The White House has mandated federal agencies to conduct risk assessments on AI tools and appoint officers, including Chief Artificial Intelligence Officers (CAIOs), for oversight. This directive, led by the Office of Management and Budget (OMB), aims to modernise government AI adoption and promote responsible use. Agencies must integrate AI oversight into their core functions, ensuring safety, security, and ethical use. CAIOs will be tasked with assessing AI’s impact on civil rights and market competition. Agencies have until December 1, 2024, to address non-compliant AI uses, emphasising swift implementation.
How will this impact global AI adoption? Ecosystm analysts share their views.
Click here to download ‘Ensuring Ethical AI: US Federal Agencies’ New Mandate’ as a PDF.
The Larger Impact: Setting a Global Benchmark

This sets a potential global benchmark for AI governance, with the U.S. leading the way in responsible AI use, inspiring other nations to follow suit. The emphasis on transparency and accountability could boost public trust in AI applications worldwide.
The appointment of CAIOs across U.S. federal agencies marks a significant shift towards ethical AI development and application. Through mandated risk management practices, such as independent evaluations and real-world testing, the government recognises AI’s profound impact on rights, safety, and societal norms.
This isn’t merely a regulatory action; it’s a foundational shift towards embedding ethical and responsible AI at the heart of government operations. The balance struck between fostering innovation and ensuring public safety and rights protection is particularly noteworthy.
This initiative reflects a deep understanding of AI’s dual-edged nature – the potential to significantly benefit society, countered by its risks.
The Larger Impact: Blueprint for Risk Management

In what is likely a world first, AI brings together technology, legal, and policy leaders in a concerted effort to put guardrails around a new technology before a major disaster materialises. These efforts span from technology firms providing a form of legal assurance for use of their products (for example Microsoft’s Customer Copyright Commitment) to parliaments ratifying AI regulatory laws (such as the EU AI Act) to the current directive of installing AI accountability in US federal agencies just in the past few months.
It is universally accepted that AI needs risk management to be responsible and acceptable – installing an accountable C-suite role is another major step of AI risk mitigation.
This is an interesting move for three reasons:
- The balance of innovation versus governance and risk management.
- Accountability mandates for each agency’s use of AI in a public and transparent manner.
- Transparency mandates regarding AI use cases and technologies, including those that may impact safety or rights.
Impact on the Private Sector: Greater Accountability

AI Governance is one of the rare occasions where government action moves faster than private sector. While the immediate pressure is now on US federal agencies (and there are 438 of them) to identify and appoint CAIOs, the announcement sends a clear signal to the private sector.
Following hot on the heels of recent AI legislation steps, it puts AI governance straight into the Boardroom. The air is getting very thin for enterprises still in denial that AI governance has advanced to strategic importance. And unlike the CFC ban in the Eighties (the Montreal protocol likely set the record for concerted global action) this time the technology providers are fully onboard.
There’s no excuse for delaying the acceleration of AI governance and establishing accountability for AI within organisations.
Impact on Tech Providers: More Engagement Opportunities

Technology vendors are poised to benefit from the medium to long-term acceleration of AI investment, especially those based in the U.S., given government agencies’ preferences for local sourcing.
In the short term, our advice to technology vendors and service partners is to actively engage with CAIOs in client agencies to identify existing AI usage in their tools and platforms, as well as algorithms implemented by consultants and service partners.
Once AI guardrails are established within agencies, tech providers and service partners can expedite investments by determining which of their platforms, tools, or capabilities comply with specific guardrails and which do not.
Impact on SE Asia: Promoting a Digital Innovation Hub

By 2030, Southeast Asia is poised to emerge as the world’s fourth-largest economy – much of that growth will be propelled by the adoption of AI and other emerging technologies.
The projected economic growth presents both challenges and opportunities, emphasizing the urgency for regional nations to enhance their AI governance frameworks and stay competitive with international standards. This initiative highlights the critical role of AI integration for private sector businesses in Southeast Asia, urging organizations to proactively address AI’s regulatory and ethical complexities. Furthermore, it has the potential to stimulate cross-border collaborations in AI governance and innovation, bridging the U.S., Southeast Asian nations, and the private sector.
It underscores the global interconnectedness of AI policy and its impact on regional economies and business practices.
By leading with a strategic approach to AI, the U.S. sets an example for Southeast Asia and the global business community to reevaluate their AI strategies, fostering a more unified and responsible global AI ecosystem.
The Risks

