Cities worldwide have been facing unexpected challenges since 2020 – and 2022 will see them continue to struggle with the after-effects of COVID-19. However, there is one thing that governments have learnt during this ongoing crisis – technology is not the only aspect of a Cities of the Future initiative. Besides technology, Cities of the Future will start revisiting organisational and institutional structures, prioritise goals, and design and deploy an architecture with data as its foundation.
Cities of the Future will focus on being:
- Safe. Driven by the ongoing healthcare crisis
- Secure. Driven by the multiple cyber attacks on critical infrastructure
- Sustainable. Driven by citizen consciousness and global efforts such as the COP26
- Smart. Driven by the need to be agile to face future uncertainties
Read on to find out what Ecosystm Advisors, Peter Carr, Randeep Sudan, Sash Mukherjee and Tim Sheedy think will be the leading Cities of the Future trends for 2022.
Click here to download Ecosystm Predicts: The Top 5 Trends for Cities of the Future in 2022
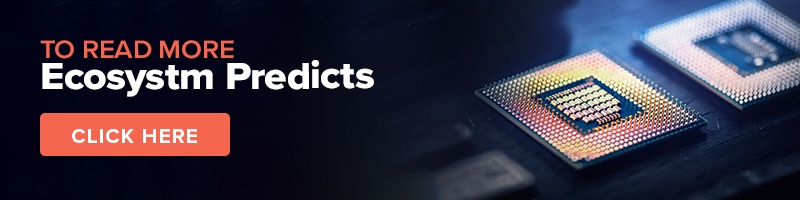
In this blog, our guest author Shameek Kundu talks about the importance of making AI/ machine learning models reliable and safe. “Getting data and algorithms right has always been important, particularly in regulated industries such as banking, insurance, life sciences and healthcare. But the bar is much higher now: more data, from more sources, in more formats, feeding more algorithms, with higher stakes.”
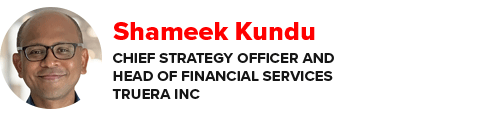
Building trust in algorithms is essential. Not (just) because regulators want it, but because it is good for customers and business. The good news is that with the right approach and tooling, it is also achievable.
Getting data and algorithms right has always been important, particularly in regulated industries such as banking, insurance, life sciences and healthcare. But the bar is much higher now: more data, from more sources, in more formats, feeding more algorithms, with higher stakes. With the increased use of Artificial Intelligence/ Machine Learning (AI/ML), today’s algorithms are also more powerful and difficult to understand.
A false dichotomy
At this point in the conversation, I get one of two reactions. One is of distrust in AI/ML and a belief that it should have little role to play in regulated industries. Another is of nonchalance; after all, most of us feel comfortable using ‘black-boxes’ (e.g., airplanes, smartphones) in our daily lives without being able to explain how they work. Why hold AI/ML to special standards?
Both make valid points. But the skeptics miss out on the very real opportunity cost of not using AI/ML – whether it is living with historical biases in human decision-making or simply not being able to do things that are too complex for a human to do, at scale. For example, the use of alternative data and AI/ML has helped bring financial services to many who have never had access before.
On the other hand, cheerleaders for unfettered use of AI/ML might be overlooking the fact that a human being (often with a limited understanding of AI/ML) is always accountable for and/ or impacted by the algorithm. And fairly or otherwise, AI/ML models do elicit concerns around their opacity – among regulators, senior managers, customers and the broader society. In many situations, ensuring that the human can understand the basis of algorithmic decisions is a necessity, not a luxury.
A way forward
Reconciling these seemingly conflicting requirements is possible. But it requires serious commitment from business and data/ analytics leaders – not (just) because regulators demand it, but because it is good for their customers and their business, and the only way to start capturing the full value from AI/ML.
1. ‘Heart’, not just ‘Head’
It is relatively easy to get people excited about experimenting with AI/ML. But when it comes to actually trusting the model to make decisions for us, we humans are likely to put up our defences. Convincing a loan approver, insurance under-writer, medical doctor or front-line sales-person to trust an AI/ML model – over their own knowledge or intuition – is as much about the ‘heart’ as the ‘head’. Helping them understand, on their own terms, how the alternative is at least as good as their current way of doing things, is crucial.
2. A Broad Church
Even in industries/ organisations that recognise the importance of governing AI/ML, there is a tendency to define it narrowly. For example, in Financial Services, one might argue that “an ML model is just another model” and expect existing Model Risk teams to deal with any incremental risks from AI/ML.
There are two issues with this approach:
First, AI/ML models tend to require a greater focus on model quality (e.g., with respect to stability, overfitting and unjust bias) than their traditional alternatives. The pace at which such models are expected to be introduced and re-calibrated is also much higher, stretching traditional model risk management approaches.
Second, poorly designed AI/ML models create second order risks. While not unique to AI/ML, these risks become accentuated due to model complexity, greater dependence on (high-volume, often non-traditional) data and ubiquitous adoption. One example is poor customer experience (e.g., badly communicated decisions) and unfair treatment (e.g., unfair denial of service, discrimination, misselling, inappropriate investment recommendations). Another is around the stability, integrity and competitiveness of financial markets (e.g., unintended collusion with other market players). Obligations under data privacy, sovereignty and security requirements could also become more challenging.
The only way to respond holistically is to bring together a broad coalition – of data managers and scientists, technologists, specialists from risk, compliance, operations and cyber-security, and business leaders.
3. Automate, Automate, Automate
A key driver for the adoption and effectiveness of AI/ ML is scalability. The techniques used to manage traditional models are often inadequate in the face of more data-hungry, widely used and rapidly refreshed AI/ML models. Whether it is during the development and testing phase, formal assessment/ validation or ongoing post-production monitoring, it is impossible to govern AI/ML at scale using manual processes alone.
o, somewhat counter-intuitively, we need more automation if we are to build and sustain trust in AI/ML. As humans are accountable for the outcomes of AI/ ML models, we can only be ‘in charge’ if we have the tools to provide us reliable intelligence on them – before and after they go into production. As the recent experience with model performance during COVID-19 suggests, maintaining trust in AI/ML models is an ongoing task.
***
I have heard people say “AI is too important to be left to the experts”. Perhaps. But I am yet to come across an AI/ML practitioner who is not keenly aware of the importance of making their models reliable and safe. What I have noticed is that they often lack suitable tools – to support them in analysing and monitoring models, and to enable conversations to build trust with stakeholders. If AI is to be adopted at scale, that must change.
Shameek Kundu is Chief Strategy Officer and Head of Financial Services at TruEra Inc. TruEra helps enterprises analyse, improve and monitor quality of machine
Have you evaluated the tech areas on your AI requirements? Get access to AI insights and key industry trends from our AI research.
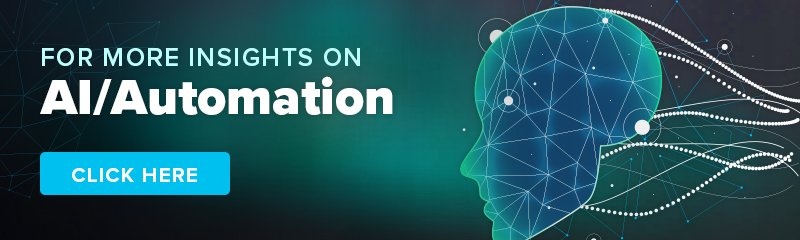