Over the past year of moderating AI roundtables, I’ve had a front-row seat to how the conversation has evolved. Early discussions often centred on identifying promising use cases and grappling with the foundational work, particularly around data readiness. More recently, attention has shifted to emerging capabilities like Agentic AI and what they mean for enterprise workflows. The pace of change has been rapid, but one theme has remained consistent throughout: ROI.
What’s changed is the depth and nuance of that conversation. As AI moves from pilot projects to core business functions, the question is no longer just if it delivers value, but how to measure it in a way that captures its true impact. Traditional ROI frameworks, focused on immediate, measurable returns, are proving inadequate when applied to AI initiatives that reshape processes, unlock new capabilities, and require long-term investment.
To navigate this complexity, organisations need a more grounded, forward-looking approach that considers not only direct gains but also enablement, scalability, and strategic relevance. Getting this right is key to both validating today’s investments and setting the stage for meaningful, sustained transformation.
Here is a summary of the key thoughts around AI ROI from multiple conversations across the Asia Pacific region.
1. Redefining ROI Beyond Short-Term Wins
A common mistake when adopting AI is using traditional ROI models that expect quick, obvious wins like cutting costs or boosting revenue right away. But AI works differently. Its real value often shows up slowly, through better decision-making, greater agility, and preparing the organisation to compete long-term.
AI projects need big upfront investments in things like improving data quality, upgrading infrastructure, and managing change. These costs are clear from the start, while the bigger benefits, like smarter predictions, faster processes, and a stronger competitive edge, usually take years to really pay off and aren’t easy to measure the usual way.
Ecosystm research finds that 60% of organisations in Asia Pacific expect to see AI ROI over two to five years, not immediately.
The most successful AI adopters get this and have started changing how they measure ROI. They look beyond just money and track things like explainability (which builds trust and helps with regulations), compliance improvements, how AI helps employees work better, and how it sparks new products or business models. These less obvious benefits are actually key to building strong, AI-ready organisations that can keep innovating and growing over time.
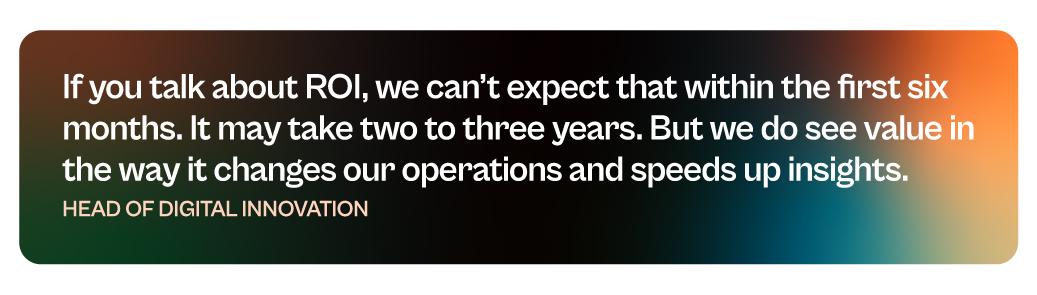
2. Linking AI to High-Impact KPIs: Problem First, Not Tech First
Successful AI initiatives always start with a clearly defined business problem or opportunity; not the technology itself. When a precise pain point is identified upfront, AI shifts from a vague concept to a powerful solution.
An industrial firm in Asia Pacific reduced production lead time by 40% by applying AI to optimise inspection and scheduling. This result was concrete, measurable, and directly tied to business goals.
This problem-first approach ensures every AI use case links to high-impact KPIs – whether reducing downtime, improving product quality, or boosting customer satisfaction. While this short-to-medium-term focus on results might seem at odds with the long-term ROI perspective, the two are complementary. Early wins secure executive buy-in and funding, giving AI initiatives the runway needed to mature and scale for sustained strategic impact.
Together, these perspectives build a foundation for scalable AI value that balances immediate relevance with future resilience.
