In AI’s early days, enterprise leaders asked a straightforward question: “What can this automate?” The focus was on speed, scale, and efficiency and AI delivered. But that question is evolving. Now, the more urgent ask is: “Can this AI understand people?”
This shift – from automation to emotional intelligence – isn’t just theoretical. It’s already transforming how organisations connect with customers, empower employees, and design digital experiences. We’re shifting to a phase of humanised AI – systems that don’t just respond accurately, but intuitively, with sensitivity to mood, tone, and need.
One of the most unexpected, and revealing, AI use cases is therapy. Millions now turn to AI chat tools to manage anxiety, process emotions, and share deeply personal thoughts. What started as fringe behaviour is fast becoming mainstream. This emotional turn isn’t a passing trend; it marks a fundamental shift in how people expect technology to relate to them.
For enterprises, this raises a critical challenge: If customers are beginning to turn to AI for emotional support, what kind of relationship do they expect from it? And what does it take to meet that expectation – not just effectively, but responsibly, and at scale?
The Rise of Chatbot Therapy
Therapy was never meant to be one of AI’s first mass-market emotional use cases; and yet, here we are.
Apps like Wysa, Serena, and Youper have been quietly reshaping the digital mental health landscape for years, offering on-demand support through chatbots. Designed by clinicians, these tools draw on established methods like Cognitive Behavioural Therapy (CBT) and mindfulness to help users manage anxiety, depression, and stress. The conversations are friendly, structured, and often, surprisingly helpful.
But something even more unexpected is happening; people are now using general-purpose AI tools like ChatGPT for therapeutic support, despite them not being designed for it. Increasingly, users are turning to ChatGPT to talk through emotions, navigate relationship issues, or manage daily stress. Reddit threads and social posts describe it being used as a therapist or sounding board. This isn’t Replika or Wysa, but a general AI assistant being shaped into a personal mental health tool purely through user behaviour.
This shift is driven by a few key factors. First, access. Traditional therapy is expensive, hard to schedule, and for many, emotionally intimidating. AI, on the other hand, is always available, listens without judgement, and never gets tired.
Tone plays a big role too. Thanks to advances in reinforcement learning and tone conditioning, models like ChatGPT are trained to respond with calm, non-judgmental empathy. The result feels emotionally safe; a rare and valuable quality for those facing anxiety, isolation, or uncertainty. A recent PLOS study found that not only did participants struggle to tell human therapists apart from ChatGPT, they actually rated the AI responses as more validating and empathetic.
And finally, and perhaps surprisingly, is trust. Unlike wellness apps that push subscriptions or ads, AI chat feels personal and agenda-free. Users feel in control of the interaction – no small thing in a space as vulnerable as mental health.
None of this suggests AI should replace professional care. Risks like dependency, misinformation, or reinforcing harmful patterns are real. But it does send a powerful signal to enterprise leaders: people now expect digital systems to listen, care, and respond with emotional intelligence.
That expectation is changing how organisations design experiences – from how a support bot speaks to customers, to how an internal wellness assistant checks in with employees during a tough week. Humanised AI is no longer a niche feature of digital companions. It’s becoming a UX standard; one that signals care, builds trust, and deepens relationships.
Digital Companionship as a Solution for Support
Ten years ago, talking to your AI meant asking Siri to set a reminder. Today, it might mean sharing your feelings with a digital companion, seeking advice from a therapy chatbot, or even flirting with a virtual persona! This shift from functional assistant to emotional companion marks more than a technological leap. It reflects a deeper transformation in how people relate to machines.
One of the earliest examples of this is Replika, launched in 2017, which lets users create personalised chatbot friends or romantic partners. As GenAI advanced, so did Replika’s capabilities, remembering past conversations, adapting tone, even exchanging voice messages. A Nature study found that 90% of Replika users reported high levels of loneliness compared to the general population, but nearly half said the app gave them a genuine sense of social support.
Replika isn’t alone. In China, Xiaoice (spun off from Microsoft in 2020) has hundreds of millions of users, many of whom chat with it daily for companionship. In elder care, ElliQ, a tabletop robot designed for seniors has shown striking results: a report from New York State’s Office for the Aging cited a 95% drop in loneliness among participants.
Even more freeform platforms like Character.AI, where users converse with AI personas ranging from historical figures to fictional characters, are seeing explosive growth. People are spending hours in conversation – not to get things done, but to feel seen, inspired, or simply less alone.
The Technical Leap: What Has Changed Since the LLM Explosion
The use of LLMs for code editing and content creation is already mainstream in most enterprises but use cases have expanded alongside the capabilities of new models. LLMs now have the capacity to act more human – to carry emotional tone, remember user preferences, and maintain conversational continuity.
Key advances include:
- Memory. Persistent context and long-term recall
- Reinforcement Learning from Human Feedback (RLHF). Empathy and safety by design
- Sentiment and Emotion Recognition. Reading mood from text, voice, and expression
- Role Prompting. Personas using brand-aligned tone and behaviour
- Multimodal Interaction. Combining text, voice, image, gesture, and facial recognition
- Privacy-Sensitive Design. On-device inference, federated learning, and memory controls
Enterprise Implications: Emotionally Intelligent AI in Action
The examples shared might sound fringe or futuristic, but they reveal something real: people are now open to emotional interaction with AI. And that shift is creating ripple effects. If your customer service chatbot feels robotic, it pales in comparison to the AI friend someone chats with on their commute. If your HR wellness bot gives stock responses, it may fall flat next to the AI that helped a user through a panic attack the night before.
The lesson for enterprises isn’t to mimic friendship or romance, but to recognise the rising bar for emotional resonance. People want to feel understood. Increasingly, they expect that even from machines.
For enterprises, this opens new opportunities to tap into both emotional intelligence and public comfort with humanised AI. Emerging use cases include:
- Customer Experience. AI that senses tone, adapts responses, and knows when to escalate
- Brand Voice. Consistent personality and tone embedded in AI interfaces
- Employee Wellness. Assistants that support mental health, coaching, and daily check-ins
- Healthcare & Elder Care. Companions offering emotional and physical support
- CRM & Strategic Communications. Emotion-aware tools that guide relationship building
Ethical Design and Guardrails
Emotional AI brings not just opportunity, but responsibility. As machines become more attuned to human feelings, ethical complexity grows. Enterprises must ensure transparency – users should always know they’re speaking to a machine. Emotional data must be handled with the same care as health data. Empathy should serve the user, not manipulate them. Healthy boundaries and human fallback must be built in, and organisations need to be ready for regulation, especially in sensitive sectors like healthcare, finance, and education.
Emotional intelligence is no longer just a human skill; it’s becoming a core design principle, and soon, a baseline expectation.
Those who build emotionally intelligent AI with integrity can earn trust, loyalty, and genuine connection at scale. But success won’t come from speed or memory alone – it will come from how the experience makes people feel.
