In my previous blogs, I outlined strategies for public sector organisations to incorporate technology into citizen services and internal processes. Building on those perspectives, let’s talk about the critical role of data in powering digital transformation across the public sector.
Effectively leveraging data is integral to delivering enhanced digital services and streamlining operations. Organisations must adopt a forward-looking roadmap that accounts for different data maturity levels – from core data foundations and emerging catalysts to future-state capabilities.
1. Data Essentials: Establishing the Bedrock
Data model. At the core of developing government e-services portals, strategic data modelling establishes the initial groundwork for scalable data infrastructures that can support future analytics, AI, and reporting needs. Effective data models define how information will be structured and analysed as data volumes grow. Beginning with an Entity-Relationship model, these blueprints guide the implementation of database schemas within database management systems (DBMS). This foundational approach ensures that the data infrastructure can accommodate the vast amounts of data generated by public services, crucial for maintaining public trust in government systems.
Cloud Databases. Cloud databases provide flexible, scalable, and cost-effective storage solutions, allowing public sector organisations to handle vast amounts of data generated by public services. Data warehouses, on the other hand, are centralised repositories designed to store structured data, enabling advanced querying and reporting capabilities. This combination allows for robust data analytics and AI-driven insights, ensuring that the data infrastructure can support future growth and evolving analytical needs.
Document management. Incorporating a document or records management system (DMS/RMS) early in the data portfolio of a government e-services portal is crucial for efficient operations. This system organises extensive paperwork and records like applications, permits, and legal documents systematically. It ensures easy storage, retrieval, and management, preventing issues with misplaced documents.
Emerging Catalysts: Unleashing Data’s Potential
Digital Twins. A digital twin is a sophisticated virtual model of a physical object or system. It surpasses traditional reporting methods through advanced analytics, including predictive insights and data mining. By creating detailed virtual replicas of infrastructure, utilities, and public services, digital twins allow for real-time monitoring, efficient resource management, and proactive maintenance. This holistic approach contributes to more efficient, sustainable, and livable cities, aligning with broader goals of urban development and environmental sustainability.
Data Fabric. Data Fabric, including Data Lakes and Data Lakehouses, represents a significant leap in managing complex data environments. It ensures data is accessible for various analyses and processing needs across platforms. Data Lakes store raw data in its original format, crucial for initial data collection when future data uses are uncertain. In Cloud DB or Data Fabric setups, Data Lakes play a foundational role by storing unprocessed or semi-structured data. Data Lakehouses combine Data Lakes’ storage with data warehouses’ querying capabilities, offering flexibility, and efficiency for handling different types of data in sophisticated environments.
Data Exchange and MOUs. Even with advanced data management technologies like data fabrics, Data Lakes, and Data Lakehouses, achieving higher maturity in digital government ecosystems often depends on establishing data-sharing agreements. Memorandums of Understanding (MoUs) exemplify these agreements, crucial for maximising efficiency and collaboration. MoUs outline terms, conditions, and protocols for sharing data beyond regulatory requirements, defining its scope, permitted uses, governance standards, and responsibilities of each party. This alignment ensures data integrity, privacy, and security while facilitating collaboration that enhances innovation and service delivery. Such agreements also pave the way for potential commercialisation of shared data resources, opening new market opportunities.
Future-Forward Capabilities: Pioneering New Frontiers
Data Mesh. Data Mesh is a decentralised approach to data architecture and organisational design, ideal for complex stakeholder ecosystems like digital conveyancing solutions. Unlike centralised models, Data Mesh allows each domain to manage its data independently. This fosters collaboration while ensuring secure and governed data sharing, essential for efficient conveyancing processes. Data Mesh enhances data quality and relevance by holding stakeholders directly accountable for their data, promoting integrity and adaptability to market changes. Its focus on interoperability and self-service data access enhances user satisfaction and operational efficiency, catering flexibly to diverse user needs within the conveyancing ecosystem.
Data Embassies. A Data Embassy stores and processes data in a foreign country under the legal jurisdiction of its origin country, beneficial for digital conveyancing solutions serving international markets. This approach ensures data security and sovereignty, governed by the originating nation’s laws to uphold privacy and legal integrity in conveyancing transactions. Data Embassies enhance resilience against physical and cyber threats by distributing data across international locations, ensuring continuous operation despite disruptions. They also foster international collaboration and trust, potentially attracting more investment and participation in global real estate markets. Technologically, Data Embassies rely on advanced data centres, encryption, cybersecurity, cloud, and robust disaster recovery solutions to maintain uninterrupted conveyancing services and compliance with global standards.