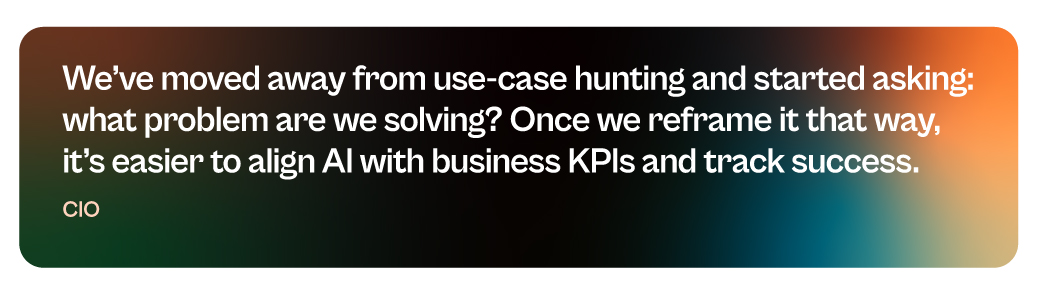
3. Tracking ROI Across the Lifecycle
A costly misconception is treating pilot projects as the final success marker. While pilots validate concepts, true ROI only begins once AI is integrated into operations, scaled organisation-wide, and sustained over time.
Ecosystm research reveals that only about 32% of organisations rigorously track AI outcomes with defined success metrics; most rely on ad-hoc or incomplete measures.
To capture real value, ROI must be measured across the full AI lifecycle. This includes infrastructure upgrades needed for scaling, ongoing model maintenance (retraining and tuning), strict data governance to ensure quality and compliance, and operational support to monitor and optimise deployed AI systems.
A lifecycle perspective acknowledges the real value – and hidden costs – emerge beyond pilots, ensuring organisations understand the total cost of ownership and sustained benefits.
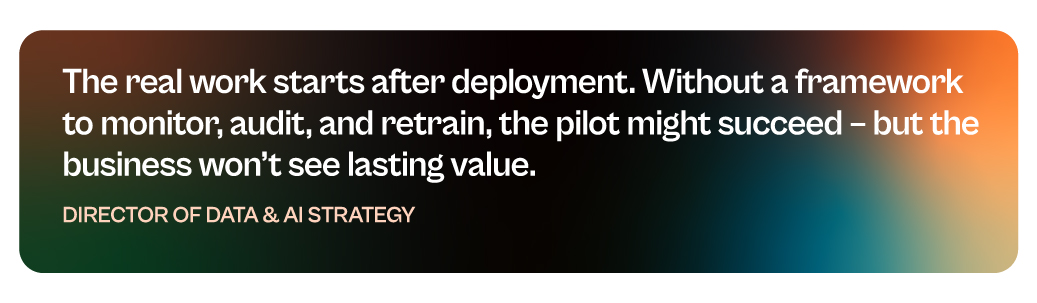
4. Strengthening the Foundations: Talent, Data, and Strategy
AI success hinges on strong foundations, not just models. Many projects fail due to gaps in skills, data quality, or strategic focus – directly blocking positive ROI and wasting resources.
Top organisations invest early in three pillars:
- Data Infrastructure. Reliable, scalable data pipelines and quality controls are vital. Poor data leads to delays, errors, higher costs, and compliance risks, hurting ROI.
- Skilled Talent. Cross-functional teams combining technical and domain expertise speed deployment, improve quality, reduce errors, and drive ongoing innovation – boosting ROI.
- Strategic Roadmap. Clear alignment with business goals ensures resources focus on high-impact projects, secures executive support, fosters collaboration, and enables measurable outcomes through KPIs.
Strengthening these fundamentals turns AI investments into consistent growth and competitive advantage.
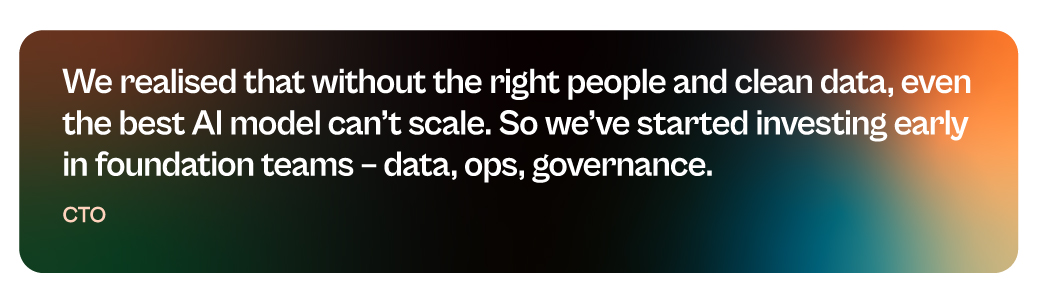
5. Navigating Tool Complexity: Toward Integrated AI Lifecycle Management
One of the biggest challenges in measuring AI ROI is tool fragmentation. The AI lifecycle spans multiple stages – data preparation, model development, deployment, monitoring, and impact tracking – and organisations often rely on different tools for each. MLOps platforms track model performance, BI tools measure KPIs, and governance tools ensure compliance, but these systems rarely connect seamlessly.