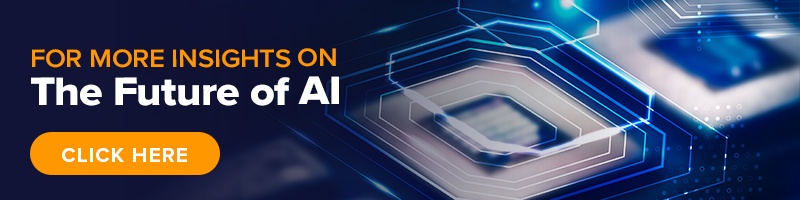
AI is not just reshaping how businesses operate — it’s redefining the CFO’s role at the centre of value creation, risk management, and operational leadership.
As stewards of capital, CFOs must cut through the hype and ensure AI investments deliver measurable business returns. As guardians of risk and compliance, they must shield their organisations from new threats — from algorithmic bias to data privacy breaches with heavy financial and reputational costs. And as leaders of their function, CFOs now have a generational opportunity to modernise finance, champion AI adoption, and build teams ready for an AI-powered future.
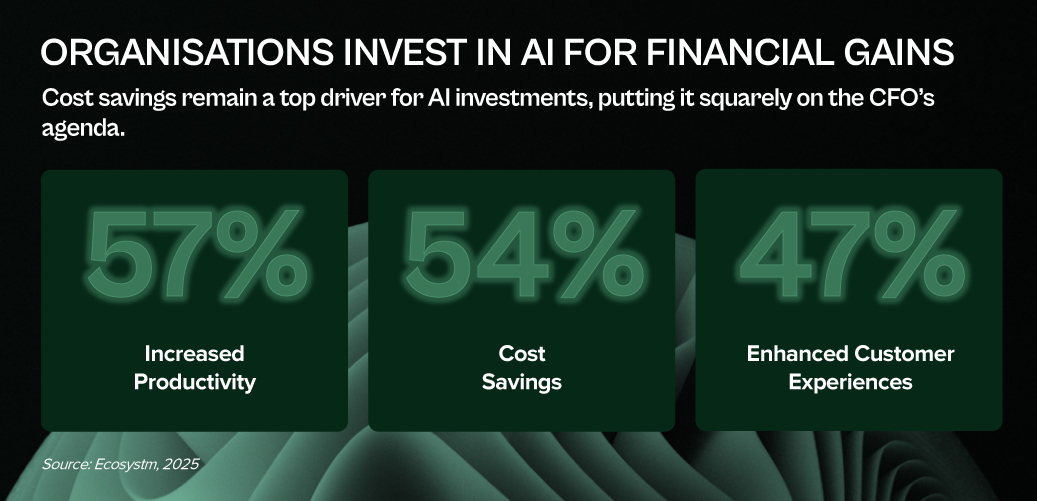
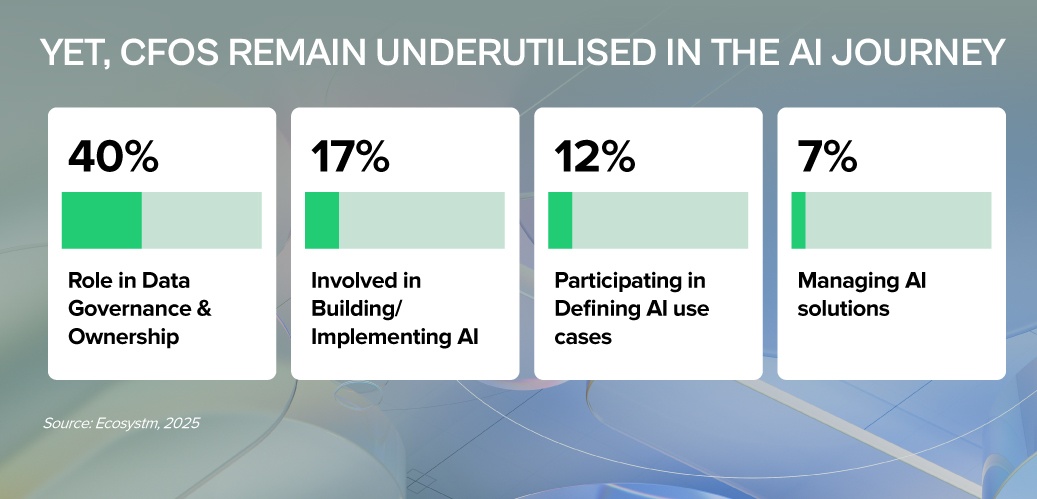
LEAD WITH RIGOUR. SAFEGUARD WITH VIGILANCE. CHAMPION WITH VISION.
That’s the CFO playbook for AI success.
Click here to download “AI Stakeholders: The Finance Perspective” as a PDF.
1. Investor & ROI Gatekeeper: Ensuring AI Delivers Value
CFOs must scrutinise AI investments with the same discipline as any major capital allocation.
- Demand Clear Business Cases. Every AI initiative should articulate the problem solved, expected gains (cost, efficiency, accuracy), and specific KPIs.
- Prioritise Tangible ROI. Focus on AI projects that show measurable impact. Start with high-return, lower-risk use cases before scaling.
- Assess Total Cost of Ownership (TCO). Go beyond upfront costs – factor in integration, maintenance, training, and ongoing AI model management.
Only 37% of Asia Pacific organisations invest in FinOps to cut costs, boost efficiency, and strengthen financial governance over tech spend.
2. Risk & Compliance Steward: Navigating AI’s New Risk Landscape
AI brings significant regulatory, compliance, and reputational risks that CFOs must manage – in partnership with peers across the business.
- Champion Data Quality & Governance. Enforce rigorous data standards and collaborate with IT, risk, and business teams to ensure accuracy, integrity, and compliance across the enterprise.
- Ensure Data Accessibility. Break down silos with CIOs and CDOs and invest in shared infrastructure that AI initiatives depend on – from data lakes to robust APIs.
- Address Bias & Safeguard Privacy. Monitor AI models to detect bias, especially in sensitive processes, while ensuring compliance.
- Protect Security & Prevent Breaches. Strengthen defences around financial and personal data to avoid costly security incidents and regulatory penalties.
3. AI Champion & Business Leader: Driving Adoption in Finance
Beyond gatekeeping, CFOs must actively champion AI to transform finance operations and build future-ready teams.
- Identify High-Impact Use Cases. Work with teams to apply AI where it solves real pain points – from automating accounts payable to improving forecasting and fraud detection.
- Build AI Literacy. Help finance teams see AI as an augmentation tool, not a threat. Invest in upskilling while identifying gaps – from data management to AI model oversight.
- Set AI Governance Frameworks. Define accountability, roles, and control mechanisms to ensure responsible AI use across finance.
