The data architecture outlines how data is managed in an organisation and is crucial for defining the data flow, data management systems required, the data processing operations, and AI applications. Data architects and engineers define data models and structures based on these requirements, supporting initiatives like data science. Before we delve into the right data architecture for your AI journey, let’s talk about the data management options. Technology leaders have the challenge of deciding on a data management system that takes into consideration factors such as current and future data needs, available skills, costs, and scalability. As data strategies become vital to business success, selecting the right data management system is crucial for enabling data-driven decisions and innovation.
Data Warehouse
A Data Warehouse is a centralised repository that stores vast amounts of data from diverse sources within an organisation. Its main function is to support reporting and data analysis, aiding businesses in making informed decisions. This concept encompasses both data storage and the consolidation and management of data from various sources to offer valuable business insights. Data Warehousing evolves alongside technological advancements, with trends like cloud-based solutions, real-time capabilities, and the integration of AI and machine learning for predictive analytics shaping its future.
Core Characteristics
- Integrated. It integrates data from multiple sources, ensuring consistent definitions and formats. This often includes data cleansing and transformation for analysis suitability.
- Subject-Oriented. Unlike operational databases, which prioritise transaction processing, it is structured around key business subjects like customers, products, and sales. This organisation facilitates complex queries and analysis.
- Non-Volatile. Data in a Data Warehouse is stable; once entered, it is not deleted. Historical data is retained for analysis, allowing for trend identification over time.
- Time-Variant. It retains historical data for trend analysis across various time periods. Each entry is time-stamped, enabling change tracking and trend analysis.
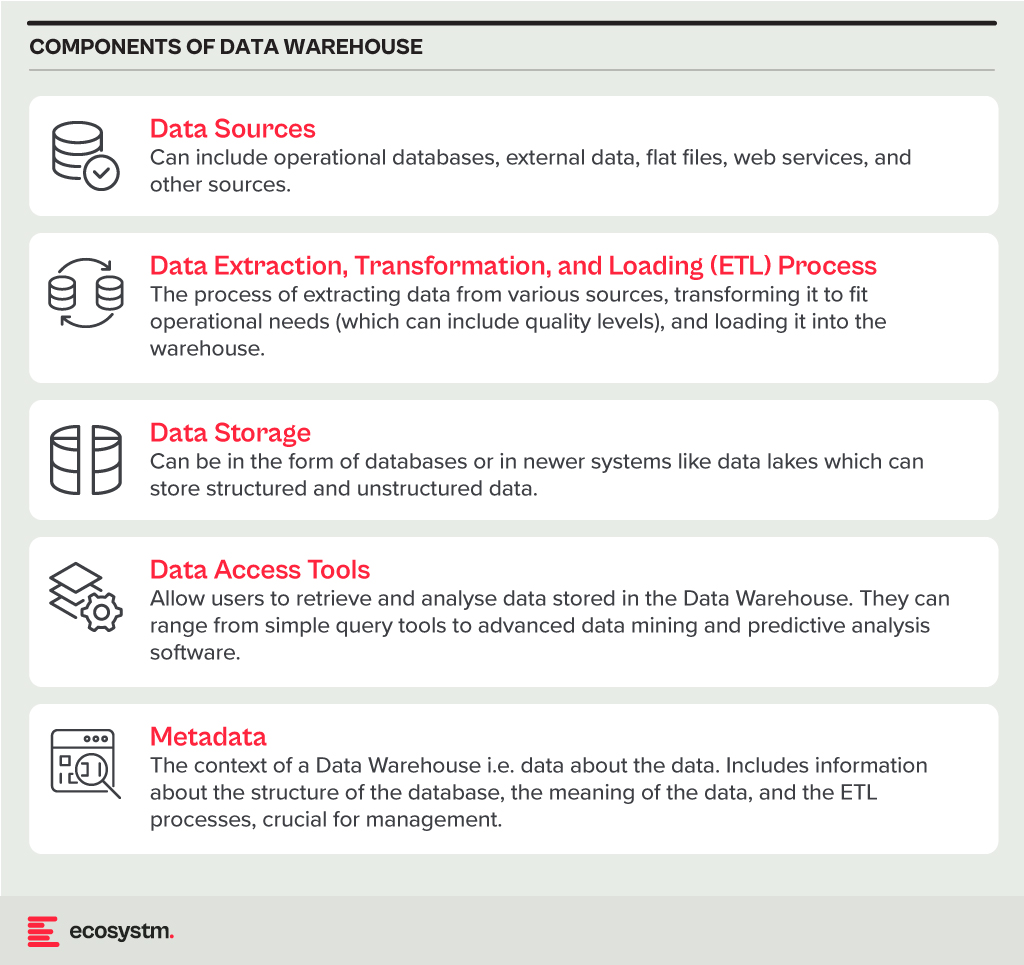
Benefits
- Better Decision Making. Data Warehouses consolidate data from multiple sources, offering a comprehensive business view for improved decision-making.
