In 2024, technology vendors have heavily invested in AI Agents, recognising their potential to drive significant value. These tools leverage well-governed, small datasets to integrate seamlessly with applications like Workday, Salesforce, ServiceNow, and Dayforce, enhancing processes and outcomes.
2025 is poised to be the year of AI Agent adoption. Designed to automate specific tasks within existing workflows, AI Agents will transform customer experiences, streamline operations, and boost efficiency. Unlike traditional AI deployments, they offer a gradual, non-disruptive approach, augmenting human capabilities without overhauling processes. As organisations adopt new software versions with embedded AI capabilities, 2025 will mark a pivotal shift in customer experience delivery.
Ecosystm analysts Audrey William, Melanie Disse, and Tim Sheedy present the top 5 trends shaping customer experience in 2025.
Click here to download ‘AI-Powered Customer Experience: Top 5 Trends for 2025’ as a PDF
1. AI Won’t Wow Many Customers in 2025
The data is in – the real focus of AI over the next few years will be on productivity and cost savings.
Senior management and boards of directors want to achieve more with less – so even when AI is being used to serve customers, it will be focused on reducing back-end and human costs.
There will be exceptions, such as the adoption of AI agents in contact centres. However, AI agents must match or exceed human performance to see broad adoption.
However, the primary focus in contact centres will be on reducing Average Handling Time (AHT), increasing call volume per agent, accelerating agent onboarding, and automating customer follow-ups.
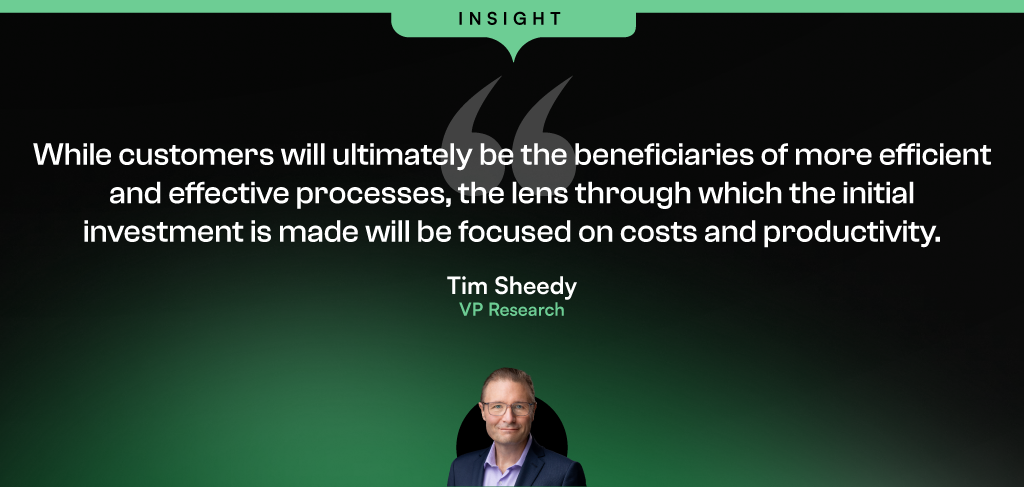
2. Organisations Will Start Treating CX as a Team Sport
As CX programs mature, 2025 will highlight the need to break down not only data and technology siloes but also organisational and cultural barriers to achieve AI-powered CX and business success.
AI and GenAI have unlocked new sources of customer data, prompting leaders to reorganise and adopt a mindset shift about CX. This involves redefining CX as a collective effort, engaging the entire organisation in the journey.
Technologies and KPIs must be aligned to drive customer AND business needs, not purely driving success in siloed areas.