This disconnect creates blind spots. Metrics sit in silos, handoffs across teams become inefficient, and linking model performance to business outcomes over time becomes manual and error prone. As AI becomes more embedded in core operations, the need for integration is becoming clear.
To close this gap, organisations are adopting unified AI lifecycle management platforms. These solutions provide a centralised view of model health, usage, and business impact, enriched with governance and collaboration features. By aligning technical and business metrics, they enable faster iteration, responsible scaling, and clearer ROI across the lifecycle.
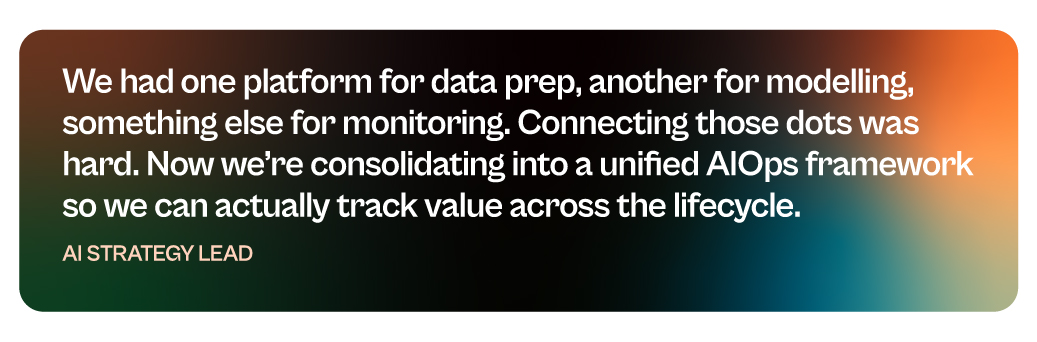
Final Thoughts: The Cost of Inaction
Measuring AI ROI isn’t just about proving cost savings; it’s a shift in how organisations think about value. AI delivers long-term gains through better decision-making, improved compliance, more empowered employees, and the capacity to innovate continuously.
Yet too often, the cost of doing nothing is overlooked. Failing to invest in AI leads to slower adaptation, inefficient processes, and lost competitive ground. Traditional ROI models, built for short-term, linear investments, don’t account for the strategic upside of early adoption or the risks of falling behind.
That’s why leading organisations are reframing the ROI conversation. They’re looking beyond isolated productivity metrics to focus on lasting outcomes: scalable governance, adaptable talent, and future-ready business models. In a fast-evolving environment, inaction carries its own cost – one that may not appear in today’s spreadsheet but will shape tomorrow’s performance.
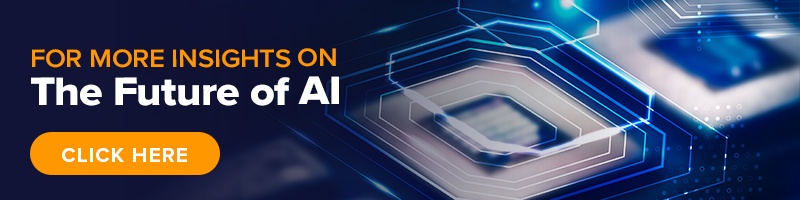
At a recently held Ecosystm roundtable, in partnership with Qlik and 121Connects, Ecosystm Principal Advisor Manoj Chugh, moderated a conversation where Indian tech and data leaders discussed building trust in data strategies. They explored ways to automate data pipelines and improve governance to drive better decisions and business outcomes. Here are the key takeaways from the session.

Data isn’t just a byproduct anymore; it’s the lifeblood of modern businesses, fuelling informed decisions and strategic growth. But with vast amounts of data, the challenge isn’t just managing it; it’s building trust. AI, once a beacon of hope, is now at risk without a reliable data foundation. Ecosystm research reveals that a staggering 66% of Indian tech leaders doubt their organisation’s data quality, and the problem of data silos is exacerbating this trust crisis.
At the Leaders Roundtable in Mumbai, I had the opportunity to moderate a discussion among data and digital leaders on the critical components of building trust in data and leveraging it to drive business value. The consensus was that building trust requires a comprehensive strategy that addresses the complexities of data management and positions the organisation for future success. Here are the key strategies that are essential for achieving these goals.
1. Adopting a Unified Data Approach
Organisations are facing a growing wave of complex workloads and business initiatives. To manage this expansion, IT teams are turning to multi-cloud, SaaS, and hybrid environments. However, this diverse landscape introduces new challenges, such as data silos, security vulnerabilities, and difficulties in ensuring interoperability between systems.

