Data analysts play a vital role in today’s data-driven world, providing crucial insights that benefit decision-making processes. For those with a knack for numbers and a passion for uncovering patterns, a career as a data analyst can be both fulfilling and lucrative – it can also be a stepping stone towards other careers in data. While a data analyst focuses on data preparation and visualisation, an AI engineer specialises in creating AI solutions, a machine learning (ML) engineer concentrates on implementing ML models, and a data scientist combines elements of data analysis and ML to derive insights and predictions from data.
Tools, Skills, and Techniques of a Data Analyst
Excel Mastery. Unlocks a powerful toolbox for data manipulation and analysis. Essential skills include using a vast array of functions for calculations and data transformation. Pivot tables become your secret weapon for summarising and analysing large datasets, while charts and graphs bring your findings to life with visual clarity. Data validation ensures accuracy, and the Analysis ToolPak and Solver provide advanced functionalities for statistical analysis and complex problem-solving. Mastering Excel empowers you to transform raw data into actionable insights.
Advanced SQL. While basic skills handle simple queries, advanced users can go deeper with sorting, aggregation, and the art of JOINs to combine data from multiple tables. Common Table Expressions (CTEs) and subqueries become your allies for crafting complex queries, while aggregate functions summarise vast amounts of data. Window functions add another layer of power, allowing calculations within query results. Mastering Advanced SQL empowers you to extract hidden insights and manage data with unparalleled precision.
Data Visualisation. Crafts impactful data stories. These tools empower you to connect to various data sources, transform raw information into a usable format, and design interactive dashboards and reports. Filters and drilldowns allow users to explore your data from different angles, while calculated fields unlock deeper analysis. Parameters add a final touch of flexibility, letting viewers customise the report to their specific needs. With tools Tableau and Power BI, complex data becomes clear and engaging.
Essential Python. This powerful language excels at data analysis and automation. Libraries like NumPy and Pandas become your foundation for data manipulation and wrangling. Scikit-learn empowers you to build ML models, while SciPy and StatsModels provide a toolkit for in-depth statistical analysis. Python’s ability to interact with APIs and web scrape data expands its reach, and its automation capabilities streamline repetitive tasks. With Essential Python, you have the power to solve complex problems.
Automating the Journey. Data analysts can be masters of efficiency, and their skills translate beautifully into AI. Scripting languages like Ansible and Terraform automate repetitive tasks. Imagine streamlining the process of training and deploying AI models – a skill that directly benefits the AI development pipeline. This proficiency in automation showcases the valuable foundation data analysts provide for building and maintaining AI systems.
Developing ML Expertise. Transitioning from data analysis to AI involves building on your existing skills to develop ML expertise. As a data analyst, you may start with basic predictive models. This knowledge is expanded in AI to include deep learning and advanced ML algorithms. Also, skills in statistical analysis and visualisation help in evaluating the performance of AI models.
Growing Your AI Skills
Becoming an AI engineer requires building on a data analysis foundation to focus on advanced skills such as:
- Deep Learning. Learning frameworks like TensorFlow and PyTorch to build and train neural networks.
- Natural Language Processing (NLP). Techniques for processing and analysing large amounts of natural language data.
- AI Ethics and Fairness. Understanding the ethical implications of AI and ensuring models are fair and unbiased.
- Big Data Technologies. Using tools like Hadoop and Spark for handling large-scale data is essential for AI applications.
The Evolution of a Data Analyst: Career Opportunities
Data analysis is a springboard to AI engineering. Businesses crave talent that bridges the data-AI gap. Your data analyst skills provide the foundation (understanding data sources and transformations) to excel in AI. As you master ML, you can progress to roles like:
- AI Engineer. Works on integrating AI solutions into products and services. They work with AI frameworks like TensorFlow and PyTorch, ensuring that AI models are incorporated into products and services in a fair and unbiased manner.
- ML Engineer. Focuses on designing and implementing ML models. They focus on preprocessing data, evaluating model performance, and collaborating with data scientists and engineers to bring models into production. They need strong programming skills and experience with big data tools and ML algorithms.
