Trust in the Banking, Financial Services, and Insurance (BFSI) industry is critical – and this amplifies the value of stolen data and fuels the motivation of malicious actors. Ransomware attacks continue to escalate, underscoring the need for fortified backup, encryption, and intrusion prevention systems. Similarly, phishing schemes have become increasingly sophisticated, placing a burden on BFSI cyber teams to educate employees, inform customers, deploy multifactor authentication, and implement fraud detection systems. While BFSI organisations work to fortify their defences, intruders continually find new avenues for profit – cyber protection is a high-stakes game of technological cat and mouse!
Some of these challenges inherent to the industry include the rise of cryptojacking – the unauthorised use of a BFSI company’s extensive computational resources for cryptocurrency mining.
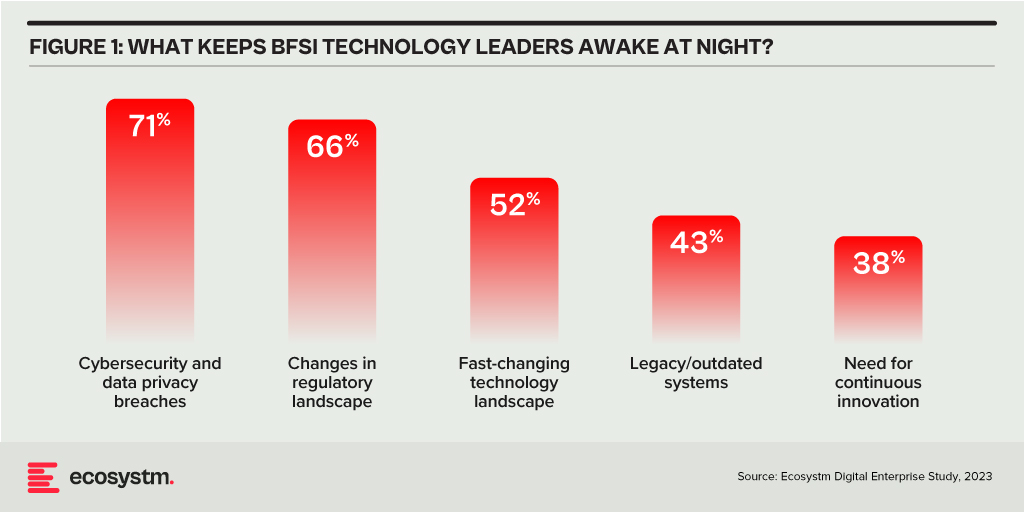
Building Trust Amidst Expanding Threat Landscape
BFSI organisations face increasing complexity in their IT landscapes. Amidst initiatives like robo-advisory, point-of-sale lending, and personalised engagements – often facilitated by cloud-based fintech providers – they encounter new intricacies. As guest access extends to bank branches and IoT devices proliferate in public settings, vulnerabilities can emerge unexpectedly. Threats may arise from diverse origins, including misconfigured ATMs, unattended security cameras, or even asset trackers. Ensuring security and maintaining customer trust requires BFSI organisations to deploy automated and intelligent security systems to respond to emerging new threats.
Ecosystm research finds that nearly 70% of BFSI organisations have the intention of adopting AI and automation for security operations, over the next two years. But the reality is that adoption is still fairly nascent. Their top cyber focus areas remain data security, risk and compliance management, and application security.
