GenAI has taken the world by storm, with organisations big and small eager to pilot use cases for automation and productivity boosts. Tech giants like Google, AWS, and Microsoft are offering cloud-based GenAI tools, but the demand is straining current infrastructure capabilities needed for training and deploying large language models (LLMs) like ChatGPT and Bard.
Understanding the Demand for Chips
The microchip manufacturing process is intricate, involving hundreds of steps and spanning up to four months from design to mass production. The significant expense and lengthy manufacturing process for semiconductor plants have led to global demand surpassing supply. This imbalance affects technology companies, automakers, and other chip users, causing production slowdowns.
Supply chain disruptions, raw material shortages (such as rare earth metals), and geopolitical situations have also had a fair role to play in chip shortages. For example, restrictions by the US on China’s largest chip manufacturer, SMIC, made it harder for them to sell to several organisations with American ties. This triggered a ripple effect, prompting tech vendors to start hoarding hardware, and worsening supply challenges.
As AI advances and organisations start exploring GenAI, specialised AI chips are becoming the need of the hour to meet their immense computing demands. AI chips can include graphics processing units (GPUs), application-specific integrated circuits (ASICs), and field-programmable gate arrays (FPGAs). These specialised AI accelerators can be tens or even thousands of times faster and more efficient than CPUs when it comes to AI workloads.
The surge in GenAI adoption across industries has heightened the demand for improved chip packaging, as advanced AI algorithms require more powerful and specialised hardware. Effective packaging solutions must manage heat and power consumption for optimal performance. TSMC, one of the world’s largest chipmakers, announced a shortage in advanced chip packaging capacity at the end of 2023, that is expected to persist through 2024.
The scarcity of essential hardware, limited manufacturing capacity, and AI packaging shortages have impacted tech providers. Microsoft acknowledged the AI chip crunch as a potential risk factor in their 2023 annual report, emphasising the need to expand data centre locations and server capacity to meet customer demands, particularly for AI services. The chip squeeze has highlighted the dependency of tech giants on semiconductor suppliers. To address this, companies like Amazon and Apple are investing heavily in internal chip design and production, to reduce dependence on large players such as Nvidia – the current leader in AI chip sales.
How are Chipmakers Responding?
NVIDIA, one of the largest manufacturers of GPUs, has been forced to pivot its strategy in response to this shortage. The company has shifted focus towards developing chips specifically designed to handle complex AI workloads, such as the A100 and V100 GPUs. These AI accelerators feature specialised hardware like tensor cores optimised for AI computations, high memory bandwidth, and native support for AI software frameworks.
While this move positions NVIDIA at the forefront of the AI hardware race, experts say that it comes at a significant cost. By reallocating resources towards AI-specific GPUs, the company’s ability to meet the demand for consumer-grade GPUs has been severely impacted. This strategic shift has worsened the ongoing GPU shortage, further straining the market dynamics surrounding GPU availability and demand.
Others like Intel, a stalwart in traditional CPUs, are expanding into AI, edge computing, and autonomous systems. A significant competitor to Intel in high-performance computing, AMD acquired Xilinx to offer integrated solutions combining high-performance central processing units (CPUs) and programmable logic devices.
Global Resolve Key to Address Shortages
Governments worldwide are boosting chip capacity to tackle the semiconductor crisis and fortify supply chains. Initiatives like the CHIPS for America Act and the European Chips Act aim to bolster domestic semiconductor production through investments and incentives. Leading manufacturers like TSMC and Samsung are also expanding production capacities, reflecting a global consensus on self-reliance and supply chain diversification. Asian governments are similarly investing in semiconductor manufacturing to address shortages and enhance their global market presence.
Japan is providing generous government subsidies and incentives to attract major foreign chipmakers such as TSMC, Samsung, and Micron to invest and build advanced semiconductor plants in the country. Subsidies have helped to bring greenfield investments in Japan’s chip sector in recent years. TSMC alone is investing over USD 20 billion to build two cutting-edge plants in Kumamoto by 2027. The government has earmarked around USD 13 billion just in this fiscal year to support the semiconductor industry.
Moreover, Japan’s collaboration with the US and the establishment of Rapidus, a memory chip firm, backed by major corporations, further show its ambitions to revitalise its semiconductor industry. Japan is also looking into advancements in semiconductor materials like silicon carbide (SiC) and gallium nitride (GaN) – crucial for powering electric vehicles, renewable energy systems, and 5G technology.
South Korea. While Taiwan holds the lead in semiconductor manufacturing volume, South Korea dominates the memory chip sector, largely due to Samsung. The country is also spending USD 470 billion over the next 23 years to build the world’s largest semiconductor “mega cluster” covering 21,000 hectares in Gyeonggi Province near Seoul. The ambitious project, a partnership with Samsung and SK Hynix, will centralise and boost self-sufficiency in chip materials and components to 50% by 2030. The mega cluster is South Korea’s bold plan to cement its position as a global semiconductor leader and reduce dependence on the US amidst growing geopolitical tensions.
Vietnam. Vietnam is actively positioning itself to become a major player in the global semiconductor supply chain amid the push to diversify away from China. The Southeast Asian nation is offering tax incentives, investing in training tens of thousands of semiconductor engineers, and encouraging major chip firms like Samsung, Nvidia, and Amkor to set up production facilities and design centres. However, Vietnam faces challenges such as a limited pool of skilled labour, outdated energy infrastructure leading to power shortages in key manufacturing hubs, and competition from other regional players like Taiwan and Singapore that are also vying for semiconductor investments.
The Potential of SLMs in Addressing Infrastructure Challenges
Small language models (SLMs) offer reduced computational requirements compared to larger models, potentially alleviating strain on semiconductor supply chains by deploying on smaller, specialised hardware.
Innovative SLMs like Google’s Gemini Nano and Mistral AI’s Mixtral 8x7B enhance efficiency, running on modest hardware, unlike their larger counterparts. Gemini Nano is integrated into Bard and available on Pixel 8 smartphones, while Mixtral 8x7B supports multiple languages and suits tasks like classification and customer support.
The shift towards smaller AI models can be pivotal to the AI landscape, democratising AI and ensuring accessibility and sustainability. While they may not be able to handle complex tasks as well as LLMs yet, the ability of SLMs to balance model size, compute power, and ethical considerations will shape the future of AI development.
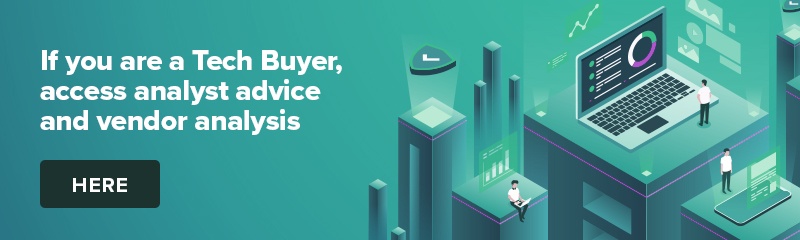
Banks, insurers, and other financial services organisations in Asia Pacific have plenty of tech challenges and opportunities including cybersecurity and data privacy management; adapting to tech and customer demands, AI and ML integration; use of big data for personalisation; and regulatory compliance across business functions and transformation journeys.
Modernisation Projects are Back on the Table
An emerging tech challenge lies in modernising, replacing, or retiring legacy platforms and systems. Many banks still rely on outdated core systems, hindering agility, innovation, and personalised customer experiences. Migrating to modern, cloud-based systems presents challenges due to complexity, cost, and potential disruptions. Insurers are evaluating key platforms amid evolving customer needs and business models; ERP and HCM systems are up for renewal; data warehouses are transforming for the AI era; even CRM and other CX platforms are being modernised as older customer data stores and models become obsolete.