Conclusion
By developing a cohesive roadmap that progressively integrates cutting-edge architectures, cross-stakeholder partnerships, and avant-garde juridical models, agencies can construct a solid data ecosystem. One where information doesn’t just endure disruption, but actively facilitates organisational resilience and accelerates mission impact. Investing in an evolutionary data strategy today lays the crucial groundwork for delivering intelligent, insight-driven public services for decades to come. The time to fortify data’s transformative potential is now.
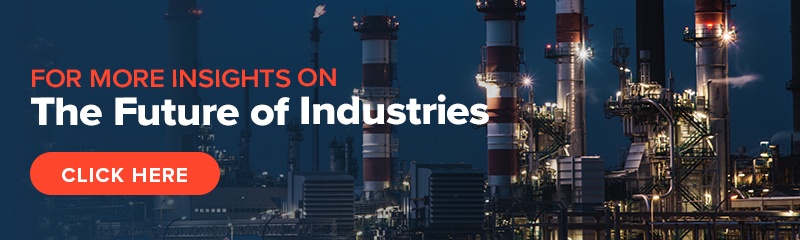
The data architecture outlines how data is managed in an organisation and is crucial for defining the data flow, data management systems required, the data processing operations, and AI applications. Data architects and engineers define data models and structures based on these requirements, supporting initiatives like data science. Before we delve into the right data architecture for your AI journey, let’s talk about the data management options. Technology leaders have the challenge of deciding on a data management system that takes into consideration factors such as current and future data needs, available skills, costs, and scalability. As data strategies become vital to business success, selecting the right data management system is crucial for enabling data-driven decisions and innovation.
Data Warehouse
A Data Warehouse is a centralised repository that stores vast amounts of data from diverse sources within an organisation. Its main function is to support reporting and data analysis, aiding businesses in making informed decisions. This concept encompasses both data storage and the consolidation and management of data from various sources to offer valuable business insights. Data Warehousing evolves alongside technological advancements, with trends like cloud-based solutions, real-time capabilities, and the integration of AI and machine learning for predictive analytics shaping its future.
Core Characteristics
- Integrated. It integrates data from multiple sources, ensuring consistent definitions and formats. This often includes data cleansing and transformation for analysis suitability.
- Subject-Oriented. Unlike operational databases, which prioritise transaction processing, it is structured around key business subjects like customers, products, and sales. This organisation facilitates complex queries and analysis.