A unified data strategy is crucial to overcome these challenges. By ensuring platform consistency, robust security, and seamless data integration, organisations can simplify data management, enhance security, and align with business goals – driving informed decisions, innovation, and long-term success.
Real-time data integration is essential for timely data availability, enabling organisations to make data-driven decisions quickly and effectively. By integrating data from various sources in real-time, businesses can gain valuable insights into their operations, identify trends, and respond to changing market conditions.
Organisations that are able to integrate their IT and operational technology (OT) systems find their data accuracy increasing. By combining IT’s digital data management expertise with OT’s real-time operational insights, organisations can ensure more accurate, timely, and actionable data. This integration enables continuous monitoring and analysis of operational data, leading to faster identification of errors, more precise decision-making, and optimised processes.
2. Enhancing Data Quality with Automation and Collaboration
As the volume and complexity of data continue to grow, ensuring high data quality is essential for organisations to make accurate decisions and to drive trust in data-driven solutions. Automated data quality tools are useful for cleansing and standardising data to eliminate errors and inconsistencies.
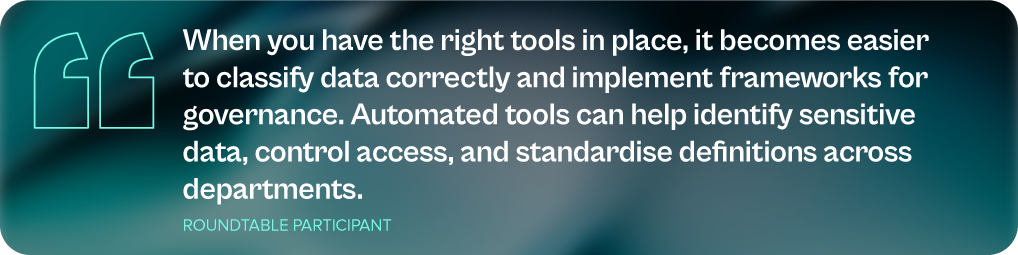
As mentioned earlier, integrating IT and OT systems can help organisations improve operational efficiency and resilience. By leveraging data-driven insights, businesses can identify bottlenecks, optimise workflows, and proactively address potential issues before they escalate. This can lead to cost savings, increased productivity, and improved customer satisfaction.
However, while automation technologies can help, organisations must also invest in training employees in data management, data visualisation, and data governance.
3. Modernising Data Infrastructure for Agility and Innovation
In today’s fast-paced business landscape, agility is paramount. Modernising data infrastructure is essential to remain competitive – the right digital infrastructure focuses on optimising costs, boosting capacity and agility, and maximising data leverage, all while safeguarding the organisation from cyber threats. This involves migrating data lakes and warehouses to cloud platforms and adopting advanced analytics tools. However, modernisation efforts must be aligned with specific business goals, such as enhancing customer experiences, optimising operations, or driving innovation. A well-modernised data environment not only improves agility but also lays the foundation for future innovations.

Technology leaders must assess whether their data architecture supports the organisation’s evolving data requirements, considering factors such as data flows, necessary management systems, processing operations, and AI applications. The ideal data architecture should be tailored to the organisation’s specific needs, considering current and future data demands, available skills, costs, and scalability.
4. Strengthening Data Governance with a Structured Approach
Data governance is crucial for establishing trust in data, and providing a framework to manage its quality, integrity, and security throughout its lifecycle. By setting clear policies and processes, organisations can build confidence in their data, support informed decision-making, and foster stakeholder trust.
A key component of data governance is data lineage – the ability to trace the history and transformation of data from its source to its final use. Understanding this journey helps organisations verify data accuracy and integrity, ensure compliance with regulatory requirements and internal policies, improve data quality by proactively addressing issues, and enhance decision-making through context and transparency.
A tiered data governance structure, with strategic oversight at the executive level and operational tasks managed by dedicated data governance councils, ensures that data governance aligns with broader organisational goals and is implemented effectively.
Are You Ready for the Future of AI?
The ultimate goal of your data management and discovery mechanisms is to ensure that you are advancing at pace with the industry. The analytics landscape is undergoing a profound transformation, promising to revolutionise how organisations interact with data. A key innovation, the data fabric, is enabling organisations to analyse unstructured data, where the true value often lies, resulting in cleaner and more reliable data models.