- Enhanced Data Quality. The ETL process ensures clean and consistent data entry, crucial for accurate analysis.
- Historical Analysis. Storing historical data enables trend analysis over time, informing future strategies.
- Improved Efficiency. Data Warehouses enable swift access and analysis of relevant data, enhancing efficiency and productivity.
Challenges
- Complexity. Designing and implementing a Data Warehouse can be complex and time-consuming.
- Cost. The cost of hardware, software, and specialised personnel can be significant.
- Data Security. Storing large amounts of sensitive data in one place poses security risks, requiring robust security measures.
Data Lake
A Data Lake is a centralised repository for storing, processing, and securing large volumes of structured and unstructured data. Unlike traditional Data Warehouses, which are structured and optimised for analytics with predefined schemas, Data Lakes retain raw data in its native format. This flexibility in data usage and analysis makes them crucial in modern data architecture, particularly in the age of big data and cloud.
Core Characteristics
- Schema-on-Read Approach. This means the data structure is not defined until the data is read for analysis. This offers more flexible data storage compared to the schema-on-write approach of Data Warehouses.
- Support for Multiple Data Types. Data Lakes accommodate diverse data types, including structured (like databases), semi-structured (like JSON, XML files), unstructured (like text and multimedia files), and binary data.
- Scalability. Designed to handle vast amounts of data, Data Lakes can easily scale up or down based on storage needs and computational demands, making them ideal for big data applications.
- Versatility. Data Lakes support various data operations, including batch processing, real-time analytics, machine learning, and data visualisation, providing a versatile platform for data science and analytics.
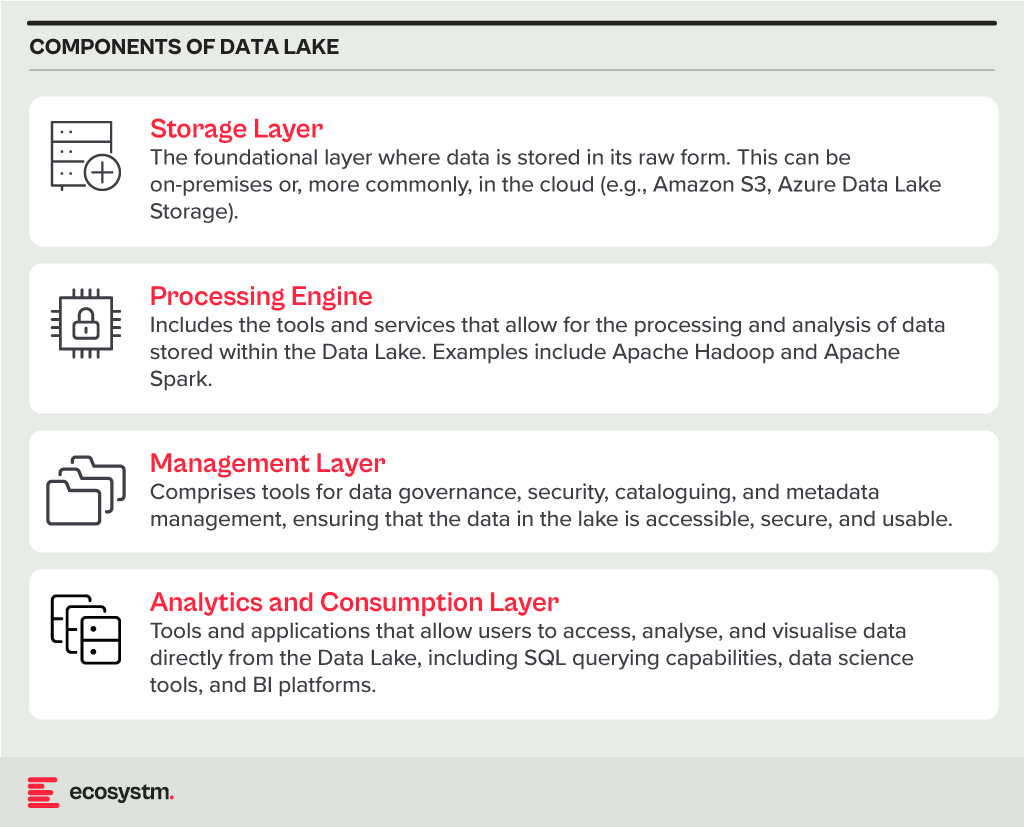
Benefits
- Flexibility. Data Lakes offer diverse storage formats and a schema-on-read approach for flexible analysis.
