 to 2022 due to concerns over energy consumption and sustainability. However, the city-state has recently relaxed this ban and announced a pilot scheme allowing companies to bid for permission to develop new facilities.
In 2023, the Singapore Economic Development Board (EDB) and the Infocomm Media Development Authority (IMDA) provisionally awarded around 80 MW of new capacity to four data centre operators: Equinix, GDS, Microsoft, and a consortium of AirTrunk and ByteDance (TikTok’s parent company). Singapore boasts a formidable digital infrastructure with 100 data centres, 1,195 cloud service providers, and 22 network fabrics. Its robust network, supported by 24 submarine cables, has made it a global cloud connectivity leader, hosting major players like AWS, Azure, IBM Softlayer, and Google Cloud.
Aware of the high energy consumption of data centres, Singapore has taken a proactive stance towards green data centre practices. A collaborative effort between the IMDA, government agencies, and industries led to the development of a “Green Data Centre Standard“. This framework guides organisations in improving data centre energy efficiency, leveraging the established ISO 50001 standard with customisations for Singapore’s context. The standard defines key performance metrics for tracking progress and includes best practices for design and operation. By prioritising green data centres, Singapore strives to reconcile its digital ambitions with environmental responsibility, solidifying its position as a leading Asian data centre hub.
Malaysia. Initiatives like MyGovCloud and the Digital Economy Blueprint are driving Malaysia’s public sector towards cloud-based solutions, aiming for 80% use of cloud storage. Tenaga Nasional Berhad also established a “green lane” for data centres, solidifying Malaysia’s commitment to environmentally responsible solutions and streamlined operations. Some of the big companies already operating include NTT Data Centers, Bridge Data Centers and Equinix.
The district of Kulai in Johor has emerged as a hotspot for data centre activity, attracting major players like Nvidia and AirTrunk. Conditional approvals have been granted to industry giants like AWS, Microsoft, Google, and Telekom Malaysia to build hyperscale data centres, aimed at making the country a leading hub for cloud services in the region. AWS also announced a new AWS Region in the country that will meet the high demand for cloud services in Malaysia.
Indonesia. With over 200 million internet users, Indonesia boasts one of the world’s largest online populations. This expanding internet economy is leading to a spike in the demand for data centre services. The Indonesian government has also implemented policies, including tax incentives and a national data centre roadmap, to stimulate growth in this sector.
Microsoft, for instance, is set to open its first regional data centre in Thailand and has also announced plans to invest USD 1.7 billion in cloud and AI infrastructure in Indonesia. The government also plans to operate 40 MW of national data centres across West Java, Batam, East Kalimantan, and East Nusa Tenggara by 2026.
Thailand. Remote work and increasing online services have led to a data centre boom, with major industry players racing to meet Thailand’s soaring data demands.
In 2021, Singapore’s ST Telemedia Global Data Centres launched its first 20 MW hyperscale facility in Bangkok. Soon after, AWS announced a USD 5 billion investment plan to bolster its cloud capacity in Thailand and the region over the next 15 years. Heavyweights like TCC Technology Group, CAT Telecom, and True Internet Data Centre are also fortifying their data centre footprints to capitalise on this explosive growth. Microsoft is also set to open its first regional data centre in the country.
Conclusion
Southeast Asia’s booming data centre market presents a goldmine of opportunity for tech investment and innovation. However, navigating this lucrative landscape requires careful consideration of legal hurdles. Data protection regulations, cross-border data transfer restrictions, and local policies all pose challenges for investors. Beyond legal complexities, infrastructure development needs and investment considerations must also be addressed. Despite these challenges, the potential rewards for companies that can navigate them are substantial.
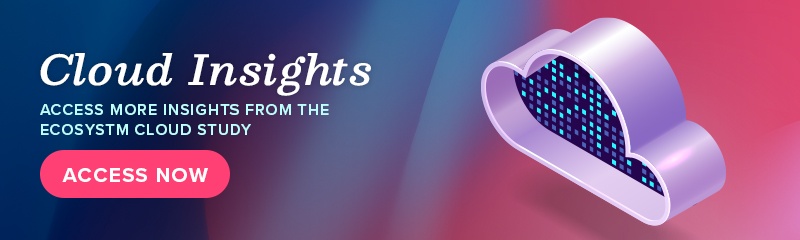
Hewlett Packard Enterprise (HPE) has entered into a definitive agreement to acquire Juniper Networks for USD 40 per share, totaling an equity value of about USD 14 Billion. This strategic move is aimed to enhance HPE’s portfolio by focusing on higher-growth solutions and reinforcing their high-margin networking business. HPE expects to double their networking business, positioning the combined entity as a leader in networking solutions. With the growing demand for secure, unified technology driven by AI and hybrid cloud trends, HPE aims to offer comprehensive, disruptive solutions that connect, protect, and analyse data from edge to cloud.
This would also be the organisation’s largest deal since becoming an independent company in 2015. The acquisition is expected to be completed by late 2024 or early 2025.
Ecosystm analysts Darian Bird and Richard Wilkins provide their insights on the HPE acquisition and its implications for the tech market.
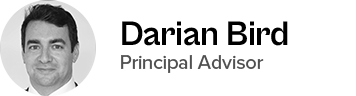
Converging Networking and Security
One of the big drawcards for HPE is Juniper’s Mist AI. The networking vendors have been racing to catch up – both in capabilities and in marketing. The acquisition though will give HPE a leadership position in network visibility and manageability. With GreenLake and soon Mist AI, HPE will have a solid AIOps story across the entire infrastructure.