For the past five years, many financial services organisations in the region have sidelined large legacy modernisation projects, opting instead to make incremental transformations around their core systems. However, it is becoming critical for them to take action to secure their long-term survival and success.
Benefits of legacy modernisation include:
- Improved operational efficiency and agility
- Enhanced customer experience and satisfaction
- Increased innovation and competitive advantage
- Reduced security risks and compliance costs
- Preparation for future technologies
However, legacy modernisation and migration initiatives carry significant risks. For instance, TSB faced a USD 62M fine due to a failed mainframe migration, resulting in severe disruptions to branch operations and core banking functions like telephone, online, and mobile banking. The migration failure led to 225,492 complaints between 2018 and 2019, affecting all 550 branches and required TSB to pay more than USD 25M to customers through a redress program.
Modernisation Options
- Rip and Replace. Replacing the entire legacy system with a modern, cloud-based solution. While offering a clean slate and faster time to value, it’s expensive, disruptive, and carries migration risks.
- Refactoring. Rewriting key components of the legacy system with modern languages and architectures. It’s less disruptive than rip-and-replace but requires skilled developers and can still be time-consuming.
- Encapsulation. Wrapping the legacy system with a modern API layer, allowing integration with newer applications and tools. It’s quicker and cheaper than other options but doesn’t fully address underlying limitations.
- Microservices-based Modernisation. Breaking down the legacy system into smaller, independent services that can be individually modernised over time. It offers flexibility and agility but requires careful planning and execution.
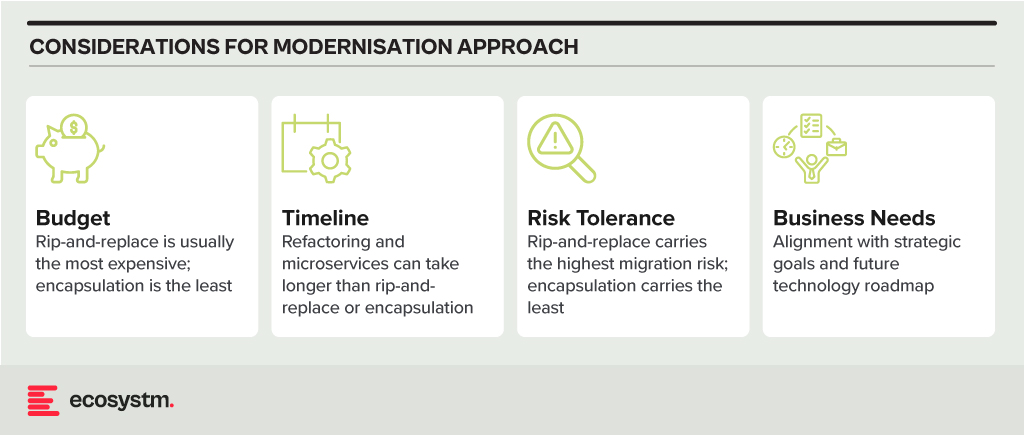
Financial Systems on the Block for Legacy Modernisation
Data Analytics Platforms. Harnessing customer data for insights and targeted offerings is vital. Legacy data warehouses often struggle with real-time data processing and advanced analytics.
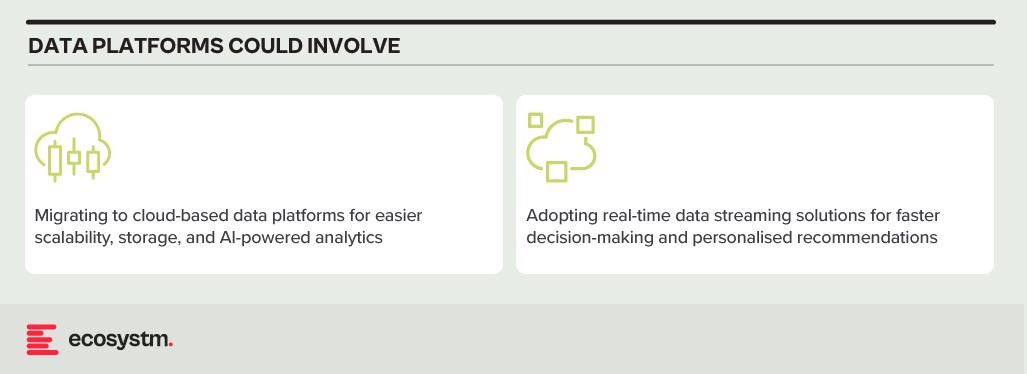
CRM Systems. Effective customer interactions require integrated CRM platforms. Outdated systems might hinder communication, personalisation, and cross-selling opportunities.
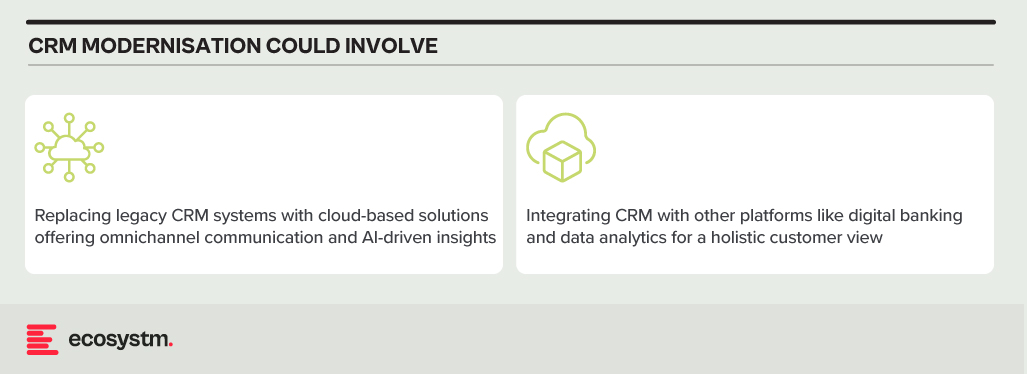
Payment Processing Systems. Legacy systems might lack support for real-time secure transactions, mobile payments, and cross-border transactions.
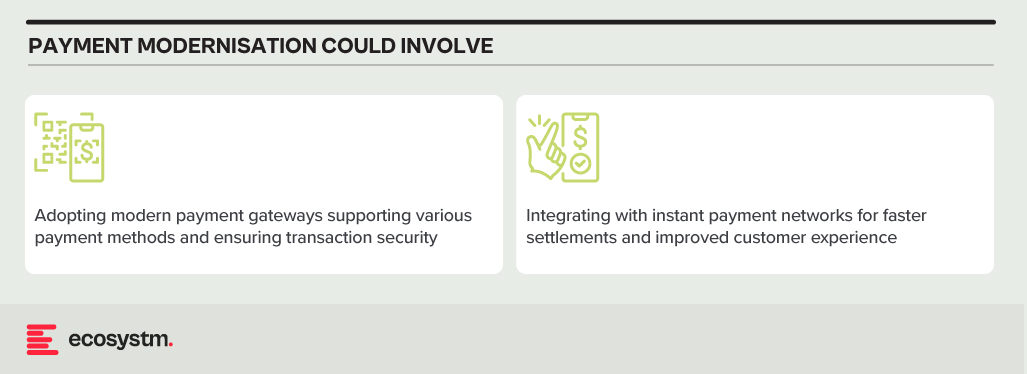
Core Banking Systems (CBS). The central nervous system of any bank, handling account management, transactions, and loan processing. Many Asia Pacific banks rely on aging, monolithic CBS with limited digital capabilities.