- Data Scientist. Bridges the gap between data analysis and AI, often involved in both data preparation and model development. They perform exploratory data analysis, develop predictive models, and collaborate with cross-functional teams to solve complex business problems. Their role requires a comprehensive understanding of both data analysis and ML, as well as strong programming and data visualisation skills.
Conclusion
Hone your data expertise and unlock a future in AI! Mastering in-demand skills like Excel, SQL, Python, and data visualisation tools will equip you to excel as a data analyst. Your data wrangling skills will be invaluable as you explore ML and advanced algorithms. Also, your existing BI knowledge translates seamlessly into building and evaluating AI models. Remember, the data landscape is constantly evolving, so continue to learn to stay at the forefront of this dynamic field. By combining your data skills with a passion for AI, you’ll be well-positioned to tackle complex challenges and shape the future of AI.
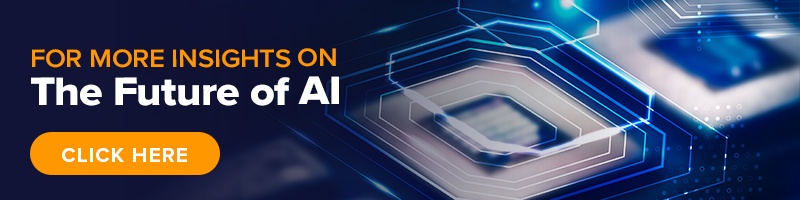
The data architecture outlines how data is managed in an organisation and is crucial for defining the data flow, data management systems required, the data processing operations, and AI applications. Data architects and engineers define data models and structures based on these requirements, supporting initiatives like data science. Before we delve into the right data architecture for your AI journey, let’s talk about the data management options. Technology leaders have the challenge of deciding on a data management system that takes into consideration factors such as current and future data needs, available skills, costs, and scalability. As data strategies become vital to business success, selecting the right data management system is crucial for enabling data-driven decisions and innovation.
Data Warehouse
A Data Warehouse is a centralised repository that stores vast amounts of data from diverse sources within an organisation. Its main function is to support reporting and data analysis, aiding businesses in making informed decisions. This concept encompasses both data storage and the consolidation and management of data from various sources to offer valuable business insights. Data Warehousing evolves alongside technological advancements, with trends like cloud-based solutions, real-time capabilities, and the integration of AI and machine learning for predictive analytics shaping its future.
Core Characteristics
- Integrated. It integrates data from multiple sources, ensuring consistent definitions and formats. This often includes data cleansing and transformation for analysis suitability.
- Subject-Oriented. Unlike operational databases, which prioritise transaction processing, it is structured around key business subjects like customers, products, and sales. This organisation facilitates complex queries and analysis.
- Non-Volatile. Data in a Data Warehouse is stable; once entered, it is not deleted. Historical data is retained for analysis, allowing for trend identification over time.
- Time-Variant. It retains historical data for trend analysis across various time periods. Each entry is time-stamped, enabling change tracking and trend analysis.