- Cost-Effectiveness. Cloud-hosted Data Lakes are cost-effective with scalable storage solutions.
- Advanced Analytics Capabilities. The raw, granular data in Data Lakes is ideal for advanced analytics, machine learning, and AI applications, providing deeper insights than traditional data warehouses.
Challenges
- Complexity and Management. Without proper management, a Data Lake can quickly become a “Data Swamp” where data is disorganised and unusable.
- Data Quality and Governance. Ensuring the quality and governance of data within a Data Lake can be challenging, requiring robust processes and tools.
- Security. Protecting sensitive data within a Data Lake is crucial, requiring comprehensive security measures.
Data Lakehouse
A Data Lakehouse is an innovative data management system that merges the strengths of Data Lakes and Data Warehouses. This hybrid approach strives to offer the adaptability and expansiveness of a Data Lake for housing extensive volumes of raw, unstructured data, while also providing the structured, refined data functionalities typical of a Data Warehouse. By bridging the gap between these two traditional data storage paradigms, Lakehouses enable more efficient data analytics, machine learning, and business intelligence operations across diverse data types and use cases.
Core Characteristics
- Unified Data Management. A Lakehouse streamlines data governance and security by managing both structured and unstructured data on one platform, reducing organizational data silos.
- Schema Flexibility. It supports schema-on-read and schema-on-write, allowing data to be stored and analysed flexibly. Data can be ingested in raw form and structured later or structured at ingestion.
- Scalability and Performance. Lakehouses scale storage and compute resources independently, handling large data volumes and complex analytics without performance compromise.
- Advanced Analytics and Machine Learning Integration. By providing direct access to both raw and processed data on a unified platform, Lakehouses facilitate advanced analytics, real-time analytics, and machine learning.
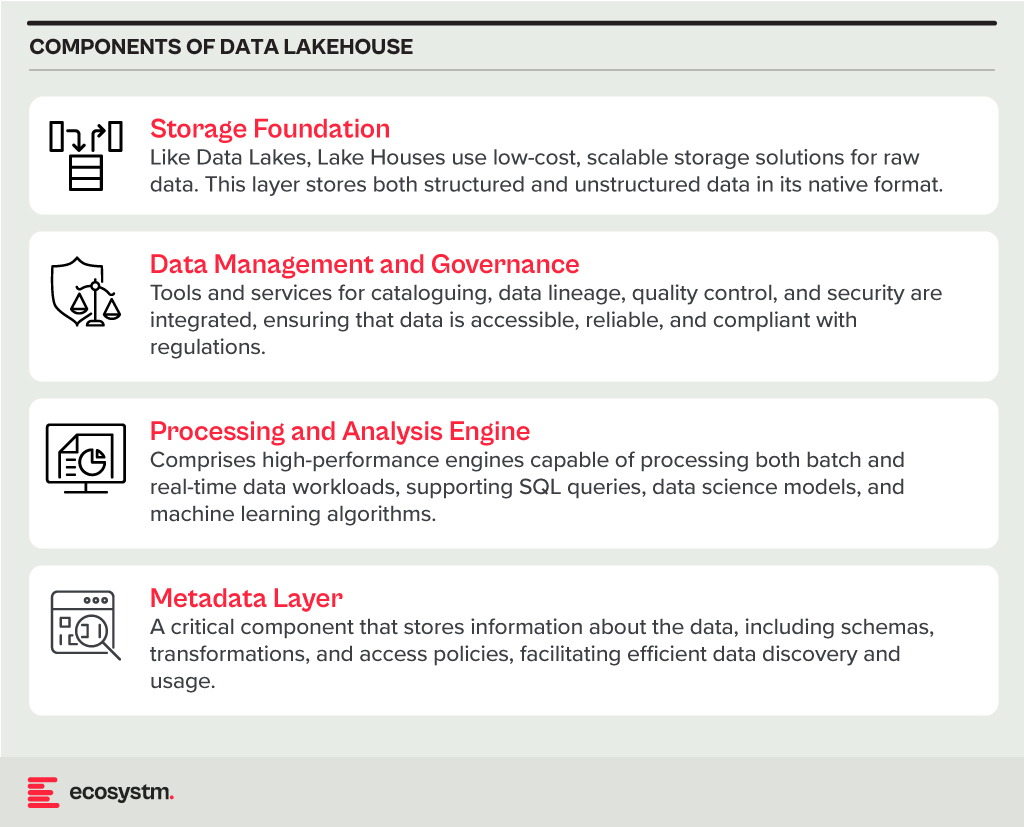
Benefits
- Versatility in Data Analysis. Lakehouses support diverse data analytics, spanning from traditional BI to advanced machine learning, all within one platform.
- Cost-Effective Scalability. The ability to scale storage and compute independently, often in a cloud environment, makes Lakehouses cost-effective for growing data needs.
