The promise of AI agents – intelligent programs or systems that autonomously perform tasks on behalf of people or systems – is enormous. These systems will augment and replace human workers, offering intelligence far beyond the simple RPA (Robotic Process Automation) bots that have become commonplace in recent years.
RPA and AI Agents both automate tasks but differ in scope, flexibility, and intelligence:
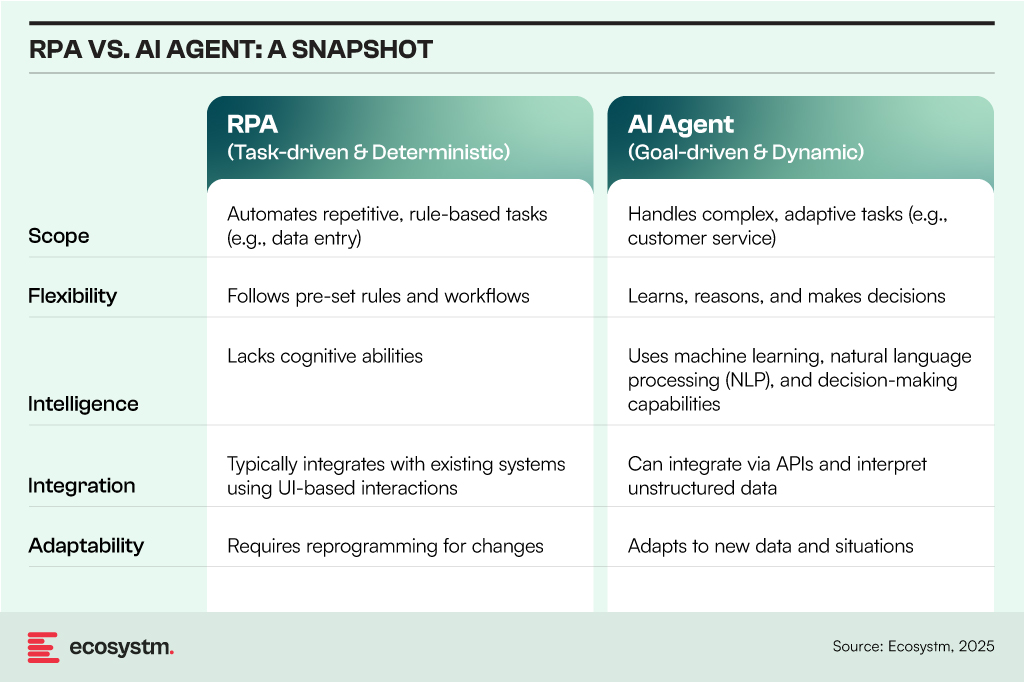
7 Lessons for AI Agents: Insights from RPA Deployments
However, in many ways, RPA and AI agents are similar – they both address similar challenges, albeit with different levels of automation and complexity. RPA adoption has shown that uncontrolled deployment leads to chaos, requiring a balance of governance, standardisation, and ongoing monitoring. The same principles apply to AI agent management, but with greater complexity due to AI’s dynamic and learning-based nature.
By learning from RPA’s mistakes, organisations can ensure AI agents deliver sustainable value, remain secure, and operate efficiently within a governed and well-managed environment.
#1 Controlling Sprawl with Centralised Governance
A key lesson from RPA adoption is that many organisations deployed RPA bots without a clear strategy, resulting in uncontrolled sprawl, duplicate bots, and fragmented automation efforts. This lack of oversight led to the rise of shadow IT practices, where business units created their own bots without proper IT involvement, further complicating the automation landscape and reducing overall effectiveness.
Application to AI Agents:
- Establish centralised governance early, ensuring alignment between IT and business units.
- Implement AI agent registries to track deployments, functions, and ownership.
- Enforce consistent policies for AI deployment, access, and version control.
#2 Standardising Development and Deployment
Bot development varied across teams, with different toolsets being used by different departments. This often led to poorly documented scripts, inconsistent programming standards, and difficulties in maintaining bots. Additionally, rework and inefficiencies arose as teams developed redundant bots, further complicating the automation process and reducing overall effectiveness.
Application to AI Agents:
- Standardise frameworks for AI agent development (e.g., predefined APIs, templates, and design patterns).
- Use shared models and foundational capabilities instead of building AI agents from scratch for each use case.
- Implement code repositories and CI/CD pipelines for AI agents to ensure consistency and controlled updates.
#3 Balancing Citizen Development with IT Control
Business users, or citizen developers, created RPA bots without adhering to IT best practices, resulting in security risks, inefficiencies, and technical debt. As a result, IT teams faced challenges in tracking and supporting business-driven automation efforts, leading to a lack of oversight and increased complexity in maintaining these bots.