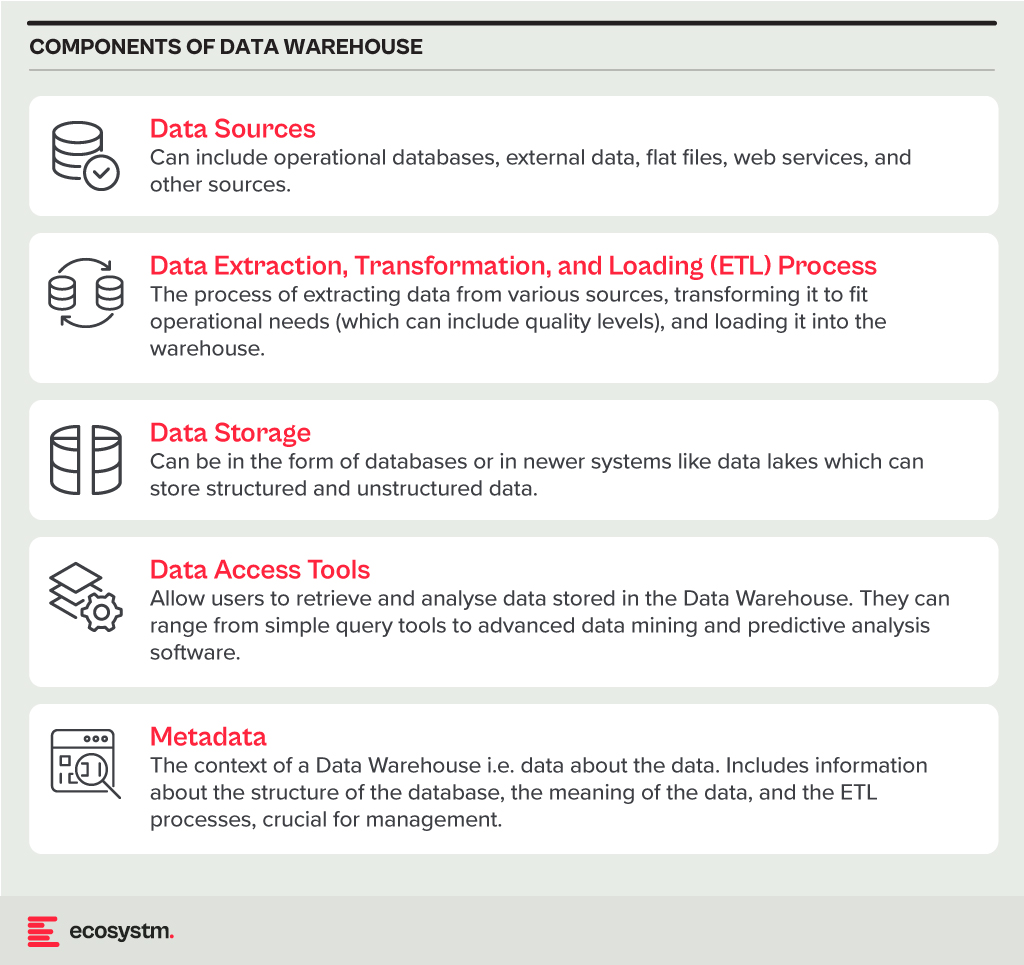
Benefits
- Better Decision Making. Data Warehouses consolidate data from multiple sources, offering a comprehensive business view for improved decision-making.
- Enhanced Data Quality. The ETL process ensures clean and consistent data entry, crucial for accurate analysis.
- Historical Analysis. Storing historical data enables trend analysis over time, informing future strategies.
- Improved Efficiency. Data Warehouses enable swift access and analysis of relevant data, enhancing efficiency and productivity.
Challenges
- Complexity. Designing and implementing a Data Warehouse can be complex and time-consuming.
- Cost. The cost of hardware, software, and specialised personnel can be significant.
- Data Security. Storing large amounts of sensitive data in one place poses security risks, requiring robust security measures.
Data Lake
A Data Lake is a centralised repository for storing, processing, and securing large volumes of structured and unstructured data. Unlike traditional Data Warehouses, which are structured and optimised for analytics with predefined schemas, Data Lakes retain raw data in its native format. This flexibility in data usage and analysis makes them crucial in modern data architecture, particularly in the age of big data and cloud.
Core Characteristics
- Schema-on-Read Approach. This means the data structure is not defined until the data is read for analysis. This offers more flexible data storage compared to the schema-on-write approach of Data Warehouses.
- Support for Multiple Data Types. Data Lakes accommodate diverse data types, including structured (like databases), semi-structured (like JSON, XML files), unstructured (like text and multimedia files), and binary data.
- Scalability. Designed to handle vast amounts of data, Data Lakes can easily scale up or down based on storage needs and computational demands, making them ideal for big data applications.
- Versatility. Data Lakes support various data operations, including batch processing, real-time analytics, machine learning, and data visualisation, providing a versatile platform for data science and analytics.
