GenAI has taken the world by storm, with organisations big and small eager to pilot use cases for automation and productivity boosts. Tech giants like Google, AWS, and Microsoft are offering cloud-based GenAI tools, but the demand is straining current infrastructure capabilities needed for training and deploying large language models (LLMs) like ChatGPT and Bard.
Understanding the Demand for Chips
The microchip manufacturing process is intricate, involving hundreds of steps and spanning up to four months from design to mass production. The significant expense and lengthy manufacturing process for semiconductor plants have led to global demand surpassing supply. This imbalance affects technology companies, automakers, and other chip users, causing production slowdowns.
Supply chain disruptions, raw material shortages (such as rare earth metals), and geopolitical situations have also had a fair role to play in chip shortages. For example, restrictions by the US on China’s largest chip manufacturer, SMIC, made it harder for them to sell to several organisations with American ties. This triggered a ripple effect, prompting tech vendors to start hoarding hardware, and worsening supply challenges.
As AI advances and organisations start exploring GenAI, specialised AI chips are becoming the need of the hour to meet their immense computing demands. AI chips can include graphics processing units (GPUs), application-specific integrated circuits (ASICs), and field-programmable gate arrays (FPGAs). These specialised AI accelerators can be tens or even thousands of times faster and more efficient than CPUs when it comes to AI workloads.
The surge in GenAI adoption across industries has heightened the demand for improved chip packaging, as advanced AI algorithms require more powerful and specialised hardware. Effective packaging solutions must manage heat and power consumption for optimal performance. TSMC, one of the world’s largest chipmakers, announced a shortage in advanced chip packaging capacity at the end of 2023, that is expected to persist through 2024.
The scarcity of essential hardware, limited manufacturing capacity, and AI packaging shortages have impacted tech providers. Microsoft acknowledged the AI chip crunch as a potential risk factor in their 2023 annual report, emphasising the need to expand data centre locations and server capacity to meet customer demands, particularly for AI services. The chip squeeze has highlighted the dependency of tech giants on semiconductor suppliers. To address this, companies like Amazon and Apple are investing heavily in internal chip design and production, to reduce dependence on large players such as Nvidia – the current leader in AI chip sales.
How are Chipmakers Responding?
NVIDIA, one of the largest manufacturers of GPUs, has been forced to pivot its strategy in response to this shortage. The company has shifted focus towards developing chips specifically designed to handle complex AI workloads, such as the A100 and V100 GPUs. These AI accelerators feature specialised hardware like tensor cores optimised for AI computations, high memory bandwidth, and native support for AI software frameworks.
While this move positions NVIDIA at the forefront of the AI hardware race, experts say that it comes at a significant cost. By reallocating resources towards AI-specific GPUs, the company’s ability to meet the demand for consumer-grade GPUs has been severely impacted. This strategic shift has worsened the ongoing GPU shortage, further straining the market dynamics surrounding GPU availability and demand.
Others like Intel, a stalwart in traditional CPUs, are expanding into AI, edge computing, and autonomous systems. A significant competitor to Intel in high-performance computing, AMD acquired Xilinx to offer integrated solutions combining high-performance central processing units (CPUs) and programmable logic devices.
Global Resolve Key to Address Shortages
Governments worldwide are boosting chip capacity to tackle the semiconductor crisis and fortify supply chains. Initiatives like the CHIPS for America Act and the European Chips Act aim to bolster domestic semiconductor production through investments and incentives. Leading manufacturers like TSMC and Samsung are also expanding production capacities, reflecting a global consensus on self-reliance and supply chain diversification. Asian governments are similarly investing in semiconductor manufacturing to address shortages and enhance their global market presence.
Japan is providing generous government subsidies and incentives to attract major foreign chipmakers such as TSMC, Samsung, and Micron to invest and build advanced semiconductor plants in the country. Subsidies have helped to bring greenfield investments in Japan’s chip sector in recent years. TSMC alone is investing over USD 20 billion to build two cutting-edge plants in Kumamoto by 2027. The government has earmarked around USD 13 billion just in this fiscal year to support the semiconductor industry.
Moreover, Japan’s collaboration with the US and the establishment of Rapidus, a memory chip firm, backed by major corporations, further show its ambitions to revitalise its semiconductor industry. Japan is also looking into advancements in semiconductor materials like silicon carbide (SiC) and gallium nitride (GaN) – crucial for powering electric vehicles, renewable energy systems, and 5G technology.