- Stay Ahead of the Curve. Monitor emerging tech that can streamline finance and bring in expert partners to fast-track AI adoption and results.
CFOs: From Gatekeepers to Growth Drivers
AI is not just a tech shift – it’s a CFO mandate. To lead, CFOs must embrace three roles: Investor, ensuring every AI bet delivers real ROI; Risk Guardian, protecting data integrity and compliance in a world of new risks; and AI Champion, embedding AI into finance teams to boost speed, accuracy, and insight.
This is how finance moves from record-keeping to value creation. With focused leadership and smart collaboration, CFOs can turn AI from buzzword to business impact.
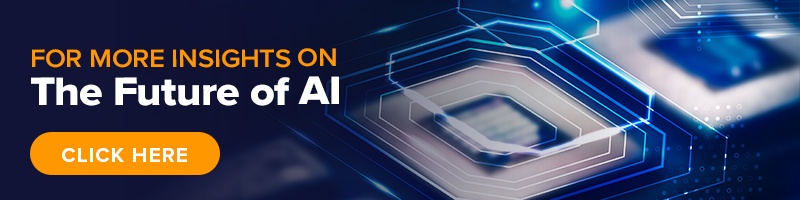
AI has rapidly transitioned from a theoretical concept to a strategic imperative, reshaping core business functions and fundamentally altering the operational landscape of technology teams. By empowering teams with increased autonomy and data-driven capabilities, organisations are positioned to realise substantial value and achieve a decisive competitive advantage.
The most profound impact of AI can be observed within tech teams. AI-driven automation of routine tasks and streamlined operations are enabling technology professionals to refocus their efforts on strategic initiatives. This shift transforms the technology function from a reactive system maintenance role to a proactive developer of intelligent infrastructure and future-oriented systems.
Ecosystm research reveals key findings that Tech Leaders need to know.
Click here to download “AI Stakeholders: The Tech Leader’s Perspective” as a PDF.
Strategic AI Deployment
Ecosystm research reveals a clear trend: technology leaders are strategically investing in the immense potential of AI. While 61% currently leverage AI for IT support and helpdesk automation, there is a clear aspiration for broader deployment across infrastructure, development, and security. 80% are prioritising cloud resource allocation and optimisation, followed by 76% focusing on network optimisation and performance monitoring, along with significant interest in software development and testing, and cyber threat detection.
One Infrastructure Leader shared that the organisation uses AI to dynamically scale infrastructure while automating maintenance to prevent outages. This approach has led to unprecedented efficiency and freed up their teams for more strategic work. The leader emphasised that AI is helping to tackle complex infrastructure challenges and is key to achieving operational excellence.
A Cyber Leader discussed the role of AI in enhancing their defense capabilities. While not a “silver bullet,” it is a powerful tool in the fight against cyber threats. AI significantly enhances threat intelligence and fraud analysis, complementing, rather than replacing, security team efforts. This integration has helped streamline security operations and improve the ability to respond to emerging risks.
AI is also making waves in software development. A Data Science Leader explained how AI quality control tools have reduced bug counts by 30%, enabling faster release cycles and a 10% improvement in internal customer satisfaction.
Collaborative AI Implementation: A Cross-Functional Approach
The successful implementation of AI requires a collaborative, cross-functional approach. The responsibility for identifying viable use cases, developing and maintaining systems, and ensuring robust data governance is distributed among various technology leadership roles. CIOs, in collaboration with business stakeholders, define strategic use cases, considering infrastructure requirements. Data Science Leaders bridge the gap between AI’s technical capabilities and practical business applications. CISOs safeguard data, while CIOs manage the systems that store and organise it.
Navigating Challenges, Prioritising Strategic AI Initiatives
Despite the acknowledged potential of AI, technology leaders must address several critical challenges, including use case prioritisation, skill gaps, and the development of comprehensive AI strategies. Nevertheless, the strategic importance of AI will continue to drive its prioritisation in 2025. Key anticipated outcomes include increased technology team productivity (56%) and technology cost optimisation (53%).
AI is no longer a supplementary tool but a core strategic asset. By strategically integrating AI, technology teams are transitioning from operational support to strategic innovation, building the intelligent systems that will define the future of business.
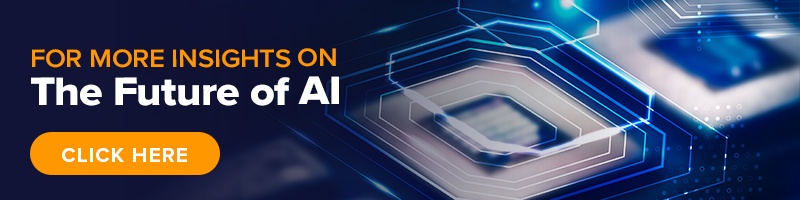
Operations leaders are on the front lines of the AI revolution. They see the transformative potential of AI and are actively driving its adoption to streamline processes, boost efficiency, and unlock new levels of performance. The value is clear: AI is no longer a futuristic concept, but a present-day necessity.
Over the past two years, Ecosystm’s research – including surveys and deep dives with business and tech leaders has confirmed this: AI is the dominant theme.
Here are some insights for Operations Leaders from our research.
Click here to download “AI Stakeholders: The Operations Perspective” as a PDF
From Streamlined Workflows to Smarter Decisions
AI is already making a tangible difference in operations. A significant 60% of operations leaders are currently leveraging AI for intelligent document processing, freeing up valuable time and resources. But this is just the beginning. The vision extends far beyond, with plans to expand AI’s reach into crucial areas like workflow analysis, fraud detection, and streamlining risk and compliance processes. Imagine AI optimising transportation routes in real-time, predicting equipment maintenance needs before they arise, or automating complex scheduling tasks. This is the operational reality AI is creating.
Real-World Impact, Real-World Examples
The impact of AI is not just theoretical. Operations leaders are witnessing firsthand how AI is driving tangible improvements. “With AI-powered vision and sensors, we’ve boosted efficiency, accuracy, and safety in our manufacturing processes,” shares one leader. Others highlight the security benefits: “From fraud detection to claims processing, AI is safeguarding our transactions and improving trust in our services.” Even complex logistical challenges are being conquered: “Our AI-driven logistics solution has cut costs, saved time, and turned complex operations into seamless processes.” These real-world examples showcase the power of AI to deliver concrete results across diverse operational functions.
Operations Takes a Seat at the AI Strategy Table (But Faces Challenges)
With 54% of organisations prioritising cost savings from AI, operations leaders are rightfully taking a seat at the AI strategy table, shaping use cases and driving adoption. A remarkable 56% of operations leaders are actively involved in defining high-value AI applications. However, a disconnect exists. Despite their influence on AI strategy, only a small fraction (7%) of operations leaders have direct data governance responsibilities. This lack of control over the very fuel that powers AI – data – creates a significant hurdle.