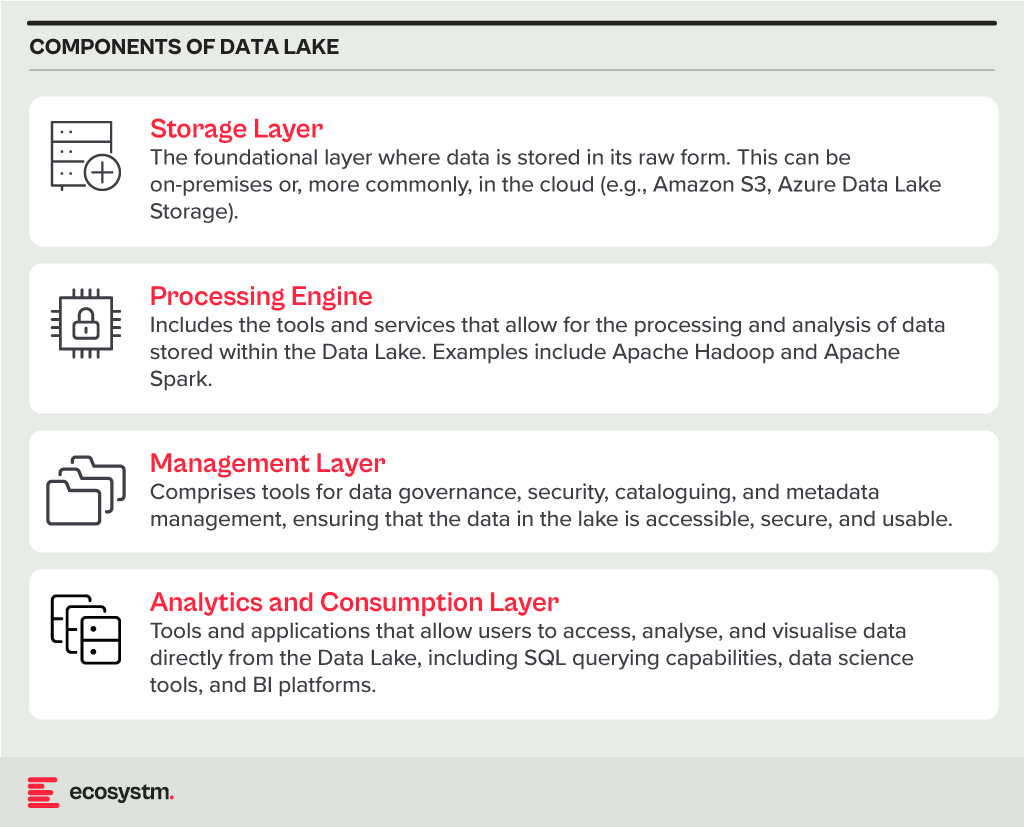
Benefits
- Flexibility. Data Lakes offer diverse storage formats and a schema-on-read approach for flexible analysis.
- Cost-Effectiveness. Cloud-hosted Data Lakes are cost-effective with scalable storage solutions.
- Advanced Analytics Capabilities. The raw, granular data in Data Lakes is ideal for advanced analytics, machine learning, and AI applications, providing deeper insights than traditional data warehouses.
Challenges
- Complexity and Management. Without proper management, a Data Lake can quickly become a “Data Swamp” where data is disorganised and unusable.
- Data Quality and Governance. Ensuring the quality and governance of data within a Data Lake can be challenging, requiring robust processes and tools.
- Security. Protecting sensitive data within a Data Lake is crucial, requiring comprehensive security measures.
Data Lakehouse
A Data Lakehouse is an innovative data management system that merges the strengths of Data Lakes and Data Warehouses. This hybrid approach strives to offer the adaptability and expansiveness of a Data Lake for housing extensive volumes of raw, unstructured data, while also providing the structured, refined data functionalities typical of a Data Warehouse. By bridging the gap between these two traditional data storage paradigms, Lakehouses enable more efficient data analytics, machine learning, and business intelligence operations across diverse data types and use cases.
Core Characteristics
- Unified Data Management. A Lakehouse streamlines data governance and security by managing both structured and unstructured data on one platform, reducing organizational data silos.
- Schema Flexibility. It supports schema-on-read and schema-on-write, allowing data to be stored and analysed flexibly. Data can be ingested in raw form and structured later or structured at ingestion.