Application to AI Agents:
- Empower business users to build and customise AI agents but within controlled environments (e.g., low-code/no-code platforms with governance layers).
- Implement AI sandboxes where experimentation is allowed but requires approval before production deployment.
- Establish clear roles and responsibilities between IT, AI governance teams, and business users.
#4 Proactive Monitoring and Maintenance
Organisations often underestimated the effort required to maintain RPA bots, resulting in failures when process changes, system updates, or API modifications occurred. As a result, bots frequently stopped working without warning, disrupting business processes and leading to unanticipated downtime and inefficiencies. This lack of ongoing maintenance and adaptation to evolving systems contributed to significant operational disruptions.
Application to AI Agents:
- Implement continuous monitoring and logging for AI agent activities and outputs.
- Develop automated retraining and feedback loops for AI models to prevent performance degradation.
- Create AI observability dashboards to track usage, drift, errors, and security incidents.
#5 Security, Compliance, and Ethical Considerations
Insufficient security measures led to data leaks and access control issues, with bots operating under overly permissive settings. Also, a lack of proactive compliance planning resulted in serious regulatory concerns, particularly within industries subject to stringent oversight, highlighting the critical need for integrating security and compliance considerations from the outset of automation deployments.
Application to AI Agents:
- Enforce role-based access control (RBAC) and least privilege access to ensure secure and controlled usage.
- Integrate explainability and auditability features to comply with regulations like GDPR and emerging AI legislation.
- Develop an AI ethics framework to address bias, ensure decision-making transparency, and uphold accountability.
#6 Cost Management and ROI Measurement
Initial excitement led to unchecked RPA investments, but many organisations struggled to measure the ROI of bots. As a result, some RPA bots became cost centres, with high maintenance costs outweighing the benefits they initially provided. This lack of clear ROI often hindered organisations from realising the full potential of their automation efforts.
Application to AI Agents:
- Define success metrics for AI agents upfront, tracking impact on productivity, cost savings, and user experience.
- Use AI workload optimisation tools to manage computing costs and avoid overconsumption of resources.
- Regularly review AI agents’ utility and retire underperforming ones to avoid AI bloat.
#7 Human Oversight and Hybrid Workflows
The assumption that bots could fully replace humans led to failures in situations where exceptions, judgment, or complex decision-making were necessary. Bots struggled to handle scenarios that required nuanced thinking or flexibility, often leading to errors or inefficiencies. The most successful implementations, however, blended human and bot collaboration, leveraging the strengths of both to optimise processes and ensure that tasks were handled effectively and accurately.
Application to AI Agents:
- Integrate AI agents into human-in-the-loop (HITL) systems, allowing humans to provide oversight and validate critical decisions.
- Establish AI escalation paths for situations where agents encounter ambiguity or ethical concerns.
- Design AI agents to augment human capabilities, rather than fully replace roles.
The lessons learned from RPA’s journey provide valuable insights for navigating the complexities of AI agent deployment. By addressing governance, standardisation, and ethical considerations, organisations
can shift from reactive problem-solving to a more strategic approach, ensuring AI tools deliver value while operating within a responsible, secure, and efficient framework.
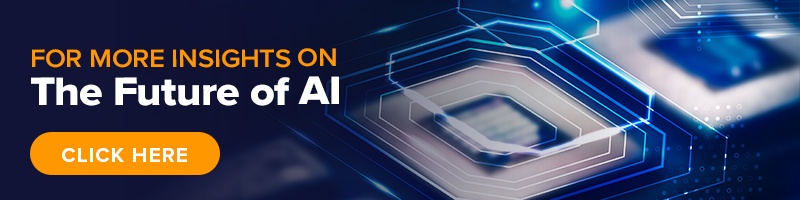
Data analysts play a vital role in today’s data-driven world, providing crucial insights that benefit decision-making processes. For those with a knack for numbers and a passion for uncovering patterns, a career as a data analyst can be both fulfilling and lucrative – it can also be a stepping stone towards other careers in data. While a data analyst focuses on data preparation and visualisation, an AI engineer specialises in creating AI solutions, a machine learning (ML) engineer concentrates on implementing ML models, and a data scientist combines elements of data analysis and ML to derive insights and predictions from data.
Tools, Skills, and Techniques of a Data Analyst
Excel Mastery. Unlocks a powerful toolbox for data manipulation and analysis. Essential skills include using a vast array of functions for calculations and data transformation. Pivot tables become your secret weapon for summarising and analysing large datasets, while charts and graphs bring your findings to life with visual clarity. Data validation ensures accuracy, and the Analysis ToolPak and Solver provide advanced functionalities for statistical analysis and complex problem-solving. Mastering Excel empowers you to transform raw data into actionable insights.