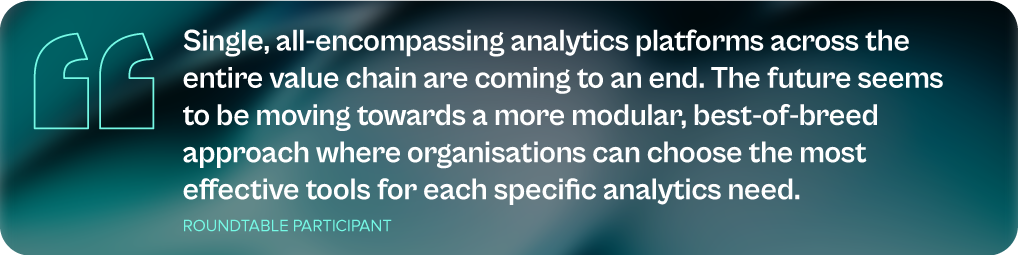
GenAI has emerged as another game-changer, empowering employees across the organisation to become citizen data scientists. This democratisation of data analytics allows for a broader range of insights and fosters a more data-driven culture. Organisations can leverage GenAI to automate tasks, generate new ideas, and uncover hidden patterns in their data.
The shift from traditional dashboards to real-time conversational tools is also reshaping how data insights are delivered and acted upon. These tools enable users to ask questions in natural language, receiving immediate and relevant answers based on the underlying data. This conversational approach makes data more accessible and actionable, empowering employees to make data-driven decisions at all levels of the organisation.
To fully capitalise on these advancements, organisations need to reassess their AI/ML strategies. By ensuring that their tech initiatives align with their broader business objectives and deliver tangible returns on investment, organisations can unlock the full potential of data-driven insights and gain a competitive edge. It is equally important to build trust in AI initiatives, through a strong data foundation. This involves ensuring data quality, accuracy, and consistency, as well as implementing robust data governance practices. A solid data foundation provides the necessary groundwork for AI and GenAI models to deliver reliable and valuable insights.
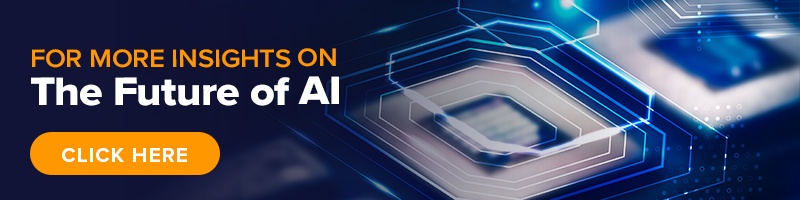
The data architecture outlines how data is managed in an organisation and is crucial for defining the data flow, data management systems required, the data processing operations, and AI applications. Data architects and engineers define data models and structures based on these requirements, supporting initiatives like data science. Before we delve into the right data architecture for your AI journey, let’s talk about the data management options. Technology leaders have the challenge of deciding on a data management system that takes into consideration factors such as current and future data needs, available skills, costs, and scalability. As data strategies become vital to business success, selecting the right data management system is crucial for enabling data-driven decisions and innovation.
Data Warehouse
A Data Warehouse is a centralised repository that stores vast amounts of data from diverse sources within an organisation. Its main function is to support reporting and data analysis, aiding businesses in making informed decisions. This concept encompasses both data storage and the consolidation and management of data from various sources to offer valuable business insights. Data Warehousing evolves alongside technological advancements, with trends like cloud-based solutions, real-time capabilities, and the integration of AI and machine learning for predictive analytics shaping its future.
Core Characteristics
- Integrated. It integrates data from multiple sources, ensuring consistent definitions and formats. This often includes data cleansing and transformation for analysis suitability.
- Subject-Oriented. Unlike operational databases, which prioritise transaction processing, it is structured around key business subjects like customers, products, and sales. This organisation facilitates complex queries and analysis.
- Non-Volatile. Data in a Data Warehouse is stable; once entered, it is not deleted. Historical data is retained for analysis, allowing for trend identification over time.
- Time-Variant. It retains historical data for trend analysis across various time periods. Each entry is time-stamped, enabling change tracking and trend analysis.