HPE has been working steadily towards becoming a player in the converged networking-security space. They integrated Silver Peak well to make a name for themselves in SD-WAN and last year acquiring Axis Security gave them the Zero Trust Network Access (ZTNA), Secure Web Gateway (SWG), and Cloud Access Security Broker (CASB) modules in the Secure Service Edge (SSE) stack. Bringing all of this to the market with Juniper’s networking prowess positions HPE as a formidable player, especially as the Secure Access Service Edge (SASE) market gains momentum.
As the market shifts towards converged SASE, there will only be more interest in the SD-WAN and SSE vendors. In just over one year, Cato Networks and Netskope have raised funds, Check Point acquired Perimeter 81, and Versa Networks has made noises about an IPO. The networking and security players are all figuring out how they can deliver a single-vendor SASE.
Although HPE’s strategic initiatives signal a robust market position, potential challenges arise from the overlap between Aruba and Juniper. However, the distinct focus on the edge and data center, respectively, may help alleviate these concerns. The acquisition also marks HPE’s foray into the telecom space, leveraging its earlier acquisition of Athonet and establishing a significant presence among service providers. This expansion enhances HPE’s overall market influence, posing a challenge to the long-standing dominance of Cisco.
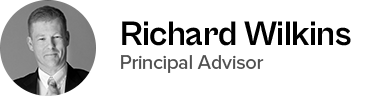
The strategic acquisition of Juniper Networks by HPE can make a transformative leap in AIOps and Software-Defined Networking (SDN). There is a potential for this to establish a new benchmark in IT management.
AI in IT Operations Transformation
The integration of Mist’s AI-driven wireless solutions and HPE’s SDN is a paradigm shift in IT operations management and will help organisations transition from a reactive to a predictive and proactive model. Mist’s predictive analytics, coupled with HPE’s SDN capabilities, empower networks to dynamically adjust to user demands and environmental changes, ensuring optimal performance and user experience. Marvis, Mist’s Virtual Network Assistant (VNA), adds conversational troubleshooting capabilities, enhancing HPE’s network solutions. The integration envisions an IT ecosystem where Juniper’s AI augments HPE’s InfoSight, providing deeper insights into network behaviour, preemptive security measures, and more autonomous IT operations.
Transforming Cloud and Edge Computing
The incorporation of Juniper’s AI into HPE’s cloud and edge computing solutions promises a significant improvement in data processing and management. AI-driven load balancing and resource allocation mechanisms will significantly enhance multi-cloud environment efficiency, ensuring robust and seamless cloud services, particularly vital in IoT applications where real-time data processing is critical. This integration not only optimises cloud operations but also has the potential to align with HPE’s commitment to sustainability, showcasing how AI advancements can contribute to energy conservation.
In summary, HPE’s acquisition of Juniper Networks, and specifically the integration of the Mist AI platform, is a pivotal step towards an AI-driven, efficient, and predictive IT infrastructure. This can redefine the standards in AIOps and SDN, creating a future where IT systems are not only reactive but also intuitively adaptive to the evolving demands of the digital landscape.
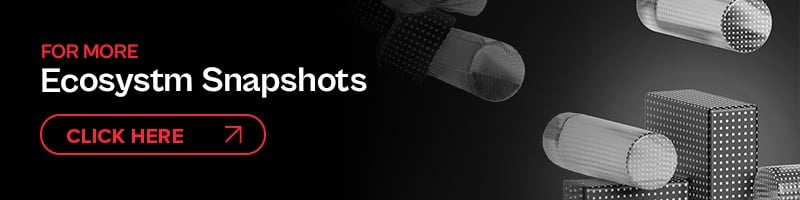
Organisations in Asia Pacific are no longer only focused on employing a cloud-first strategy – they want to host the infrastructure and workloads where it makes the most sense; and expect a seamless integration across multiple cloud environments.
While cloud can provide the agile infrastructure that underpins application modernisation, innovative leaders recognise that it is only the first step on the path towards developing AI-powered organisations. The true value of cloud is in the data layer, unifying data around the network, making it securely available wherever it is needed, and infusing AI throughout the organisation.
Cloud provides a dynamic and powerful platform on which organisations can build AI. Pre-trained foundational models, pay-as-you-go graphics superclusters, and automated ML tools for citizen data scientists are now all accessible from the cloud even to start-ups.
Organisations should assess the data and AI capabilities of their cloud providers rather than just considering it an infrastructure replacement. Cloud providers should use native services or integrations to manage the data lifecycle from labelling to model development, and deployment.
In this Ecosystm Byte, sponsored by Oracle, Ecosystm Principal Advisor, Darian Bird presents the top 5 trends for Cloud in 2023 and beyond. Read on to find out more.
Download ‘The Top 5 Cloud Trends for 2023 & Beyond’ as a PDF
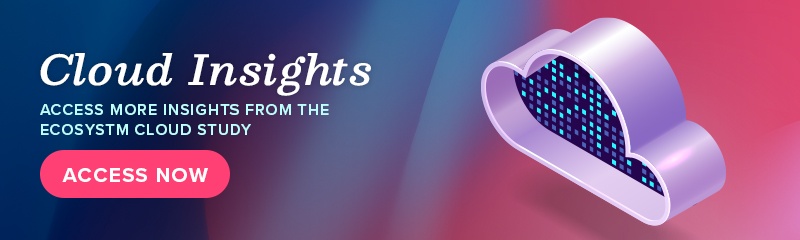
In this Insight, guest author Anupam Verma talks about how a smart combination of technologies such as IoT, edge computing and AI/machine learning can be a game changer for the Financial Services industry. “With the rise in the number of IoT devices and increasing financial access, edge computing will find its place in the sun and complement (and not compete) with cloud computing.”
