What if your bank could predict your financial needs before you even realised them? Imagine a financial institution that understands your behaviours, anticipates your needs, and engages with you meaningfully in real time. That’s the power of modern marketing. We’re not talking about one-size-fits-all campaigns; we’re talking about creating personalised experiences that resonate on an individual level. Financial institutions are increasingly recognising that when marketing is done right, it becomes a powerful driver of customer loyalty and growth.
Take Netflix, for example – the frontrunner in personalisation. They don’t just serve content; they study audience preferences to deliver exactly what each viewer wants. Now, picture banks doing the same. Banks can apply the same principles by building a complete view of each customer based on transactions, preferences, and lifestyle signals. This allows them to deliver personalised content, products, and nudges that help customers make smarter financial decisions – and feel more understood in the process.
The Opportunity: Marketing Beyond the Surface
The real opportunity for banks today is to reimagine marketing – not as a support function, but as a strategic growth engine. This means pulling marketing out of the back office and placing it at the heart of business decision-making. When marketing is deeply embedded in strategy, aligned with business goals, and backed by executive support, it becomes a force multiplier. Cross-functional teams start working in sync, campaigns become smarter and more personalised, and outcomes become measurable and meaningful. The impact? Rapid customer growth, stronger retention, and sharper product uptake. This reimagination hinges on two foundational shifts:
- Culture Shift. Agile, cross-functional teams that focus on delivering specific customer outcomes rather than managing marketing channels in silos. These teams are empowered to act quickly, experiment, and refine based on feedback.
- Tech-Enabled Precision. A well-governed MarTech stack that enables real-time personalisation by effectively using data, decisioning models, and delivery mechanisms. This means moving from siloed systems to unified, intelligent platforms.
When these shifts align, marketing transforms from a function into a growth engine – fuelling innovation and building deeper customer relationships.
Embracing Modern Marketing Principles
Modern marketing starts with a few foundational shifts.
1. Embedding Marketing into Business Strategy. Marketing needs to be part of business strategy from the start – not bolted on at the end. When marketers have a seat at the table during strategy planning, they can shape priorities, align efforts, and measure impact more effectively.
This requires:
- Early involvement in strategic decision-making
- Marketing scorecards derived from business KPIs
- A unified voice across product, marketing, and operations
Also, Internal teams must be in sync with customer promises made externally. This means training, communication, and shared understanding across departments to ensure every touchpoint reinforces a consistent message. Whether it’s a branch interaction, a call centre response, or a chatbot exchange – each moment becomes a reflection of the brand’s commitment.
By embedding marketing within the business from the outset and aligning it with internal delivery mechanisms, organisations are better positioned to deliver on their promises and build lasting trust.
2. Turning Data into Action: Using MarTech for Smarter Decisions and Delivery. Modern marketing runs on data – but it only matters if it drives action. Banks need a systematic approach to turning raw data into relevant, timely customer experiences.
- Organise. Cleanse and consolidate data to form a single view of each customer.
- Decide. Apply AI/ML models to extract insights, make predictions, and personalise offerings.
- Deliver. Communicate through the most effective channels at the right moment.
But a smart stack needs structure. MarTech governance is essential to ensure tech investments are strategic – not duplicative. A cross-functional steering committee, with voices from marketing, IT, compliance, and business leadership, should guide decisions. This keeps tools aligned with business goals and ensures interoperability.
Equally important is fostering a culture of experimentation. A/B testing and continuous learning should be baked into campaign design – not as add-ons, but as core capabilities. This sharpens performance and fuels innovation by quickly scaling what works. Each test becomes a feedback loop, feeding a smarter, more agile marketing engine.
Embedding these practices into the data-decisioning-delivery loop helps banks move from scattered insights to coordinated, high-impact engagement.
3. Outcome-Focused Teams and Operating Models. Rather than being structured around traditional channels, high-performing marketing teams operate around customer journeys and outcomes. Agile squads can be created for:
- Seamless onboarding
- Product activation and adoption
- Retention and cross-sell strategies
These squads have to be multidisciplinary – combining marketing, data science, engineering, and product. They need to be empowered to experiment, move fast, and own KPIs tied to customer impact.
Outcome-driven teams focus on delivering measurable value – not just activity. For instance, a squad working on onboarding might track completion rates and activation time, while a cross-sell squad may target conversion on tailored offers. The shift to outcome-oriented delivery ensures that efforts are tied to clear business metrics, with faster feedback loops and accountability.
By storing, organising, and analysing customer data, banks equip these teams to predict needs more accurately. Insights fuel smarter decisions – enabling real-time, context-aware offers. Paired with agile delivery, this data-led approach makes every interaction timely, relevant, and impactful.
This approach ensures agility, accountability, and focus. By reducing reliance on rigid departmental structures, teams can iterate quickly and deliver greater value across the customer lifecycle.
The Modern Marketing Imperative
As customer needs and expectations evolve, marketing must adapt to modern standards, with a tighter alignment between business and marketing to deliver delightful customer experiences and impactful outcomes. These are the new imperatives for growth.
These principles are not just theoretical – they’re actionable steps that enable banks to anticipate needs, deliver hyper-personalised experiences, and build lasting relationships. By embracing this shift, banks can move from reactive to proactive, empowering customers to make smarter financial decisions while driving loyalty and growth. Ultimately, the future of banking lies in a personalised, integrated, and data-driven experience for every customer.
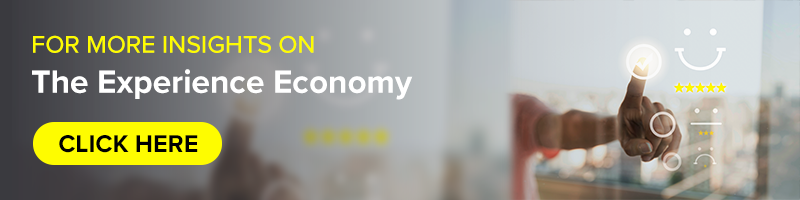
The Manufacturing sector, traditionally defined by stable processes and infrastructure, is now facing a pivotal shift. Rapid technological advancements and shifting global market dynamics have rendered incremental improvements inadequate for long-term competitiveness and growth. To thrive, manufacturers must fundamentally reimagine their entire value chain.
By embracing intelligent systems, enhancing agility, and proactively shaping future-ready operations, organisations can navigate today’s industrial complexities and position themselves for sustained success.
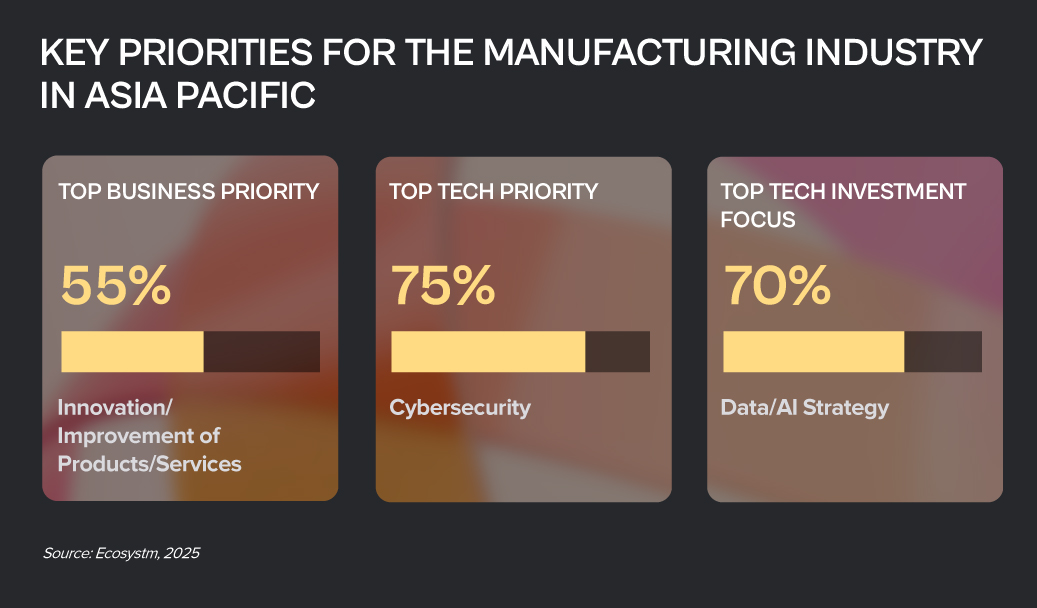
Here are recent examples of Manufacturing transformation in the Asia Pacific.