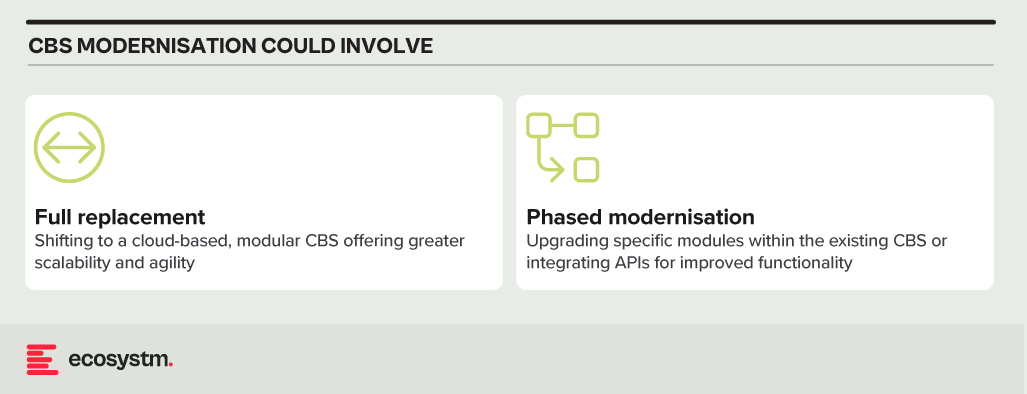
Digital Banking Platforms. While several Asia Pacific banks provide basic online banking, genuine digital transformation requires mobile-first apps with features such as instant payments, personalised financial management tools, and seamless third-party service integration.
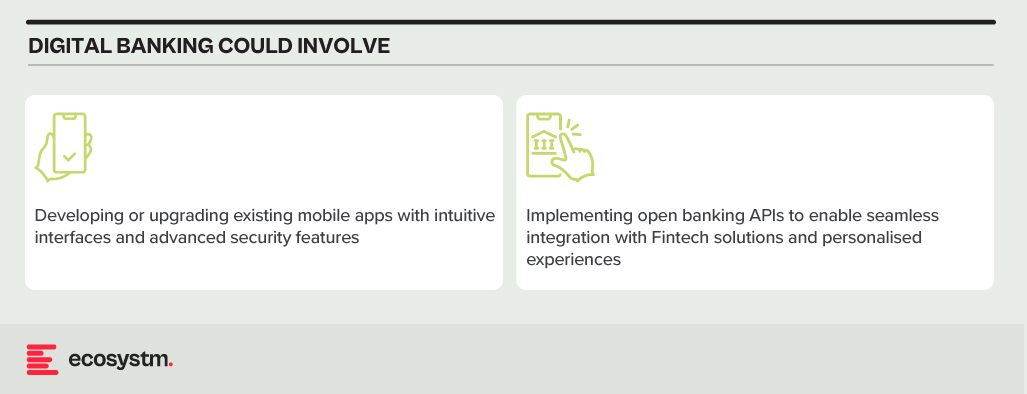
Modernising Technical Approaches and Architectures
Numerous technical factors need to be addressed during modernisation, with decisions needing to be made upfront. Questions around data migration, testing and QA, change management, data security and development methodology (agile, waterfall or hybrid) need consideration.
Best practices in legacy migration have taught some lessons.
Adopt a data fabric platform. Many organisations find that centralising all data into a single warehouse or platform rarely justifies the time and effort invested. Businesses continually generate new data, adding sources, and updating systems. Managing data where it resides might seem complex initially. However, in the mid to longer term, this approach offers clearer benefits as it reduces the likelihood of data discrepancies, obsolescence, and governance challenges.
Focus modernisation on the customer metrics and journeys that matter. Legacy modernisation need not be an all-or-nothing initiative. While systems like mainframes may require complete replacement, even some mainframe-based software can be partially modernised to enable services for external applications and processes. Assess the potential of modernising components of existing systems rather than opting for a complete overhaul of legacy applications.
Embrace the cloud and SaaS. With the growing network of hyperscaler cloud locations and data centres, there’s likely to be a solution that enables organisations to operate in the cloud while meeting data residency requirements. Even if not available now, it could align with the timeline of a multi-year legacy modernisation project. Whenever feasible, prioritise SaaS over cloud-hosted applications to streamline management, reduce overhead, and mitigate risk.
Build for customisation for local and regional needs. Many legacy applications are highly customised, leading to inflexibility, high management costs, and complexity in integration. Today, software providers advocate minimising configuration and customisation, opting for “out-of-the-box” solutions with room for localisation. The operations in different countries may require reconfiguration due to varying regulations and competitive pressures. Architecting applications to isolate these configurations simplifies system management, facilitating continuous improvement as new services are introduced by platform providers or ISV partners.
Explore the opportunity for emerging technologies. Emerging technologies, notably AI, can significantly enhance the speed and value of new systems. In the near future, AI will automate much of the work in data migration and systems integration, reducing the need for human involvement. When humans are required, low-code or no-code tools can expedite development. Private 5G services may eliminate the need for new network builds in branches or offices. AIOps and Observability can improve system uptime at lower costs. Considering these capabilities in platform decisions and understanding the ecosystem of partners and providers can accelerate modernisation journeys and deliver value faster.
Don’t Let Analysis Paralysis Slow Down Your Journey!
Yes, there are a lot of decisions that need to be made; and yes, there is much at stake if things go wrong! However, there’s a greater risk in not taking action. Maintaining a laser-focus on the customer and business outcomes that need to be achieved will help align many decisions. Keeping the customer experience as the guiding light ensures organisations are always moving in the right direction.
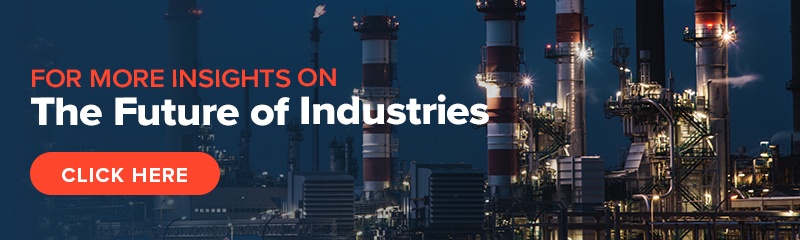
Since officially separating from IBM in November last year, Kyndryl has been busy cementing some heavyweight partnerships. The alliances with Microsoft, Google, and VMware demonstrate its intention to build hybrid cloud solutions with whoever it needs to, rather than favouring the Big Blue or Red Hat. The SAP tie-up hints at a future of migrating ERP workloads to the cloud and even an eye on moving up the application stack. Last week Kyndryl announced it is working with Nokia to provide private 5G and LTE networks to enable Industry 4.0 solutions. The first customer reference for the partnership is Dow, deploying both real-world and proof-of-concept applications for worker safety and collaboration and asset tracking.
The Partnership
Kyndryl has a competitive networking services unit, particularly in partnership with Cisco. Its focus has been on SD-WAN, campus networks, and network management as part of broader cloud services deals. This 5G partnership with Nokia is its first serious effort to work with one of the major carrier-grade vendors using cellular technology. It creates an opportunity for Kyndryl to position itself as a provider of services that underpin IoT and edge applications, rather than only cloud, which has until now been its main strength.
Prior to the Kyndryl announcement, Nokia was already developing private 5G solutions under the moniker Digital Automation Cloud (DAC). A key customer is Volkswagen, using the network to connect robots and wireless assembly tools. Over-the-air vehicle updates are also tested over the private network. Volkswagen operates in a dedicated 3.7-3.8 GHz band, which was allocated by the Federal Network Agency in Germany. This illustrates a third option for accessing spectrum, which will become an important consideration in private 5G rollouts.