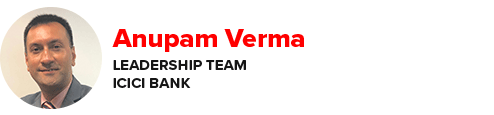
The number of IoT devices have now crossed the population of planet earth. The buzz around the Internet of Things (IoT) refuses to go down and many believe that with 5G rollouts and edge computing, the adoption will rise exponentially in the next 5 years.
The IoT is described as the network of physical objects (“things”) embedded with sensors and software to connect and exchange data with other devices over the internet. Edge computing allows IoT devices to process data near the source of generation and consumption. This could be in the device itself (e.g. sensors), or close to the device in a small data centre. Typically, edge computing is advantageous for mission-critical applications which require near real-time decision making and low latency. Other benefits include improved data security by avoiding the risk of interception of data in transfer channels, less network traffic and lower cost. Edge computing provides an alternative to sending data to a centralised cloud.
In the 5G era, a smart combination of technologies such as IoT, edge computing and AI/machine learning will be a game changer. Multiple uses cases from self-driving vehicles to remote monitoring and maintenance of machinery are being discussed. How do we see IoT and the Edge transforming Financial Services?
Before we go into how these technologies can transforming the industry, let us look at current levels of perception and adoption (Figure 1).
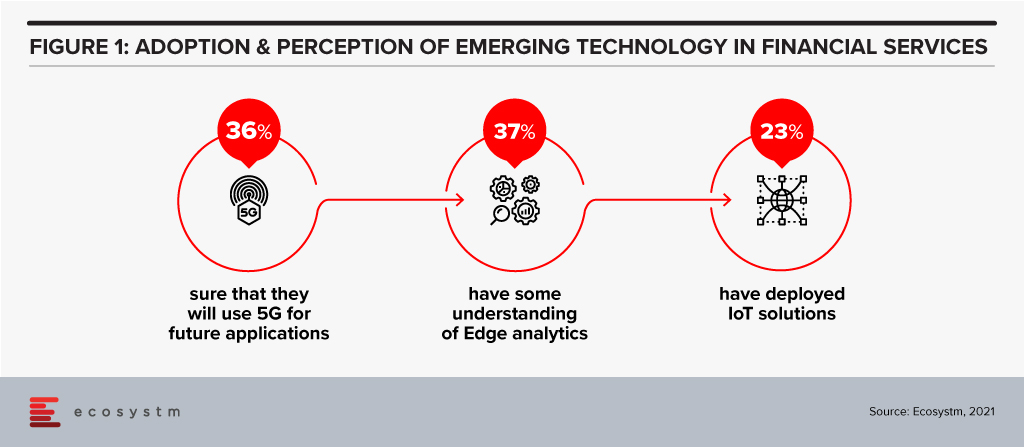
There is definitely a need for greater awareness of the capabilities and limitations of these emerging technologies in the Financial Services.
Transformation of Financial Services
The BFSI sector is increasingly moving away from selling a product to creating a seamless customer journey. Financial transactions, whether it is payment, transfer of money, or a loan can be invisible, and Edge computing will augment the customer experience. This cannot be achieved without having real-time data and analytics to create an updated 360-degree profile of the customer at all times. This data could come from multiple IoT devices, channels and partners that can interface and interact with the customer. A lot of use cases around personalisation would not be possible without edge computing. The Edge here would mean faster processing and smoother experience leading to customer delight and a higher trust quotient.
With IoT, customers can bank anywhere anytime using connected devices like wearables (smartwatches, fitness trackers etc). People can access account details, contextual offers at their current location or make payments without even needing a smartphone.

Use Cases of IoT & Edge in Financial Services
IT and Digital Leaders in Financial Services are aware of the benefits of IoT and there are some use cases that most of them think will help transform Financial Services (Figure 2).
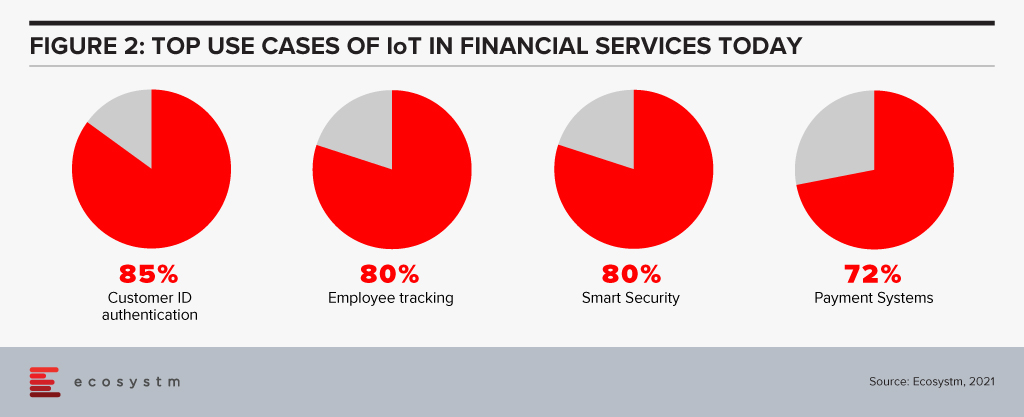
However, there are many more potential use cases. Here are some use cases whose volume will only grow every day to fuel incessant data generation, consumption and processing at the Edge.