- Scalability and Performance. Lakehouses scale storage and compute resources independently, handling large data volumes and complex analytics without performance compromise.
- Advanced Analytics and Machine Learning Integration. By providing direct access to both raw and processed data on a unified platform, Lakehouses facilitate advanced analytics, real-time analytics, and machine learning.
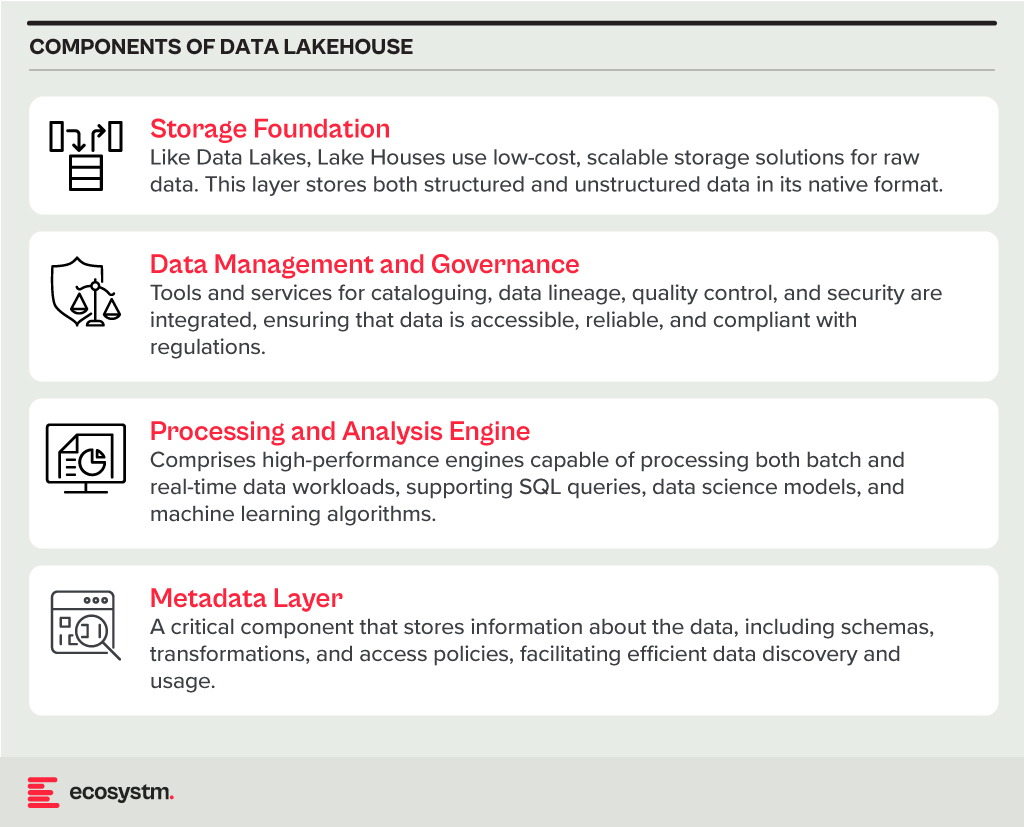
Benefits
- Versatility in Data Analysis. Lakehouses support diverse data analytics, spanning from traditional BI to advanced machine learning, all within one platform.
- Cost-Effective Scalability. The ability to scale storage and compute independently, often in a cloud environment, makes Lakehouses cost-effective for growing data needs.
- Improved Data Governance. Centralising data management enhances governance, security, and quality across all types of data.
Challenges
- Complexity in Implementation. Designing and implementing a Lakehouse architecture can be complex, requiring expertise in both Data Lakes and Data Warehouses.
- Data Consistency and Quality. Though crucial for reliable analytics, ensuring data consistency and quality across diverse data types and sources can be challenging.
- Governance and Security. Comprehensive data governance and security strategies are required to protect sensitive information and comply with regulations.
The choice between Data Warehouse, Data Lake, or Lakehouse systems is pivotal for businesses in harnessing the power of their data. Each option offers distinct advantages and challenges, requiring careful consideration of organisational needs and goals. By embracing the right data management system, organisations can pave the way for informed decision-making, operational efficiency, and innovation in the digital age.