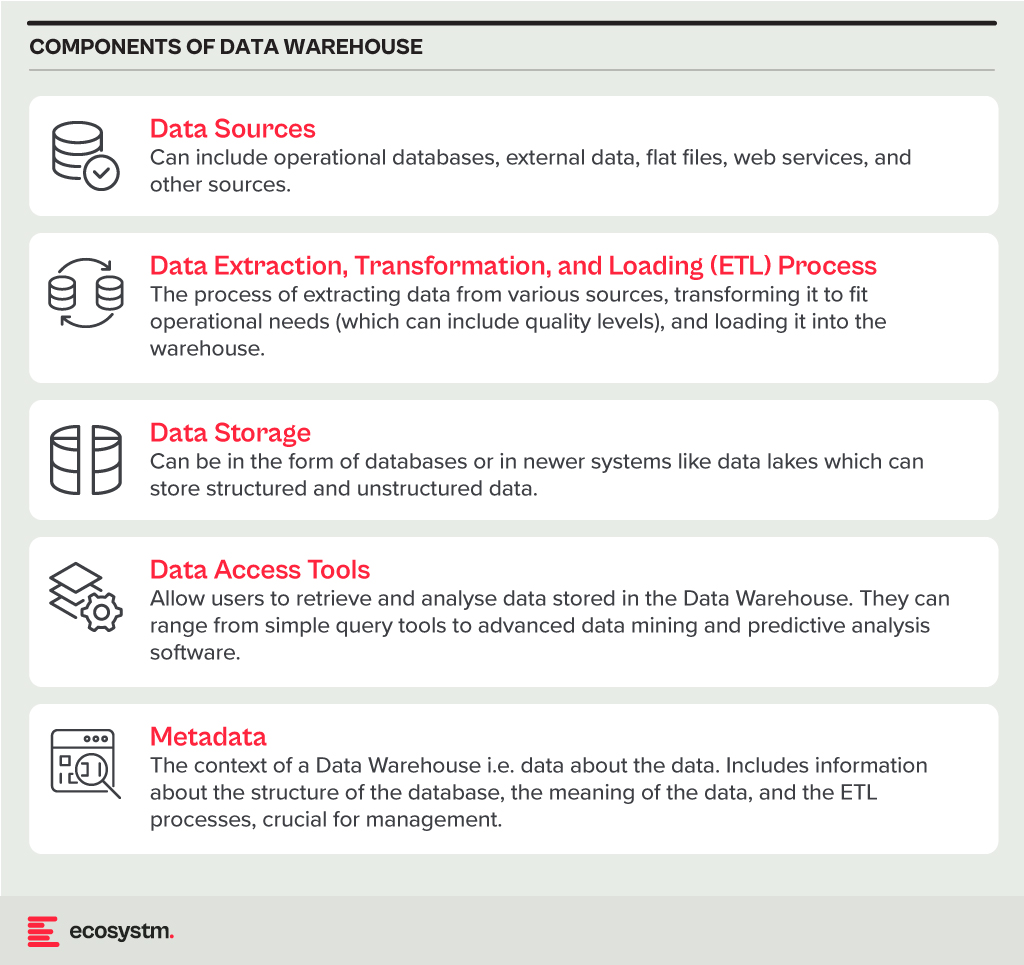
Benefits
- Better Decision Making. Data Warehouses consolidate data from multiple sources, offering a comprehensive business view for improved decision-making.
- Enhanced Data Quality. The ETL process ensures clean and consistent data entry, crucial for accurate analysis.
- Historical Analysis. Storing historical data enables trend analysis over time, informing future strategies.
- Improved Efficiency. Data Warehouses enable swift access and analysis of relevant data, enhancing efficiency and productivity.
Challenges
- Complexity. Designing and implementing a Data Warehouse can be complex and time-consuming.
- Cost. The cost of hardware, software, and specialised personnel can be significant.
- Data Security. Storing large amounts of sensitive data in one place poses security risks, requiring robust security measures.
Data Lake
A Data Lake is a centralised repository for storing, processing, and securing large volumes of structured and unstructured data. Unlike traditional Data Warehouses, which are structured and optimised for analytics with predefined schemas, Data Lakes retain raw data in its native format. This flexibility in data usage and analysis makes them crucial in modern data architecture, particularly in the age of big data and cloud.
Core Characteristics
- Schema-on-Read Approach. This means the data structure is not defined until the data is read for analysis. This offers more flexible data storage compared to the schema-on-write approach of Data Warehouses.
- Support for Multiple Data Types. Data Lakes accommodate diverse data types, including structured (like databases), semi-structured (like JSON, XML files), unstructured (like text and multimedia files), and binary data.
- Scalability. Designed to handle vast amounts of data, Data Lakes can easily scale up or down based on storage needs and computational demands, making them ideal for big data applications.
- Versatility. Data Lakes support various data operations, including batch processing, real-time analytics, machine learning, and data visualisation, providing a versatile platform for data science and analytics.