South Korea. While Taiwan holds the lead in semiconductor manufacturing volume, South Korea dominates the memory chip sector, largely due to Samsung. The country is also spending USD 470 billion over the next 23 years to build the world’s largest semiconductor “mega cluster” covering 21,000 hectares in Gyeonggi Province near Seoul. The ambitious project, a partnership with Samsung and SK Hynix, will centralise and boost self-sufficiency in chip materials and components to 50% by 2030. The mega cluster is South Korea’s bold plan to cement its position as a global semiconductor leader and reduce dependence on the US amidst growing geopolitical tensions.
Vietnam. Vietnam is actively positioning itself to become a major player in the global semiconductor supply chain amid the push to diversify away from China. The Southeast Asian nation is offering tax incentives, investing in training tens of thousands of semiconductor engineers, and encouraging major chip firms like Samsung, Nvidia, and Amkor to set up production facilities and design centres. However, Vietnam faces challenges such as a limited pool of skilled labour, outdated energy infrastructure leading to power shortages in key manufacturing hubs, and competition from other regional players like Taiwan and Singapore that are also vying for semiconductor investments.
The Potential of SLMs in Addressing Infrastructure Challenges
Small language models (SLMs) offer reduced computational requirements compared to larger models, potentially alleviating strain on semiconductor supply chains by deploying on smaller, specialised hardware.
Innovative SLMs like Google’s Gemini Nano and Mistral AI’s Mixtral 8x7B enhance efficiency, running on modest hardware, unlike their larger counterparts. Gemini Nano is integrated into Bard and available on Pixel 8 smartphones, while Mixtral 8x7B supports multiple languages and suits tasks like classification and customer support.
The shift towards smaller AI models can be pivotal to the AI landscape, democratising AI and ensuring accessibility and sustainability. While they may not be able to handle complex tasks as well as LLMs yet, the ability of SLMs to balance model size, compute power, and ethical considerations will shape the future of AI development.
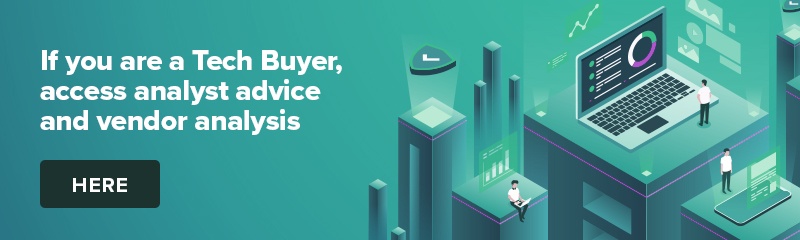
Last week, NVIDIA announced that it had agreed to acquire UK-based chip company Arm from Japanese conglomerate SoftBank in a deal estimated to be worth USD 40 billion. In 2016, SoftBank had acquired Arm for USD 32 billion. The deal is set to unite two major chip companies; power data centres and mobile devices for the age of AI and high-performance computing; and accelerate innovation in the enterprise and consumer market.
Rationale for the Deal
NVIDIA has long been the industry leader in graphics chips (GPUs), and a smaller but significantly profitable player in the chip stakes. With graphic processing being a key component in AI applications like facial recognition, NVIDIA was quick to capitalise. This allowed it to move into data centres – an area long dominated by Intel who still holds the lion’s share of this market. NVIDIA’s data centre business has grown tremendously – from near zero less than ten years ago to nearly USD 3 billion in the first two quarters of this fiscal year. It contributes 42% of the company’s total sales.
The gaming PC market has been the fastest-growing segment in the PC market. The rare shining light in an otherwise stagnant-to-slightly declining market. NVIDIA has benefited greatly from this with a huge jump in their graphics revenues. Its GeForce brand is one of the most desired in the industry. However, with their success in AI, NVIDIA’s ambition has now grown well beyond the graphics market. Last year NVIDIA acquired Mellanox – who makes specialised networking products especially in the area of high-performance computing, data centres, cloud computing – for almost USD 7 billion. There is clearly a desire to expand the company’s footprint and position itself as a broad-based player in the data centre and cloud space focused on AI computing needs.
The acquisition of Arm though adds a whole new dimension. Arm is the leading technology provider in the mobile chip market. A staggering 90% of smartphones are estimated to use Arm technology. Arm is the colossus of the small chip industry – having crossed 20 billion in unit shipments in 2019.
Acquiring Arm is likely to result in NVIDIA now having a play in the effervescent smartphone market. But the company is possibly eyeing a different prize. Jensen Huang, Founder and CEO of NVIDIA said “AI is the most powerful technology force of our time and has launched a new wave of computing. In the years ahead, trillions of computers running AI will create a new internet-of-things that is thousands of times larger than today’s internet-of-people. Our combination will create a company fabulously positioned for the age of AI.”