- Smart Homes. IoT devices like Alexa/Google Home have capabilities to become “bank in a speaker” with edge computing.
- In-Sync Omnichannels. IoT devices can be synced with other banking channels. A customer may start a transaction on an IoT device and complete it in a branch. Facial recognition can be used to identify the customer after he/she walks in and synced IoT devices will ensure that the transaction is completed without any steps repeated (zero re-work) thereby enhancing customer satisfaction.
- Virtual Relationship Managers. In a digital branch, the customer may use Virtual Reality (VR) headsets to engage with virtual relationship managers and relevant experts. Gamification using VR can be amazingly effective in the area of financial literacy and financial planning.
- Home and Auto Purchase. VR may also find use in home and auto purchase processes with financing built into it. The entire customer journey will have a much smoother experience with edge computing.
- Auto and Health Insurance. Companies can use IoT (device installed in the vehicle) plus edge computing to monitor and improve driving behaviour, eventually rewarding safety with lower premiums. The growth in electric mobility will continue to provide the basis for auto insurance. Companies can use wearables to monitor crucial health parameters and exercising habits. The creation of real-time dynamic rewards around it can change behaviour towards a healthier lifestyle. Awareness, longevity, rising costs and pandemic will only fuel this sector’s growth.
- Payments. Device to device contactless payment protocol is picking up and IoT and edge computing can create next-gen revolution in payments. Your EV could have an embedded wallet and pay for its parking and toll.
- Branch/ATM. IoT sensors and CCTV footage from branches/ATMs can be utilised in real-time to improve branch productivity as well as customer engagement, at the same time enhancing security. It could also help in other situations like low cash levels in ATMs and malfunctions. Sending live video streams for video analytics to the cloud can be expensive. By processing data within the device or on-premises, the Edge can help lower costs and reduce latency.
- Trading in Securities. Another area where response time matters is algorithmic trading. Edge computing will help to quickly process and analyse a large amount of data streaming real-time from multiple feeds and react appropriately.
- Trade Finance. Real-time tracking of goods may add a different dimension to the risk, pricing and transparency of supply chains.
Cloud vs Edge
The decision to use cloud or edge will depend on multiple considerations. At the same time, all the data from IoT devices need not go to the cloud for processing and choke network bandwidth. In fact, some of this data need not be stored forever (like video feeds etc). As a result, with the rise in the number of IoT devices and increasing financial access, edge computing will find its place in the sun and complement (and not compete) with cloud computing.
The views and opinions mentioned in the article are personal.
Anupam Verma is part of the Leadership team at ICICI Bank and his responsibilities have included leading the Bank’s strategy in South East Asia to play a significant role in capturing Investment, NRI remittance, and trade flows between SEA and India.
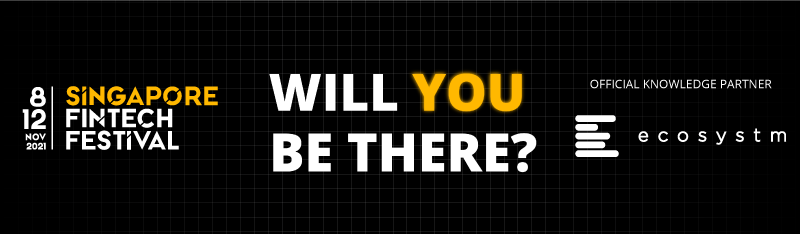
As we return to the office, there is a growing reliance on devices to tell us how safe and secure the environment is for our return. And in specific application areas, such as Healthcare and Manufacturing, IoT data is critical for decision-making. In some sectors such as Health and Wellness, IoT devices collect personally identifiable information (PII). IoT technology is so critical to our current infrastructures that the physical wellbeing of both individuals and organisations can be at risk.
Trust & Data
IoT are also vulnerable to breaches if not properly secured. And with a significant increase in cybersecurity events over the last year, the reliance on data from IoT is driving the need for better data integrity. Security features such as data integrity and device authentication can be accomplished through the use of digital certificates and these features need to be designed as part of the device prior to manufacturing. Because if you cannot trust either the IoT devices and their data, there is no point in collecting, running analytics, and executing decisions based on the information collected.
We discuss the role of embedding digital certificates into the IoT device at manufacture to enable better security and ongoing management of the device.
Securing IoT Data from the Edge
So much of what is happening on networks in terms of real-time data collection happens at the Edge. But because of the vast array of IoT devices connecting at the Edge, there has not been a way of baking trust into the manufacture of the devices. With a push to get the devices to market, many manufacturers historically have bypassed efforts on security. Devices have been added on the network at different times from different sources.
There is a need to verify the IoT devices and secure them, making sure to have an audit trail on what you are connecting to and communicating with.
So from a product design perspective, this leads us to several questions:
- How do we ensure the integrity of data from devices if we cannot authenticate them?
- How do we ensure that the operational systems being automated are controlled as intended?
- How do we authenticate the device on the network making the data request?