Further challenges include data access across siloed systems, limiting the ability to gain a holistic view, difficulty in identifying and prioritising the most impactful AI use cases, and persistent skills shortages. These barriers, while significant, are not deterring operations leaders.
The Future is AI-Driven
Despite these challenges, operations leaders are doubling down on AI. A striking 7 out of 10 plan to prioritise AI investments in 2025, driven by the pursuit of greater cost savings. And the biggest data effort on the horizon? Identifying and prioritising better use cases for AI. This focus on practical applications demonstrates a clear understanding: the future of operations is inextricably linked to the power of AI. By addressing the challenges they face and focusing on strategic implementation, operations leaders are poised to unlock the full potential of AI and transform their organisations.
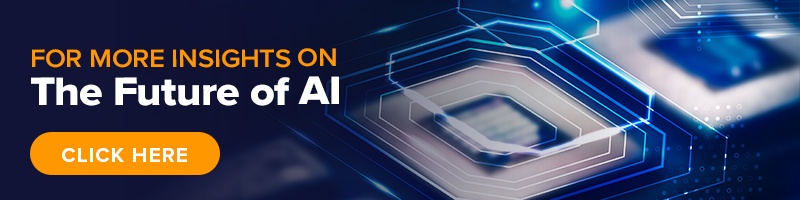
AI has broken free from the IT department. It’s no longer a futuristic concept but a present-day reality transforming every facet of business. Departments across the enterprise are now empowered to harness AI directly, fuelling innovation and efficiency without waiting for IT’s stamp of approval. The result? A more agile, data-driven organisation where AI unlocks value and drives competitive advantage.
Ecosystm’s research over the past two years, including surveys and in-depth conversations with business and technology leaders, confirms this trend: AI is the dominant theme. And while the potential is clear, the journey is just beginning.
Here are key AI insights for HR Leaders from our research.
Click here to download “AI Stakeholders: The HR Perspective” as a PDF.
HR: Leading the Charge (or Should Be)
Our research reveals a fascinating dynamic in HR. While 54% of HR leaders currently use AI for recruitment (scanning resumes, etc.), their vision extends far beyond. A striking majority plan to expand AI’s reach into crucial areas: 74% for workforce planning, 68% for talent development and training, and 62% for streamlining employee onboarding.
The impact is tangible, with organisations already seeing significant benefits. GenAI has streamlined presentation creation for bank employees, allowing them to focus on content rather than formatting and improving efficiency. Integrating GenAI into knowledge bases has simplified access to internal information, making it quicker and easier for employees to find answers. AI-driven recruitment screening is accelerating hiring in the insurance sector by analysing resumes and applications to identify top candidates efficiently. Meanwhile, AI-powered workforce management systems are transforming field worker management by optimising job assignments, enabling real-time tracking, and ensuring quick responses to changes.
The Roadblocks and the Opportunity
Despite this promising outlook, HR leaders face significant hurdles. Limited exploration of use cases, the absence of a unified organisational AI strategy, and ethical concerns are among the key barriers to wider AI deployments.
Perhaps most concerning is the limited role HR plays in shaping AI strategy. While 57% of tech and business leaders cite increased productivity as the main driver for AI investments, HR’s influence is surprisingly weak. Only 20% of HR leaders define AI use cases, manage implementation, or are involved in governance and ownership. A mere 8% primarily manage AI solutions.
This disconnect represents a massive opportunity.
2025 and Beyond: A Call to Action for HR
Despite these challenges, our research indicates HR leaders are prioritising AI for 2025. Increased productivity is the top expected outcome, while three in ten will focus on identifying better HR use cases as part of a broader data-centric approach.
The message is clear: HR needs to step up and claim its seat at the AI table. By proactively defining use cases, championing ethical considerations, and collaborating closely with tech teams, HR can transform itself into a strategic driver of AI adoption, unlocking the full potential of this transformative technology for the entire organisation. The future of HR is intelligent, and it’s time for HR leaders to embrace it.
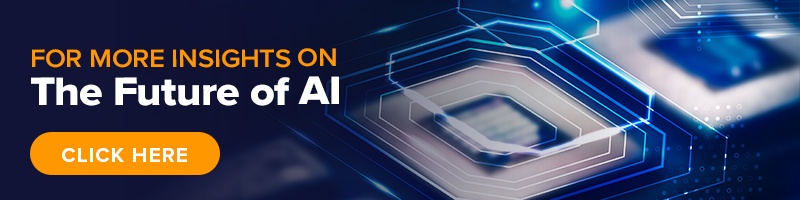
Over the past year, Ecosystm has conducted extensive research, including surveys and in-depth conversations with industry leaders, to uncover the most pressing topics and trends. And unsurprisingly, AI emerged as the dominant theme.
Here are some insights from our research.
Click here to download ‘AI in BFSI: Success Stories & Insights’ as a PDF
From personalised recommendations to streamlined operations, AI is transforming the products, services and processes in the BFSI industries. While leaders realise that AI holds significant potential, turning that potential into reality is often tough. Many BFSI organisations struggle to move beyond AI pilots because of some key barriers.