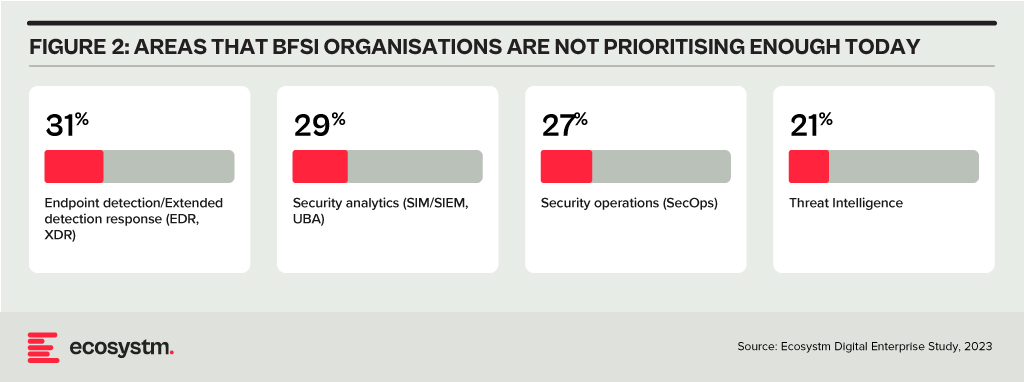
Addressing Alert Fatigue and Control Challenges
According to Ecosystm research, 50% of BFSI organisations use more than 50 security tools to secure their infrastructure – and these are only the known tools. Cyber leaders are not only challenged with finding, assessing, and deploying the right tools, they are also challenged with managing them. Management challenges include a lack of centralised control across assets and applications and handling a high volume of security events and false positives.
Software updates and patches within the IT environment are crucial for security operations to identify and address potential vulnerabilities. Management of the IT environment should be paired with greater automation – event correlation, patching, and access management can all be improved through reduced manual processes.
Security operations teams must contend with the thousands of alerts that they receive each day. As a result, security analysts suffer from alert fatigue and struggle to recognise critical issues and novel threats. There is an urgency to deploy solutions that can help to reduce noise. For many organisations, an AI-augmented security team could de-prioritise 90% of alerts and focus on genuine risks.
Taken a step further, tools like AIOps can not only prioritise alerts but also respond to them. Directing issues to the appropriate people, recommending actions that can be taken by operators directly in a collaboration tool, and rules-based workflows performed automatically are already possible. Additionally, by evaluating past failures and successes, AIOps can learn over time which events are likely to become critical and how to respond to them. This brings us closer to the dream of NoOps, where security operations are completely automated.
Threat Intelligence and Visibility for a Proactive Cyber Approach
New forms of ransomware, phishing schemes, and unidentified vulnerabilities in cloud are emerging to exploit the growing attack surface of financial services organisations. Security operations teams in the BFSI sector spend most of their resources dealing with incoming alerts, leaving them with little time to proactively investigate new threats. It is evident that organisations require a partner that has the scale to maintain a data lake of threats identified by a broad range of customers even within the same industry. For greater predictive capabilities, threat intelligence should be based on research carried out on the dark web to improve situational awareness. These insights can help security operations teams to prepare for future attacks. Regular reporting to keep CIOs and CISOs informed of the changing threat landscape can also ease the mind of executives.
To ensure services can be delivered securely, BFSI organisations require additional visibility of traffic on their networks. The ability to not only inspect traffic as it passes through the firewall but to see activity within the network is critical in these increasingly complex environments. Network traffic anomaly detection uses machine learning to recognise typical traffic patterns and generates alerts for abnormal activity, such as privilege escalation or container escape. The growing acceptance of BYOD has also made device visibility more complex. By employing AI and adopting a zero-trust approach, devices can be profiled and granted appropriate access automatically. Network operators gain visibility of unknown devices and can easily enforce policies on a segmented network.
Intelligent Cyber Strategies
Here is what BFSI CISOs should prioritise to build a cyber resilient organisation.
Automation. The volume of incoming threats has grown beyond the capability of human operators to investigate manually. Increase the level of automation in your SOC to minimise the routine burden on the security operations team and allow them to focus on high-risk threats.
Cyberattack simulation exercises. Many security teams are too busy dealing with day-to-day operations to perform simulation exercises. However, they are a vital component of response planning. Organisation-wide exercises – that include security, IT operations, and communications teams – should be conducted regularly.
An AIOps topology map. Identify where you have reliable data sources that could be analysed by AIOps. Then select a domain by assessing the present level of observability and automation, IT skills gap, frequency of threats, and business criticality. As you add additional domains and the system learns, the value you realise from AIOps will grow.