Private 5G Use Cases
Private 5G has several benefits such as low latency, long-range, support for many users per access point, and provision for devices that are mobile due to handover. It is unlikely that it will completely replace other technologies, like wireless LAN, but it is very compelling for certain use cases.
Private 5G is useful on large sites, like mines, ports, farms, and warehouses where connected machines are moving about or some devices – like perimeter security cameras – are just out of reach. Utilities, like power, gas, and water, with infrastructure that needs to be monitored over long distances, will also start looking at it as a part of their predictive maintenance and resiliency systems. Low latency will become increasingly important as we see more and more customer-facing digital services delivered on-site and autonomous robots in the production environment.
Another major benefit of private 5G compared to operating on public service is that data can remain within the organisation’s own network for as long as possible, providing more security and control.
Private 5G Gaining Popularity
There has been a lot of activity over the last year in this space, with the hyperscalers, telecom providers and network equipment vendors developing private 5G offerings.
Last year, the AWS Private 5G was announced, a managed service that includes core network hardware, small-cell radio units, SIM cards, servers, and software. The service operates over a shared spectrum, like the Citizens Broadband Radio Service (CBRS) in the US, where the initial preview will be available. CBRS is considered a lightly licenced band. This builds on AWS’s private multi-access edge compute (MEC) solution, released in conjunction with Verizon to integrate AWS Outposts with private 5G operating in licenced spectrum. A customer reference highlighted was low latency, high throughput analysis of video feeds from manufacturing robots at Corning.
Similarly, Microsoft launched a private MEC offering last year, a cloud and software stack designed for operators, systems integrators, and ISVs to deploy private 5G solutions. The system is built up of components from Azure and its acquisition of Metaswitch. AT&T is an early partner bringing a solution to the market built on Microsoft’s technology and the operator’s licenced spectrum. Microsoft highlighted use cases such as asset tracking in logistics, factory operations in manufacturing, and experiments with AI-infused video analytics to improve worker safety.
The Future
Organisations are likely to begin testing private 5G this year for Industry 4.0 applications, either at single sites in the case of factories or in select geographic areas for Utilities. Early applications will mostly focus on simple connectivity for mobile machines or remote equipment. In the longer term, however, the benefits of private 5G will become more apparent as AI applications, such as video analysis and autonomous machines become more prevalent. This will require the full ecosystem of players, including telecom providers, network vendors, cloud hyperscalers, systems integrators, and IoT providers.
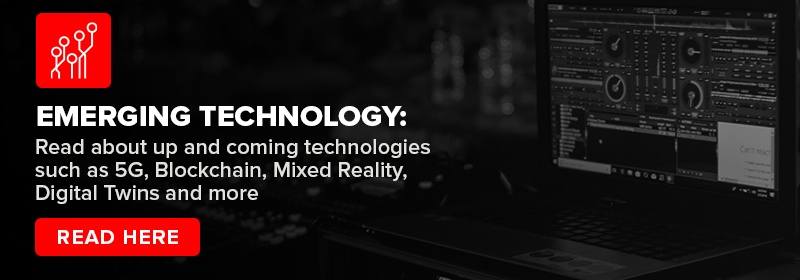
Telecom companies’ spending on utilities (electricity, fuel, and water) amounted to an estimated 5.2% of OpEx (excluding depreciation and amortisation) in 2020, a bit up from the previous three years. There is modest evidence that 5G adoption is driving costs higher. Early adopters, such as China Mobile, Ooredoo, Swisscom, Telecom Italia, and all three of Korea’s big telecom providers (SKT, KT, and LG Uplus) saw increases in utilities spend in 2020. Increases generally weren’t big but serve as a good reminder that telecom providers will need to seek out energy efficient equipment, software, and network architectures as 5G penetration grows.
Telecom providers also need to rise to the challenge of truly serving as enablers of sustainability. Rather than just viewing energy as a cost centre, they should work with customers and partners to move rapidly towards green energy and reductions in usage.
Some providers are already on this path, but not nearly enough.
At the COP26 there was a strong focus on collective action aimed at curtailing climate change trends. It is all the more important for private companies to take voluntary action. Unfortunately, they’ll only do that if they get public credit for such actions, or if the changes turn out to save money.
Telecom providers have a role to play here. They have an intimate relationship with millions of customers, and an understanding of how their behavior impacts energy usage. It’s time for them to start monetising the insights, help their customers decrease consumption, save cash and position themselves as climate friendly. Recent offerings from European telecoms such as KPN, Swisscom and Vodafone point in the right direction.
Click here to download Sustainability in the Telecom Industry as a PDF
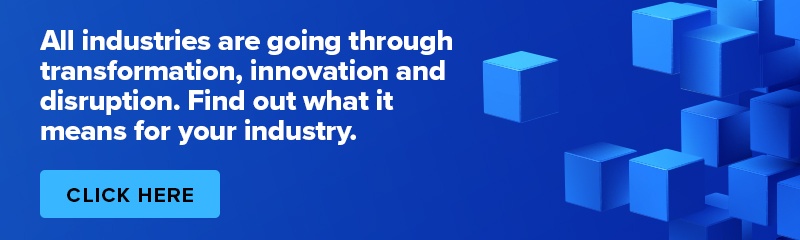
2020 was a watershed year for the industry as they proved to be the backbone for the rapid changes in work practices, communication and entertainment. This has led telecom providers to embark on their won digital transformation journeys.
The challenges continue for the industry, especially as 5G has not yet delivered on the early promises. Telecom operators today are having to provide cutting-edge services and top-notch customer experience as they continue to be challenged by new market entrants and strong regulatory pressures.
In 2022, telecom providers will be driven by the need to innovate and improve their product and service lines; improve customer experience; comply with changing regulations; and to optimise costs.
Read on to find out what Ecosystm Analysts Darian Bird and Matt Walker think will be the key trends in the telecom industry in 2022.
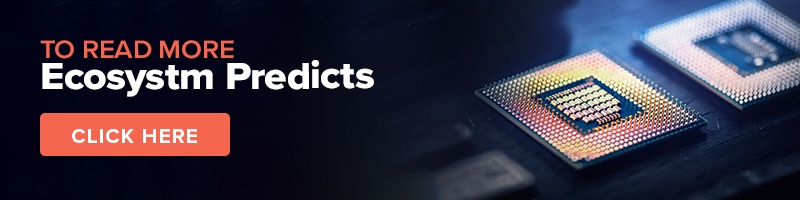
In this Insight, guest author Anupam Verma talks about how a smart combination of technologies such as IoT, edge computing and AI/machine learning can be a game changer for the Financial Services industry. “With the rise in the number of IoT devices and increasing financial access, edge computing will find its place in the sun and complement (and not compete) with cloud computing.”
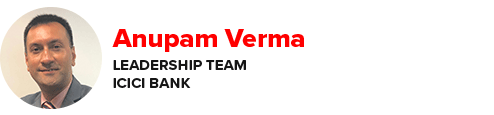
The number of IoT devices have now crossed the population of planet earth. The buzz around the Internet of Things (IoT) refuses to go down and many believe that with 5G rollouts and edge computing, the adoption will rise exponentially in the next 5 years.
The IoT is described as the network of physical objects (“things”) embedded with sensors and software to connect and exchange data with other devices over the internet. Edge computing allows IoT devices to process data near the source of generation and consumption. This could be in the device itself (e.g. sensors), or close to the device in a small data centre. Typically, edge computing is advantageous for mission-critical applications which require near real-time decision making and low latency. Other benefits include improved data security by avoiding the risk of interception of data in transfer channels, less network traffic and lower cost. Edge computing provides an alternative to sending data to a centralised cloud.