Click here to download “Future Forward: Reimagining Manufacturing” as a PDF.
Intelligent Automation & Efficiency
Komatsu Australia, a global industrial equipment manufacturer, tackled growing inefficiencies in its small parts department, where teams manually processed hundreds of PDF invoices daily from more than 250 suppliers.
To streamline this, the company deployed intelligent automation – AI now extracts and validates data from invoices against purchase orders and inputs it directly into the legacy mainframe.
The impact has been sharp: over 300 hours saved annually for one supplier, 1,100 invoices processed in three weeks, and a dramatic drop in manual errors. Employees have shifted to higher-value tasks, and a citizen developer program is enabling staff to build custom automation tools. With a scalable framework in place, Komatsu has not only transformed invoice processing but also set the stage for broader automation across the enterprise.
Data-Driven Insights & Agility
Berger Paints India Ltd., a leader in paints and coatings, needed to scale fast amid rising database loads and complex on-prem systems.
In response, Berger Paints migrated its mission-critical databases and core business applications – covering finance, manufacturing, sales, and asset management – to a high-performance cloud platform.
This shift boosted operational efficiency by 25%, doubled reporting and system response times, and enhanced scalability and disaster recovery with geographically distributed cloud regions. The move simplified access to data, driving faster, insight-driven decision-making. With streamlined infrastructure management and optimised costs, Berger Paints is now poised to leverage advanced technologies like AI/ML, setting the stage for continued innovation and growth.
Connected Operations & Customer Centricity
JSW Steel, one of India’s leading steel producers, set out to shift from a plant-centric model to a customer-first approach. The challenge: integrating complex systems like ERP, CRM, and manufacturing to streamline operations and improve order fulfillment.
With a robust integration platform, JSW Steel connected over 32 systems using 120+ APIs – automating processes and enabling real-time data flow across orders, inventory, pricing, and production.
The results speak for themselves: faster order fulfillment, reduced cost-to-serve, and real-time visibility that optimises scheduling. Scalable, composable APIs now support growth, while a 99.7% success rate across 7.2 million API calls ensures reliability. JSW Steel has transformed how it operates – running faster, serving smarter, and delivering better customer experiences across the entire order-to-cash journey.
Modernising Core Systems & Foundational Transformation
Fujitsu General, a global leader in air conditioning systems, was constrained by a 30-year-old COBOL-based mainframe and fragmented processes. The legacy system posed a Y2K-like risk and limited operational agility.
The company implemented a modern, unified ERP platform to eliminate risk, streamline operations, and boost agility.
By integrating functions across sales, production, procurement, accounting, and HR and addressing unique business needs with low-code development, the company created a clean, adaptable core system. Robust integration connected disparate data sources, while a central repository eliminated silos. The transformation delivered seamless end-to-end operations, standardised workflows, improved agility, and real-time insights – setting Fujitsu General up for continued innovation and long-term resilience.
Powering Growth with a Modern Network
As a critical supplier to India’s infrastructure boom, Hindalco needed to modernise its network across 55 sites – improving app performance, enabling real-time insights, and building a future-ready, sustainable foundation.
Hindalco replaced its ageing hub-and-spoke model with a modern mesh architecture using SD-WAN.
The new architecture prioritised key app traffic, simplified cloud access, and enabled segmentation. Centralised orchestration and SSE integration brought automation and robust security. The impact: 30% lower costs, 50% faster apps, real-time visibility, rapid deployment, and smarter bandwidth. Hindalco now runs on a lean, secure digital backbone – built for agility, performance, and scale.
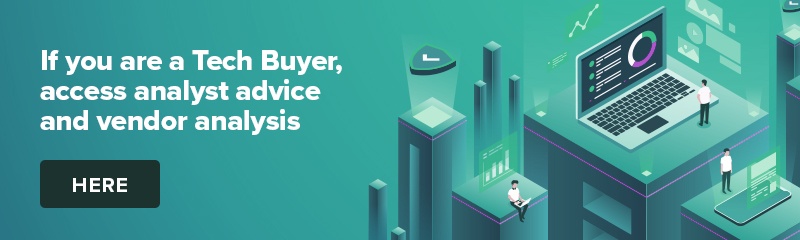
India is undergoing a remarkable transformation across various industries, driven by rapid technological advancements, evolving consumer preferences, and a dynamic economic landscape. From the integration of new-age technologies like GenAI to the adoption of sustainable practices, industries in India are redefining their operations and strategies to stay competitive and relevant.
Here are some organisations that are leading the way.
Download ‘From Tradition to Innovation: Industry Transformation in India’ as a PDF
Redefining Customer Experience in the Financial Sector
Financial inclusion. India’s largest bank, the State Bank of India, is leading financial inclusion with its YONO app, to enhance accessibility. Initial offerings include five core banking services: cash withdrawals, cash deposits, fund transfers, balance inquiries, and mini statements, with plans to include account opening and social security scheme enrollments.
Customer Experience. ICICI Bank leverages RPA to streamline repetitive tasks, enhancing customer service with its virtual assistant, iPal, for handling queries and transactions. HDFC Bank customer preference insights to offer tailored financial solutions, while Axis Bank embraces a cloud-first strategy to digitise its platform and improve customer interfaces.
Indian banks are also collaborating with fintechs to harness new technologies for better customer experiences. YES Bank has partnered with Paisabazaar to simplify loan applications, and Canara HSBC Life Insurance has teamed up with Artivatic.AI to enhance its insurance processes via an AI-driven platform.
Improving Healthcare Access
Indian healthcare organisations are harnessing technology to enhance efficiency, improve patient experiences, and enable remote care.
Apollo Hospitals has launched an automated patient monitoring system that alerts experts to health deteriorations, enabling timely interventions through remote monitoring. Manipal Hospitals’ video consultation app reduces emergency department pressure by providing medical advice, lab report access, bill payments, appointment bookings, and home healthcare requests, as well as home medication delivery and Fitbit monitoring. Omni Hospitals has also implemented AI-based telemedicine for enhanced patient engagement and remote monitoring.
The government is also driving the improvement of healthcare access. eSanjeevani is the world’s largest government-owned telemedicine system, with the capacity to handle up to a million patients a day.
Driving Retail Agility & Consumer Engagement
India’s Retail sector, the fourth largest globally, contributes over 10% of the nation’s GDP. To stay competitive and meet evolving consumer demands, Indian retailers are rapidly adopting digital technologies, from eCommerce platforms to AI.
Omnichannel Strategies. Reliance Retail integrates physical stores with digital platforms like JioMart to boost sales and customer engagement. Tata CLiQ’s “phygital” approach merges online and offline shopping for greater convenience while Shoppers Stop uses RFID and data analytics for improved in-store experiences, online shopping, and targeted marketing.
Retail AI. Flipkart’s AI-powered shopping assistant, Flippi uses ML for conversational product discovery and intuitive guidance. BigBasket employs IoT-led AI to optimise supply chain and improve product quality.
Reshaping the Automotive Landscape
Tech innovation, from AI/ML to connected vehicle technologies, is revolutionising the Automotive sector. This shift towards software-defined vehicles and predictive supply chain management underscores the industry’s commitment to efficiency, transparency, safety, and environmental sustainability.