Using a Public Key Infrastructure (PKI) approach maintains assurance, integrity and confidentiality of data streams. PKI has become an important way to secure IoT device applications, and this needs to be built into the design of the device. Device authentication is also an important component, in addition to securing data streams. With good design and a PKI management that is up to the task you should be able to proceed with confidence in the data created at the Edge.
Johnson Controls/DigiCert have designed a new way of managing PKI certification for IoT devices through their partnership and integration of the DigiCert ONE™ PKI management platform and the Johnson Controls OpenBlue IoT device platform. Based on an advanced, container-based design, DigiCert ONE allows organisations to implement robust PKI deployment and management in any environment, roll out new services and manage users and devices across your organisation at any scale no matter the stage of their lifecycle. This creates an operational synergy within the Operational Technology (OT) and IoT spaces to ensure that hardware, software and communication remains trusted throughout the lifecycle.

Rationale on the Role of Certification in IoT Management
Digital certificates ensure the integrity of data and device communications through encryption and authentication, ensuring that transmitted data are genuine and have not been altered or tampered with. With government regulations worldwide mandating secure transit (and storage) of PII data, PKI can help ensure compliance with the regulations by securing the communication channel between the device and the gateway.
Connected IoT devices interact with each other through machine to machine (M2M) communication. Each of these billions of interactions will require authentication of device credentials for the endpoints to prove the device’s digital identity. In such scenarios, an identity management approach based on passwords or passcodes is not practical, and PKI digital certificates are by far the best option for IoT credential management today.
Creating lifecycle management for connected devices, including revocation of expired certificates, is another example where PKI can help to secure IoT devices. Having a robust management platform that enables device management, revocation and renewal of certificates is a critical component of a successful PKI. IoT devices will also need regular patches and upgrades to their firmware, with code signing being critical to ensure the integrity of the downloaded firmware – another example of the close linkage between the IoT world and the PKI world.
Summary
PKI certification benefits both people and processes. PKI enables identity assurance while digital certificates validate the identity of the connected device. Use of PKI for IoT is a necessary trend for sense of trust in the network and for quality control of device management.
Identifying the IoT device is critical in managing its lifespan and recognizing its legitimacy in the network. Building in the ability for PKI at the device’s manufacture is critical to enable the device for its lifetime. By recognizing a device, information on it can be maintained in an inventory and its lifecycle and replacement can be better managed. Once a certificate has been distributed and certified, having the control of PKI systems creates life-cycle management.
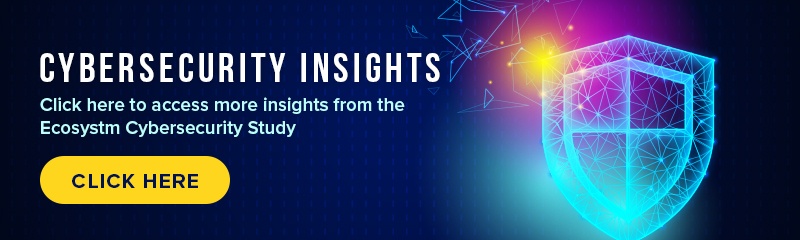
Two years ago at Discover, HP Enterprise’s President and CEO, Antonio Neri promised that all of HPE’s portfolio would be available ‘as a service’ within three years.
At the current Discover virtual events, HPE made a series of announcements to showcase that GreenLake is on its way to meet that ambitious goal in 2022. HPE continues to evolve their enterprise capabilities, as is demonstrated by their acquisitions of Determined AI and Zerto.
Ecosystm Advisors, Alan Hesketh, Darian Bird, and Niloy Mukherjee comment on how HPE is preparing for the Hybrid world and the key announcements at HPE Discover, 2021 including GreenLake, Lighthouse, and Aurora.
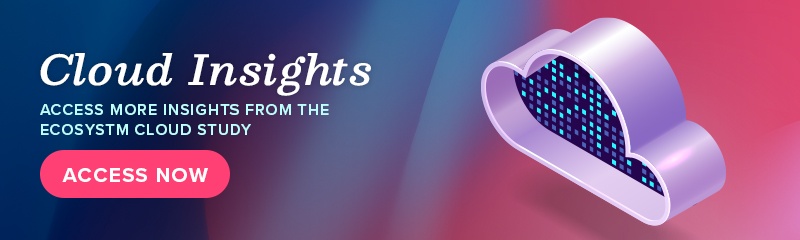
Organisations have found that it is not always desirable to send data to the cloud due to concerns about latency, connectivity, energy, privacy and security. So why not create learning processes at the Edge?
What challenges does IoT bring?
Sensors are now generating such an increasing volume of data that it is not practical that all of it be sent to the cloud for processing. From a data privacy perspective, some sensor data is sensitive and sending data and images to the cloud will be subject to privacy and security constraints.
Regardless of the speed of communications, there will always be a demand for more data from more sensors – along with more security checks and higher levels of encryption – causing the potential for communication bottlenecks.
As the network hardware itself consumes power, sending a constant stream of data to the cloud can be taxing for sensor devices. The lag caused by the roundtrip to the cloud can be prohibitive in applications that require real-time response inputs.
Machine learning (ML) at the Edge should be prioritised to leverage that constant flow of data and address the requirement for real-time responses based on that data. This should be aided by both new types of ML algorithms and by visual processing units (VPUs) being added to the network.
By leveraging ML on Edge networks in production facilities, for example, companies can look out for potential warning signs and do scheduled maintenance to avoid any nasty surprises. Remember many sensors are linked intrinsically to public safety concerns such as water processing, supply of gas or oil, and public transportation such as metros or trains.