- Improved Data Governance. Centralising data management enhances governance, security, and quality across all types of data.
Challenges
- Complexity in Implementation. Designing and implementing a Lakehouse architecture can be complex, requiring expertise in both Data Lakes and Data Warehouses.
- Data Consistency and Quality. Though crucial for reliable analytics, ensuring data consistency and quality across diverse data types and sources can be challenging.
- Governance and Security. Comprehensive data governance and security strategies are required to protect sensitive information and comply with regulations.
The choice between Data Warehouse, Data Lake, or Lakehouse systems is pivotal for businesses in harnessing the power of their data. Each option offers distinct advantages and challenges, requiring careful consideration of organisational needs and goals. By embracing the right data management system, organisations can pave the way for informed decision-making, operational efficiency, and innovation in the digital age.
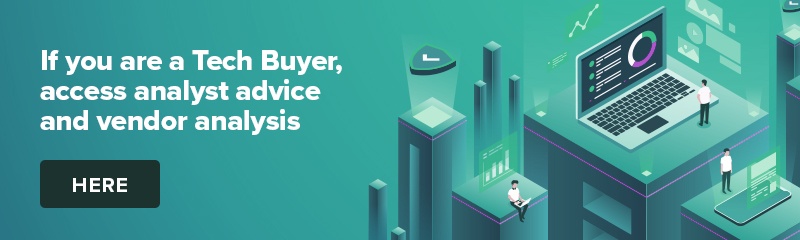
In my previous Ecosystm Insights, I covered how to choose the right database for the success of any application or project. Often organisations select cloud-based databases for the scalability, flexibility, and cost-effectiveness.
Here’s a look at some prominent cloud-based databases and guidance on the right cloud-based database for your organisational needs.
Click here to download ‘Databases Demystified. Cloud-Based Databases’ as a PDF.
Amazon RDS (Relational Database Service)
Pros.
Managed Service. Automates database setup, maintenance, and scaling, allowing you to focus on application development.
Scalability. Easily scales database’s compute and storage resources with minimal downtime.
Variety of DB Engines. Supports multiple database engines, including MySQL, PostgreSQL, MariaDB, Oracle, and SQL Server.
Cons.
Cost. Can be expensive for larger databases or high-throughput applications.
Complex Pricing. The pricing model can be complex to understand, with costs for storage, I/O, and data transfer.
Google Cloud SQL
Pros.
Fully Managed. Takes care of database management tasks like replication, patch management, and backups.
Integration. Seamlessly integrates with other GCP services, enhancing data analytics and machine learning capabilities.
Security. Offers robust security features, including data encryption at rest and in transit.
Cons.
Limited Customisation. Compared to managing your own database, there are limitations on configurations and fine-tuning.
