In this blog, our guest author Shameek Kundu talks about the importance of making AI/ machine learning models reliable and safe. “Getting data and algorithms right has always been important, particularly in regulated industries such as banking, insurance, life sciences and healthcare. But the bar is much higher now: more data, from more sources, in more formats, feeding more algorithms, with higher stakes.”
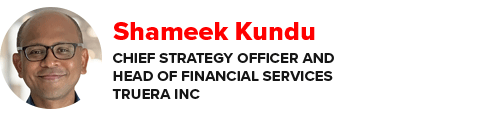
Building trust in algorithms is essential. Not (just) because regulators want it, but because it is good for customers and business. The good news is that with the right approach and tooling, it is also achievable.
Getting data and algorithms right has always been important, particularly in regulated industries such as banking, insurance, life sciences and healthcare. But the bar is much higher now: more data, from more sources, in more formats, feeding more algorithms, with higher stakes. With the increased use of Artificial Intelligence/ Machine Learning (AI/ML), today’s algorithms are also more powerful and difficult to understand.
A false dichotomy
At this point in the conversation, I get one of two reactions. One is of distrust in AI/ML and a belief that it should have little role to play in regulated industries. Another is of nonchalance; after all, most of us feel comfortable using ‘black-boxes’ (e.g., airplanes, smartphones) in our daily lives without being able to explain how they work. Why hold AI/ML to special standards?
Both make valid points. But the skeptics miss out on the very real opportunity cost of not using AI/ML – whether it is living with historical biases in human decision-making or simply not being able to do things that are too complex for a human to do, at scale. For example, the use of alternative data and AI/ML has helped bring financial services to many who have never had access before.
On the other hand, cheerleaders for unfettered use of AI/ML might be overlooking the fact that a human being (often with a limited understanding of AI/ML) is always accountable for and/ or impacted by the algorithm. And fairly or otherwise, AI/ML models do elicit concerns around their opacity – among regulators, senior managers, customers and the broader society. In many situations, ensuring that the human can understand the basis of algorithmic decisions is a necessity, not a luxury.
A way forward
Reconciling these seemingly conflicting requirements is possible. But it requires serious commitment from business and data/ analytics leaders – not (just) because regulators demand it, but because it is good for their customers and their business, and the only way to start capturing the full value from AI/ML.
1. ‘Heart’, not just ‘Head’
It is relatively easy to get people excited about experimenting with AI/ML. But when it comes to actually trusting the model to make decisions for us, we humans are likely to put up our defences. Convincing a loan approver, insurance under-writer, medical doctor or front-line sales-person to trust an AI/ML model – over their own knowledge or intuition – is as much about the ‘heart’ as the ‘head’. Helping them understand, on their own terms, how the alternative is at least as good as their current way of doing things, is crucial.
2. A Broad Church
Even in industries/ organisations that recognise the importance of governing AI/ML, there is a tendency to define it narrowly. For example, in Financial Services, one might argue that “an ML model is just another model” and expect existing Model Risk teams to deal with any incremental risks from AI/ML.
There are two issues with this approach:
First, AI/ML models tend to require a greater focus on model quality (e.g., with respect to stability, overfitting and unjust bias) than their traditional alternatives. The pace at which such models are expected to be introduced and re-calibrated is also much higher, stretching traditional model risk management approaches.
Second, poorly designed AI/ML models create second order risks. While not unique to AI/ML, these risks become accentuated due to model complexity, greater dependence on (high-volume, often non-traditional) data and ubiquitous adoption. One example is poor customer experience (e.g., badly communicated decisions) and unfair treatment (e.g., unfair denial of service, discrimination, misselling, inappropriate investment recommendations). Another is around the stability, integrity and competitiveness of financial markets (e.g., unintended collusion with other market players). Obligations under data privacy, sovereignty and security requirements could also become more challenging.
The only way to respond holistically is to bring together a broad coalition – of data managers and scientists, technologists, specialists from risk, compliance, operations and cyber-security, and business leaders.
3. Automate, Automate, Automate
A key driver for the adoption and effectiveness of AI/ ML is scalability. The techniques used to manage traditional models are often inadequate in the face of more data-hungry, widely used and rapidly refreshed AI/ML models. Whether it is during the development and testing phase, formal assessment/ validation or ongoing post-production monitoring, it is impossible to govern AI/ML at scale using manual processes alone.
o, somewhat counter-intuitively, we need more automation if we are to build and sustain trust in AI/ML. As humans are accountable for the outcomes of AI/ ML models, we can only be ‘in charge’ if we have the tools to provide us reliable intelligence on them – before and after they go into production. As the recent experience with model performance during COVID-19 suggests, maintaining trust in AI/ML models is an ongoing task.