- Non-Volatile. Data in a Data Warehouse is stable; once entered, it is not deleted. Historical data is retained for analysis, allowing for trend identification over time.
- Time-Variant. It retains historical data for trend analysis across various time periods. Each entry is time-stamped, enabling change tracking and trend analysis.
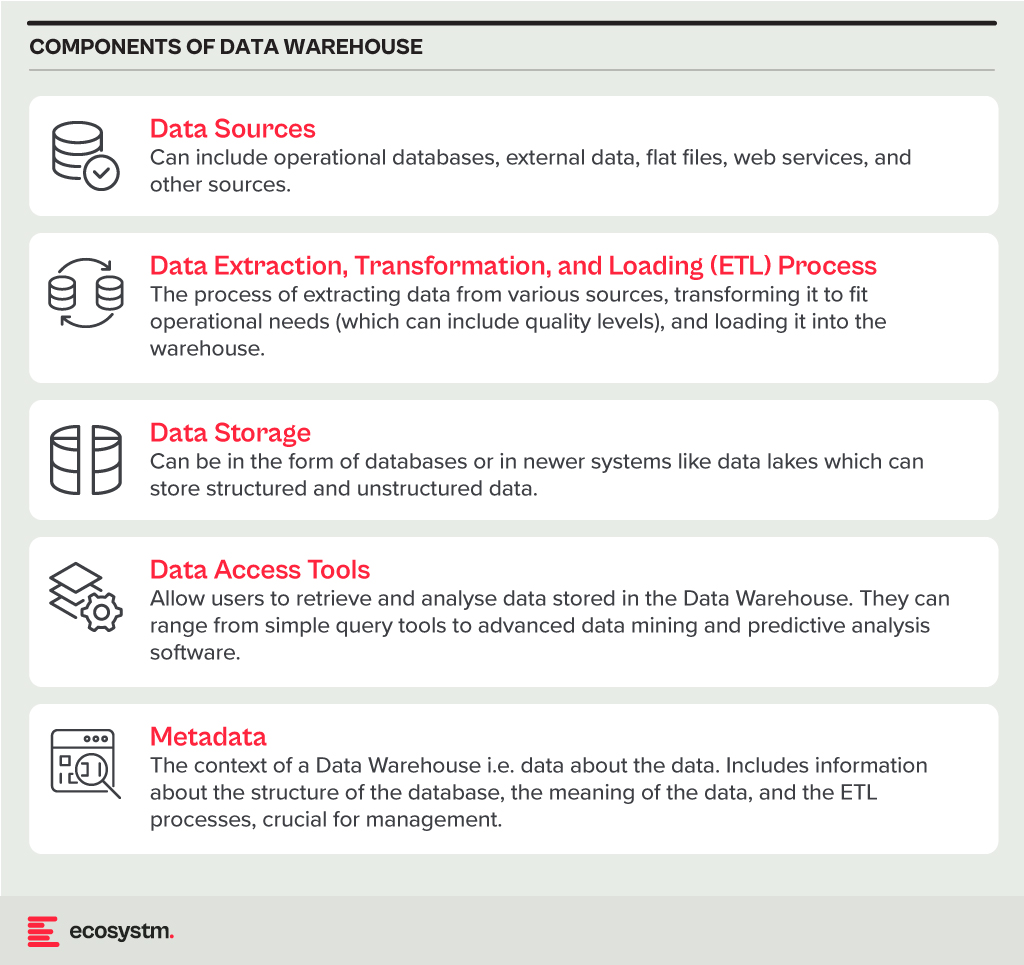
Benefits
- Better Decision Making. Data Warehouses consolidate data from multiple sources, offering a comprehensive business view for improved decision-making.
- Enhanced Data Quality. The ETL process ensures clean and consistent data entry, crucial for accurate analysis.
- Historical Analysis. Storing historical data enables trend analysis over time, informing future strategies.
- Improved Efficiency. Data Warehouses enable swift access and analysis of relevant data, enhancing efficiency and productivity.
Challenges
- Complexity. Designing and implementing a Data Warehouse can be complex and time-consuming.
- Cost. The cost of hardware, software, and specialised personnel can be significant.
- Data Security. Storing large amounts of sensitive data in one place poses security risks, requiring robust security measures.
Data Lake
A Data Lake is a centralised repository for storing, processing, and securing large volumes of structured and unstructured data. Unlike traditional Data Warehouses, which are structured and optimised for analytics with predefined schemas, Data Lakes retain raw data in its native format. This flexibility in data usage and analysis makes them crucial in modern data architecture, particularly in the age of big data and cloud.
Core Characteristics
- Schema-on-Read Approach. This means the data structure is not defined until the data is read for analysis. This offers more flexible data storage compared to the schema-on-write approach of Data Warehouses.
- Support for Multiple Data Types. Data Lakes accommodate diverse data types, including structured (like databases), semi-structured (like JSON, XML files), unstructured (like text and multimedia files), and binary data.
- Scalability. Designed to handle vast amounts of data, Data Lakes can easily scale up or down based on storage needs and computational demands, making them ideal for big data applications.
- Versatility. Data Lakes support various data operations, including batch processing, real-time analytics, machine learning, and data visualisation, providing a versatile platform for data science and analytics.