In the 5G era, a smart combination of technologies such as IoT, edge computing and AI/machine learning will be a game changer. Multiple uses cases from self-driving vehicles to remote monitoring and maintenance of machinery are being discussed. How do we see IoT and the Edge transforming Financial Services?
Before we go into how these technologies can transforming the industry, let us look at current levels of perception and adoption (Figure 1).
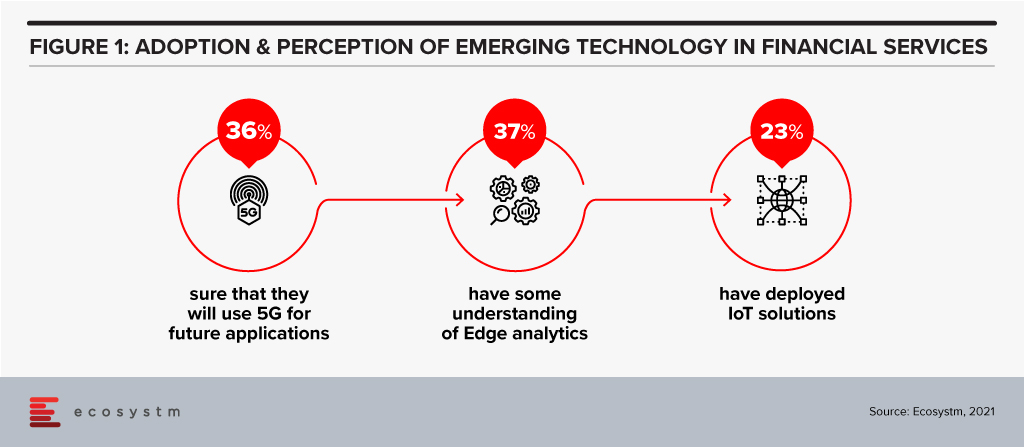
There is definitely a need for greater awareness of the capabilities and limitations of these emerging technologies in the Financial Services.
Transformation of Financial Services
The BFSI sector is increasingly moving away from selling a product to creating a seamless customer journey. Financial transactions, whether it is payment, transfer of money, or a loan can be invisible, and Edge computing will augment the customer experience. This cannot be achieved without having real-time data and analytics to create an updated 360-degree profile of the customer at all times. This data could come from multiple IoT devices, channels and partners that can interface and interact with the customer. A lot of use cases around personalisation would not be possible without edge computing. The Edge here would mean faster processing and smoother experience leading to customer delight and a higher trust quotient.
With IoT, customers can bank anywhere anytime using connected devices like wearables (smartwatches, fitness trackers etc). People can access account details, contextual offers at their current location or make payments without even needing a smartphone.

Use Cases of IoT & Edge in Financial Services
IT and Digital Leaders in Financial Services are aware of the benefits of IoT and there are some use cases that most of them think will help transform Financial Services (Figure 2).
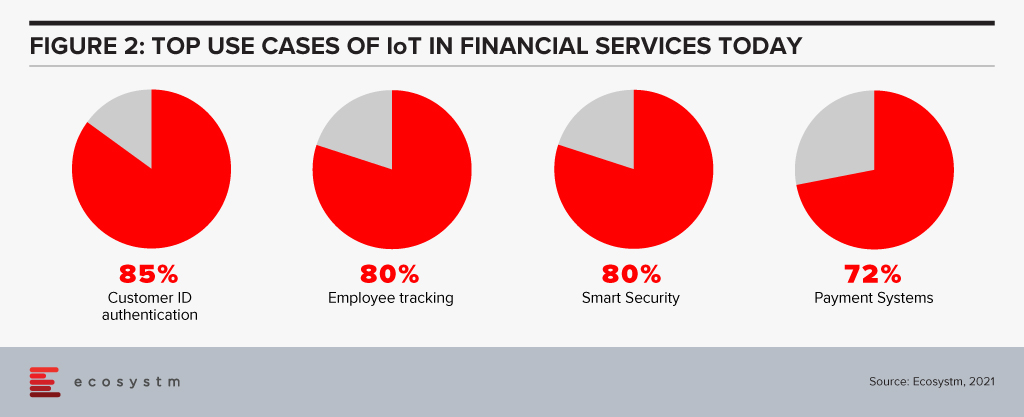
However, there are many more potential use cases. Here are some use cases whose volume will only grow every day to fuel incessant data generation, consumption and processing at the Edge.
- Smart Homes. IoT devices like Alexa/Google Home have capabilities to become “bank in a speaker” with edge computing.
- In-Sync Omnichannels. IoT devices can be synced with other banking channels. A customer may start a transaction on an IoT device and complete it in a branch. Facial recognition can be used to identify the customer after he/she walks in and synced IoT devices will ensure that the transaction is completed without any steps repeated (zero re-work) thereby enhancing customer satisfaction.
- Virtual Relationship Managers. In a digital branch, the customer may use Virtual Reality (VR) headsets to engage with virtual relationship managers and relevant experts. Gamification using VR can be amazingly effective in the area of financial literacy and financial planning.
- Home and Auto Purchase. VR may also find use in home and auto purchase processes with financing built into it. The entire customer journey will have a much smoother experience with edge computing.
- Auto and Health Insurance. Companies can use IoT (device installed in the vehicle) plus edge computing to monitor and improve driving behaviour, eventually rewarding safety with lower premiums. The growth in electric mobility will continue to provide the basis for auto insurance. Companies can use wearables to monitor crucial health parameters and exercising habits. The creation of real-time dynamic rewards around it can change behaviour towards a healthier lifestyle. Awareness, longevity, rising costs and pandemic will only fuel this sector’s growth.
- Payments. Device to device contactless payment protocol is picking up and IoT and edge computing can create next-gen revolution in payments. Your EV could have an embedded wallet and pay for its parking and toll.
- Branch/ATM. IoT sensors and CCTV footage from branches/ATMs can be utilised in real-time to improve branch productivity as well as customer engagement, at the same time enhancing security. It could also help in other situations like low cash levels in ATMs and malfunctions. Sending live video streams for video analytics to the cloud can be expensive. By processing data within the device or on-premises, the Edge can help lower costs and reduce latency.
- Trading in Securities. Another area where response time matters is algorithmic trading. Edge computing will help to quickly process and analyse a large amount of data streaming real-time from multiple feeds and react appropriately.
- Trade Finance. Real-time tracking of goods may add a different dimension to the risk, pricing and transparency of supply chains.
Cloud vs Edge
The decision to use cloud or edge will depend on multiple considerations. At the same time, all the data from IoT devices need not go to the cloud for processing and choke network bandwidth. In fact, some of this data need not be stored forever (like video feeds etc). As a result, with the rise in the number of IoT devices and increasing financial access, edge computing will find its place in the sun and complement (and not compete) with cloud computing.
The views and opinions mentioned in the article are personal.
Anupam Verma is part of the Leadership team at ICICI Bank and his responsibilities have included leading the Bank’s strategy in South East Asia to play a significant role in capturing Investment, NRI remittance, and trade flows between SEA and India.