U.S. government agencies face the challenge of sourcing experts in technology, legal frameworks, risk management, privacy regulations, civil rights, and security, while also identifying ongoing AI initiatives. Establishing a unified definition of AI and cataloguing processes involving ML, algorithms, or GenAI is essential, given AI’s integral role in organisational processes over the past two decades.
However, there’s a risk that focusing on AI governance may hinder adoption.
The role should prioritise establishing AI guardrails to expedite compliant initiatives while flagging those needing oversight. While these guardrails will facilitate “safe AI” investments, the documentation process could potentially delay progress.
The initiative also echoes a 20th-century mindset for a 21st-century dilemma. Hiring leaders and forming teams feel like a traditional approach. Today, organisations can increase productivity by considering AI and automation as initial solutions. Investing more time upfront to discover initiatives, set guardrails, and implement AI decision-making processes could significantly improve CAIO effectiveness from the outset.
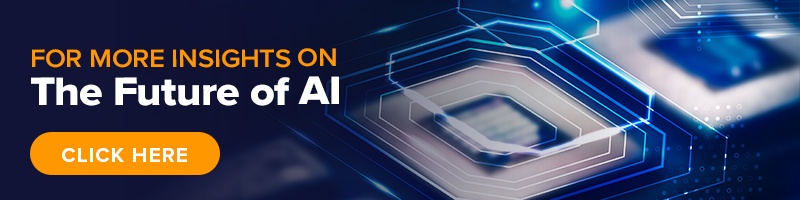
In this Insight, guest author Anirban Mukherjee lists out the key challenges of AI adoption in traditional organisations – and how best to mitigate these challenges. “I am by no means suggesting that traditional companies avoid or delay adopting AI. That would be akin to asking a factory to keep using only steam as power, even as electrification came in during early 20th century! But organisations need to have a pragmatic strategy around what will undoubtedly be a big, but necessary, transition.”
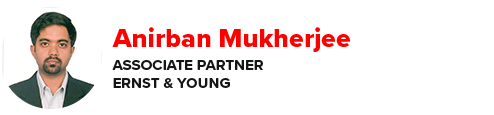
After years of evangelising digital adoption, I have more of a nuanced stance today – supporting a prudent strategy, especially where the organisation’s internal capabilities/technology maturity is in question. I still see many traditional organisations burning budgets in AI adoption programs with low success rates, simply because of poor choices driven by misplaced expectations. Without going into the obvious reasons for over-exuberance (media-hype, mis-selling, FOMO, irrational valuations – the list goes on), here are few patterns that can be detected in those organisations that have succeeded getting value – and gloriously so!
Data-driven decision-making is a cultural change. Most traditional organisations have a point person/role accountable for any important decision, whose “neck is on the line”. For these organisations to change over to trusting AI decisions (with its characteristic opacity, and stochastic nature of recommendations) is often a leap too far.
Work on your change management, but more crucially, strategically choose business/process decision points (aka use-cases) to acceptably AI-enable.
Technical choice of ML modeling needs business judgement too. The more flexible non-linear models that increase prediction accuracy, invariably suffer from lower interpretability – and may be a poor choice in many business contexts. Depending upon business data volumes and accuracy, model bias-variance tradeoffs need to be made. Assessing model accuracy and its thresholds (false-positive-false-negative trade-offs) are similarly nuanced. All this implies that organisation’s domain knowledge needs to merge well with data science design. A pragmatic approach would be to not try to be cutting-edge.
Look to use proven foundational model-platforms – such as those for NLP, visual analytics – for first use cases. Also note that not every problem needs AI; a lot can be sorted through traditional programming (“if-then automation”) and should be. The dirty secret of the industry is that the power of a lot of products marketed as “AI-powered” is mostly traditional logic, under the hood!
In getting results from AI, most often “better data trumps better models”. Practically, this means that organisations need to spend more on data engineering effort, than on data science effort. The CDO/CIO organisation needs to build the right balance of data competencies and tools.
Get the data readiness programs started – yesterday! While the focus of data scientists is often on training an AI model, deployment of the trained model online is a whole other level of technical challenge (particularly when it comes to IT-OT and real-time integrations).
It takes time to adopt AI in traditional organisations. Building up training data and model accuracy is a slow process. Organisational changes take time – and then you have to add considerations such as data standardisation; hygiene and integration programs; and the new attention required to build capabilities in AIOps, AI adoption and governance.
Typically plan for 3 years – monitor progress and steer every 6 months. Be ready to kill “zombie” projects along the way. Train the executive team – not to code, but to understand the technology’s capabilities and limitations. This will ensure better informed buyers/consumers and help drive adoption within the organisation.
I am by no means suggesting that traditional companies avoid or delay adopting AI. That would be akin to asking a factory to keep using only steam as power, even as electrification came in during early 20th century! But organisations need to have a pragmatic strategy around what will undoubtedly be a big, but necessary, transition.
These opinions are personal (and may change with time), but definitely informed through a decade of involvement in such journeys. It is not too early for any organisation to start – results are beginning to show for those who started earlier, and we know what they got right (and wrong).
I would love to hear your views, or even engage with you on your journey!
The views and opinions mentioned in the article are personal.
Anirban Mukherjee has more than 25 years of experience in operations excellence and technology consulting across the globe, having led transformations in Energy, Engineering, and Automotive majors. Over the last decade, he has focused on Smart Manufacturing/Industry 4.0 solutions that integrate cutting-edge digital into existing operations.
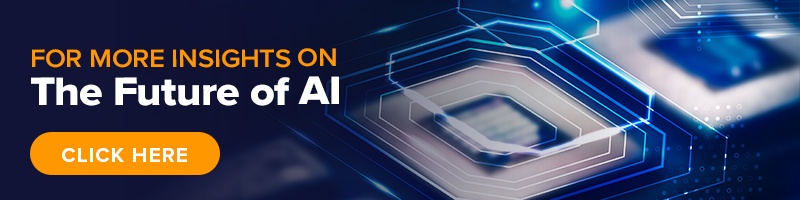