Maruti Suzuki’s multi-pronged approach includes collaborating with over 60 startups through its MAIL program and engaging Accenture to drive tech change. Maruti has digitised 24 out of 26 customer touchpoints, tracking every interaction to enhance customer service. In the Auto OEM space, they are shifting to software-defined vehicles and operating models.
Tata Motors is leveraging cloud, AI/ML, and IoT to enhancing efficiency, improving safety, and driving sustainability across its operations. Key initiatives include connected vehicles, automated driving, dealer management, cybersecurity, electric powertrains, sustainability, and supply chain optimisation.
Streamlining India’s Logistics Sector
India’s logistics industry is on the cusp of a digital revolution as it embraces cutting-edge technologies to streamline processes and reduce environmental impact.
Automation and Predictive Analytics. Automation is transforming warehousing operations in India, with DHL India automating sortation centres to handle 6,000 shipments per hour. Predictive analytics is reshaping logistics decision-making, with Delhivery optimising delivery routes to ensure timely service.
Sustainable Practices. The logistics sector contributes one-third of global carbon emissions. To combat this, Amazon India will convert its delivery fleet to 100% EVs by 2030 to reduce emissions and fuel costs. Blue Energy Motors is also producing 10,000 heavy-duty LNG trucks annually for zero-emission logistics.
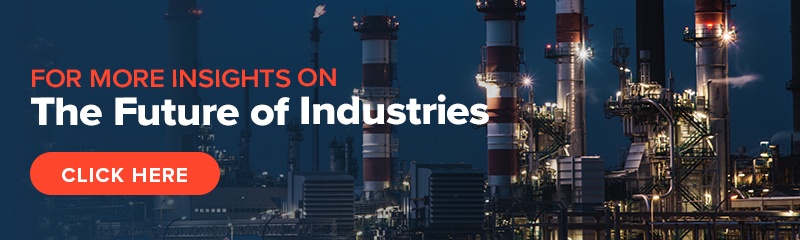
At a recently held Ecosystm roundtable, in partnership with Qlik and 121Connects, Ecosystm Principal Advisor Manoj Chugh, moderated a conversation where Indian tech and data leaders discussed building trust in data strategies. They explored ways to automate data pipelines and improve governance to drive better decisions and business outcomes. Here are the key takeaways from the session.

Data isn’t just a byproduct anymore; it’s the lifeblood of modern businesses, fuelling informed decisions and strategic growth. But with vast amounts of data, the challenge isn’t just managing it; it’s building trust. AI, once a beacon of hope, is now at risk without a reliable data foundation. Ecosystm research reveals that a staggering 66% of Indian tech leaders doubt their organisation’s data quality, and the problem of data silos is exacerbating this trust crisis.
At the Leaders Roundtable in Mumbai, I had the opportunity to moderate a discussion among data and digital leaders on the critical components of building trust in data and leveraging it to drive business value. The consensus was that building trust requires a comprehensive strategy that addresses the complexities of data management and positions the organisation for future success. Here are the key strategies that are essential for achieving these goals.
1. Adopting a Unified Data Approach
Organisations are facing a growing wave of complex workloads and business initiatives. To manage this expansion, IT teams are turning to multi-cloud, SaaS, and hybrid environments. However, this diverse landscape introduces new challenges, such as data silos, security vulnerabilities, and difficulties in ensuring interoperability between systems.

A unified data strategy is crucial to overcome these challenges. By ensuring platform consistency, robust security, and seamless data integration, organisations can simplify data management, enhance security, and align with business goals – driving informed decisions, innovation, and long-term success.
Real-time data integration is essential for timely data availability, enabling organisations to make data-driven decisions quickly and effectively. By integrating data from various sources in real-time, businesses can gain valuable insights into their operations, identify trends, and respond to changing market conditions.
Organisations that are able to integrate their IT and operational technology (OT) systems find their data accuracy increasing. By combining IT’s digital data management expertise with OT’s real-time operational insights, organisations can ensure more accurate, timely, and actionable data. This integration enables continuous monitoring and analysis of operational data, leading to faster identification of errors, more precise decision-making, and optimised processes.
2. Enhancing Data Quality with Automation and Collaboration
As the volume and complexity of data continue to grow, ensuring high data quality is essential for organisations to make accurate decisions and to drive trust in data-driven solutions. Automated data quality tools are useful for cleansing and standardising data to eliminate errors and inconsistencies.
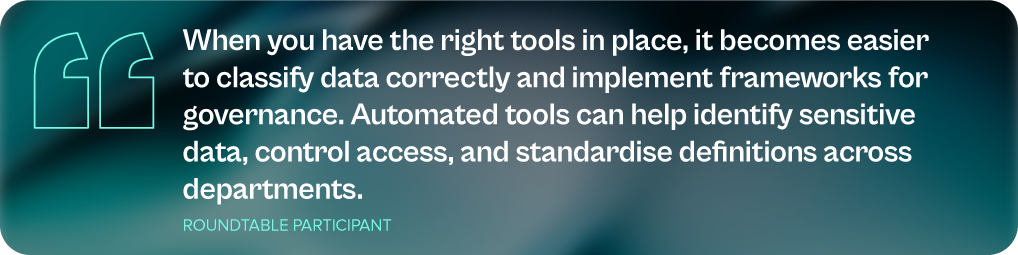
As mentioned earlier, integrating IT and OT systems can help organisations improve operational efficiency and resilience. By leveraging data-driven insights, businesses can identify bottlenecks, optimise workflows, and proactively address potential issues before they escalate. This can lead to cost savings, increased productivity, and improved customer satisfaction.
However, while automation technologies can help, organisations must also invest in training employees in data management, data visualisation, and data governance.
3. Modernising Data Infrastructure for Agility and Innovation
In today’s fast-paced business landscape, agility is paramount. Modernising data infrastructure is essential to remain competitive – the right digital infrastructure focuses on optimising costs, boosting capacity and agility, and maximising data leverage, all while safeguarding the organisation from cyber threats. This involves migrating data lakes and warehouses to cloud platforms and adopting advanced analytics tools. However, modernisation efforts must be aligned with specific business goals, such as enhancing customer experiences, optimising operations, or driving innovation. A well-modernised data environment not only improves agility but also lays the foundation for future innovations.

Technology leaders must assess whether their data architecture supports the organisation’s evolving data requirements, considering factors such as data flows, necessary management systems, processing operations, and AI applications. The ideal data architecture should be tailored to the organisation’s specific needs, considering current and future data demands, available skills, costs, and scalability.
4. Strengthening Data Governance with a Structured Approach
Data governance is crucial for establishing trust in data, and providing a framework to manage its quality, integrity, and security throughout its lifecycle. By setting clear policies and processes, organisations can build confidence in their data, support informed decision-making, and foster stakeholder trust.
A key component of data governance is data lineage – the ability to trace the history and transformation of data from its source to its final use. Understanding this journey helps organisations verify data accuracy and integrity, ensure compliance with regulatory requirements and internal policies, improve data quality by proactively addressing issues, and enhance decision-making through context and transparency.
A tiered data governance structure, with strategic oversight at the executive level and operational tasks managed by dedicated data governance councils, ensures that data governance aligns with broader organisational goals and is implemented effectively.
Are You Ready for the Future of AI?
The ultimate goal of your data management and discovery mechanisms is to ensure that you are advancing at pace with the industry. The analytics landscape is undergoing a profound transformation, promising to revolutionise how organisations interact with data. A key innovation, the data fabric, is enabling organisations to analyse unstructured data, where the true value often lies, resulting in cleaner and more reliable data models.