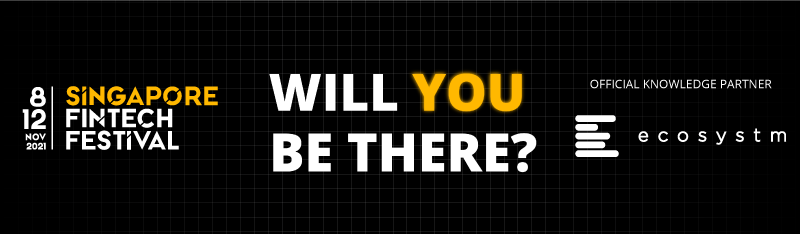
The rollout of 5G combined with edge computing in remote locations will change the way maintenance is carried out in the field. Historically, service teams performed maintenance either in a reactive fashion – fixing equipment when it broke – or using a preventative calendar-based approach. Neither of these methods is satisfactory, with the former being too late and resulting in failure while the latter is necessarily too early, resulting in excessive expenditure and downtime. The availability of connected sensors has allowed service teams to shift to condition monitoring without the need for taking equipment offline for inspections. The advent of analytics takes this approach further and has given us optimised scheduling in the form of predictive maintenance.
The next step is prescriptive maintenance in which AI can recommend action based on current and predicted condition according to expected usage or environmental circumstances. This could be as simple as alerting an operator to automatically ordering parts and scheduling multiple servicing tasks depending on forecasted production needs in the short term.
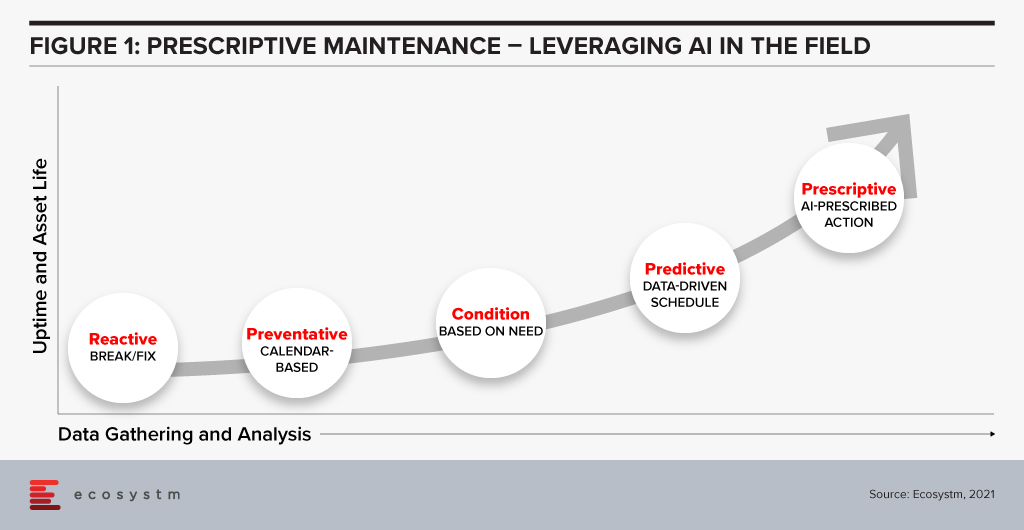
Prescriptive maintenance has only become possible with the advancement of AI and digital twin technology, but imminent improvements in connectivity and computing will take servicing to a new level. The rollout of 5G will give a boost to bandwidth, reduce latency, and increase the number of connections possible. Equipment in remote locations – such as transmission lines or machinery in resource industries – will benefit from the higher throughput of 5G connectivity, either as part of an operator’s network rollout or a private on-site deployment. Mobile machinery, particularly vehicles, which can include hundreds of sensors will no longer be required to wait until arrival before the condition can be assessed. Furthermore, vehicles equipped with external sensors can inspect stationary infrastructure as it passes by.
Edge computing – either carried out by miniature onboard devices or at smaller scale data centres embedded in 5G networks – ensure that intensive processing can be carried out closer to equipment than with a typical cloud environment. Bandwidth hungry applications, such as video and time series analysis, can be conducted with only meta data transmitted immediately and full archives uploaded with less urgency.
Prescriptive Maintenance with 5G and the Edge – Use Cases
- Transportation. Bridges built over railway lines equipped with high-speed cameras can monitor passing trains to inspect for damage. Data-intensive video analysis can be conducted on local devices for a rapid response while selected raw data can be uploaded to the cloud over 5G to improve inference models.
- Mining. Private 5G networks built-in remote sites can provide connectivity between fixed equipment, vehicles, drones, robotic dogs, workers, and remote operations centres. Autonomous haulage trucks can be monitored remotely and in the event of a breakdown, other vehicles can be automatically redirected to prevent dumping queues.
- Utilities. Emergency maintenance needs can be prioritised before extreme weather events based on meteorological forecasts and their impact on ageing parts. Machine learning can be used to understand location-specific effects of, for example, salt content in off-shore wind turbine cables. Early detection of turbine rotor cracks can recommend shutdown during high-load periods.

Data as an Asset
Effective prescriptive maintenance only becomes possible after the accumulation and integration of multiple data sources over an extended period. Inference models should understand both normal and abnormal equipment performance in various conditions, such as extreme weather, during incorrect operation, or when adjacent parts are degraded. For many smaller organisations or those deploying new equipment, the necessary volume of data will not be available without the assistance of equipment manufacturers. Moreover, even manufacturers will not have sufficient data on interaction with complementary equipment. This provides an opportunity for large operators to sell their own inference models as a new revenue stream. For example, an electrical grid operator in North America can partner with a similar, but smaller organisation in Europe to provide operational data and maintenance recommendations. Similarly, telecom providers, regional transportation providers, logistics companies, and smart cities will find industry players in other geographies that they do not naturally compete with.
Recommendations
- Employing multiple sensors. Baseline conditions and failure signatures are improved using machine learning based on feeds from multiple sensors, such as those that monitor vibration, sound, temperature, pressure, and humidity. The use of multiple sensors makes it possible to not only identify potential failure but also the reason for it and can therefore more accurately prescribe a solution to prevent an outage.
- Data assessment and integration. Prescriptive maintenance is most effective when multiple data sources are unified as inputs. Identify the location of these sources, such as ERP systems, time series on site, environmental data provided externally, or even in emails or on paper. A data fabric should be considered to ensure insights can be extracted from data no matter the environment it resides in.
- Automated action. Reduce the potential for human error or delay by automatically generating alerts and work orders for resource managers and service staff in the event of anomaly detection. Criticality measures should be adopted to help prioritise maintenance tasks and reduce alert noise.
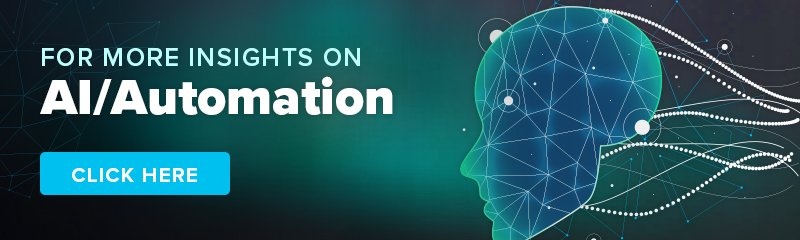
Last week I wrote about the need to remove hype from reality when it comes to AI. But what will ensure that your AI projects succeed?
It is quite obvious that success is determined by human aspects rather than technological factors. We have identified four key organisational actions that enable successful AI implementation at scale (Figure 1).