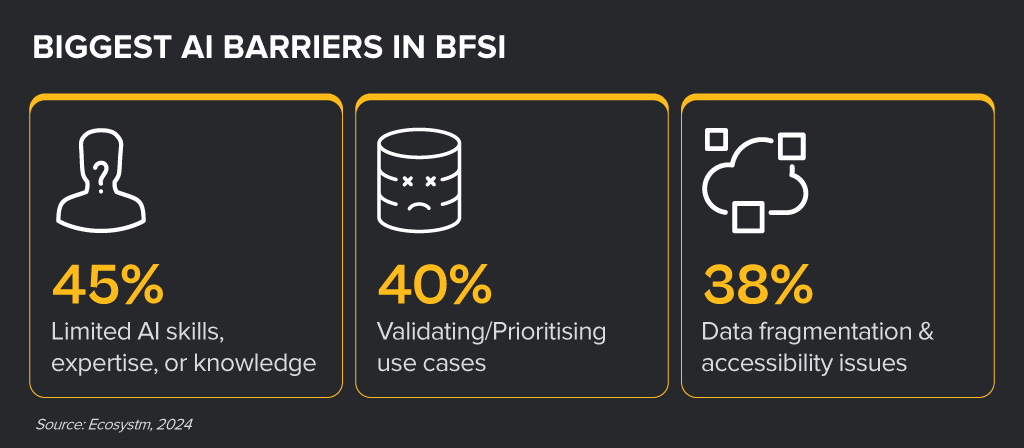
Despite the challenges, BFSI organisations are witnessing early AI success in these 3 areas:
- 1. Customer Service & Engagement
- 2. Risk Management & Fraud Detection
- 3. Process Automation & Efficiency
Customer Service & Engagement Use Cases
- Virtual Assistants and Chatbots. Delivering real-time product information and customer support
- Customer Experience Analysis. Analysing data to uncover trends and improve user experiences
- Personalised Recommendations. Providing tailored financial products based on user behaviour and preferences
“While we remain cautious about customer-facing applications, many of our AI use cases provide valuable customer insights to our employees. Human-in-the-loop is still a critical consideration.” – INSURANCE CX LEADER
Risk Management & Fraud Detection Use Cases
- Enhanced Credit Scoring. Improved assessment of creditworthiness and risks
- Advanced Fraud Detection. Easier detection and prevention of fraudulent activities
- Comprehensive Risk Strategy. Assessment of risk factors to develop effective strategies
“We deployed enterprise-grade AI models that are making a significant impact in specialised areas like credit decisioning and risk modelling.” – BANKING DATA LEADER
Process Automation and Efficiency
- Backend Process Streamlining. Automating workflows and processes to boost efficiency
- Loan & Claims Processing. Speeding up application and approval processes
- Invoice Processing. Automating invoice management to minimise errors
“Our focus is on creating a mindset where employees see AI as a tool that can augment their capabilities rather than replace them.” – BANKING COO
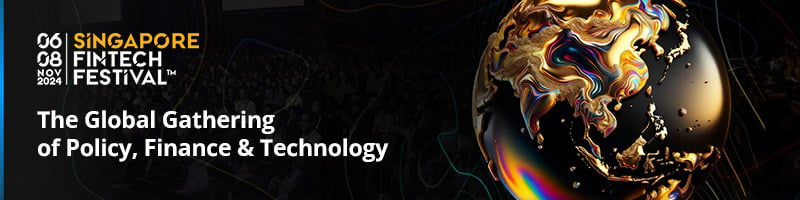
Large organisations in the banking and financial services industry have come a long way over the past two decades in cutting costs, restructuring IT systems and redefining customer relationship management. And, as if that was not enough, they now face the challenge of having to adapt to ongoing global technological shifts or the challenge of having to “do something with AI” without being AI-ready in terms of strategy, skills and culture.
Most organisations in the industry have started approaching AI implementation in a conventional way, based on how they have historically managed IT initiatives. Their first attempts at experimenting with AI have led to rapid conclusions forming seven common myths. However, as experience with AI grows, these myths are gradually being debunked. Let us put these myths to a reality check.
1. We can rely solely on external tech companies
Even in a highly regulated industry like banking and financial services, internal processes and data management practices can vary significantly from one institution to another. Experience shows that while external providers – many of whom lack direct industry experience – can offer solutions tailored to the more obvious use cases and provide customisation, they fall short when it comes to identifying less apparent opportunities and driving fundamental changes in workflows. No one understands an institution’s data better than its own employees. Therefore, a key success factor in AI implementation is active internal ownership, involving employees directly rather than delegating the task entirely to external parties. While technology providers are essential partners, organisations must also cultivate their own internal understanding of AI to ensure successful implementation.
2. AI is here to be applied to single use cases
In the early stages of experimenting with AI, many financial institutions treated it as a side project, focusing on developing minimum viable products and solving isolated problems to explore what worked and what didn’t. Given their inherently risk-averse nature, organisations often approached AI cautiously, addressing one use case at a time to avoid disrupting their broader IT landscape or core business. However, with AI’s potential for deep transformation, the financial services industry has an opportunity not only to address inefficiencies caused by manual, time-consuming tasks but also to question how data is created, captured, and used from the outset. This requires an ecosystem of visionary minds in the industry who join forces and see beyond deal generation.
3. We can staff AI projects with our highly motivated junior employees and let our senior staff focus on what they do best – managing the business
Financial institutions that still view AI as a side hustle, secondary to their day-to-day operations, often assign junior employees to handle AI implementation. However, this can be a mistake. AI projects involve numerous small yet critical decisions, and team members need the authority and experience to make informed judgments that align with the organisation’s goals. Also, resistance to change often comes from those who were not involved in shaping or developing the initiative. Experience shows that project teams with a balanced mix of seniority and diversity in perspectives tend to deliver the best results, ensuring both strategic insight and operational engagement.
4. AI projects do not pay off
Compared to conventional IT projects, the business cases for AI implementation – especially when limited to solving a few specific use cases – often do not pay off over a period of two to three years. Traditional IT projects can usually be executed with minimal involvement of subject matter experts, and their costs are easier to estimate based on reference projects. In contrast, AI projects are highly experimental, requiring multiple iterations, significant involvement from experts, and often lacking comparable reference projects. When AI solutions address only small parts of a process, the benefits may not be immediately apparent. However, if AI is viewed as part of a long-term transformational journey, gradually integrating into all areas of the organisation and unlocking new business opportunities over the next five to ten years, the true value of AI becomes clear. A conventional business case model cannot fully capture this long-term payoff.
5. We are on track with AI if we have several initiatives ongoing
Many financial institutions have begun their AI journey by launching multiple, often unrelated, use case-based projects. The large number of initiatives can give top management a false sense of progress, as if they are fully engaged in AI. However, investors and project teams often ask key questions: Where are these initiatives leading? How do they contribute? What is the AI vision and strategy, and how does it align with the business strategy? If these answers remain unclear, it’s difficult to claim that the organisation is truly on track with AI. To ensure that AI initiatives are truly impactful and aligned with business objectives, organisations must have a clear AI vision and strategy – and not rely on number of initiatives to measure progress.
6. AI implementation projects always exceed their deadlines
AI solutions in the banking and financial services industry are rarely off-the-shelf products. In cases of customisation or in-house development, particularly when multiple model-building iterations and user tests are required, project delays of three to nine months can occur. This is largely because organisations want to avoid rolling out solutions that do not perform reliably. The goal is to ensure that users have a positive experience with AI and embrace the change. Over time, as an organisation becomes more familiar with AI implementation, the process will become faster.
7. We upskill our people by giving them access to AI training
Learning by doing has always been and will remain the most effective way to learn, especially with technology. Research has shown that 90% of knowledge acquired in training is forgotten after a week if it is not applied. For organisations, the best way to digitally upskill employees is to involve them in AI implementation projects, even if it’s just a few hours per week. To evaluate their AI readiness or engagement, organisations could develop new KPIs, such as the average number of hours an employee actively engages in AI implementation or the percentage of employees serving as subject matter experts in AI projects.
Which of these myths have you believed, and where do you already see changes?