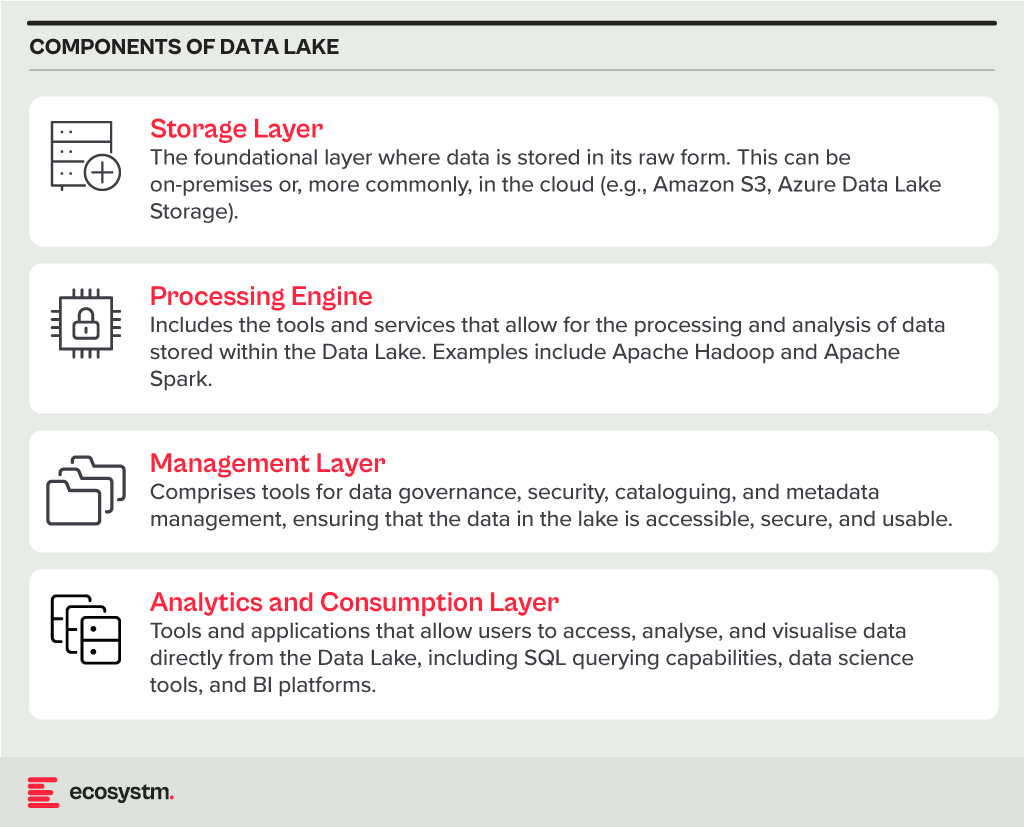
Benefits
- Flexibility. Data Lakes offer diverse storage formats and a schema-on-read approach for flexible analysis.
- Cost-Effectiveness. Cloud-hosted Data Lakes are cost-effective with scalable storage solutions.
- Advanced Analytics Capabilities. The raw, granular data in Data Lakes is ideal for advanced analytics, machine learning, and AI applications, providing deeper insights than traditional data warehouses.
Challenges
- Complexity and Management. Without proper management, a Data Lake can quickly become a “Data Swamp” where data is disorganised and unusable.
- Data Quality and Governance. Ensuring the quality and governance of data within a Data Lake can be challenging, requiring robust processes and tools.
- Security. Protecting sensitive data within a Data Lake is crucial, requiring comprehensive security measures.
Data Lakehouse
A Data Lakehouse is an innovative data management system that merges the strengths of Data Lakes and Data Warehouses. This hybrid approach strives to offer the adaptability and expansiveness of a Data Lake for housing extensive volumes of raw, unstructured data, while also providing the structured, refined data functionalities typical of a Data Warehouse. By bridging the gap between these two traditional data storage paradigms, Lakehouses enable more efficient data analytics, machine learning, and business intelligence operations across diverse data types and use cases.
Core Characteristics
- Unified Data Management. A Lakehouse streamlines data governance and security by managing both structured and unstructured data on one platform, reducing organizational data silos.
- Schema Flexibility. It supports schema-on-read and schema-on-write, allowing data to be stored and analysed flexibly. Data can be ingested in raw form and structured later or structured at ingestion.
- Scalability and Performance. Lakehouses scale storage and compute resources independently, handling large data volumes and complex analytics without performance compromise.
- Advanced Analytics and Machine Learning Integration. By providing direct access to both raw and processed data on a unified platform, Lakehouses facilitate advanced analytics, real-time analytics, and machine learning.