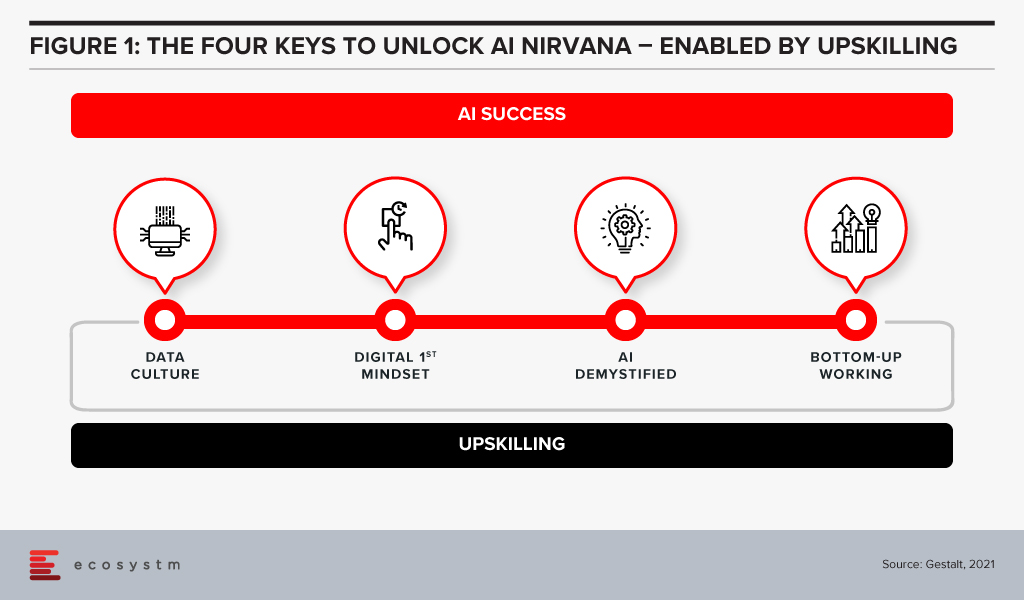
#1 Establish a Data Culture
The traditional focus for companies has been on ensuring access to good, clean data sets and the proper use of that data. Ecosystm research shows that only 28% of organisations focused on customer service, also focus on creating a data-driven organisational culture. But our experience has shown that culture is more critical than having the data. Does the organisation have a culture of using data to drive decisions? Does every level of the organisation understand and use data insights to do their day-to-day jobs? Is decision-making data-driven and decentralised, needing to be escalated only when there is ambiguity or need for strategic clarity? Do business teams push for new data sources when they are not able to get the insights they need?
Without this kind of culture, it may be possible to implement individual pieces of automation in a specific area or process, applying brute force to see it through. In order to transform the business and truly extract the power of AI, we advise organisations to build a culture of data-driven decision-making first. That organisational mindset, will make you capable implementing AI at scale. Focusing on changing the organisational culture will deliver greater returns than trying to implement piecemeal AI projects – even in the short to mid-term.
#2 Ingrain a Digital-First Mindset
Assuming a firm has passed the data culture hurdle, it needs to consider whether it has adopted a digital-first mindset. AI is one of many technologies that impact businesses, along with AR/VR, IoT, 5G, cloud and Blockchain to name a few. Today’s environment requires firms to be capable of utilising a variety of these technologies – often together – and possessing a workforce capable of using these digital tools.
A workforce with the digital-first mindset looks for a digital solution to problems wherever appropriate. They have a good understanding of digital technologies relevant to their space and understand key digital methodologies – such as Customer 360 to deliver a truly superior customer experience or Agile methodologies to successfully manage AI at scale.
AI needs business managers at the operational levels to work with IT or AI tech teams to pinpoint processes that are right for AI. They need to make an estimation based on historical data of what specific problems require an AI solution. This is enabled by the digital-first mindset.
#3 Demystify AI
The next step is to get business leaders, functional leaders, and business operational teams – not just those who work with AI – to acquire a basic understanding of AI.
They do not need to learn the intricacies of programming or how to create neural networks or anything nearly as technical in nature. However, all levels from the leadership down should have a solid understanding of what AI can do, the basics of how it works, how the process of training data results in improved outcomes and so on. They need to understand the continuous learning nature of AI solutions, getting better over time. While AI tools may recommend an answer, human insight is often needed to make a correct decision off this recommendation.

#4 Drive Implementation Bottom-Up
AI projects need alignment, objectives, strategy – and leadership and executive buy-in. But a very important aspect of an AI-driven organisation that is able to build scalable AI, is letting projects run bottom up.
As an example, a reputed Life Sciences company embarked on a multi-year AI project to improve productivity. They wanted to use NLP, Discovery, Cognitive Assist and ML to augment clinical proficiency of doctors and expected significant benefits in drug discovery and clinical trials by leveraging the immense dataset that was built over the last 20 years.
The company ran this like any other transformation project, with a central program management team taking the lead with the help of an AI Centre of Competency. These two teams developed a compelling business case, and identified initial pilots aligned with the long-term objectives of the program. However, after 18 months, they had very few tangible outcomes. Everyone including doctors, research scientists, technicians, and administrators, who participated in the program had their own interpretation of what AI was not able to do.
Discussion revealed that the doctors and researchers felt that they were training AI to replace themselves. Seeing a tool trying to mimic the same access and understanding of numerous documents baffled them at best. They were not ready to work with AI programs step-by-step to help AI tools learn and discover new insights.
At this point, we suggested approaching the project bottom-up – wherein the participating teams would decide specific projects to take up. This developed a culture where teams collaborated as well as competed with each other, to find new ways to use AI. Employees were shown a roadmap of how their jobs would be enhanced by offloading routine decisions to AI. They were shown that AI tools augment the employees’ cognitive capabilities and made them more effective.
The team working on critical trials found these tools extremely useful and were able to collaborate with other organisations specialising in similar trials. They created the metadata and used ML algorithms to discover new insights. Working bottom-up led to a very successful AI deployment.
We have seen time and again that while leadership may set the strategy and objectives, it is best to let the teams work bottom-up to come up with the projects to implement.
#5 Invest in Upskilling
The four “keys” are important to build an AI-powered, future-proof enterprise. They are all human related – and when they come together to work as a winning formula is when organisations invest in upskilling. Upskilling is the common glue and each factor requires specific kinds of upskilling (Figure 2).
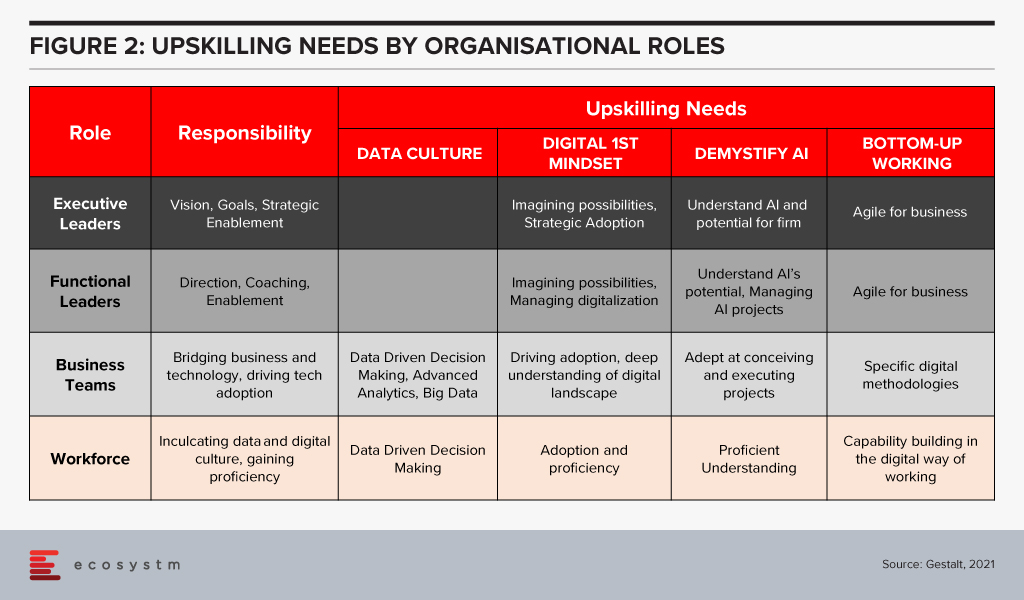
Upskilling needs vary by organisational level and the key being addressed. The bottom line is that upskilling is a universal requirement for driving AI at scale, successfully. And many organisations are realising it fast – Bosch and DBS Bank are some of the notable examples.