With thoughts of self-driving cars, connected homes, smartphones, IoT, edge computing – all seamlessly working with each other, the acquisition of Arm provides NVIDIA a unique position in this market. As the number of connected devices explodes, as many billions of sensors become an ubiquitous part of 21st century living, there is going to be a huge demand for low power processing everywhere. Having that market may turn out to be a larger prize than the smartphone market. The possibilities are endless.
While this deal is supposed to be worth around USD 40 billion, somewhere between USD 23-28 billion is going to be paid in the form of NVIDIA stock. This brings us to an extremely interesting dynamic. At the beginning of 2016 NVIDIA’s market cap was less than USD 20 billion. Mighty Intel was at USD 150 billion. AMD the other player in the market for chips who also sell graphics was at a mere USD 2 billion. In July this year, NVIDIA’s value passed Intel’s and today it is sitting at around USD 300 billion! Intel with a recent dip is now close to USD 200 billion. AMD too with all the tech-fueled growth in recent years has grown to just shy of USD 100 billion market cap.
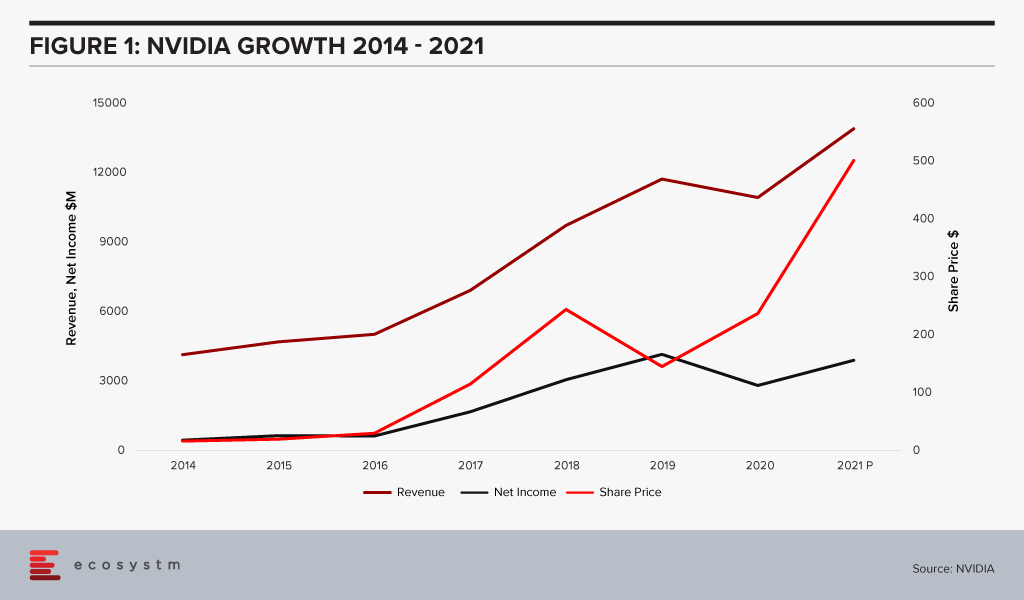
What this tells us is that the stock portion of the deal is cheaper for NVIDIA today by around 55% compared to if this deal was consummated on 1st January 2020. If there was a right time for NVIDIA to buy – it is now. This also shows the way the company has grown revenue at a massive clip powered by Gaming PCs and AI. The deal to buy Arm appears to be a very good idea, which would establish NVIDIA as a leader in the chip industry moving forward.
Ecosystm Comments
While there appears to be some good reasons for this deal and there are some very exciting possibilities for both NVIDIA and Arm, there are some challenges.
The tech industry is littered with examples of large mergers and splits that did not pan out. Given that this is a large deal between two businesses without a large overlap, this partnership needs to be handled with a great deal of care and thought. The right people need to be retained. Customer trust needs to be retained.
Arm so far has been successful as a neutral provider of IP and design. It does not make chips, far less any downstream products. It therefore does not compete with any of the vendors licensing its technology. NVIDIA competes with Arm’s customers. The deal might create significant misgivings in the minds of many customers about sharing of information like roadmaps and pricing. Both companies have been making repeated statements that they will ensure separation of the businesses to avoid conflicts.
However, it might prove to be difficult for NVIDIA and Arm to do the delicate dance of staying at arm’s length (pun intended) while at the same time obtaining synergies. Collaborating on technology development might prove to be difficult as well, if customer roadmaps cannot be discussed.
Business today also cannot escape the gravitational force of geo-politics. Given the current US-China spat, the Chinese media and various other agencies are already opposing this deal. Chinese companies are going to be very wary of using Arm technology if there is a chance the tap can be suddenly shut down by the US government. China accounts for about 25% of Arm’s market in units. One of the unintended consequences which could emerge from this is the empowerment of a new competitor in this space.