***
I have heard people say “AI is too important to be left to the experts”. Perhaps. But I am yet to come across an AI/ML practitioner who is not keenly aware of the importance of making their models reliable and safe. What I have noticed is that they often lack suitable tools – to support them in analysing and monitoring models, and to enable conversations to build trust with stakeholders. If AI is to be adopted at scale, that must change.
Shameek Kundu is Chief Strategy Officer and Head of Financial Services at TruEra Inc. TruEra helps enterprises analyse, improve and monitor quality of machine
Have you evaluated the tech areas on your AI requirements? Get access to AI insights and key industry trends from our AI research.
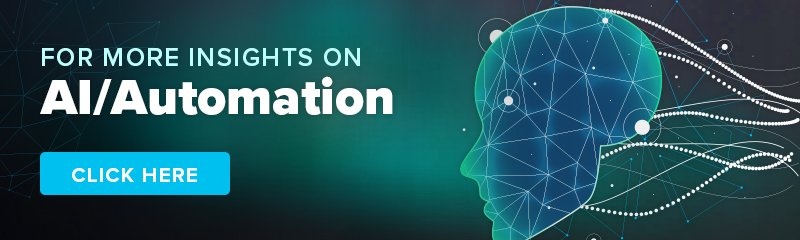
The disruption that we faced in 2020 has created a new appetite for adoption of technology and digital in a shorter period. Crises often present opportunities – and the FinTech and Financial Services industries benefitted from the high adoption of digital financial services and eCommerce. In 2021, there will be several drivers to the transformation of the Financial Services industry – the rise of the gig economy will give access to a larger talent pool; the challenges of government aid disbursement will be mitigated through tech adoption; compliance will come sharply back into focus after a year of ad-hoc technology deployments; and social and environmental awareness will create a greater appetite for green financing. However, the overarching driver will be the heightened focus on the individual consumer (Figure 1).
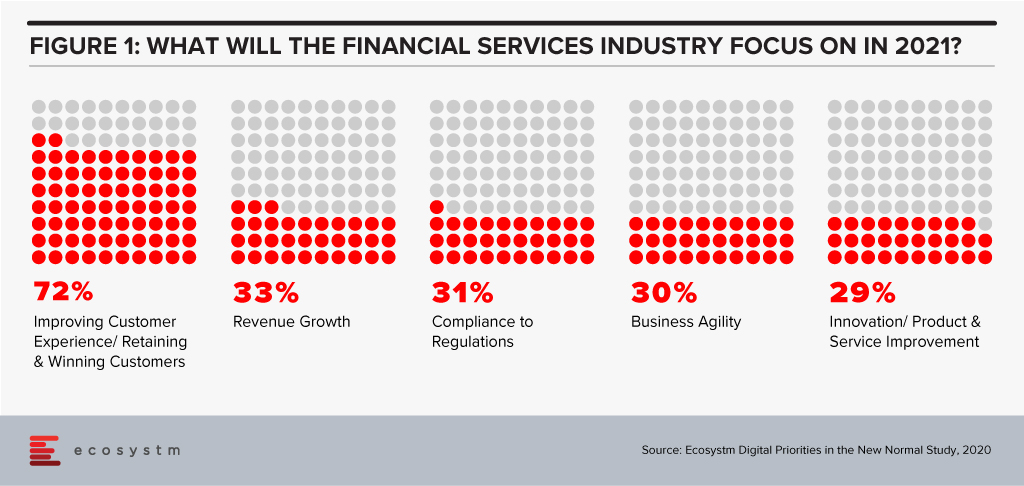
2021 will finally see consumers at the core of the digital financial ecosystem.
Ecosystm Advisors Dr. Alea Fairchild, Amit Gupta and Dheeraj Chowdhry present the top 5 Ecosystm predictions for FinTech in 2021 – written in collaboration with the Singapore FinTech Festival. This is a summary of the predictions; the full report (including the implications) is available to download for free on the Ecosystm platform.
The Top 5 FinTech Trends for 2021
#1 The New Decade of the ‘Empowered’ Consumer Will Propel Green Finance and Sustainability Considerations Beyond Regulators and Corporates
We have seen multiple countries set regulations and implement Emissions Trading Systems (ETS) and 2021 will see Environmental, Social and Governance (ESG) considerations growing in importance in the investment decisions for asset managers and hedge funds. Efforts for ESG standards for risk measurement will benefit and support that effort.
The primary driver will not only be regulatory frameworks – rather it will be further propelled by consumer preferences. The increased interest in climate change, sustainable business investments and ESG metrics will be an integral part of the reaction of the society to assist in the global transition to a greener and more humane economy in the post-COVID era. Individuals and consumers will demand FinTech solutions that empower them to be more environmentally and socially responsible. The performance of companies on their ESG ratings will become a key consideration for consumers making investment decisions. We will see corporate focus on ESG become a mainstay as a result – driven by regulatory frameworks and the consumer’s desire to place significant important on ESG as an investment criterion.