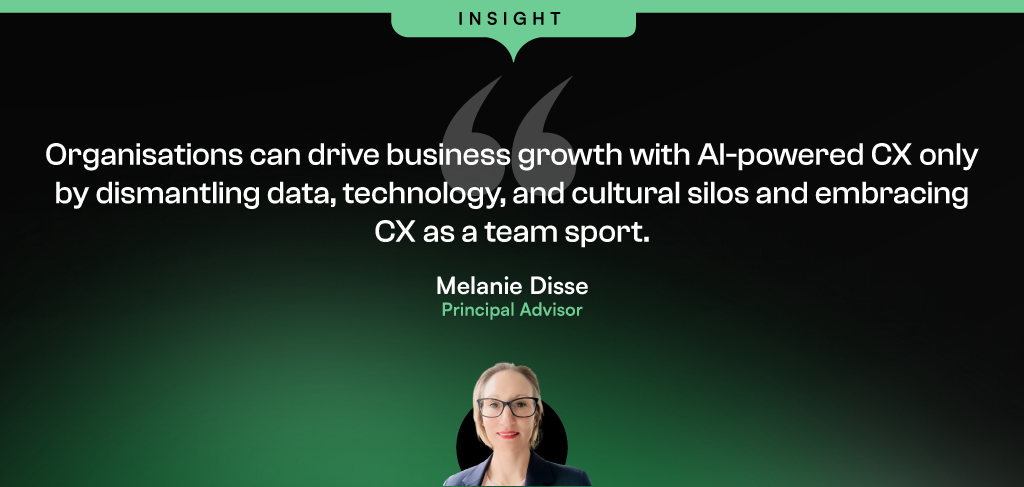
3. The First “AGI Agents” Will Emerge
AI Agents are set to explode in 2025, but even more disruptive developments in AI are on the horizon.
As conversational computing gains traction, fuelled by advances in GenAI and progress toward AGI, “Complex AI Agents” will emerge.
These “AGI Agents” will mimic certain human-like capabilities, though not fully replicating human cognition, earning their “Agent” designation.
The first use cases will likely be in software development, where these agents will act as intelligent platforms capable of transforming a described digital process or service into reality. They may include design, inbuilt testing, quality assurance, and the ability to learn from existing IP (e.g., “create an app with the same capabilities as X”).
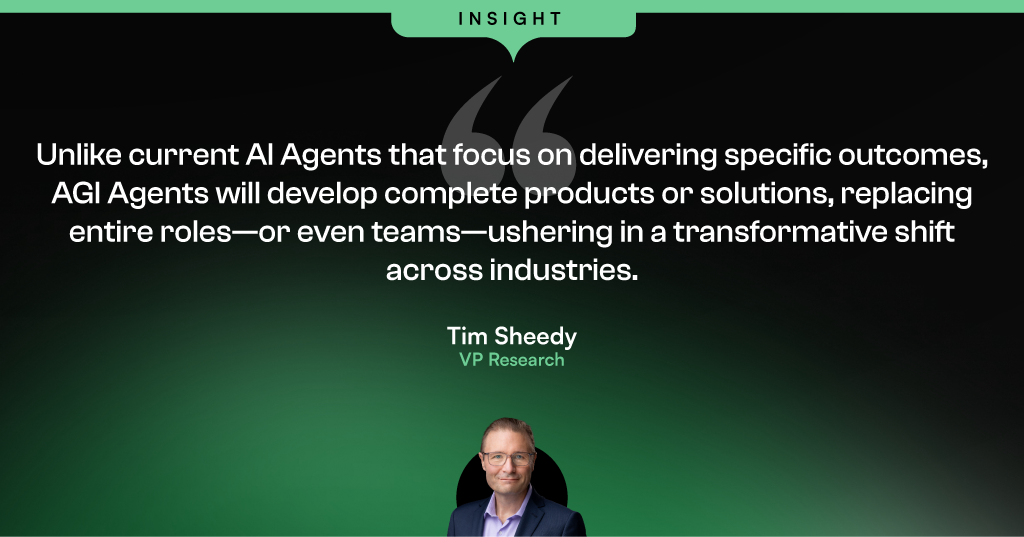
4. Intelligent AI Bots Will Enhance Contact Centre Efficiency
The often-overlooked aspect of CX is the “operational side”, where Operations Managers face significant challenges in maintaining a real-time pulse on contact centre activities.
For most organisations, this remains a highly manual and reactive process. Intelligent workflow bots can revolutionise this by acting as gatekeepers, instantly identifying issues and triggering real-time corrective actions. These bots can even halt processes causing customer dissatisfaction, ensuring problems are addressed proactively.
Operational inefficiencies, such as back-office delays, unanswered emails, and slow issue containment, create constant headaches. Integrating bots into contact centre operations will significantly reduce time wasted on these inefficiencies, enhancing both employee and customer experiences.
