At a recently held Ecosystm roundtable, in partnership with Qlik and 121Connects, Ecosystm Principal Advisor Manoj Chugh, moderated a conversation where Indian tech and data leaders discussed building trust in data strategies. They explored ways to automate data pipelines and improve governance to drive better decisions and business outcomes. Here are the key takeaways from the session.

Data isn’t just a byproduct anymore; it’s the lifeblood of modern businesses, fuelling informed decisions and strategic growth. But with vast amounts of data, the challenge isn’t just managing it; it’s building trust. AI, once a beacon of hope, is now at risk without a reliable data foundation. Ecosystm research reveals that a staggering 66% of Indian tech leaders doubt their organisation’s data quality, and the problem of data silos is exacerbating this trust crisis.
At the Leaders Roundtable in Mumbai, I had the opportunity to moderate a discussion among data and digital leaders on the critical components of building trust in data and leveraging it to drive business value. The consensus was that building trust requires a comprehensive strategy that addresses the complexities of data management and positions the organisation for future success. Here are the key strategies that are essential for achieving these goals.
1. Adopting a Unified Data Approach
Organisations are facing a growing wave of complex workloads and business initiatives. To manage this expansion, IT teams are turning to multi-cloud, SaaS, and hybrid environments. However, this diverse landscape introduces new challenges, such as data silos, security vulnerabilities, and difficulties in ensuring interoperability between systems.

A unified data strategy is crucial to overcome these challenges. By ensuring platform consistency, robust security, and seamless data integration, organisations can simplify data management, enhance security, and align with business goals – driving informed decisions, innovation, and long-term success.
Real-time data integration is essential for timely data availability, enabling organisations to make data-driven decisions quickly and effectively. By integrating data from various sources in real-time, businesses can gain valuable insights into their operations, identify trends, and respond to changing market conditions.
Organisations that are able to integrate their IT and operational technology (OT) systems find their data accuracy increasing. By combining IT’s digital data management expertise with OT’s real-time operational insights, organisations can ensure more accurate, timely, and actionable data. This integration enables continuous monitoring and analysis of operational data, leading to faster identification of errors, more precise decision-making, and optimised processes.
2. Enhancing Data Quality with Automation and Collaboration
As the volume and complexity of data continue to grow, ensuring high data quality is essential for organisations to make accurate decisions and to drive trust in data-driven solutions. Automated data quality tools are useful for cleansing and standardising data to eliminate errors and inconsistencies.
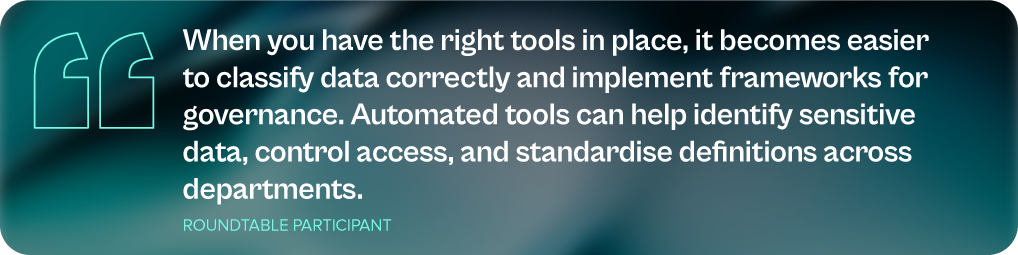
As mentioned earlier, integrating IT and OT systems can help organisations improve operational efficiency and resilience. By leveraging data-driven insights, businesses can identify bottlenecks, optimise workflows, and proactively address potential issues before they escalate. This can lead to cost savings, increased productivity, and improved customer satisfaction.
However, while automation technologies can help, organisations must also invest in training employees in data management, data visualisation, and data governance.
3. Modernising Data Infrastructure for Agility and Innovation
In today’s fast-paced business landscape, agility is paramount. Modernising data infrastructure is essential to remain competitive – the right digital infrastructure focuses on optimising costs, boosting capacity and agility, and maximising data leverage, all while safeguarding the organisation from cyber threats. This involves migrating data lakes and warehouses to cloud platforms and adopting advanced analytics tools. However, modernisation efforts must be aligned with specific business goals, such as enhancing customer experiences, optimising operations, or driving innovation. A well-modernised data environment not only improves agility but also lays the foundation for future innovations.