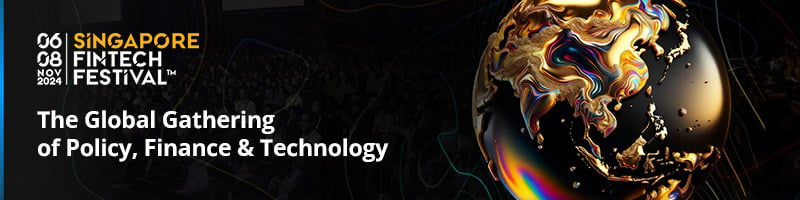
The White House has mandated federal agencies to conduct risk assessments on AI tools and appoint officers, including Chief Artificial Intelligence Officers (CAIOs), for oversight. This directive, led by the Office of Management and Budget (OMB), aims to modernise government AI adoption and promote responsible use. Agencies must integrate AI oversight into their core functions, ensuring safety, security, and ethical use. CAIOs will be tasked with assessing AI’s impact on civil rights and market competition. Agencies have until December 1, 2024, to address non-compliant AI uses, emphasising swift implementation.
How will this impact global AI adoption? Ecosystm analysts share their views.
Click here to download ‘Ensuring Ethical AI: US Federal Agencies’ New Mandate’ as a PDF.
The Larger Impact: Setting a Global Benchmark

This sets a potential global benchmark for AI governance, with the U.S. leading the way in responsible AI use, inspiring other nations to follow suit. The emphasis on transparency and accountability could boost public trust in AI applications worldwide.
The appointment of CAIOs across U.S. federal agencies marks a significant shift towards ethical AI development and application. Through mandated risk management practices, such as independent evaluations and real-world testing, the government recognises AI’s profound impact on rights, safety, and societal norms.
This isn’t merely a regulatory action; it’s a foundational shift towards embedding ethical and responsible AI at the heart of government operations. The balance struck between fostering innovation and ensuring public safety and rights protection is particularly noteworthy.
This initiative reflects a deep understanding of AI’s dual-edged nature – the potential to significantly benefit society, countered by its risks.
The Larger Impact: Blueprint for Risk Management

In what is likely a world first, AI brings together technology, legal, and policy leaders in a concerted effort to put guardrails around a new technology before a major disaster materialises. These efforts span from technology firms providing a form of legal assurance for use of their products (for example Microsoft’s Customer Copyright Commitment) to parliaments ratifying AI regulatory laws (such as the EU AI Act) to the current directive of installing AI accountability in US federal agencies just in the past few months.
It is universally accepted that AI needs risk management to be responsible and acceptable – installing an accountable C-suite role is another major step of AI risk mitigation.
This is an interesting move for three reasons:
- The balance of innovation versus governance and risk management.
- Accountability mandates for each agency’s use of AI in a public and transparent manner.
- Transparency mandates regarding AI use cases and technologies, including those that may impact safety or rights.
Impact on the Private Sector: Greater Accountability

AI Governance is one of the rare occasions where government action moves faster than private sector. While the immediate pressure is now on US federal agencies (and there are 438 of them) to identify and appoint CAIOs, the announcement sends a clear signal to the private sector.
Following hot on the heels of recent AI legislation steps, it puts AI governance straight into the Boardroom. The air is getting very thin for enterprises still in denial that AI governance has advanced to strategic importance. And unlike the CFC ban in the Eighties (the Montreal protocol likely set the record for concerted global action) this time the technology providers are fully onboard.
There’s no excuse for delaying the acceleration of AI governance and establishing accountability for AI within organisations.
Impact on Tech Providers: More Engagement Opportunities

Technology vendors are poised to benefit from the medium to long-term acceleration of AI investment, especially those based in the U.S., given government agencies’ preferences for local sourcing.
In the short term, our advice to technology vendors and service partners is to actively engage with CAIOs in client agencies to identify existing AI usage in their tools and platforms, as well as algorithms implemented by consultants and service partners.
Once AI guardrails are established within agencies, tech providers and service partners can expedite investments by determining which of their platforms, tools, or capabilities comply with specific guardrails and which do not.
Impact on SE Asia: Promoting a Digital Innovation Hub

By 2030, Southeast Asia is poised to emerge as the world’s fourth-largest economy – much of that growth will be propelled by the adoption of AI and other emerging technologies.
The projected economic growth presents both challenges and opportunities, emphasizing the urgency for regional nations to enhance their AI governance frameworks and stay competitive with international standards. This initiative highlights the critical role of AI integration for private sector businesses in Southeast Asia, urging organizations to proactively address AI’s regulatory and ethical complexities. Furthermore, it has the potential to stimulate cross-border collaborations in AI governance and innovation, bridging the U.S., Southeast Asian nations, and the private sector.
It underscores the global interconnectedness of AI policy and its impact on regional economies and business practices.
By leading with a strategic approach to AI, the U.S. sets an example for Southeast Asia and the global business community to reevaluate their AI strategies, fostering a more unified and responsible global AI ecosystem.
The Risks

U.S. government agencies face the challenge of sourcing experts in technology, legal frameworks, risk management, privacy regulations, civil rights, and security, while also identifying ongoing AI initiatives. Establishing a unified definition of AI and cataloguing processes involving ML, algorithms, or GenAI is essential, given AI’s integral role in organisational processes over the past two decades.
However, there’s a risk that focusing on AI governance may hinder adoption.
The role should prioritise establishing AI guardrails to expedite compliant initiatives while flagging those needing oversight. While these guardrails will facilitate “safe AI” investments, the documentation process could potentially delay progress.
The initiative also echoes a 20th-century mindset for a 21st-century dilemma. Hiring leaders and forming teams feel like a traditional approach. Today, organisations can increase productivity by considering AI and automation as initial solutions. Investing more time upfront to discover initiatives, set guardrails, and implement AI decision-making processes could significantly improve CAIO effectiveness from the outset.
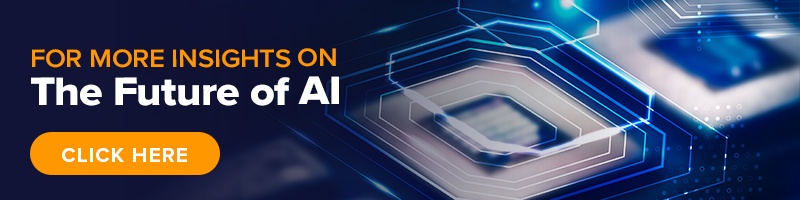
In 2024, business and technology leaders will leverage the opportunity presented by the attention being received by Generative AI engines to test and integrate AI comprehensively across the business. Many organisations will prioritise the alignment of their initial Generative AI initiatives with broader AI strategies, establishing distinct short-term and long-term goals for their AI investments.