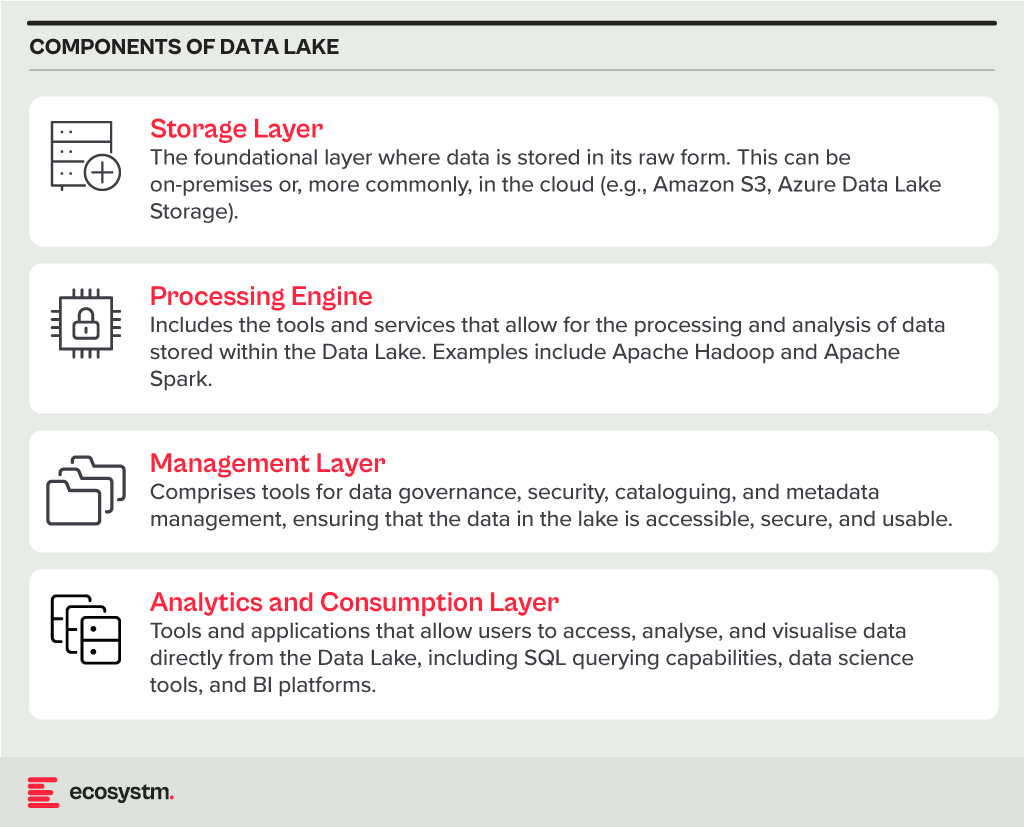
Benefits
- Flexibility. Data Lakes offer diverse storage formats and a schema-on-read approach for flexible analysis.
- Cost-Effectiveness. Cloud-hosted Data Lakes are cost-effective with scalable storage solutions.
- Advanced Analytics Capabilities. The raw, granular data in Data Lakes is ideal for advanced analytics, machine learning, and AI applications, providing deeper insights than traditional data warehouses.
Challenges
- Complexity and Management. Without proper management, a Data Lake can quickly become a “Data Swamp” where data is disorganised and unusable.
- Data Quality and Governance. Ensuring the quality and governance of data within a Data Lake can be challenging, requiring robust processes and tools.
- Security. Protecting sensitive data within a Data Lake is crucial, requiring comprehensive security measures.
Data Lakehouse
A Data Lakehouse is an innovative data management system that merges the strengths of Data Lakes and Data Warehouses. This hybrid approach strives to offer the adaptability and expansiveness of a Data Lake for housing extensive volumes of raw, unstructured data, while also providing the structured, refined data functionalities typical of a Data Warehouse. By bridging the gap between these two traditional data storage paradigms, Lakehouses enable more efficient data analytics, machine learning, and business intelligence operations across diverse data types and use cases.
Core Characteristics
- Unified Data Management. A Lakehouse streamlines data governance and security by managing both structured and unstructured data on one platform, reducing organizational data silos.
- Schema Flexibility. It supports schema-on-read and schema-on-write, allowing data to be stored and analysed flexibly. Data can be ingested in raw form and structured later or structured at ingestion.
- Scalability and Performance. Lakehouses scale storage and compute resources independently, handling large data volumes and complex analytics without performance compromise.
- Advanced Analytics and Machine Learning Integration. By providing direct access to both raw and processed data on a unified platform, Lakehouses facilitate advanced analytics, real-time analytics, and machine learning.