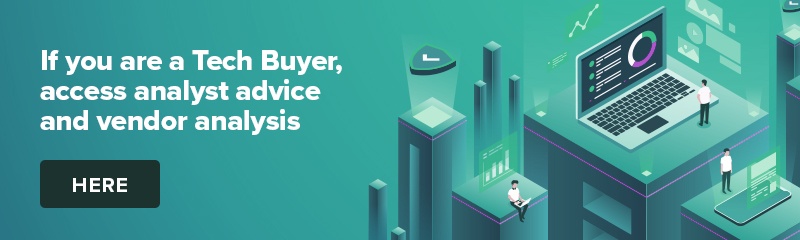
“Innovation is seeing what everybody has seen and thinking what nobody has thought”- Dr. Albert Szent-Györgyi (Discovered Vitamin C)
Innovation over the last 200 years has catapulted the human race into a world that is quite different from where it was 2 million years ago. So, what is the next wave in innovation? The answer seems to be in the interspace between industries, technologies, countries, domains and more.
Fusion innovation is a lateral innovation technique involving fruitful collision and fusion of different industries, fields, markets/countries, organisational silos, technologies, personalities, experiences, and skillsets towards creating radical, high-value outputs – newer ideas, products, and even industries. This is groundbreaking when it comes to digital innovation and its application in new ways.
Results have been big. Here are some examples:
- Crafting the world’s first digital music deal with Nokia ringtones to spawn the USD 2 billion ringtone industry
- Fusing satellite technology in radio is now a $24 billion enterprise – SiriusXM
- Ted Saad and team fusing art and business, technology and teams, and cultures and lifestyles – to win multiple Emmy awards
- Quantum chemistry, mathematical modeling, anthropology and management, fusing to create a new field – social network analysis
The Success Factor of Fusion Innovation
One secret of success of such radical innovation is a fundamental of design thinking – empathy. A lot of digital innovations either fail at the drawing board due to lack of purpose or at the budget approval stage due to lack of a business case and scalability. Successful digital innovations that have seen large scale adoption are pretty good at addressing end-user pain points, are scalable, and have a strong return on investment.
Examining the breeding grounds of digital innovations driving todays’ world – especially Artificial Intelligence (AI), automation, big data, blockchain and Internet of Things (IoT), we realise that the human potential to co-create stands out as the ultimate game changer.
Dr. C J Meadows, a pioneer in studying Fusion Innovation notes the following traits in “fusioney” people:
- Outward openness. This involves scanning broadly (including outside your industry) for ideas and technologies, engaging with an eclectic variety of people inside and outside the organisation. This is important especially in identifying unique opportunities for collaboration with cross-industry thought leaders. An excellent example of this would be the many emerging use cases in resource management using IoT in smart cities.
- Inward openness. This relates to people in an organisation exhibiting curiosity, depth and play and discovering their own inner design. The transformation story of graphics group to Pixar animations is an example of innovation driven by inward openness.
- Collecting. This involves ideating, curating, indexing and sharing ideas within and across functional divisions in a group or organisation. This not only enables lateral innovation, it also triggers “fusioneers” to see patterns and connections which others might have missed. Team wiki is an example of knowledge sharing within and across teams in an organisation that has revolutionised the art of problem solving in the technology industry.
- Sensing. This involves a great deal of empathy as well as having a deep appreciation of ideas that can propel the human race forward. Most automation use cases in contact centre operations that address repetitive and mundane tasks are a result of design thinking workshops that focus on the human pain points.
- Fusing. This involves absorbing many things around us and combining it in new ways. Hackathons conducted by many technology corporations have brought many interesting cross-functional solutions in the digital space that have been unique yet amazingly effective.
The future of digital innovation holds exciting possibilities with advancements in computational power, fusion of technologies and ideas, advancements in digital contracts. Lateral innovation techniques like Fusion can be expected to have a significant role in fueling this space for the years to come.
This is a contributed article from Nandakumar Kumar, a DBA Scholar at SP Jain School of Global Management.