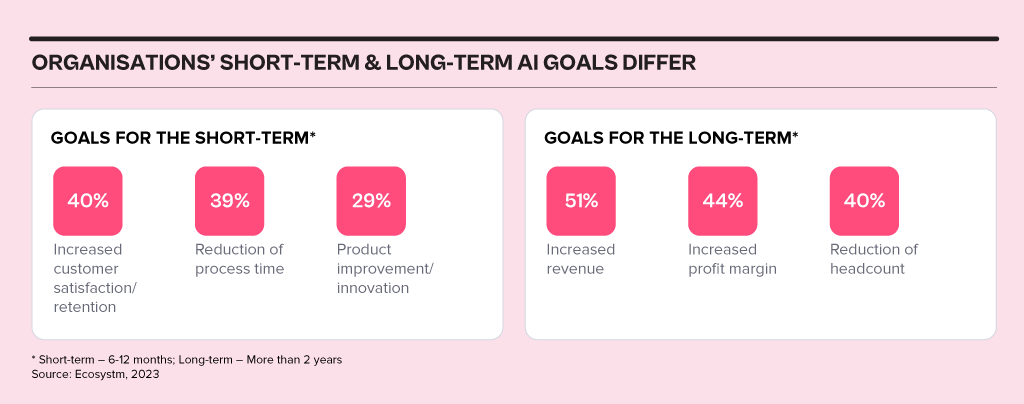
AI adoption will influence business processes, technology skills, and, in turn, reshape the product/service offerings of AI providers.
Ecosystm analysts Achim Granzen, Peter Carr, Richard Wilkins, Tim Sheedy, and Ullrich Loeffler present the top 5 AI trends in 2024.
Click here to download ‘Ecosystm Predicts: Top 5 AI Trends in 2024.
#1 By the End of 2024, Gen AI Will Become a ‘Hygiene Factor’ for Tech Providers
AI has widely been commended as the ‘game changer’ that will create and extend the divide between adopters and laggards and be the deciding factor for success and failure.
Cutting through the hype, strategic adoption of AI is still at a nascent stage and 2024 will be another year where companies identify use cases, experiment with POCs, and commit renewed efforts to get their data assets in order.
The biggest impact of AI will be derived from integrated AI capability in standard packaged software and products – and this will include Generative AI. We will see a plethora of product releases that seamlessly weave Generative AI into everyday tools generating new value through increased efficiency and user-friendliness.
Technology will be the first industry where AI becomes the deciding factor between success and failure; tech providers will be forced to deliver on their AI promises or be left behind.
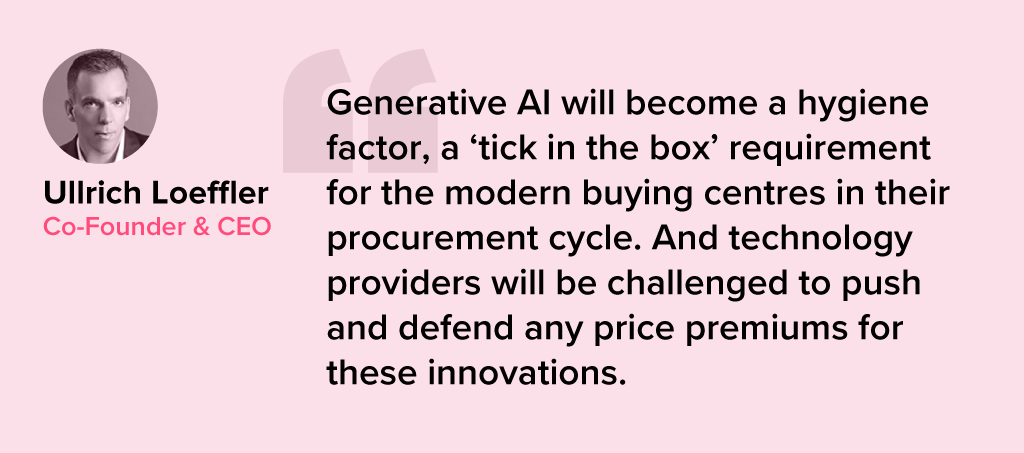
#2 Gen AI Will Disrupt the Role of IT Architects
Traditionally, IT has relied on three-tier architectures for applications, that faced limitations in scalability and real-time responsiveness. The emergence of microservices, containerisation, and serverless computing has paved the way for event-driven designs, a paradigm shift that decouples components and use events like user actions or data updates as triggers for actions across distributed services. This approach enhances agility, scalability, and flexibility in the system.
The shift towards event-driven designs and advanced architectural patterns presents a compelling challenge for IT Architects, as traditionally their role revolved around designing, planning and overseeing complex systems.
Generative AI is progressively demonstrating capabilities in architectural design through pattern recognition, predictive analytics, and automated decision-making.
With the adoption of Generative AI, the role of an IT Architect will change into a symbiotic relationship where human expertise collaborates with AI insights.
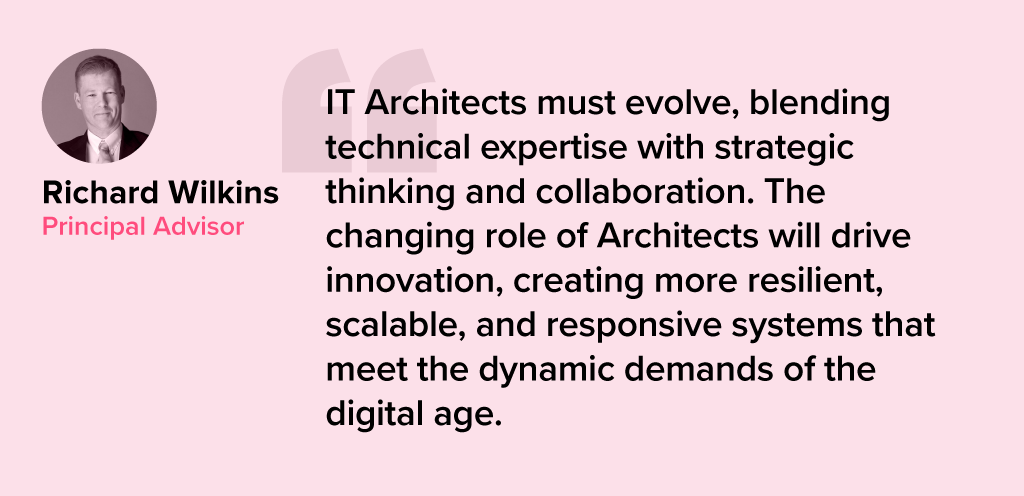
#3 Gen AI Adoption Will be Confined to Specific Use Cases
A little over a year ago, a new era in AI began with the initial release of OpenAI’s ChatGPT. Since then, many organisations have launched Generative AI pilots.
In its second-year enterprises will start adoption – but in strictly defined and limited use cases. Examples such as Microsoft Copilot demonstrate an early adopter route. While productivity increases for individuals can be significant, its enterprise impact is unclear (at this time).
But there are impactful use cases in enterprise knowledge and document management. Organisations across industries have decades (or even a century) of information, including digitised documents and staff expertise. That treasure trove of information can be made accessible through cognitive search and semantic answering, driven by Generative AI.