#2 Consumers Will Truly Be ‘Front and Centre’ in Reshaping the Financial Services Digital Ecosystems
Consumers will also shape the market because of the way they exercise their choices when it comes to transactional finance. They will opt for more discrete solutions – like microfinance, micro-insurances, multiple digital wallets and so on. Even long-standing customers will no longer be completely loyal to their main financial institutions. This will in effect take away traditional business from established financial institutions. Digital transformation will need to go beyond just a digital Customer Experience and will go hand-in-hand with digital offerings driven by consumer choice.
As a result, we will see the emergence of stronger digital ecosystems and partnerships between traditional financial institutions and like-minded FinTechs. As an example, platforms such as the API Exchange (APIX) will get a significant boost and play a crucial role in this emerging collaborative ecosystem. APIX was launched by AFIN, a non-profit organisation established in 2018 by the ASEAN Bankers Association (ABA), International Finance Corporation (IFC), a member of the World Bank Group, and the Monetary Authority of Singapore (MAS). Such platforms will create a level playing field across all tiers of the Financial Services innovation ecosystem by allowing industry participants to Discover, Design and rapidly Deploy innovative digital solutions and offerings.
#3 APIfication of Banking Will Become Mainstream
2020 was the year when banks accepted FinTechs into their product and services offerings – 2021 will see FinTech more established and their technology offerings becoming more sophisticated and consumer-led. These cutting-edge apps will have financial institutions seeking to establish partnerships with them, licensing their technologies and leveraging them to benefit and expand their customer base. This is already being called the “APIficiation” of banking. There will be more emphasis on the partnerships with regulated licensed banking entities in 2021, to gain access to the underlying financial products and services for a seamless customer experience.
This will see the growth of financial institutions’ dependence on third-party developers that have access to – and knowledge of – the financial institutions’ business models and data. But this also gives them an opportunity to leverage the existent Fintech innovations especially for enhanced customer engagement capabilities (Prediction #2).
#4 AI & Automation Will Proliferate in Back-Office Operations
From quicker loan origination to heightened surveillance against fraud and money laundering, financial institutions will push their focus on back-office automation using machine learning, AI and RPA tools (Figure 3). This is not only to improve efficiency and lower risks, but to further enhance the customer experience. AI is already being rolled out in customer-facing operations, but banks will actively be consolidating and automating their mid and back-office procedures for efficiency and automation transition in the post COVID-19 environment. This includes using AI for automating credit operations, policy making and data audits and using RPA for reducing the introduction of errors in datasets and processes.
There is enormous economic pressure to deliver cost savings and reduce risks through the adoption of technology. Financial Services leaders believe that insights gathered from compliance should help other areas of the business, and this requires a completely different mindset. Given the manual and semi-automated nature of current AML compliance, human-only efforts slow down processing timelines and impact business productivity. KYC will leverage AI and real-time environmental data (current accounts, mortgage payment status) and integration of third-party data to make the knowledge richer and timelier in this adaptive economic environment. This will make lending risk assessment more relevant.
#5 Driven by Post Pandemic Recovery, Collaboration Will Shape FinTech Regulation
Travel corridors across border controls have started to push the boundaries. Just as countries develop new processes and policies based on shared learning from other countries, FinTech regulators will collaborate to harmonise regulations that are similar in nature. These collaborative regulators will accelerate FinTech proliferation and osmosis i.e. proliferation of FinTechs into geographies with lower digital adoption.
Data corridors between countries will be the other outcome of this collaboration of FinTech regulators. Sharing of data in a regulated environment will advance data science and machine learning to new heights assisting credit models, AI, and innovations in general. The resulting ‘borderless nature’ of FinTech and the acceleration of policy convergence across several previously siloed regulators will result in new digital innovations. These Trusted Data Corridors between economies will be further driven by the desire for progressive governments to boost the Digital Economy in order to help the post-pandemic recovery.
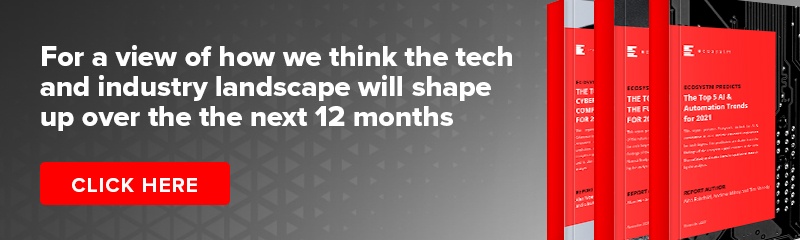