NVIDIA and Arm will need to take a very strategic long-term view, get communication out well ahead of the market and reassure their customers, ensuring they retain their trust. If they manage this well then they can reap huge benefits from their merger.
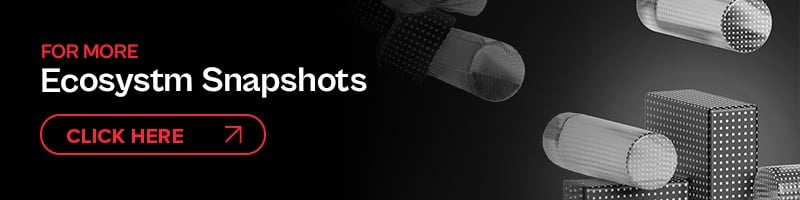
Two things happened recently that 99% of the ICT world would normally miss. After all microprocessor and chip interconnect technology is quite the geek area where we generally don’t venture into. So why would I want to bring this to your attention?
We are excited about the innovation that analytics, machine learning (ML) and all things real time processing will bring to our lives and the way we run our business. The data center, be it on an enterprise premise or truly on a cloud service provider’s infrastructure is being pressured to provide compute, memory, input/output (I/O) and storage requirements to take advantage of the hardware engineers would call ‘accelerators’. In its most simple form, an accelerator microprocessor does the specialty work for ML and analytics algorithms while the main microprocessor is trying to hold everything else together to ensure that all of the silicon parts are in sync. If we have a ML accelerator that is too fast with its answers, it will sit and wait for everyone else as its outcomes squeezed down a narrow, slow pipe or interconnect – in other words, the servers that are in the data center are not optimized for these workloads. The connection between the accelerators and the main components becomes the slowest and weakest link…. So now back to the news of the day.
A new high speed CPU-to-device interconnect standard, the Common Express Link (CXL) 1.0 was announced by Intel and a consortium of leading technology companies (Huawei and Cisco in the network infrastructure space, HPE and Dell EMC in the server hardware market, and Alibaba, Facebook, Google and Microsoft for the cloud services provider markets). CXL joins a crowded field of other standards already in the server link market including CAPI, NVLINK, GEN-Z and CCIX. CXL is being positioned to improve the performance of the links between FPGA and GPUs, the most common accelerators to be involved in ML-like workloads.
Of course there were some names that were absent from the launch – Arm, AMD, Nvidia, IBM, Amazon and Baidu. Each of them are members of the other standards bodies and probably are playing the waiting game.
Now let’s pause for a moment and look at the other announcement that happened at the same time. Nvidia and Mellanox announced that the two companies had reached a definitive agreement under which Nvidia will acquire Mellanox for $6.9 billion. Nvidia puts the acquisition reasons as “The data and compute intensity of modern workloads in AI, scientific computing and data analytics is growing exponentially and has put enormous performance demands on hyperscale and enterprise datacenters. While computing demand is surging, CPU performance advances are slowing as Moore’s law has ended. This has led to the adoption of accelerated computing with Nvidia GPUs and Mellanox’s intelligent networking solutions.”
So to me it seems that despite Intel working on CXL for four years, it looks like they might have been outbid by Nvidia for Mellanox. Mellanox has been around for 20 years and was the major supplier of Infiniband, a high speed interconnect that is common in high performance workloads and very well accepted by the HPC industry. (Note: Intel was also one of the founders of the Infiniband Trade Association, IBTA, before they opted to refocus on the PCI bus). With the growing need for fast links between the accelerators and the microprocessors, it would seem like Mellanox persistence had paid off and now has the market coming to it. One can’t help but think that as soon as Intel knew that Nvidia was getting Mellanox, it pushed forward with the CXL announcement – rumors that have had no response from any of the parties.
Advice for Tech Suppliers:
The two announcements are great for any vendor who is entering the AI, intense computing world using graphics and floating point arithmetic functions. We know that more digital-oriented solutions are asking for analytics based outcomes so there will be a growing demand for broader commoditized server platforms to support them. Tech suppliers should avoid backing or picking one of either the CXL or Infiniband at the moment until we see how the CXL standard evolves and how nVidia integrates Mellanox.
Advice for Tech Users:
These two announcements reflect innovation that is generally so far away from the end user, that it can go unnoticed. However, think about how USB (Universal Serial Bus) has changed the way we connect devices to our laptops, servers and other mobile devices. The same will true for this connection as more and more data is both read and outcomes generated by the ‘accelerators’ for the way we drive our cars, digitize our factories, run our hospitals, and search the Internet. Innovation in this space just got a shot in the arm from these two announcements.