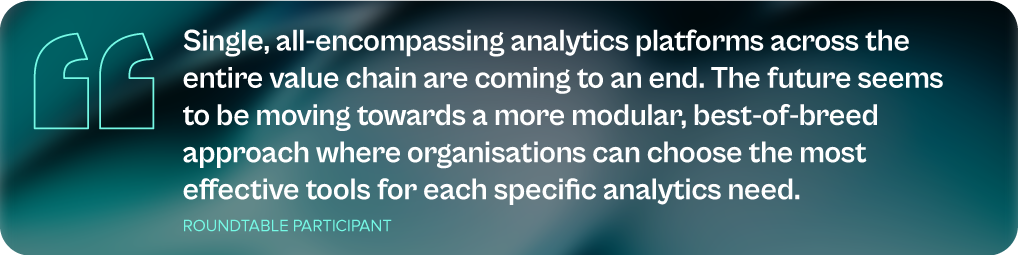
GenAI has emerged as another game-changer, empowering employees across the organisation to become citizen data scientists. This democratisation of data analytics allows for a broader range of insights and fosters a more data-driven culture. Organisations can leverage GenAI to automate tasks, generate new ideas, and uncover hidden patterns in their data.
The shift from traditional dashboards to real-time conversational tools is also reshaping how data insights are delivered and acted upon. These tools enable users to ask questions in natural language, receiving immediate and relevant answers based on the underlying data. This conversational approach makes data more accessible and actionable, empowering employees to make data-driven decisions at all levels of the organisation.
To fully capitalise on these advancements, organisations need to reassess their AI/ML strategies. By ensuring that their tech initiatives align with their broader business objectives and deliver tangible returns on investment, organisations can unlock the full potential of data-driven insights and gain a competitive edge. It is equally important to build trust in AI initiatives, through a strong data foundation. This involves ensuring data quality, accuracy, and consistency, as well as implementing robust data governance practices. A solid data foundation provides the necessary groundwork for AI and GenAI models to deliver reliable and valuable insights.
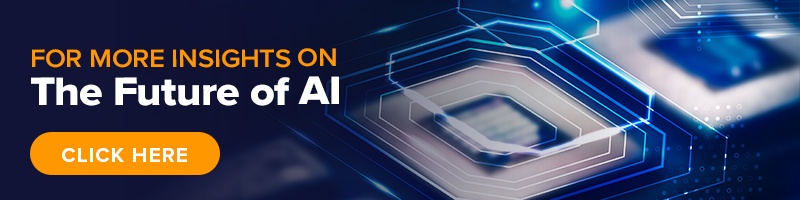
As AI adoption continues to surge, the tech infrastructure market is undergoing a significant transformation. Traditional IT infrastructure providers are facing increasing pressure to innovate and adapt to the evolving demands of AI-powered applications. This shift is driving the development of new technologies and solutions that can support the intensive computational requirements and data-intensive nature of AI workloads.
At Lenovo’s recently held Asia Pacific summit in Shanghai they detailed their ‘AI for All’ strategy as they prepare for the next computing era. Building on their history as a major force in the hardware market, new AI-ready offerings will be prominent in their enhanced portfolio.
At the same time, Lenovo is adding software and services, both homegrown and with partners, to leverage their already well-established relationships with client IT teams. Sustainability is also a crucial message as it seeks to address the need for power efficiency and zero waste lifecycle management in their products.
Ecosystm Advisor Darian Bird comment on Lenovo’s recent announcements and messaging.
Click here to download Lenovo’s Innovation Roadmap: Takeaways from the APAC Analyst Summit as a PDF
1. Lenovo’s AI Strategy
Lenovo’s AI strategy focuses on launching AI PCs that leverage their computing legacy.
As the adoption of GenAI increases, there’s a growing need for edge processing to enhance privacy and performance. Lenovo, along with Microsoft, is introducing AI PCs with specialised components like CPUs, GPUs, and AI accelerators (NPUs) optimised for AI workloads.
Energy efficiency is vital for AI applications, opening doors for mobile-chip makers like Qualcomm. Lenovo’s latest ThinkPads, featuring Qualcomm’s Snapdragon X Elite processors, support Microsoft’s Copilot+ features while maximising battery life during AI tasks.
Lenovo is also investing in small language models (SLMs) that run directly on laptops, offering GenAI capabilities with lower resource demands. This allows users to interact with PCs using natural language for tasks like file searches, tech support, and personal management.
2. Lenovo’s Computer Vision Solutions
Lenovo stands out as one of the few computing hardware vendors that manufactures its own systems.
Leveraging precision engineering, Lenovo has developed solutions to automate production lines. By embedding computer vision in processes like quality inspection, equipment monitoring, and safety supervision, Lenovo customises ML algorithms using customer-specific data. Clients like McLaren Automotive use this technology to detect flaws beyond human capability, enhancing product quality and speeding up production.
Lenovo extends their computer vision expertise to retail, partnering with Sensormatic and Everseen to digitise branch operations. By analysing camera feeds, Lenovo’s solutions optimise merchandising, staffing, and design, while their checkout monitoring system detects theft and scanning errors in real-time. Australian customers have seen significant reductions in retail shrinkage after implementation.
3. AI in Action: Autonomous Robots
Like other hardware companies, Lenovo is experimenting with new devices to futureproof their portfolio.
Earlier this year, Lenovo unveiled the Daystar Bot GS, a six-legged robotic dog and an upgrade from their previous wheeled model. Resembling Boston Dynamics’ Spot but with added legs inspired by insects for enhanced stability, the bot is designed for challenging environments. Lenovo is positioning it as an automated monitoring assistant for equipment inspection and surveillance, reducing the need for additional staff. Power stations in China are already using the robot to read meters, detect temperature anomalies, and identify defective equipment.
Although it is likely to remain a niche product in the short term, the robot is an avenue for Lenovo to showcase their AI wares on a physical device, incorporating computer vision and self-guided movement.
Considerations for Lenovo’s Future Growth
Lenovo outlined an AI vision leveraging their expertise in end user computing, manufacturing, and retail. While the strategy aligns with Lenovo’s background, they should consider the following:
Hybrid AI. Initially, AI on PCs will address performance and privacy issues, but hybrid AI – integrating data across devices, clouds, and APIs – will eventually dominate.
Data Transparency & Control. The balance between convenience and privacy in AI is still unclear. Evolving transparency and control will be crucial as users adapt to new AI tools.
AI Ecosystem. AI’s value lies in data, applications, and integration, not just hardware. Hardware vendors must form deeper partnerships in these areas, as Lenovo’s focus on industry-specific solutions demonstrates.
Enhanced Experience. AI enhances operational efficiency and customer experience. Offloading level one support to AI not only cuts costs but also resolves issues faster than live agents.
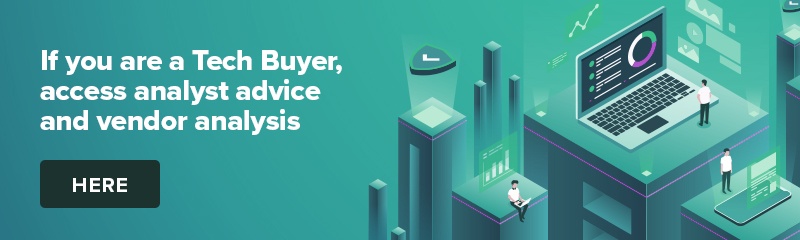
Data analysts play a vital role in today’s data-driven world, providing crucial insights that benefit decision-making processes. For those with a knack for numbers and a passion for uncovering patterns, a career as a data analyst can be both fulfilling and lucrative – it can also be a stepping stone towards other careers in data. While a data analyst focuses on data preparation and visualisation, an AI engineer specialises in creating AI solutions, a machine learning (ML) engineer concentrates on implementing ML models, and a data scientist combines elements of data analysis and ML to derive insights and predictions from data.
Tools, Skills, and Techniques of a Data Analyst
Excel Mastery. Unlocks a powerful toolbox for data manipulation and analysis. Essential skills include using a vast array of functions for calculations and data transformation. Pivot tables become your secret weapon for summarising and analysing large datasets, while charts and graphs bring your findings to life with visual clarity. Data validation ensures accuracy, and the Analysis ToolPak and Solver provide advanced functionalities for statistical analysis and complex problem-solving. Mastering Excel empowers you to transform raw data into actionable insights.