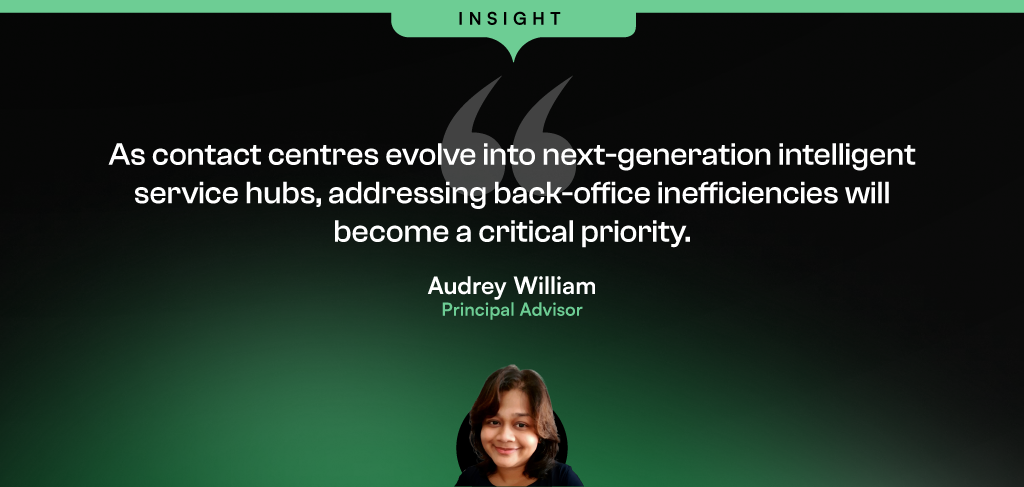
5. Employee Experience Will Catch Up to CX Maturity
Employee experience (EX) has traditionally lagged behind CX in focus and technology investment. However, AI-powered technologies are now enabling organisations to apply CX use cases to EX efforts, using advanced data analysis, summaries, and recommendations.
AI and GenAI tools will enhance understanding of employee satisfaction and engagement while predicting churn and retention drivers.
HR teams and leaders will leverage these tools to optimise performance management and improve hiring and retention outcomes.
Additionally, organisations will begin to connect EX with financial performance, identifying key drivers of engagement and linking them to business success. This shift will position EX as a strategic priority, integral to achieving organisational goals.
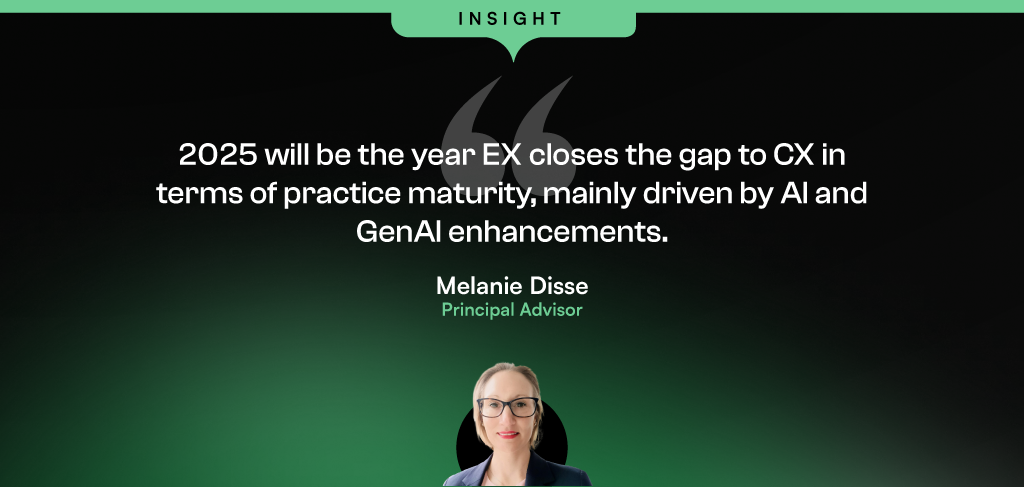
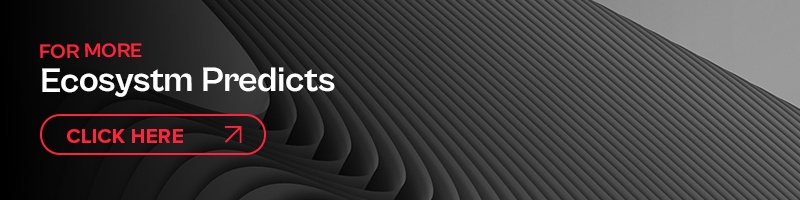