A trusted intelligence partner. Extend your security operations team by working with a partner that can provide threat intelligence unattainable to most individual organisations. Threat intelligence providers can pool insights gathered from a diversity of client engagements and dedicated researchers. By leveraging the experience of a partner, BFSI organisations can better plan for how they will respond to inevitable breaches.
Conclusion
An effective cybersecurity strategy demands a comprehensive approach that incorporates technology, education, and policies while nurturing a culture of security awareness throughout the organisation. CISOs face the daunting task of safeguarding their organisations against relentless cyber intrusion attempts by cybercriminals, who often leverage cutting-edge automated intrusion technologies.
To maintain an advantage over these threats, cybersecurity teams must have access to continuous threat intelligence; automation will be essential in addressing the shortage of security expertise and managing the overwhelming volume and frequency of security events. Collaborating with a specialised partner possessing both scale and experience is often the answer for organisations that want to augment their cybersecurity teams with intelligent, automated agents capable of swiftly
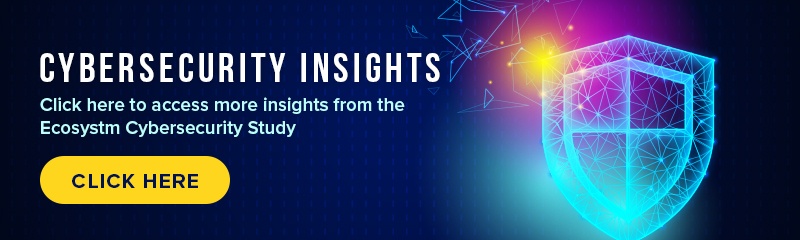
Traditional network architectures are inherently fragile, often relying on a single transport type to connect branches, production facilities, and data centres. The imperative for networks to maintain resilience has grown significantly, particularly due to the delivery of customer-facing services at branches and the increasing reliance on interconnected machines in operational environments. The cost of network downtime can now be quantified in terms of both lost customers and reduced production.
Distributed Enterprises Face New Challenges
As the importance of maintaining resiliency grows, so does the complexity of network management. Distributed enterprises must provide connectivity under challenging conditions, such as:
- Remote access for employees using video conferencing
- Local breakout for cloud services to avoid backhauling
- IoT devices left unattended in public places
- Customers accessing digital services at the branch or home
- Sites in remote areas requiring the same quality of service
Network managers require intelligent tools to remain in control without adding any unnecessary burden to end users. The number of endpoints and speed of change has made it impossible for human operators to manage without assistance from AI.
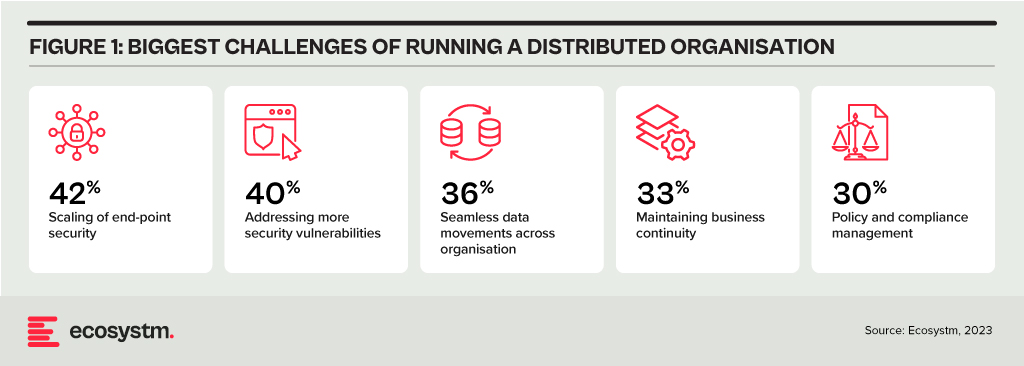
AI-Enhanced Network Management
Modern network operations centres are enhancing their visibility by aggregating data from diverse systems and consolidating them within a unified management platform. Machine learning (ML) and AI are employed to analyse data originating from enterprise networks, telecom Points of Presence (PoPs), IoT devices, cloud service providers, and user experience monitoring. These technologies enable the early identification of network issues before they reach critical levels. Intelligent networks can suggest strategies to enhance network resilience, forecast how modifications may impact performance, and are increasingly capable of autonomous responses to evolving conditions.
