In my last Ecosystm Insights, I outlined various database options available to you. The challenge lies in selecting the right one. Selecting the right database is crucial for the success of any application or project. It involves understanding your data, the operations you’ll perform, scalability requirements, and more. Here is a guide that will walk you through key considerations and steps to choose the most suitable database from the list I shared last week.
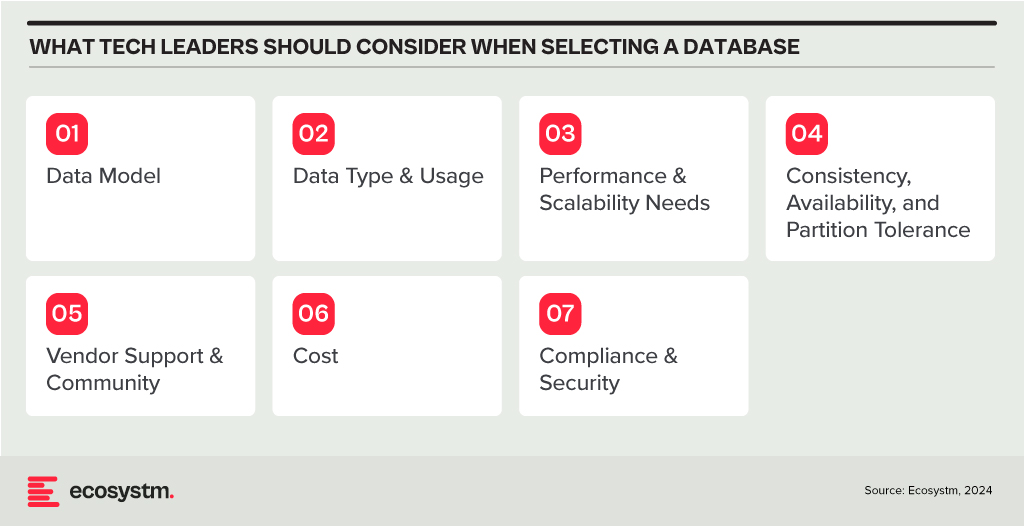
Understand Your Data Model
Relational (RDBMS) vs. NoSQL. Choose RDBMS if your data is structured and relational, requiring complex queries and transactions with ACID (Atomicity, Consistency, Isolation, Durability) properties. Opt for NoSQL if you have unstructured or semi-structured data, need to scale horizontally, or require flexibility in your schema design.
Consider the Data Type and Usage
Document Databases are ideal for storing, retrieving, and managing document-oriented information. They’re great for content management systems, ecommerce applications, and handling semi-structured data like JSON, XML.
Key-Value Stores shine in scenarios where quick access to data is needed through a key. They’re perfect for caching and storing user sessions, configurations, or any scenario where the lookup is based on a unique key.
Wide-Column Stores offer flexibility and scalability for storing and querying large volumes of data across many servers, suitable for big data applications, real-time analytics, and high-speed transactions.
Graph Databases are designed for data intensely connected through relationships, ideal for social networks, recommendation engines, and fraud detection systems where relationships between data points are key.
Time-Series Databases are optimised for storing and querying sequential data points indexed in time order. Use them for monitoring systems, IoT applications, and financial trading systems where time-stamped data is critical.
Spatial Databases support spatial data types and queries, making them suitable for geographic information systems (GIS), location-based services, and applications requiring spatial indexing and querying capabilities.
Assess Performance and Scalability Needs
In-Memory Databases like Redis offer high throughput and low latency for scenarios requiring rapid access to data, such as caching, session storage, and real-time analytics.
Distributed Databases like Cassandra or CouchDB are designed to run across multiple machines, offering high availability, fault tolerance, and scalability for applications with global reach and massive scale.
Evaluate Consistency, Availability, and Partition Tolerance (CAP Theorem)
Understand the trade-offs between consistency, availability, and partition tolerance. For example, if your application requires strong consistency, consider databases that prioritise consistency and partition tolerance (CP) like MongoDB or relational databases. If availability is paramount, look towards databases that offer availability and partition tolerance (AP) like Cassandra or CouchDB.
Other Considerations
Check for Vendor Support and Community. Evaluate the support and stability offered by vendors or open-source communities. Established products like Oracle Database, Microsoft SQL Server, and open-source options like PostgreSQL and MongoDB have robust support and active communities.
Cost. Consider both initial and long-term costs, including licenses, hardware, maintenance, and scalability. Open-source databases can reduce upfront costs, but ensure you account for support and operational expenses.
Compliance and Security. Ensure the database complies with relevant regulations (GDPR, HIPAA, etc.) and offers robust security features to protect sensitive data.
Try Before You Decide. Prototype your application with shortlisted databases to evaluate their performance, ease of use, and compatibility with your application’s requirements.
Conclusion
Selecting the right database is a strategic decision that impacts your application’s functionality, performance, and scalability. By carefully considering your data model, type of data, performance needs, and other factors like cost, support, and security, you can identify the database that best fits your project’s needs. Always stay informed about the latest developments in database technologies to make educated decisions as your requirements evolve.
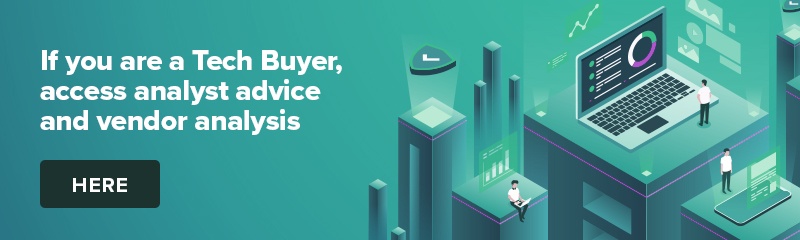
Why do we use AI? The goal of a business in adding intelligence is to enhance business decision-making, and growing revenue and profit within the framework of its business model.