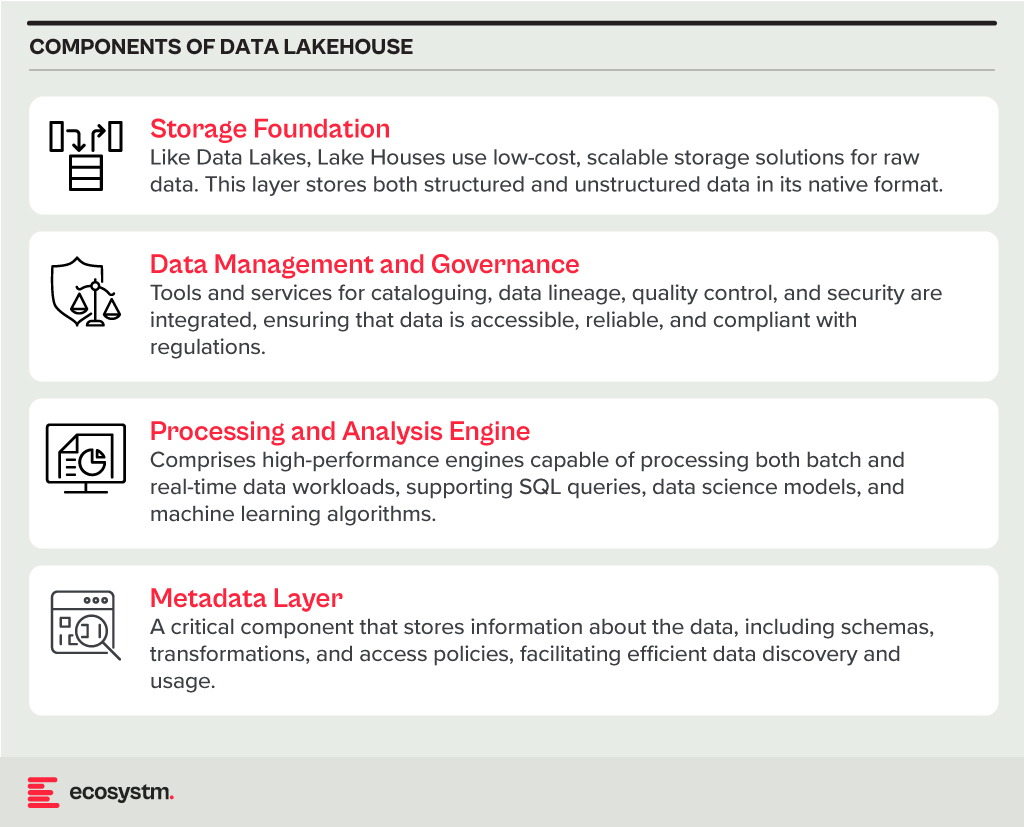
Benefits
- Versatility in Data Analysis. Lakehouses support diverse data analytics, spanning from traditional BI to advanced machine learning, all within one platform.
- Cost-Effective Scalability. The ability to scale storage and compute independently, often in a cloud environment, makes Lakehouses cost-effective for growing data needs.
- Improved Data Governance. Centralising data management enhances governance, security, and quality across all types of data.
Challenges
- Complexity in Implementation. Designing and implementing a Lakehouse architecture can be complex, requiring expertise in both Data Lakes and Data Warehouses.
- Data Consistency and Quality. Though crucial for reliable analytics, ensuring data consistency and quality across diverse data types and sources can be challenging.
- Governance and Security. Comprehensive data governance and security strategies are required to protect sensitive information and comply with regulations.
The choice between Data Warehouse, Data Lake, or Lakehouse systems is pivotal for businesses in harnessing the power of their data. Each option offers distinct advantages and challenges, requiring careful consideration of organisational needs and goals. By embracing the right data management system, organisations can pave the way for informed decision-making, operational efficiency, and innovation in the digital age.
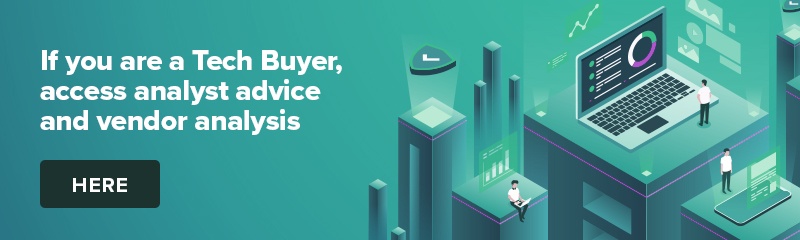