Ecosystm research shows that deploying IoT has its set of challenges (Figure 1) – many of these challenges can be mitigated by processing data at the Edge.
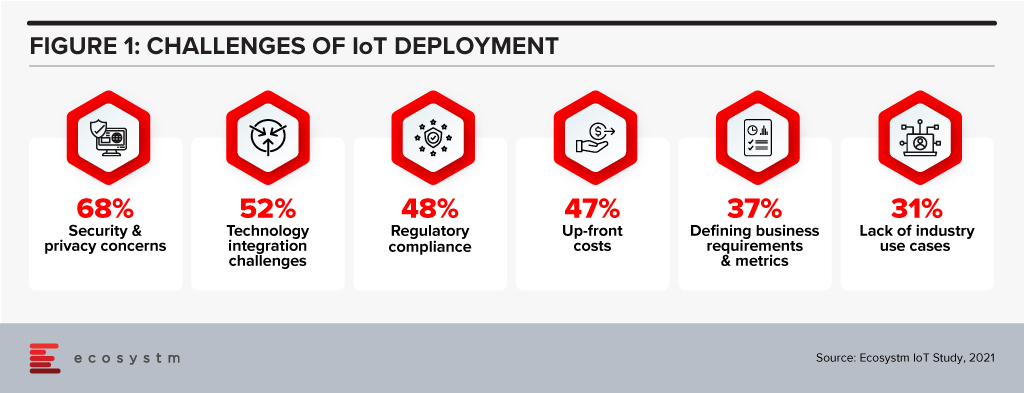
Predictive analytics is a fundamental value proposition for IoT, where responding faster to issues or taking action before issues occur, is key to a high return on investment. So, using edge computing for machine learning located within or close to the point of data gathering can in some cases be a more practical or socially beneficial approach.
In IoT the role of an edge computer is to pre-process data and act before the data is passed on to the main server. This allows a faster, low latency response and minimal traffic between the cloud server processing and the Edge. However, a better understanding of the benefits of edge computing is required if it has to be beneficial for a number of outcomes.
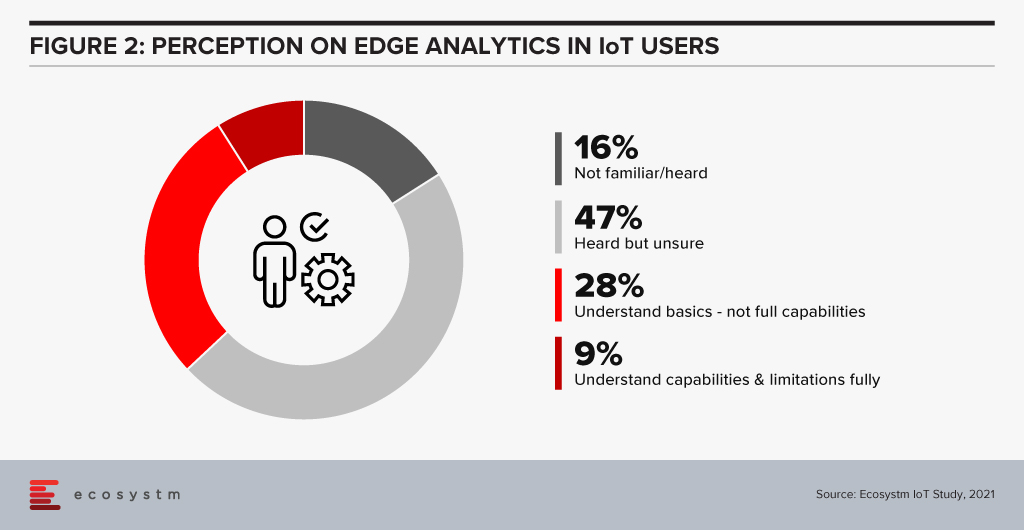

If we can get machine learning happening in the field, at the Edge, then we reduce the time lag and also create an extra trusted layer in unmanned production or automated utilities situations. This can create more trusted environments in terms of possible threats to public services.
What kind of examples of machine learning in the field can we see?
Healthcare
Health systems can improve hospital patient flow through machine learning (ML) at the Edge. ML offers predictive models to assist decision-makers with complex hospital patient flow information based on near real-time data.
For example, an academic medical centre created an ML pipeline that leveraged all its data – patient administration, EHR and clinical and claims data – to create learnings that could predict length of stay, emergency department (ED) arrival models, ED admissions, aggregate discharges, and total bed census. These predictive models proved effective as the medical centre reduced patient wait times and staff overtime and was able to demonstrate improved patient outcomes. And for a medical centre that use sensors to monitor patients and gather requests for medicine or assistance, Edge processing means keeping private healthcare data in-house rather than sending it off to cloud servers.
Retail
A retail store could use numerous cameras for self-checkout and inventory management and to monitor foot traffic. Such specific interaction details could slow down a network and can be replaced by an on-site Edge server with lower latency and a lower total cost. This is useful for standalone grocery pop-up sites such as in Sweden and Germany.
In Retail, k-nearest neighbours is often used in ML for abnormal activity analysis – this learning algorithm can also be used for visual pattern recognition used as part of retailers’ loss prevention tactics.
Summary
Working with the data locally on the Edge, creates reduced latency, reduced cloud usage and costs, independence from a network connection, more secure data, and increased data privacy.