How much is your organisation invested in upskilling for AI implementation at scale? Share your stories in the comment box below.
Written with contributions from Ravi Pattamatta and Ratnesh Prasad
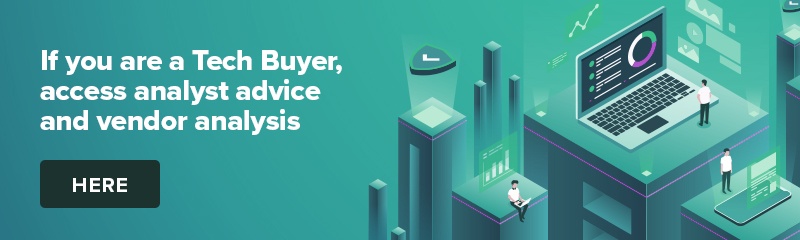
Since the start of this millennium, no region has transformed as much as Asia. There has been significant paradigm shifts in the region and the perception that innovation starts in the US or in Europe and percolates through to Asia after a time lag, has been shattered. Asia is constantly demonstrating how dynamic, and technology-focused it is. This is getting fueled by the impact of the growing middle class on consumerism and the spirit of innovation across the region. The region has also seen a surge in new and upcoming business leaders who are embracing change and looking beyond success to creating impact.
What is Driving Innovation in Asia?
The “If you ain’t got it, build it” attitude. One of the key drivers of this shift is the age of the average population in Asia. According to the UN the Asia Pacific region has nearly 60% of the world’s youth population (between the age of 17-24). With youth comes dynamism, a desire to change the world, and innovation. As this age group enters the workforce, they will transform their lives and the companies they work in. They are already showing a spirit of agility when it comes to solving challenges – they will build what they do not have.
The Need to enable Foundational Shifts. The younger generation is more aware of environmental, social and governance issues that the world continues to face. Many of the countries in the region are emerging economies, where these issues become more apparent. COVID-19 has also inculcated an empathy in people and they are thinking of future success in terms of impact. The desire to enable foundational shifts is giving direction to the transformation journey in the region. The wonderful new paradigm that is the Digital Economy allows us to cut across all segments; and technology and its advancements has immense potential to create a more sustainable and inclusive future for the world.
Realising the Power of Momentum. The pandemic has caused major disruptions in the region. But every crisis also presents an opportunity to perhaps re-imagine a brighter world through a digital lens. The other thing that the pandemic has done is made people and organisations realise that to succeed they need to be open to change – and that momentum is important. As organisations had to pivot fast, they realised what I have been saying for years – we shouldn’t “let perfect get in the way of better”. This adaptability and the readiness to fail fast and learn from the mistakes early for eventual success, is leading to faster and more agile transformation journeys.
Where are we seeing the most impact?
Industries are Transforming. There are industries such as Healthcare and Education that had to transform out of a necessity and urgency brought about by the COVID-19 pandemic. This has led to a greater impetus for change and optimism in these industries. These industries will continue to transform as governments focus significantly on creating “Social Safety Nets” and technology plays a key role in enabling critical services across Health, Education and Food Security. Then there are industries, such as the Financial Services and Retail, that had a strong customer focus and were well on their digital journeys before the pandemic. The pandemic boosted these efforts.
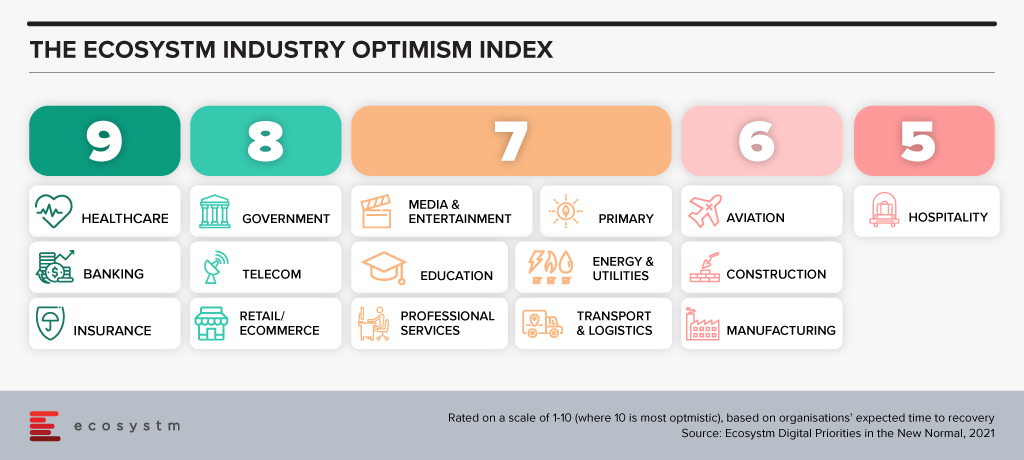
But these are not the only industries that are transforming. There are industries that have been impacted more than others. There are several instances of how organisations in these industries are demonstrating not only resilience but innovation. The Travel & Hospitality industry has had several such instances. As business models evolve the industry will see significant changes in digital channels to market, booking engines, corporate service offerings and others, as the overall Digital Strategy is overhauled.
Technologies are Evolving. Organisations depended on their tech partners to help them make the fast pivot required to survive and succeed in the last year – and tech companies have not disappointed. They have evolved their capabilities and continue to offer innovative solutions that can solve many of the ongoing business challenges that organisations face in their innovation journey. More and more technologies such as AI, machine learning, robotics, and digital twins are getting enmeshed together to offer better options for business growth, process efficiency and customer engagement. And the 5G rollouts will only accelerate that. The initial benefits being realized from early adoption of 5G has been for consumers. But there is a much bigger impact that is waiting to be realised as 5G empowers governments and businesses to make critical decisions at the edge.
Tech Start-ups are Flourishing. There are immense opportunities for technology start-ups to grow their market presence through innovative products and services. To succeed these companies need to have a strong investment roadmap; maintain a strong focus on customer engagement; and offer technology solutions that can fulfil the global needs of their customers. Technologies that promote efficiency and eliminate mundane tasks for humans are the need of the hour. However, as the reliance on technology-led transformation increases, tech vendors are becoming acutely aware that they cannot be best-in-class across the different technologies that an organisation will require to transform. Here is where having a robust partner ecosystem helps. Partnerships are bringing innovation to scale in Asia.
We can expect Asia to emerge as a powerhouse as businesses continue to innovate, embed technology in their product and service offerings – and as tech start-ups continue to support their innovation journeys.
Ecosystm CEO Amit Gupta gets face to face with Garrett Ilg, President Asia Pacific & Japan, Oracle to discuss the rise of the Asia Digital economies, the impact of the growing middle class on consumerism and the spirit of innovation across the region.
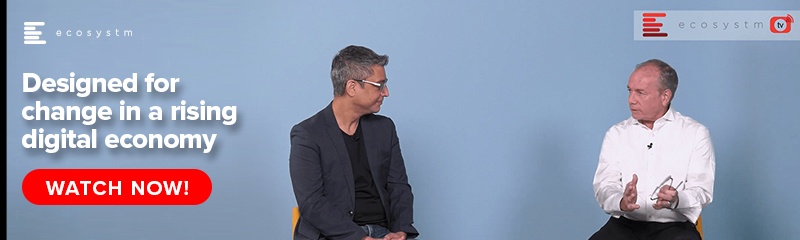