Egress Costs. Data transfer costs (especially egress) can add up if you have high data movement needs.
Azure SQL Database
Pros.
Highly Scalable. Offers a scalable service that can dynamically adapt to your application’s needs.
Advanced Features. Includes advanced security features, AI-based performance optimisation, and automated updates.
Integration. Deep integration with other Azure services and Microsoft products.
Cons.
Learning Curve. The wide array of options and settings might be overwhelming for new users.
Cost for High Performance. Higher-tier performance levels can become costly.
MongoDB Atlas
Pros.
Flexibility. Offers a flexible document database that is ideal for unstructured data.
Global Clusters. Supports global clusters to improve access speeds for distributed applications.
Fully Managed. Provides a fully managed service, including automated backups, patches, and security.
Cons.
Cost at Scale. While it offers a free tier, costs can grow significantly with larger deployments and higher performance requirements.
Indexing Limitations. Efficient querying requires proper indexing, which can become complex as your dataset grows.
Amazon DynamoDB
Pros.
Serverless. Offers a serverless NoSQL database that scales automatically with your application’s demands.
Performance. Delivers single-digit millisecond performance at any scale.
Durability and Availability. Provides built-in security, backup, restore, and in-memory caching for internet-scale applications.
Cons.
Pricing Model. Pricing can be complex and expensive, especially for read/write throughput and storage.
Learning Curve. Different from traditional SQL databases, requiring time to learn best practices for data modeling and querying.
Selection Considerations
Data Model Compatibility. Ensure the database supports the data model you plan to use (relational, document, key-value, etc.).
Scalability and Performance Needs. Assess whether the database can meet your application’s scalability and performance requirements.
Cost. Understand the pricing model and estimate monthly costs based on your expected usage.
Security and Compliance. Check for security features and compliance with regulations relevant to your industry.
Integration with Existing Tools. Consider how well the database integrates with your current application ecosystem and development tools.
Vendor Lock-in. Be aware of the potential for vendor lock-in and consider the ease of migrating data to other services if needed.
Choosing the right cloud-based database involves balancing these factors to find the best fit for your application’s requirements and your organisation’s budget and skills.
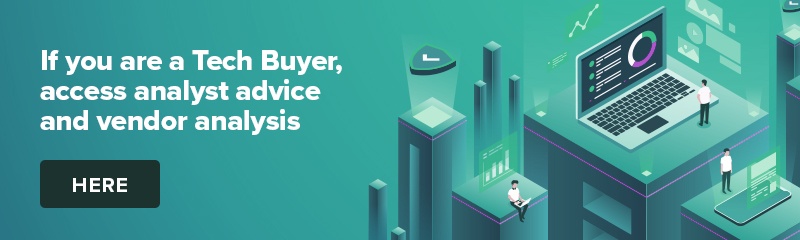
In my last Ecosystm Insights, I outlined various database options available to you. The challenge lies in selecting the right one. Selecting the right database is crucial for the success of any application or project. It involves understanding your data, the operations you’ll perform, scalability requirements, and more. Here is a guide that will walk you through key considerations and steps to choose the most suitable database from the list I shared last week.
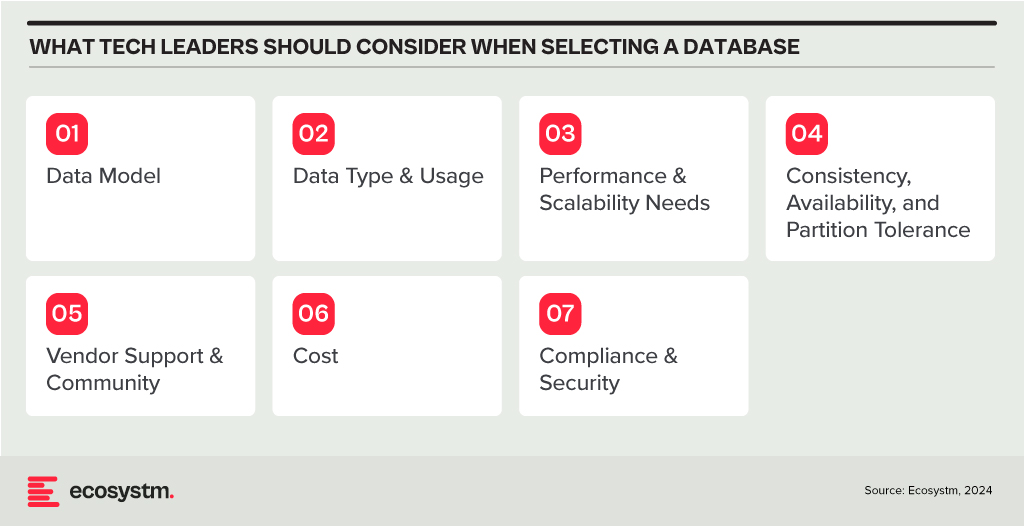
Understand Your Data Model
Relational (RDBMS) vs. NoSQL. Choose RDBMS if your data is structured and relational, requiring complex queries and transactions with ACID (Atomicity, Consistency, Isolation, Durability) properties. Opt for NoSQL if you have unstructured or semi-structured data, need to scale horizontally, or require flexibility in your schema design.