Advanced SQL. While basic skills handle simple queries, advanced users can go deeper with sorting, aggregation, and the art of JOINs to combine data from multiple tables. Common Table Expressions (CTEs) and subqueries become your allies for crafting complex queries, while aggregate functions summarise vast amounts of data. Window functions add another layer of power, allowing calculations within query results. Mastering Advanced SQL empowers you to extract hidden insights and manage data with unparalleled precision.
Data Visualisation. Crafts impactful data stories. These tools empower you to connect to various data sources, transform raw information into a usable format, and design interactive dashboards and reports. Filters and drilldowns allow users to explore your data from different angles, while calculated fields unlock deeper analysis. Parameters add a final touch of flexibility, letting viewers customise the report to their specific needs. With tools Tableau and Power BI, complex data becomes clear and engaging.
Essential Python. This powerful language excels at data analysis and automation. Libraries like NumPy and Pandas become your foundation for data manipulation and wrangling. Scikit-learn empowers you to build ML models, while SciPy and StatsModels provide a toolkit for in-depth statistical analysis. Python’s ability to interact with APIs and web scrape data expands its reach, and its automation capabilities streamline repetitive tasks. With Essential Python, you have the power to solve complex problems.
Automating the Journey. Data analysts can be masters of efficiency, and their skills translate beautifully into AI. Scripting languages like Ansible and Terraform automate repetitive tasks. Imagine streamlining the process of training and deploying AI models – a skill that directly benefits the AI development pipeline. This proficiency in automation showcases the valuable foundation data analysts provide for building and maintaining AI systems.
Developing ML Expertise. Transitioning from data analysis to AI involves building on your existing skills to develop ML expertise. As a data analyst, you may start with basic predictive models. This knowledge is expanded in AI to include deep learning and advanced ML algorithms. Also, skills in statistical analysis and visualisation help in evaluating the performance of AI models.
Growing Your AI Skills
Becoming an AI engineer requires building on a data analysis foundation to focus on advanced skills such as:
- Deep Learning. Learning frameworks like TensorFlow and PyTorch to build and train neural networks.
- Natural Language Processing (NLP). Techniques for processing and analysing large amounts of natural language data.
- AI Ethics and Fairness. Understanding the ethical implications of AI and ensuring models are fair and unbiased.
- Big Data Technologies. Using tools like Hadoop and Spark for handling large-scale data is essential for AI applications.
The Evolution of a Data Analyst: Career Opportunities
Data analysis is a springboard to AI engineering. Businesses crave talent that bridges the data-AI gap. Your data analyst skills provide the foundation (understanding data sources and transformations) to excel in AI. As you master ML, you can progress to roles like:
- AI Engineer. Works on integrating AI solutions into products and services. They work with AI frameworks like TensorFlow and PyTorch, ensuring that AI models are incorporated into products and services in a fair and unbiased manner.
- ML Engineer. Focuses on designing and implementing ML models. They focus on preprocessing data, evaluating model performance, and collaborating with data scientists and engineers to bring models into production. They need strong programming skills and experience with big data tools and ML algorithms.
- Data Scientist. Bridges the gap between data analysis and AI, often involved in both data preparation and model development. They perform exploratory data analysis, develop predictive models, and collaborate with cross-functional teams to solve complex business problems. Their role requires a comprehensive understanding of both data analysis and ML, as well as strong programming and data visualisation skills.
Conclusion
Hone your data expertise and unlock a future in AI! Mastering in-demand skills like Excel, SQL, Python, and data visualisation tools will equip you to excel as a data analyst. Your data wrangling skills will be invaluable as you explore ML and advanced algorithms. Also, your existing BI knowledge translates seamlessly into building and evaluating AI models. Remember, the data landscape is constantly evolving, so continue to learn to stay at the forefront of this dynamic field. By combining your data skills with a passion for AI, you’ll be well-positioned to tackle complex challenges and shape the future of AI.
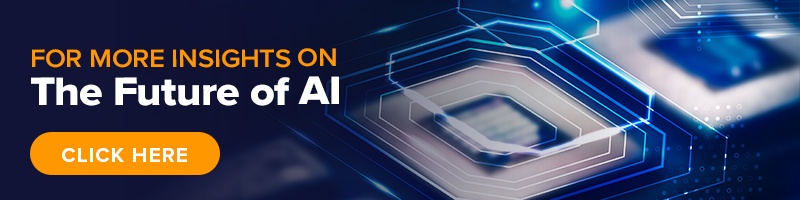
The data architecture outlines how data is managed in an organisation and is crucial for defining the data flow, data management systems required, the data processing operations, and AI applications. Data architects and engineers define data models and structures based on these requirements, supporting initiatives like data science. Before we delve into the right data architecture for your AI journey, let’s talk about the data management options. Technology leaders have the challenge of deciding on a data management system that takes into consideration factors such as current and future data needs, available skills, costs, and scalability. As data strategies become vital to business success, selecting the right data management system is crucial for enabling data-driven decisions and innovation.
Data Warehouse
A Data Warehouse is a centralised repository that stores vast amounts of data from diverse sources within an organisation. Its main function is to support reporting and data analysis, aiding businesses in making informed decisions. This concept encompasses both data storage and the consolidation and management of data from various sources to offer valuable business insights. Data Warehousing evolves alongside technological advancements, with trends like cloud-based solutions, real-time capabilities, and the integration of AI and machine learning for predictive analytics shaping its future.
Core Characteristics
- Integrated. It integrates data from multiple sources, ensuring consistent definitions and formats. This often includes data cleansing and transformation for analysis suitability.
- Subject-Oriented. Unlike operational databases, which prioritise transaction processing, it is structured around key business subjects like customers, products, and sales. This organisation facilitates complex queries and analysis.
- Non-Volatile. Data in a Data Warehouse is stable; once entered, it is not deleted. Historical data is retained for analysis, allowing for trend identification over time.
- Time-Variant. It retains historical data for trend analysis across various time periods. Each entry is time-stamped, enabling change tracking and trend analysis.
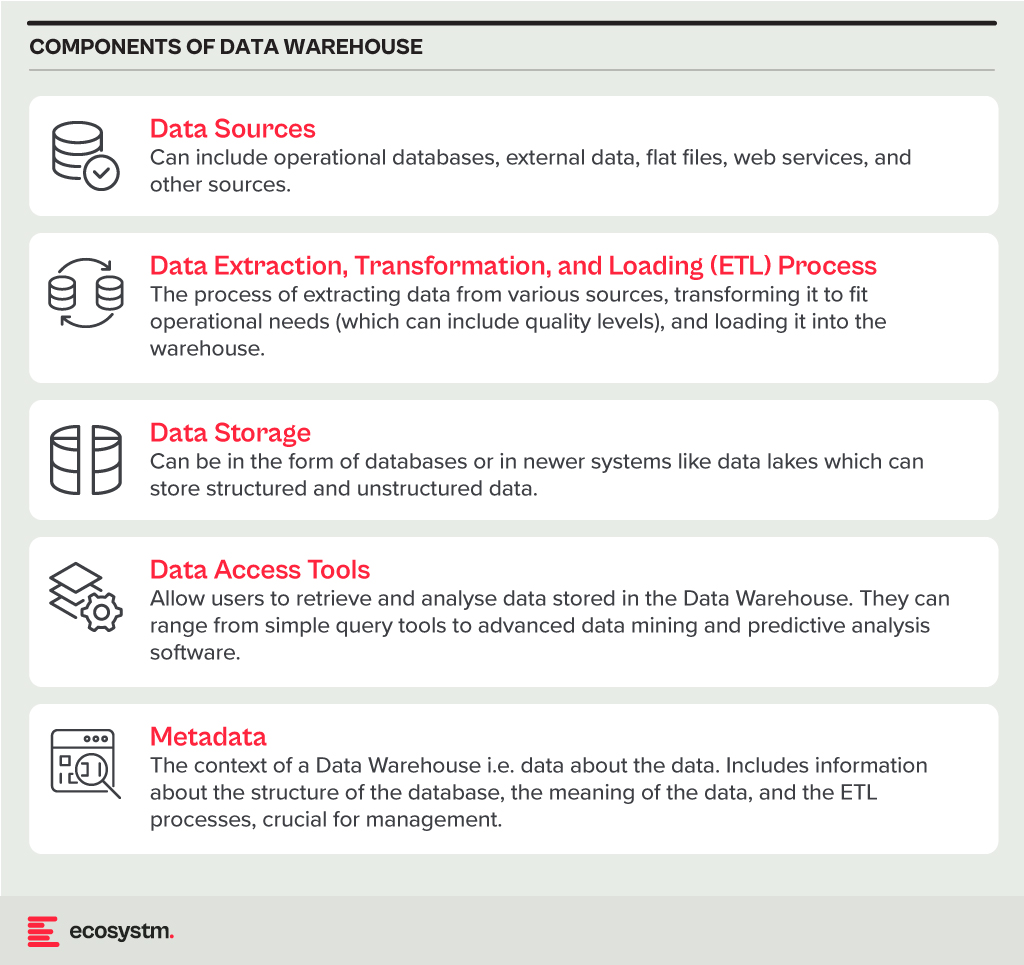
Benefits
- Better Decision Making. Data Warehouses consolidate data from multiple sources, offering a comprehensive business view for improved decision-making.