Technology leaders must assess whether their data architecture supports the organisation’s evolving data requirements, considering factors such as data flows, necessary management systems, processing operations, and AI applications. The ideal data architecture should be tailored to the organisation’s specific needs, considering current and future data demands, available skills, costs, and scalability.
4. Strengthening Data Governance with a Structured Approach
Data governance is crucial for establishing trust in data, and providing a framework to manage its quality, integrity, and security throughout its lifecycle. By setting clear policies and processes, organisations can build confidence in their data, support informed decision-making, and foster stakeholder trust.
A key component of data governance is data lineage – the ability to trace the history and transformation of data from its source to its final use. Understanding this journey helps organisations verify data accuracy and integrity, ensure compliance with regulatory requirements and internal policies, improve data quality by proactively addressing issues, and enhance decision-making through context and transparency.
A tiered data governance structure, with strategic oversight at the executive level and operational tasks managed by dedicated data governance councils, ensures that data governance aligns with broader organisational goals and is implemented effectively.
Are You Ready for the Future of AI?
The ultimate goal of your data management and discovery mechanisms is to ensure that you are advancing at pace with the industry. The analytics landscape is undergoing a profound transformation, promising to revolutionise how organisations interact with data. A key innovation, the data fabric, is enabling organisations to analyse unstructured data, where the true value often lies, resulting in cleaner and more reliable data models.
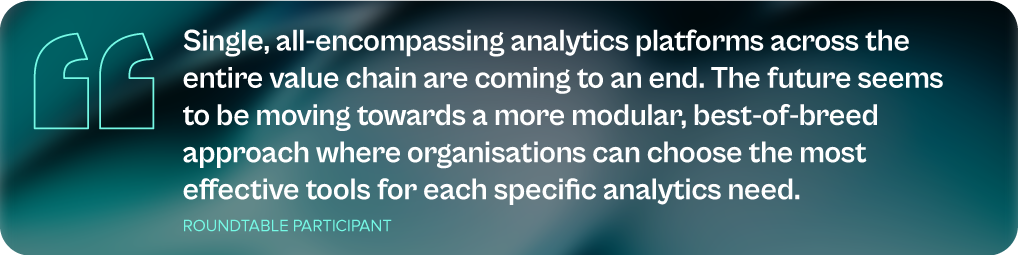
GenAI has emerged as another game-changer, empowering employees across the organisation to become citizen data scientists. This democratisation of data analytics allows for a broader range of insights and fosters a more data-driven culture. Organisations can leverage GenAI to automate tasks, generate new ideas, and uncover hidden patterns in their data.
The shift from traditional dashboards to real-time conversational tools is also reshaping how data insights are delivered and acted upon. These tools enable users to ask questions in natural language, receiving immediate and relevant answers based on the underlying data. This conversational approach makes data more accessible and actionable, empowering employees to make data-driven decisions at all levels of the organisation.
To fully capitalise on these advancements, organisations need to reassess their AI/ML strategies. By ensuring that their tech initiatives align with their broader business objectives and deliver tangible returns on investment, organisations can unlock the full potential of data-driven insights and gain a competitive edge. It is equally important to build trust in AI initiatives, through a strong data foundation. This involves ensuring data quality, accuracy, and consistency, as well as implementing robust data governance practices. A solid data foundation provides the necessary groundwork for AI and GenAI models to deliver reliable and valuable insights.
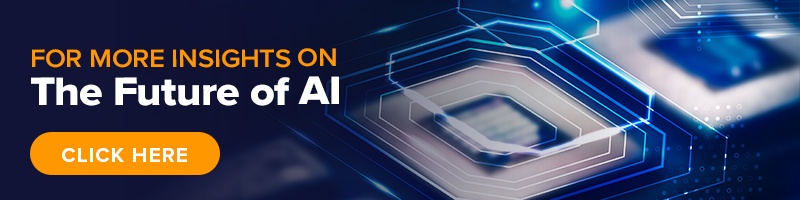