The problem many organisations face is that they understand their own core competence in their own industry, but they do not understand how to tweak and enhance business processes to make the business run better. For example, AI can help transform the way companies run their production lines, enabling greater efficiency by enhancing human capabilities, providing real-time insights, and facilitating design and product innovation. But first, one has to be able to understand and digest the data within the organisation that would allow that to happen.
Ecosystm research shows that AI adoption crosses the gambit of business processes (Figure 1), but not all firms are process optimised to achieve those goals internally.
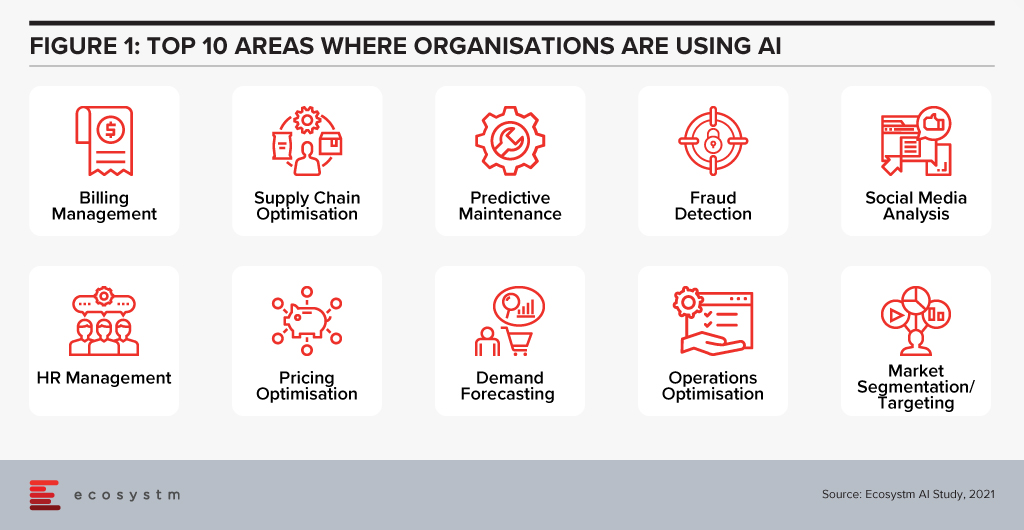
The initial landscape for AI services primarily focused on tech companies building AI products into their own solutions to power their own services. So, the likes of Amazon, Google and Apple were investing in people and processes for their own enhancements.
As the benefits of AI are more relevant in a post-pandemic world with staff and resource shortages, non-tech firms are becoming interested in applying those advantages to their own business processes.
AI for Decisions
Recent start-up ventures in AI are focusing on non-tech companies and offering services to get them to use AI within their own business models. Peak AI says that their technology can help enterprises that work with physical products to make better, AI-based evaluations and decisions, and has recently closed a funding round of USD 21 million.
The relevance of this is around the terminology that Peak AI has introduced. They call what they offer “Decision Intelligence” and are crafting a market space around it. Peak’s basic premise was to build AI not as a business goal for itself but as a business service aided by a solution and limited to particular types of added value. The goal of Peak AI is to identify where Decision Intelligence can add value, and help the company build a business case that is both achievable and commercially viable.
For example, UK hard landscaping manufacturer Marshalls worked with Peak AI to streamline their bid process with contractors. This allows customers to get the answers they need in terms of bid decisions and quotes quickly and efficiently, significantly speeding up the sales cycle.

AI-as-a-Service is not a new concept. Canadian start-up Element AI tried to create an AI services business for non-tech companies to use as they might these days use consulting services. It never quite got there, though, and was acquired by ServiceNow last year. Peak AI is looking at specific elements such as sales, planning and supply chain for physical products in how decisions are made and where adding some level of automation in the decision is beneficial. The Peak AI solution, CODI (Connected Decision Intelligence) sits as a layer of intelligence that between the other systems, ingesting the data and aiding in its utilisation.
The added tool to create a data-ingestion layer for business decision-making is quite a trend right now. For example, IBM’s Causal Inference 360 Toolkit offers access to multiple tools that can move the decision-making processes from “best guess” to concrete answers based on data, aiding data scientists to apply and understand causal inference in their models.
Implications on Business Processes
The bigger problem is not the volume of data, but the interpretation of it.
Data warehouses and other ways of gathering data to a central or cloud-based location to digest is also not new. The real challenge lies with the interpretation of what the data means and what decisions can be fine-tuned with this data. This implies that data modelling and process engineers need to be involved. Not every company has thought through the possible options for their processes, nor are they necessarily ready to implement these new processes both in terms of resources and priorities. This also requires data harmonisation rules, consistent data quality and managed data operations.
Given the increasing flow of data in most organisations, external service providers for AI solution layers embedded in the infrastructure as data filters could be helpful in making sense of what exists. And they can perhaps suggest how the processes themselves can be readjusted to match the growth possibilities of the business itself. This is likely a great footprint for the likes of Accenture, KPMG and others as process wranglers.
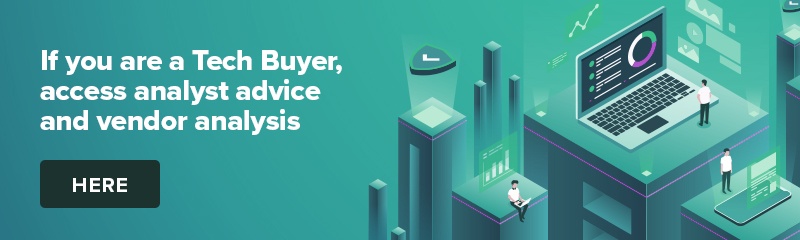