- Enhanced Data Quality. The ETL process ensures clean and consistent data entry, crucial for accurate analysis.
- Historical Analysis. Storing historical data enables trend analysis over time, informing future strategies.
- Improved Efficiency. Data Warehouses enable swift access and analysis of relevant data, enhancing efficiency and productivity.
Challenges
- Complexity. Designing and implementing a Data Warehouse can be complex and time-consuming.
- Cost. The cost of hardware, software, and specialised personnel can be significant.
- Data Security. Storing large amounts of sensitive data in one place poses security risks, requiring robust security measures.
Data Lake
A Data Lake is a centralised repository for storing, processing, and securing large volumes of structured and unstructured data. Unlike traditional Data Warehouses, which are structured and optimised for analytics with predefined schemas, Data Lakes retain raw data in its native format. This flexibility in data usage and analysis makes them crucial in modern data architecture, particularly in the age of big data and cloud.
Core Characteristics
- Schema-on-Read Approach. This means the data structure is not defined until the data is read for analysis. This offers more flexible data storage compared to the schema-on-write approach of Data Warehouses.
- Support for Multiple Data Types. Data Lakes accommodate diverse data types, including structured (like databases), semi-structured (like JSON, XML files), unstructured (like text and multimedia files), and binary data.
- Scalability. Designed to handle vast amounts of data, Data Lakes can easily scale up or down based on storage needs and computational demands, making them ideal for big data applications.
- Versatility. Data Lakes support various data operations, including batch processing, real-time analytics, machine learning, and data visualisation, providing a versatile platform for data science and analytics.
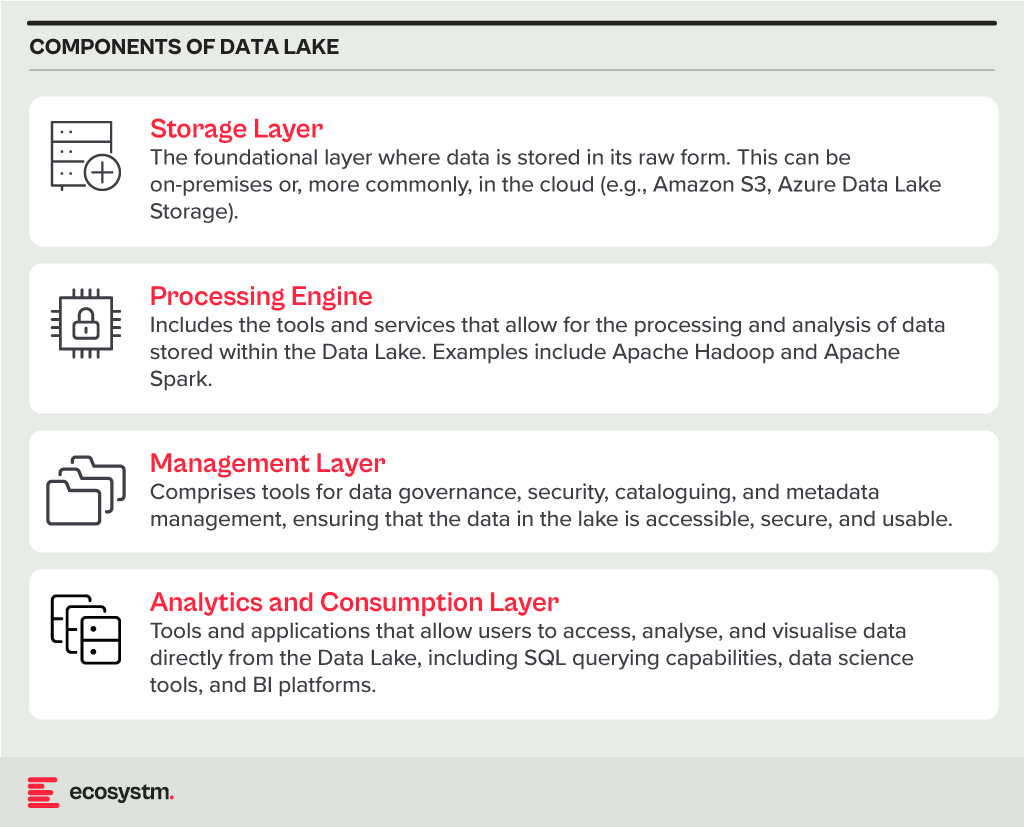
Benefits
- Flexibility. Data Lakes offer diverse storage formats and a schema-on-read approach for flexible analysis.
- Cost-Effectiveness. Cloud-hosted Data Lakes are cost-effective with scalable storage solutions.
- Advanced Analytics Capabilities. The raw, granular data in Data Lakes is ideal for advanced analytics, machine learning, and AI applications, providing deeper insights than traditional data warehouses.
Challenges
- Complexity and Management. Without proper management, a Data Lake can quickly become a “Data Swamp” where data is disorganised and unusable.
- Data Quality and Governance. Ensuring the quality and governance of data within a Data Lake can be challenging, requiring robust processes and tools.
- Security. Protecting sensitive data within a Data Lake is crucial, requiring comprehensive security measures.
Data Lakehouse
A Data Lakehouse is an innovative data management system that merges the strengths of Data Lakes and Data Warehouses. This hybrid approach strives to offer the adaptability and expansiveness of a Data Lake for housing extensive volumes of raw, unstructured data, while also providing the structured, refined data functionalities typical of a Data Warehouse. By bridging the gap between these two traditional data storage paradigms, Lakehouses enable more efficient data analytics, machine learning, and business intelligence operations across diverse data types and use cases.
Core Characteristics
- Unified Data Management. A Lakehouse streamlines data governance and security by managing both structured and unstructured data on one platform, reducing organizational data silos.
- Schema Flexibility. It supports schema-on-read and schema-on-write, allowing data to be stored and analysed flexibly. Data can be ingested in raw form and structured later or structured at ingestion.
- Scalability and Performance. Lakehouses scale storage and compute resources independently, handling large data volumes and complex analytics without performance compromise.
- Advanced Analytics and Machine Learning Integration. By providing direct access to both raw and processed data on a unified platform, Lakehouses facilitate advanced analytics, real-time analytics, and machine learning.