Here are some critical ways that AI/ML can help build resilient networks.
- Alert Noise Reduction. Network operations centres face thousands of alerts each day. As a result, operators battle with alert fatigue and are challenged to identify critical issues. Through the application of ML, contemporary monitoring tools can mitigate false positives, categorise interconnected alerts, and assist operators in prioritising the most pressing concerns. An operations team, augmented with AI capabilities could potentially de-prioritise up to 90% of alerts, allowing a concentrated focus on factors that impact network performance and resilience.
- Data Lakes. Networking vendors are building their own proprietary data lakes built upon telemetry data generated by the infrastructure they have deployed at customer sites. This vast volume of data allows them to use ML to create a tailored baseline for each customer and to recommend actions to optimise the environment.
- Root Cause Analysis. To assist network operators in diagnosing an issue, AIOps can sift through thousands of data points and correlate them to identify a root cause. Through the integration of alerts with change feeds, operators can understand the underlying causes of network problems or outages. By using ML to understand the customer’s unique environment, AIOps can progressively accelerate time to resolution.
- Proactive Response. As management layers become capable of recommending corrective action, proactive response also becomes possible, leading to self-healing networks. With early identification of sub-optimal conditions, intelligent systems can conduct load balancing, redirect traffic to higher performing SaaS regions, auto-scale cloud instances, or terminate selected connections.
- Device Profiling. In a BYOD environment, network managers require enhanced visibility to discover devices and enforce appropriate policies on them. Automated profiling against a validated database ensures guest access can be granted without adding friction to the onboarding process. With deep packet inspection, devices can be precisely classified based on behaviour patterns.
- Dynamic Bandwidth Aggregation. A key feature of an SD-WAN is that it can incorporate diverse transport types, such as fibre, 5G, and low earth orbit (LEO) satellite connectivity. Rather than using a simple primary and redundant architecture, bandwidth aggregation allows all circuits to be used simultaneously. By infusing intelligence into the SD-WAN layer, the process of path selection can dynamically prioritise traffic by directing it over higher quality or across multiple links. This approach guarantees optimal performance, even in the face of network degradation.
- Generative AI for Process Efficiency. Every tech company is trying to understand how they can leverage the power of Generative AI, and networking providers are no different. The most immediate use case will be to improve satisfaction and scalability for level 1 and level 2 support. A Generative AI-enabled service desk could provide uninterrupted support during high-volume periods, such as during network outages, or during off-peak hours.
Initiating an AI-Driven Network Management Journey
Network managers who take advantage of AI can build highly resilient networks that maximise uptime, deliver consistently high performance, and remain secure. Some important considerations when getting started include:
- Data Catalogue. Take stock of the data sources that are available to you, whether they come from network equipment telemetry, applications, or the data lake of a managed services provider. Understand how they can be integrated into an AIOps solution.
- Start Small. Begin with a pilot in an area where good data sources are available. This will help you assess the impact that AI could have on reducing alerts, improving mean time to repair (MTTR), increasing uptime, or addressing the skills gap.
- Develop an SD-WAN/SASE Roadmap. Many advanced AI benefits are built into an SD-WAN or SASE. Most organisations already have or will soon adopt SD-WAN but begin assessing the SASE framework to decide if it is suitable for your organisation.
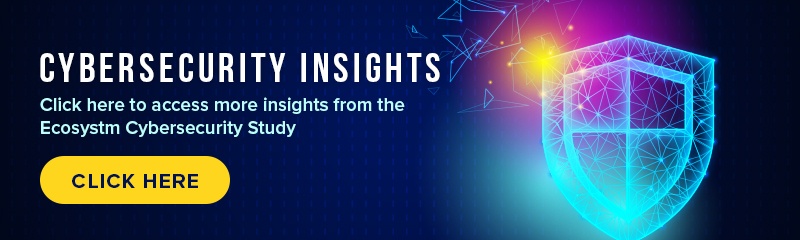