Consider the Data Type and Usage
Document Databases are ideal for storing, retrieving, and managing document-oriented information. They’re great for content management systems, ecommerce applications, and handling semi-structured data like JSON, XML.
Key-Value Stores shine in scenarios where quick access to data is needed through a key. They’re perfect for caching and storing user sessions, configurations, or any scenario where the lookup is based on a unique key.
Wide-Column Stores offer flexibility and scalability for storing and querying large volumes of data across many servers, suitable for big data applications, real-time analytics, and high-speed transactions.
Graph Databases are designed for data intensely connected through relationships, ideal for social networks, recommendation engines, and fraud detection systems where relationships between data points are key.
Time-Series Databases are optimised for storing and querying sequential data points indexed in time order. Use them for monitoring systems, IoT applications, and financial trading systems where time-stamped data is critical.
Spatial Databases support spatial data types and queries, making them suitable for geographic information systems (GIS), location-based services, and applications requiring spatial indexing and querying capabilities.
Assess Performance and Scalability Needs
In-Memory Databases like Redis offer high throughput and low latency for scenarios requiring rapid access to data, such as caching, session storage, and real-time analytics.
Distributed Databases like Cassandra or CouchDB are designed to run across multiple machines, offering high availability, fault tolerance, and scalability for applications with global reach and massive scale.
Evaluate Consistency, Availability, and Partition Tolerance (CAP Theorem)
Understand the trade-offs between consistency, availability, and partition tolerance. For example, if your application requires strong consistency, consider databases that prioritise consistency and partition tolerance (CP) like MongoDB or relational databases. If availability is paramount, look towards databases that offer availability and partition tolerance (AP) like Cassandra or CouchDB.
Other Considerations
Check for Vendor Support and Community. Evaluate the support and stability offered by vendors or open-source communities. Established products like Oracle Database, Microsoft SQL Server, and open-source options like PostgreSQL and MongoDB have robust support and active communities.
Cost. Consider both initial and long-term costs, including licenses, hardware, maintenance, and scalability. Open-source databases can reduce upfront costs, but ensure you account for support and operational expenses.
Compliance and Security. Ensure the database complies with relevant regulations (GDPR, HIPAA, etc.) and offers robust security features to protect sensitive data.
Try Before You Decide. Prototype your application with shortlisted databases to evaluate their performance, ease of use, and compatibility with your application’s requirements.
Conclusion
Selecting the right database is a strategic decision that impacts your application’s functionality, performance, and scalability. By carefully considering your data model, type of data, performance needs, and other factors like cost, support, and security, you can identify the database that best fits your project’s needs. Always stay informed about the latest developments in database technologies to make educated decisions as your requirements evolve.
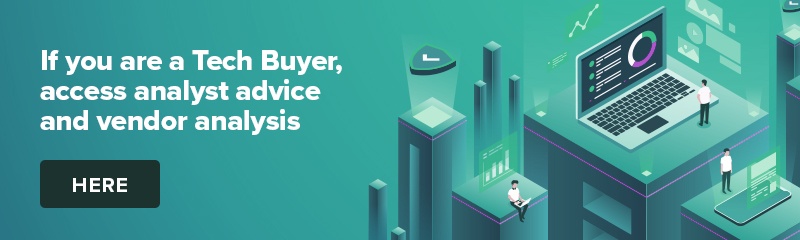