Cloud and Edge computing that uses machine learning can together provide the best of both worlds: decentralised local storage, processing and reaction, and then uploading to the cloud, enabling additional insights, data backups (redundancy), and remote access.
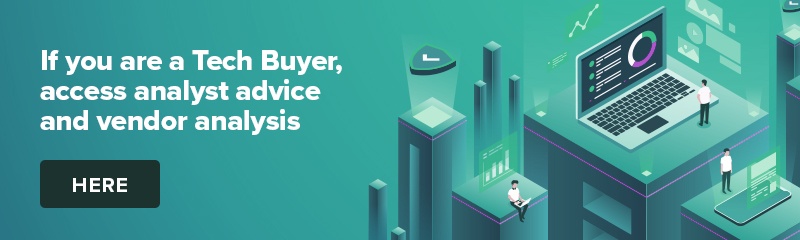
The last year has really pushed the Education sector into transforming both its teaching and learning practices. The urgency of the situation accelerated the use of networking to extend the reach and range of educational opportunities for remote learning.
Education technology has rushed to embrace opportunities to facilitate a new normal for Education. This new normal must enable and support education access, experiences, and outcomes as well as aid in developing strong relationships within Education ecosystems.
Education technology, commonly known as EdTech, focuses on leveraging emerging technologies like cloud and AI to deliver interactive and multimedia coursework over online platforms. This also requires a state-of-the-art network to support. 5G provides instantaneous access to cloud services. Use of 5G – as well as network function virtualisation (NFV), network slicing, and multi-access edge computing (MEC) – has the capability of delivering significant performance benefits across these emerging educational applications and use cases.
At present, many educational institutions are aware of the possibilities, but are not active users of 5G network infrastructure (Figure 1).
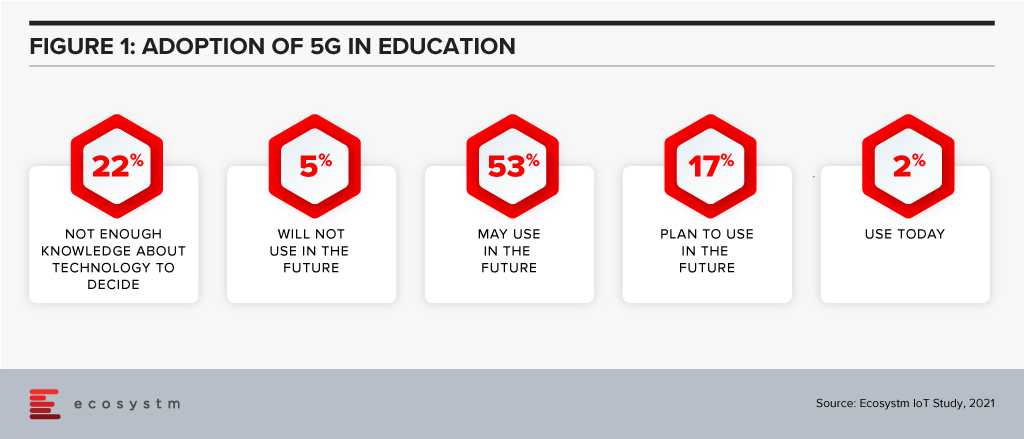
Educational institutions plan to do some near-term investments but are not clear in what areas to apply the enhanced capabilities (Figure 2).
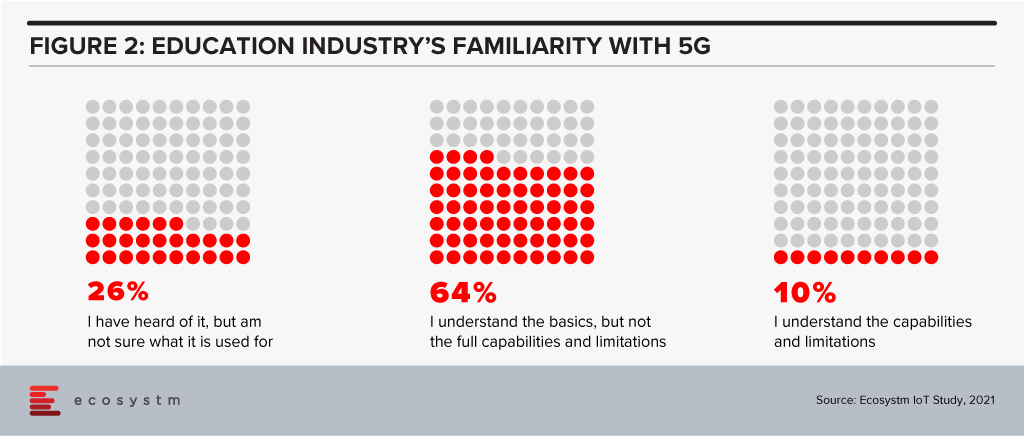
Role of the Network in Adaptive Learning
In their recent whitepaper, network provider Ciena talks about “the concept of an adaptive learning strategy – a technology-based teaching method that replaces the traditional one-size-fits-all teaching style with one that is more personalised to individual students. This approach leverages next-generation learning technologies to analyse a student’s performance and reactions to digital content in real-time, and modifies the lesson based on that data.”
To create an adaptive learning strategy that can be individualised, these learners need to be enabled by technology to be immersed in a learning experience, complete with multimedia and access to a knowledge base for information. And this is where a solid 5G network implementation can create access and bandwidth to the resources required.