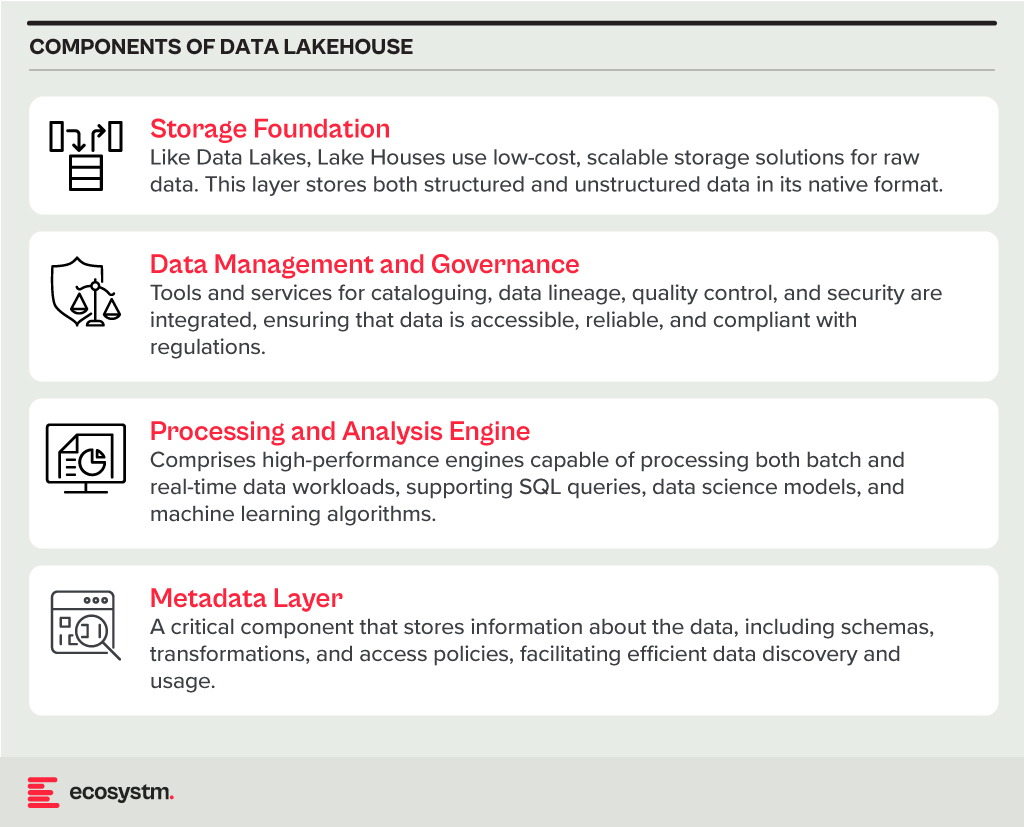
Benefits
- Versatility in Data Analysis. Lakehouses support diverse data analytics, spanning from traditional BI to advanced machine learning, all within one platform.
- Cost-Effective Scalability. The ability to scale storage and compute independently, often in a cloud environment, makes Lakehouses cost-effective for growing data needs.
- Improved Data Governance. Centralising data management enhances governance, security, and quality across all types of data.
Challenges
- Complexity in Implementation. Designing and implementing a Lakehouse architecture can be complex, requiring expertise in both Data Lakes and Data Warehouses.
- Data Consistency and Quality. Though crucial for reliable analytics, ensuring data consistency and quality across diverse data types and sources can be challenging.
- Governance and Security. Comprehensive data governance and security strategies are required to protect sensitive information and comply with regulations.
The choice between Data Warehouse, Data Lake, or Lakehouse systems is pivotal for businesses in harnessing the power of their data. Each option offers distinct advantages and challenges, requiring careful consideration of organisational needs and goals. By embracing the right data management system, organisations can pave the way for informed decision-making, operational efficiency, and innovation in the digital age.
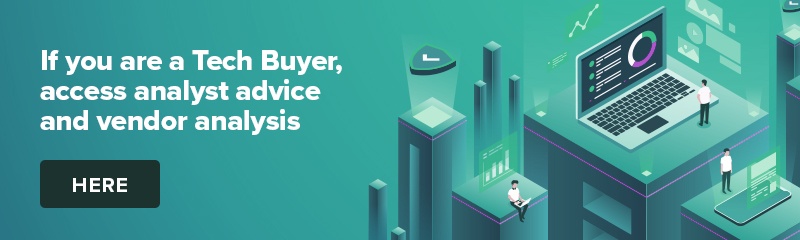
Historically, data scientists have been the linchpins in the world of AI and machine learning, responsible for everything from data collection and curation to model training and validation. However, as the field matures, we’re witnessing a significant shift towards specialisation, particularly in data engineering and the strategic role of Large Language Models (LLMs) in data curation and labelling. The integration of AI into applications is also reshaping the landscape of software development and application design.
The Growth of Embedded AI
AI is being embedded into applications to enhance user experience, optimise operations, and provide insights that were previously inaccessible. For example, natural language processing (NLP) models are being used to power conversational chatbots for customer service, while machine learning algorithms are analysing user behaviour to customise content feeds on social media platforms. These applications leverage AI to perform complex tasks, such as understanding user intent, predicting future actions, or automating decision-making processes, making AI integration a critical component of modern software development.
This shift towards AI-embedded applications is not only changing the nature of the products and services offered but is also transforming the roles of those who build them. Since the traditional developer may not possess extensive AI skills, the role of data scientists is evolving, moving away from data engineering tasks and increasingly towards direct involvement in development processes.
The Role of LLMs in Data Curation
The emergence of LLMs has introduced a novel approach to handling data curation and processing tasks traditionally performed by data scientists. LLMs, with their profound understanding of natural language and ability to generate human-like text, are increasingly being used to automate aspects of data labelling and curation. This not only speeds up the process but also allows data scientists to focus more on strategic tasks such as model architecture design and hyperparameter tuning.
The accuracy of AI models is directly tied to the quality of the data they’re trained on. Incorrectly labelled data or poorly curated datasets can lead to biased outcomes, mispredictions, and ultimately, the failure of AI projects. The role of data engineers and the use of advanced tools like LLMs in ensuring the integrity of data cannot be overstated.
The Impact on Traditional Developers
Traditional software developers have primarily focused on writing code, debugging, and software maintenance, with a clear emphasis on programming languages, algorithms, and software architecture. However, as applications become more AI-driven, there is a growing need for developers to understand and integrate AI models and algorithms into their applications. This requirement presents a challenge for developers who may not have specialised training in AI or data science. This is seeing an increasing demand for upskilling and cross-disciplinary collaboration to bridge the gap between traditional software development and AI integration.
Clear Role Differentiation: Data Engineering and Data Science
In response to this shift, the role of data scientists is expanding beyond the confines of traditional data engineering and data science, to include more direct involvement in the development of applications and the embedding of AI features and functions.
Data engineering has always been a foundational element of the data scientist’s role, and its importance has increased with the surge in data volume, variety, and velocity. Integrating LLMs into the data collection process represents a cutting-edge approach to automating the curation and labelling of data, streamlining the data management process, and significantly enhancing the efficiency of data utilisation for AI and ML projects.
Accurate data labelling and meticulous curation are paramount to developing models that are both reliable and unbiased. Errors in data labelling or poorly curated datasets can lead to models that make inaccurate predictions or, worse, perpetuate biases. The integration of LLMs into data engineering tasks is facilitating a transformation, freeing them from the burdens of manual data labelling and curation. This has led to a more specialised data scientist role that allocates more time and resources to areas that can create greater impact.
The Evolving Role of Data Scientists
Data scientists, with their deep understanding of AI models and algorithms, are increasingly working alongside developers to embed AI capabilities into applications. This collaboration is essential for ensuring that AI models are effectively integrated, optimised for performance, and aligned with the application’s objectives.
- Model Development and Innovation. With the groundwork of data preparation laid by LLMs, data scientists can focus on developing more sophisticated and accurate AI models, exploring new algorithms, and innovating in AI and ML technologies.
- Strategic Insights and Decision Making. Data scientists can spend more time analysing data and extracting valuable insights that can inform business strategies and decision-making processes.