Advanced SQL. While basic skills handle simple queries, advanced users can go deeper with sorting, aggregation, and the art of JOINs to combine data from multiple tables. Common Table Expressions (CTEs) and subqueries become your allies for crafting complex queries, while aggregate functions summarise vast amounts of data. Window functions add another layer of power, allowing calculations within query results. Mastering Advanced SQL empowers you to extract hidden insights and manage data with unparalleled precision.
Data Visualisation. Crafts impactful data stories. These tools empower you to connect to various data sources, transform raw information into a usable format, and design interactive dashboards and reports. Filters and drilldowns allow users to explore your data from different angles, while calculated fields unlock deeper analysis. Parameters add a final touch of flexibility, letting viewers customise the report to their specific needs. With tools Tableau and Power BI, complex data becomes clear and engaging.
Essential Python. This powerful language excels at data analysis and automation. Libraries like NumPy and Pandas become your foundation for data manipulation and wrangling. Scikit-learn empowers you to build ML models, while SciPy and StatsModels provide a toolkit for in-depth statistical analysis. Python’s ability to interact with APIs and web scrape data expands its reach, and its automation capabilities streamline repetitive tasks. With Essential Python, you have the power to solve complex problems.
Automating the Journey. Data analysts can be masters of efficiency, and their skills translate beautifully into AI. Scripting languages like Ansible and Terraform automate repetitive tasks. Imagine streamlining the process of training and deploying AI models – a skill that directly benefits the AI development pipeline. This proficiency in automation showcases the valuable foundation data analysts provide for building and maintaining AI systems.
Developing ML Expertise. Transitioning from data analysis to AI involves building on your existing skills to develop ML expertise. As a data analyst, you may start with basic predictive models. This knowledge is expanded in AI to include deep learning and advanced ML algorithms. Also, skills in statistical analysis and visualisation help in evaluating the performance of AI models.
Growing Your AI Skills
Becoming an AI engineer requires building on a data analysis foundation to focus on advanced skills such as:
- Deep Learning. Learning frameworks like TensorFlow and PyTorch to build and train neural networks.
- Natural Language Processing (NLP). Techniques for processing and analysing large amounts of natural language data.
- AI Ethics and Fairness. Understanding the ethical implications of AI and ensuring models are fair and unbiased.
- Big Data Technologies. Using tools like Hadoop and Spark for handling large-scale data is essential for AI applications.
The Evolution of a Data Analyst: Career Opportunities
Data analysis is a springboard to AI engineering. Businesses crave talent that bridges the data-AI gap. Your data analyst skills provide the foundation (understanding data sources and transformations) to excel in AI. As you master ML, you can progress to roles like:
- AI Engineer. Works on integrating AI solutions into products and services. They work with AI frameworks like TensorFlow and PyTorch, ensuring that AI models are incorporated into products and services in a fair and unbiased manner.
- ML Engineer. Focuses on designing and implementing ML models. They focus on preprocessing data, evaluating model performance, and collaborating with data scientists and engineers to bring models into production. They need strong programming skills and experience with big data tools and ML algorithms.
- Data Scientist. Bridges the gap between data analysis and AI, often involved in both data preparation and model development. They perform exploratory data analysis, develop predictive models, and collaborate with cross-functional teams to solve complex business problems. Their role requires a comprehensive understanding of both data analysis and ML, as well as strong programming and data visualisation skills.
Conclusion
Hone your data expertise and unlock a future in AI! Mastering in-demand skills like Excel, SQL, Python, and data visualisation tools will equip you to excel as a data analyst. Your data wrangling skills will be invaluable as you explore ML and advanced algorithms. Also, your existing BI knowledge translates seamlessly into building and evaluating AI models. Remember, the data landscape is constantly evolving, so continue to learn to stay at the forefront of this dynamic field. By combining your data skills with a passion for AI, you’ll be well-positioned to tackle complex challenges and shape the future of AI.
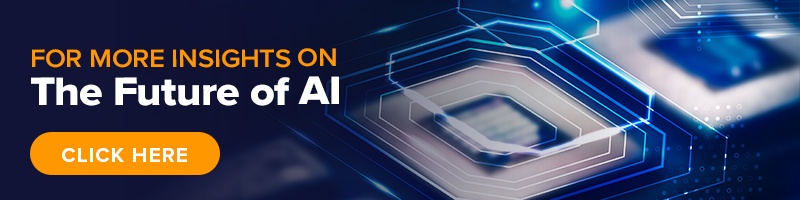
The process of developing advertising campaigns is evolving with the increasing use of artificial intelligence (AI). Advertisers want to optimise the amount of data at their disposal to craft better campaigns and drive more impact. Since early 2020, there has been a real push to integrate AI to help measure the effectiveness of campaigns and where to allocate ad spend. This now goes beyond media targeting and includes planning, analytics and creative. AI can assist in pattern matching, tailoring messages through AI-enabled hyper-personalisation, and analysing traffic to communicate through pattern identification of best times and means of communication. AI is being used to create ad copy; and social media and online advertising platforms are starting to roll out tools that help advertisers create better ads.