Example of 5G and Immersive Learning
An example of adaptive learning where the technology not only supports but challenges the learner can be found in a BT-led new immersive classroom developed within the Muirfield Centre in Cumbernauld, North Lanarkshire, using innovative technology to transform a classroom into an engaging and digital learning environment.
Pupils at Carbrain Primary School, Cumbernauld, were the first to dive into the new experience with an underwater lesson about the ocean. The 360-degree room creates a digital projection that uses all four classroom walls and the ceiling to bring the real-world into an immersive experience for students. The concept aims to push beyond traditional methods of teaching to create an inclusive digital experience that helps explain abstract and challenging concepts through a 3D model. It will also have the potential to support students with learning difficulties in developing imagination, creative and critical thinking, and problem-solving skills. BT has deployed its 5G Rapid Site solution to support 5G innovation and digital transformation of UK’s Education sector. The solution is made possible through the EE 5G network which brings ultrafast speeds and enhanced reliability to classrooms.
Conclusion
5G is expected to provide network improvement in the areas of latency, energy efficiency, the accuracy of terminal location, reliability, and availability – therefore creating the ability to better leverage cloud capacity.
With the greater bandwidth that 5G provides, learners and instructors, can connect virtually from any location with minimal disruption with more devices than on previous networks. This allows students to enjoy a rich learning experience and not be disadvantaged by their location for remote learning, or by the uncertainty of educational access. This also provides more possibilities of exploration and discovery beyond the physical confines of the classroom and puts those resources in the hands of eager learners.
As educational institutions reopen, institutions are looking at ways to redesign the education experience. Connected devices are helping schools and universities expand the boundaries of education. Explore what the IoT-enabled future of education would look like
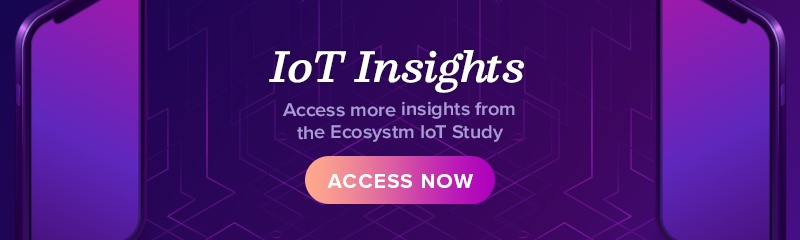
Last week AT&T announced a partnership with Fortinet to expand their managed security services portfolio. This partnership provides global managed Secure Access Service Edge (SASE) solutions at scale. The solution uses Fortinet’s SASE stack which unifies software-defined wide-area network (SD-WAN) and network security capabilities into AT&T managed cybersecurity framework. Additionally, AT&T SASE and Fortinet will integrate with AT&T Alien Labs Threat Intelligence platform, a threat intelligence unit to enhance detection and response. AT&T has plans to update its managed SASE service during the year and will continue to bring more options.
Talking about the AT&T-Fortinet partnership, Ecosystm Principal Advisor, Ashok Kumar says, “This move continues the trend of the convergence of networking and security solutions. AT&T is positioning themselves well with their integrated offer of network and security services to address the needs of global enterprises.”
Convergence of Network & Security
AT&T’s improved global managed security service includes features such as secure web gateway, firewall-as-a service, cloud access security broker (CASB) and zero-trust access, which provides security teams and analysts with unified capabilities across the cloud, networks and endpoints. The solution aims to enable enterprises to create a more resilient network bringing the core capabilities of the two companies that will reduce operational costs and deliver a unified offering.
Last year AT&T also partnered with Cisco to expand its SD-WAN solution and to support AT&T Managed Services using Cisco’s vManage controller through a single management interface. Over the past years multiple vendors including Fortinet have developed comprehensive SASE solution capabilities through partnerships or acquisitions to provide a unified offering. Last year Fortinet acquired Opaq, a SASE cloud provider to bolster their security capabilities through OPAQ’s patented Zero Trust Network Access (ZTNA) cloud solution and to strengthen SD-WAN, security and edge package.
The Push Towards Flexible Networking
Kumar says, “The pandemic has created a higher demand and value for secure networking services. Enterprises experienced greater number of phishing and malware attacks last year with the sudden increase in work-from-home users. The big question enterprises need to ask themselves is whether legacy networks can support their evolving business priorities.”
“As global economies look to recover, securing remote users working from anywhere, with full mobility, will be a high priority for all enterprises. Enterprises need to evaluate mobile SASE services that provide frictionless identity management with seamless user experiences, and be compatible with the growing adoption of 5G services in 2021 and beyond.”
The Top 5 Telecommunications & Mobility Trends that will dominate the telecom industry to watch out for in 2021. Signup for Free to download the report.
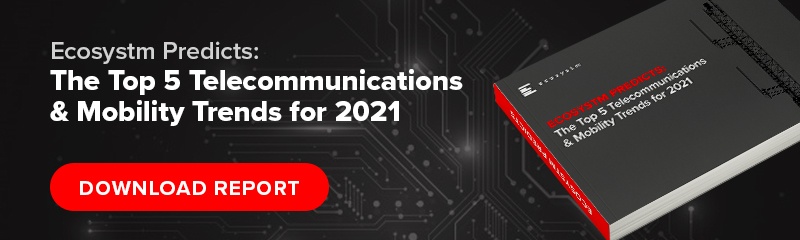