- Cross-disciplinary Collaboration. This shift also enables data scientists to engage more deeply in interdisciplinary collaboration, working closely with other departments to ensure that AI and ML technologies are effectively integrated into broader business processes and objectives.
- AI Feature Design. Data scientists are playing a crucial role in designing AI-driven features of applications, ensuring that the use of AI adds tangible value to the user experience.
- Model Integration and Optimisation. Data scientists are also involved in integrating AI models into the application architecture, optimising them for efficiency and scalability, and ensuring that they perform effectively in production environments.
- Monitoring and Iteration. Once AI models are deployed, data scientists work on monitoring their performance, interpreting outcomes, and making necessary adjustments. This iterative process ensures that AI functionalities continue to meet user needs and adapt to changing data landscapes.
- Research and Continued Learning. Finally, the transformation allows data scientists to dedicate more time to research and continued learning, staying ahead of the rapidly evolving field of AI and ensuring that their skills and knowledge remain cutting-edge.
Conclusion
The integration of AI into applications is leading to a transformation in the roles within the software development ecosystem. As applications become increasingly AI-driven, the distinction between software development and AI model development is blurring. This convergence needs a more collaborative approach, where traditional developers gain AI literacy and data scientists take on more active roles in application development. The evolution of these roles highlights the interdisciplinary nature of building modern AI-embedded applications and underscores the importance of continuous learning and adaptation in the rapidly advancing field of AI.
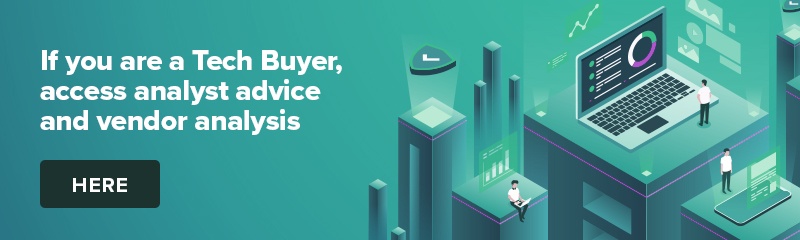
Healthcare delivery and healthtech have made significant strides; yet, the fundamental challenges in healthcare have remained largely unchanged for decades. The widespread acceptance and integration of digital solutions in recent years have supported healthcare providers’ primary goals of enhancing operational efficiency, better resource utilisation (with addressing skill shortages being a key driver), improving patient experience, and achieving better clinical outcomes. With governments pushing for advancements in healthcare outcomes at sustainable costs, the concept of value-based healthcare has gained traction across the industry.
Technology-driven Disruption
Healthcare saw significant disruptions four years ago, and while we will continue to feel the impact for the next decade, one positive outcome was witnessing the industry’s ability to transform amid such immense pressure. I am definitely not suggesting another healthcare calamity! But disruptions can have a positive impact – and I believe that technology will continue to disrupt healthcare at pace. Recently, my colleague Tim Sheedy shared his thoughts on how 2024 is poised to become the year of the AI startup, highlighting innovative options that organisations should consider in their AI journeys. AI startups and innovators hold the potential to further the “good disruption” that will transform healthcare.
Of course, there are challenges associated, including concerns on ethical and privacy-related issues, the reliability of technology – particularly while scaling – and on professional liability. However, the industry cannot overlook the substantial number of innovative startups that are using AI technologies to address some of the most pressing challenges in the healthcare industry.
Why Now?
AI is not new to healthcare. Many would cite the development of MYCIN – an early AI program aimed at identifying treatments for blood infections – as the first known example. It did kindle interest in research in AI and even during the 1980s and 1990s, AI brought about early healthcare breakthroughs, including faster data collection and processing, enhanced precision in surgical procedures, and research and mapping of diseases.
Now, healthcare is at an AI inflection point due to a convergence of three significant factors.
- Advanced AI. AI algorithms and capabilities have become more sophisticated, enabling them to handle complex healthcare data and tasks with greater accuracy and efficiency.
- Demand for Accessible Healthcare. Healthcare systems globally are striving for better care amid resource constraints, turning to AI for efficiency, cost reduction, and broader access.
- Consumer Demand. As people seek greater control over their health, personalised care has become essential. AI can analyse vast patient data to identify health risks and customise care plans, promoting preventative healthcare.
Promising Health AI Startups
As innovative startups continue to emerge in healthcare, we’re particularly keeping an eye on those poised to revolutionise diagnostics, care delivery, and wellness management. Here are some examples.
DIAGNOSTICS
- Claritas HealthTech has created advanced image enhancement software to address challenges in interpreting unclear medical images, improving image clarity and precision. A cloud-based platform with AI diagnostic tools uses their image enhancement technology to achieve greater predictive accuracy.
- Ibex offers Galen, a clinical-grade, multi-tissue platform to detect and grade cancers, that integrate with third-party digital pathology software solutions, scanning platforms, and laboratory information systems.
- MEDICAL IP is focused on advancing medical imaging analysis through AI and 3D technologies (such as 3D printing, CAD/CAM, AR/VR) to streamline medical processes, minimising time and costs while enhancing patient comfort.
- Verge Genomics is a biopharmaceutical startup employing systems biology to expedite the development of life-saving treatments for neurodegenerative diseases. By leveraging patient genomes, gene expression, and epigenomics, the platform identifies new therapeutic gene targets, forecasts effective medications, and categorises patient groups for enhanced clinical efficacy.
- X-Zell focuses on advanced cytology, diagnosing diseases through single atypical cells or clusters. Their plug-and-play solution detects, visualises, and digitises these phenomena in minimally invasive body fluids. With no complex specimen preparation required, it slashes the average sample-to-diagnosis time from 48 hours to under 4 hours.
CARE DELIVERY
- Abridge specialises in automating clinical notes and medical discussions for physicians, converting patient-clinician conversations into structured clinical notes in real time, powered by GenAI. It integrates seamlessly with EMRs such as Epic.
- Waltz Health offers AI-driven marketplaces aimed at reducing costs and innovative consumer tools to facilitate informed care decisions. Tailored for payers, pharmacies, and consumers, they introduce a fresh approach to pricing and reimbursing prescriptions that allows consumers to purchase medication at the most competitive rates, improving accessibility.
- Acorai offers a non-invasive intracardiac pressure monitoring device for heart failure management, aimed at reducing hospitalisations and readmissions. The technology can analyse acoustics, vibratory, and waveform data using ML to monitor intracardiac pressures.
WELLNESS MANAGEMENT
- Anya offers AI-driven support for women navigating life stages such as fertility, pregnancy, parenthood, and menopause. For eg. it provides support during the critical first 1,001 days of the parental journey, with personalised advice, tracking of developmental milestones, and connections with healthcare professionals.
- Dacadoo’s digital health engagement platform aims to motivate users to adopt healthier lifestyles through gamification, social connectivity, and personalised feedback. By analysing user health data, AI algorithms provide tailored insights, goal-setting suggestions, and challenges.
Conclusion
There is no question that innovative startups can solve many challenges for the healthcare industry. But startups flourish because of a supportive ecosystem. The health innovation ecosystem needs to be a dynamic network of stakeholders committed to transforming the industry and health outcomes – and this includes healthcare providers, researchers, tech companies, startups, policymakers, and patients. Together we can achieve the longstanding promise of accessible, cost-effective, and patient-centric healthcare.
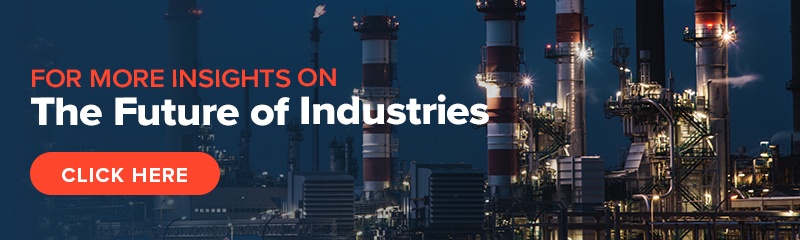