Ecosystm research shows that Media companies report optimisation, targeting and administrative functions such as billing are aided by AI use (Figure 1). However, the trend of Media companies leveraging AI for content design and media analysis is growing.
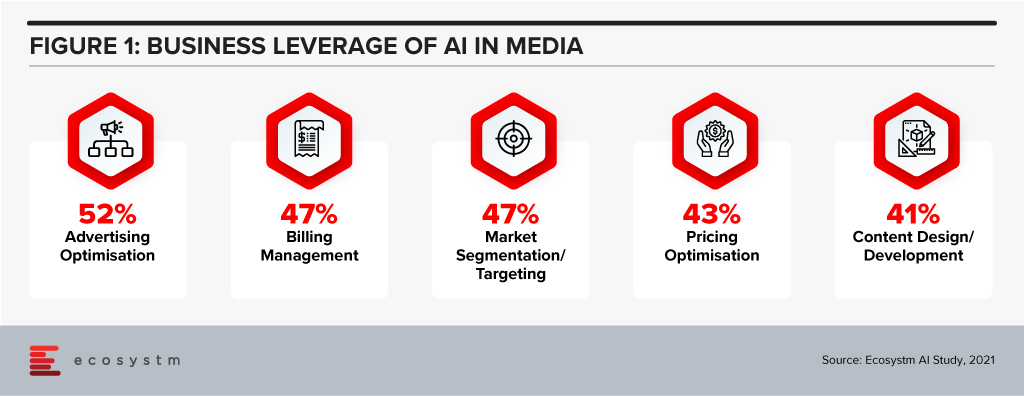
WPP Strengthening Tech Capabilities
This week, WPP announced the acquisition of Satalia, a UK-based company, who will consult with all WPP agencies globally to promote AI capabilities across the company and help shape the company’s AI strategy, including research and development, AI ethics, partnerships, talent and products.
It was announced that Satalia, whose clients include BT, DFS, DS Smith, PwC, Gigaclear, Tesco and Unilever, will join Wunderman Thompson Commerce to work on the technology division of their global eCommerce consultancy. Prior to the acquisition, Satalia had launched tools such as Satalia Workforce to automate work assignments; and Satalia Delivery, for automated delivery routes and schedules. The tools have been adopted by companies including PwC, DFS, Selecta and Australian supermarket chain Woolworths.
Like other global advertising organisations, WPP has been focused on expanding the experience, commerce and technology parts of the business, most recently acquiring Brazilian software engineering company DTI Digital in February. WPP also launched their own global data consultancy, Choreograph, in April. Choreograph is WPP’s newly formed global data products and technology company focused on helping brands activate new customer experiences by turning data into intelligence. This article from last year from the WPP CTO is an interesting read on their technology strategy, especially their move to cloud to enable their strategy.

Ethics & AI – The Right Focus
The acquisition of Satalia will give WPP and opportunity to evaluate important areas such as AI ethics, partnerships and talent which will be significantly important in the medium term. AI ethics in advertising is also a longer-term discussion. With AI and machine learning, the system learns patterns that help steer targeting towards audiences that are more likely to convert and identify the best places to get your message in front of these buyers. If done responsibly it should provide consumers with the ability to learn about and purchase relevant products and services. However, as we have recently discussed, AI has two main forms of bias – underrepresented data and developer bias – that also needs to be looked into.
Summary
The role of AI in the orchestration of the advertising process is developing rapidly. Media firms are adopting cloud platforms, making IP investments, and developing partnerships to build the support they can offer with their advertising services. The use of AI in advertising will help mature and season the process to be even more tailored to customer preferences.
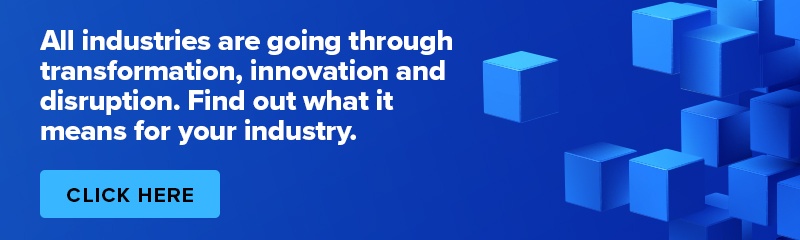