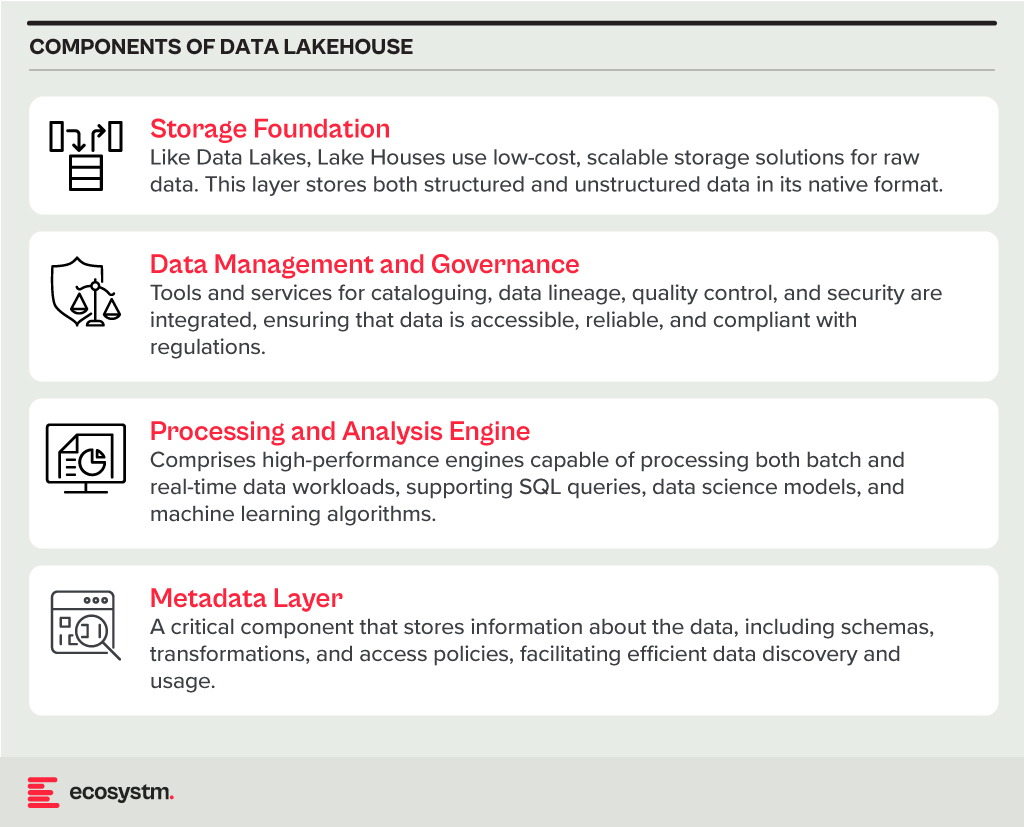
Benefits
- Versatility in Data Analysis. Lakehouses support diverse data analytics, spanning from traditional BI to advanced machine learning, all within one platform.
- Cost-Effective Scalability. The ability to scale storage and compute independently, often in a cloud environment, makes Lakehouses cost-effective for growing data needs.
- Improved Data Governance. Centralising data management enhances governance, security, and quality across all types of data.
Challenges
- Complexity in Implementation. Designing and implementing a Lakehouse architecture can be complex, requiring expertise in both Data Lakes and Data Warehouses.
- Data Consistency and Quality. Though crucial for reliable analytics, ensuring data consistency and quality across diverse data types and sources can be challenging.
- Governance and Security. Comprehensive data governance and security strategies are required to protect sensitive information and comply with regulations.
The choice between Data Warehouse, Data Lake, or Lakehouse systems is pivotal for businesses in harnessing the power of their data. Each option offers distinct advantages and challenges, requiring careful consideration of organisational needs and goals. By embracing the right data management system, organisations can pave the way for informed decision-making, operational efficiency, and innovation in the digital age.
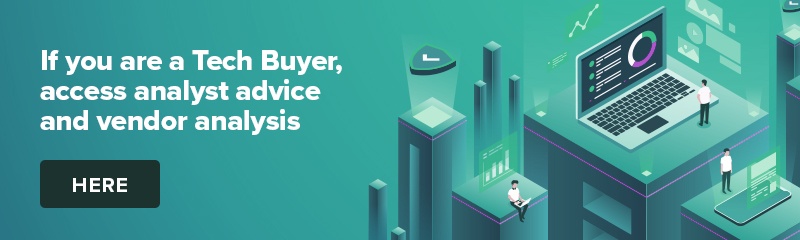