Generative AI will provide organisations with a way to access, distill, and create value out of that data – a task that may well be impossible to achieve in any other way.
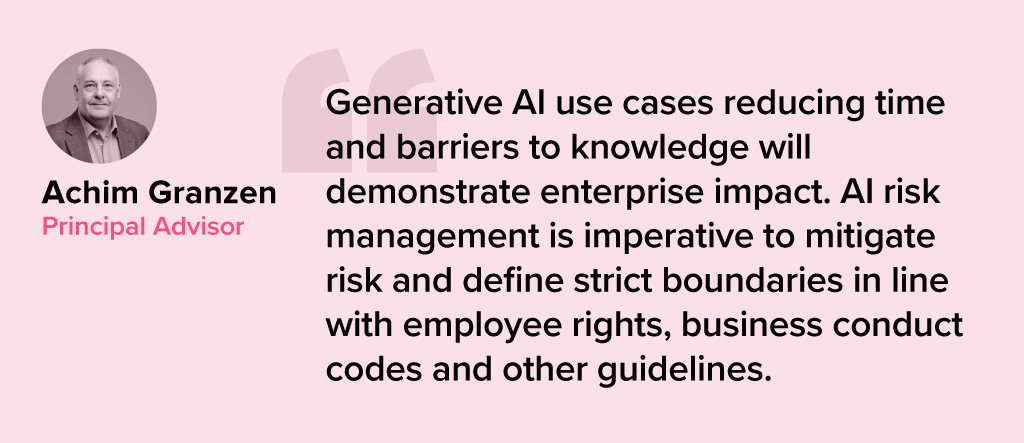
#4 Gen AI Will Get Press Inches; ‘Traditional’ AI Will Do the Hard Work
While the use cases for Generative AI will continue to expand, the deployment models and architectures for enterprise Generative AI do not add up – yet.
Running Generative AI in organisations’ data centres is costly and using public models for all but the most obvious use cases is too risky. Most organisations opt for a “small target” strategy, implementing Generative AI in isolated use cases within specific processes, teams, or functions. Justifying investment in hardware, software, and services for an internal AI platform is challenging when the payback for each AI initiative is not substantial.
“Traditional AI/ML” will remain the workhorse, with a significant rise in use cases and deployments. Organisations are used to investing for AI by individual use cases. Managing process change and training is also more straightforward with traditional AI, as the changes are implemented in a system or platform, eliminating the need to retrain multiple knowledge workers.
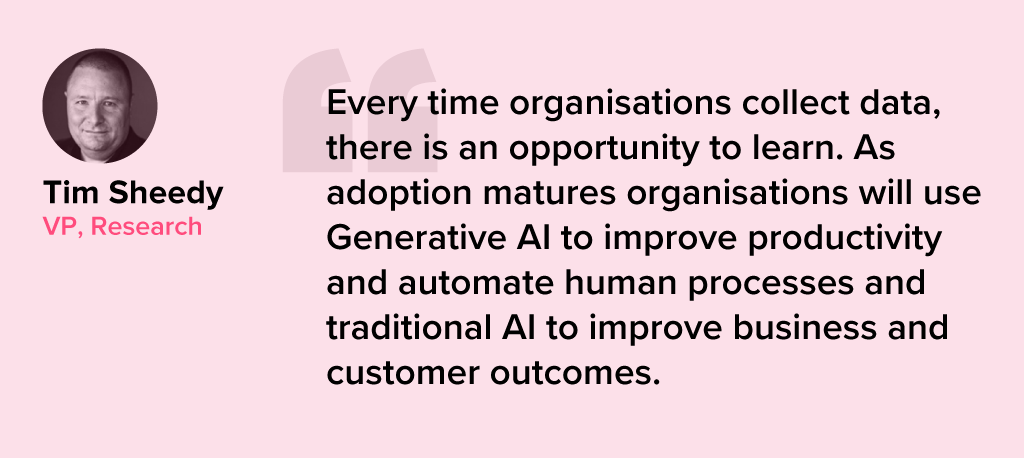
#5 AI Will Pioneer a 21st Century BPM Renaissance
As we near the 25-year milestone of the 21st century, it becomes clear that many businesses are still operating with 20th-century practices and philosophies.
AI, however, represents more than a technological breakthrough; it offers a new perspective on how businesses operate and is akin to a modern interpretation of Business Process Management (BPM). This development carries substantial consequences for digital transformation strategies. To fully exploit the potential of AI, organisations need to commit to an extensive and ongoing process spanning the collection, organisation, and expansion of data, to integrating these insights at an application and workflow level.
The role of AI will transcend technological innovation, becoming a driving force for substantial business transformation. Sectors that specialise in workflow, data management, and organisational transformation are poised to see the most growth in 2024 because of this shift.
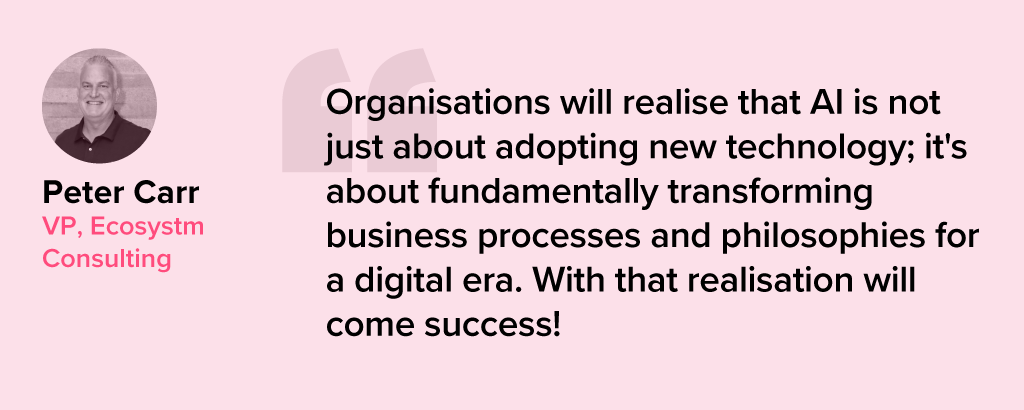
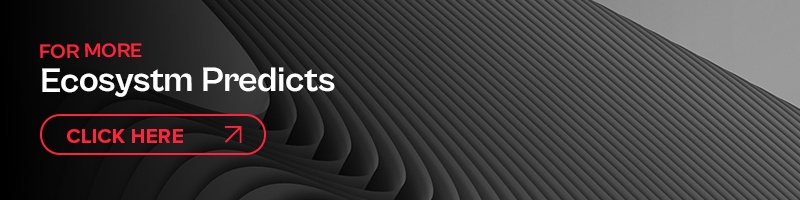